- Reporting Bias: Definition, Types, Examples & Mitigation

Reporting bias is a type of selection bias that occurs when only certain observations are reported or published. Reporting bias can greatly impact the accuracy of results, and it is important to consider reporting bias when conducting research. In this article, we will discuss reporting bias, the types, and the examples.
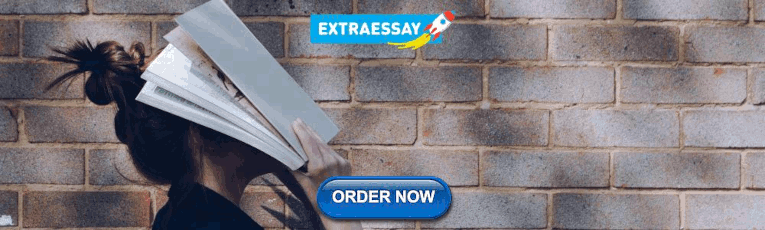
What is Reporting Bias?
Reporting bias is a type of selection bias that occurs when the results of a study are skewed due to the way they are reported. Reporting bias happens when researchers or scientists choose to report only certain data, even though other data may exist that would have influenced their findings.
For example, if you were to conduct a study on the effects of eating chocolate on mice and only used mice from a particular region, then your results could be skewed because they would not be representative of all mice. Reporting bias can also occur when the data is manipulated before it’s reported, as in the case of cherry-picking or data mining.
This can result in unreliable or biased results reported by an organization or individual. The most common form of reporting bias is selection bias. This is when participants in a study are chosen based on their ability to influence the outcome or for other reasons that might create an inaccurate picture of reality.
Read: What is Participant Bias? How to Detect & Avoid It
Another reporting bias occurs when researchers do not report all the results of their studies. They may leave out information because they don’t think it’s important or because they want to make their findings seem more impressive than they are.
This is why there’s a field of science dedicated to trying to determine what happened in studies where there was reporting bias: a meta-analysis. A meta-analysis is an analysis of multiple studies on the same topic done by different researchers, which can help provide more insight into whether a particular finding is factual or not.
Read: Selection Bias in Research: Types, Examples & Impact
Types of Reporting Bias
1. outcome reporting bias.
Outcome reporting bias occurs when an outcome that was not originally planned for (or expected) is reported in favor of the hypothesis being tested. This can be due to either a conscious or unconscious decision made by the researcher.
For example, suppose you wanted to test whether eating more vegetables improves health outcomes. If you find that people who ate more vegetables were healthier than those who didn’t and then decide that this means eating vegetables improves health, you may have fallen victim to outcome reporting bias because it was never your intention to study how vegetables affect health outcomes.
2. Publication bias
Publication bias is another form of reporting bias where journals or other publications only print positive results from studies conducted on their topic(s). This means that only positive results are published, leading readers to believe that there’s no need for further research on the topic because all of the relevant evidence has already been collected. This can also lead to the “file drawer” phenomenon where negative results are not published because they will not contribute positively to the researcher’s reputation or career advancement.
Read: 21 Chrome Extensions for Academic Researchers in 2022
3. Knowledge reporting bias
Knowledge reporting bias refers to the fact that researchers may not report all their knowledge about a topic or experiment because they feel it isn’t important enough or doesn’t fit their hypothesis.
Here’s an example: let’s say two researchers are studying whether people feel healthier if they eat vegetables every day versus if they eat vegetables once or twice per week and one researcher finds no difference between eating vegetables daily vs. once per week, but the other did find a health improvement when people ate veggies daily versus once per week. The first researcher might decide not to report this finding and then prevent the readers from knowing that possibility.
4. Multiple (duplicate) publication bias
Multiple publication bias is when a study is published repeatedly either due to changes in methodology or because the same data is analyzed differently by different researchers. This can lead to false conclusions about the effectiveness of a treatment or program because it skews the results towards positive outcomes.
5. Time lag bias
Time lag bias occurs when a study is conducted with no follow-up measures, so it doesn’t get published until much later when someone revisits the topic and does another study on it (with follow-up data). When this happens, researchers are unable to determine whether or not there has been any change over time due to intervention or other factors because they don’t have any data from before intervention began.
6. Citation bias
Citation bias occurs when one researcher cites another researcher’s work as evidence for their argument without acknowledging that it was cited from someone else’s paper first; essentially making it look like they came up with an idea on their own instead of building off someone else’s work.
Explore: Citation Styles in Research Writing: MLA, APA, IEEE
Examples of Reporting Bias
For example, if you were studying how many people with blue eyes wear glasses and found that only 40% of people with blue eyes wear glasses, then it could be because they didn’t include all the people with blue eyes in your study. It could also be because there are other factors at play that make fewer people with blue eyes wear glasses such as those who don’t wear glasses may not feel like wearing them.
Reporting bias can also happen when someone running a survey or experiment asks leading questions. For example, instead of asking “Do you like eating bananas?” they might say “Bananas are delicious,” or instead of asking “Have you ever eaten bananas?” they might say “Wouldn’t it be nice if we could eat bananas every day?” The first question does not affect the outcome, but if someone answers yes to the second question, it is because they think they have to agree with the pollster’s statement.
Read: Undercoverage Bias: Definition, Examples in Survey Research
If you ask people to rate their performance as a manager on a scale from 1 to 5, and then you ask them about their coworkers’ performance, they might tell you that all their coworkers are doing great (because they don’t want to look bad). This is an example of reporting bias because it skews the results of your study by making it seem like everyone is performing well when in reality, it may be that some people are doing poorly.
Effects and Implications of Reporting Bias
Reporting bias can lead to false conclusions being drawn from experiments and may even lead to harm for patients or subjects involved in a study. For example, if a researcher does not report all of their data, it could lead them to think that their treatment works better than it does.
This would result in them prescribing this treatment to patients who don’t need it or causing other researchers to replicate their experiment with incorrect results. When a study sample is selected based on reporting, the results are likely to be biased in favor of positive results: if people are more likely to report positive events than negative events, then they’ll also be more likely to not report at all.
Reporting bias can have serious implications for your survey and your business. If you’re trying to figure out whether your product/service is effective at solving problems for customers (or not), then reporting bias can make it hard for you to get an accurate lead on how well it works across different demographics and situations.
Read: Survey Errors To Avoid: Types, Sources, Examples, Mitigation
How to Prevent or Manage Reporting Bias
- Be honest with yourself about whether or not your results are statistically significant enough to be considered valid. If they are not, then don’t pretend they are.
- You can also try running some more experiments before publishing your findings so that there’s more evidence available for readers who might want more proof before believing what was found during your experiment.
- Consider using statistics or data to back up your claims whenever possible.
- You can also manage it by collecting and reporting data from additional sources to provide an alternative view of the data. This can be done by asking colleagues who have not been involved in the project to review the results for plausibility, or by running a second analysis that uses more conservative assumptions.
Read: Research Bias: Definition, Types + Examples
Reporting bias is a phenomenon in which the reporting of a study is biased by the researcher’s expectations of what they want to find and it can be caused by many factors. You must practice transparency in your research as that would help you better manage reporting bias.
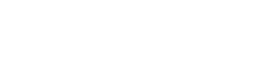
Connect to Formplus, Get Started Now - It's Free!
- citation bias
- knowledge reporting bias
- outcome-reporting bias
- publication bias
- recall bias
- recall limitation
- reporting tools
- time lag bias
- busayo.longe
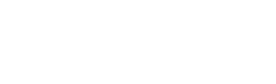
You may also like:
Projective Techniques In Surveys: Definition, Types & Pros & Cons
Introduction When you’re conducting a survey, you need to find out what people think about things. But how do you get an accurate and...

Recall Bias: Definition, Types, Examples & Mitigation
This article will discuss the impact of recall bias in studies and the best ways to avoid them during research.
Selection Bias in Research: Types, Examples & Impact
In this article, we’ll discuss the effects of selection bias, how it works, its common effects and the best ways to minimize it.
What is Publication Bias? (How to Detect & Avoid It)
In this article, we will do a deep dive into publication bias, how to reduce or avoid it, and other types of biases in research.
Formplus - For Seamless Data Collection
Collect data the right way with a versatile data collection tool. try formplus and transform your work productivity today..
Catalogue of Bias
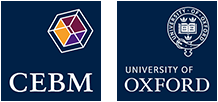
Reporting biases
A systematic distortion that arises from the selective disclosure or withholding of information by parties involved in the design, conduct, analysis, or dissemination of a study or research findings
Table of Contents
Preventive steps.
- Further resources
Reporting biases is an umbrella term that covers a range of different types of biases. It is described as the most significant form of scientific misconduct ( Al-Marzouki et al. 2005 ). Reporting biases have been recognised for hundreds of years, dating back to the 17th century ( Dickersin & Chambers, 2010 ). Since then, various definitions of reporting biases have been proposed:
- The Dictionary of Epidemiology defines reporting bias as the “s elective revelation or suppression of information (e.g., about past medical history, smoking, sexual experiences) or of study results .”
- The Cochrane Handbook states it arises “ when the dissemination of research findings is influenced by the nature and direction of results .”
- The James Lind Library states “ biased reporting of research occurs when the direction or statistical significance of results influence whether and how research is reported. ”
Our definition of reporting biases is a distortion of presented information from research due to the selective disclosure or withholding of information by parties involved with regards to the topic selected for study and the design, conduct, analysis, or dissemination of study methods, findings or both. Researchers have previously described seven types of reporting biases, including publication bias , time-lag bias, multiple (duplicate) publication bias, location bias, citation bias, language bias and outcome reporting bias ( Higgins & Green. 2011 ). Figure 1 illustrates where reporting biases can occur in the lifecycle of research and provides several examples of reporting biases.
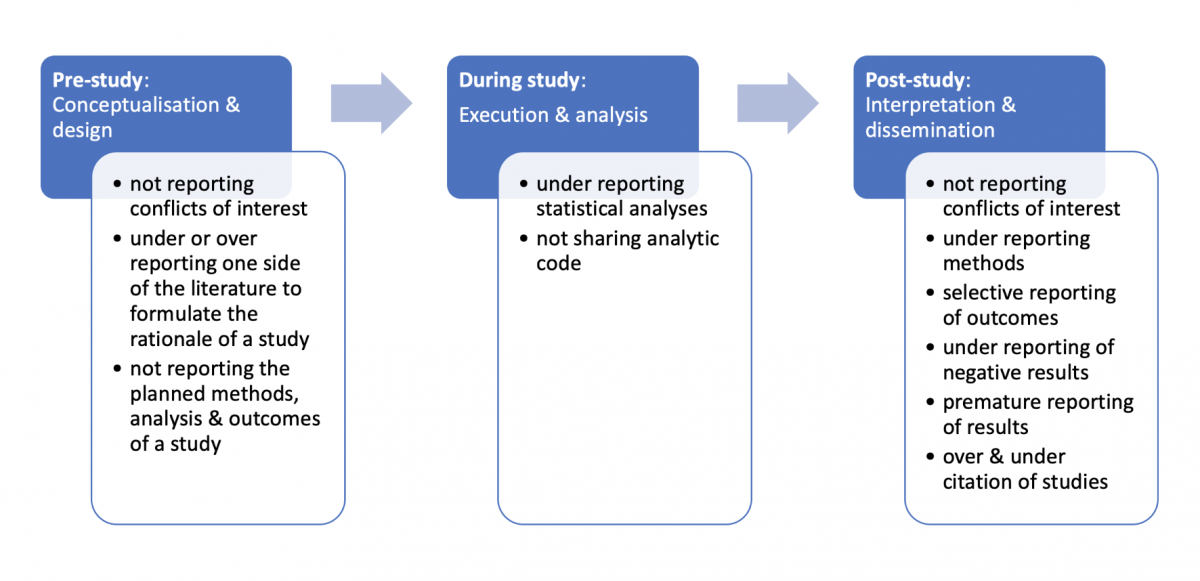
Download the powerpoint: Reporting biases
A narrative review conducted by McGauran and colleagues (2010) found reporting biases are a widespread phenomenon in the medical literature. They identified reporting biases in 50 types of pharmacological, surgical, diagnostic and preventative interventions which included the withholding of study data or the active attempt by manufacturers to suppress the publication of findings.
A systematic review by Jones and colleagues (2015) compared the outcomes of randomised controlled trials specified in registered protocols with those in subsequent peer-reviewed journal articles. There were discrepancies between prespecified and reported outcomes in a third of the studies. Thirteen per cent of trials introduced a new outcome in the published articles compared with those specified in the registered protocols.
In a cohort study of Cochrane systematic reviews, Saini and colleagues (2014) found 86% of reviews did not report data on the main harm outcome of interest.
Another cohort study found considerable inconsistency in the reporting of adverse events when comparing sponsors databases with study protocols ( Scharf & Colevas, 2006 ). In 14 of the 22 included studies, the number of adverse events in the sponsor’s database differed from the published articles by 20% or more.
When more detailed information for interventions was analysed for oseltamivir trials , over half (55%) of the previous risk of bias assessments were reclassified from ‘low’ risk of bias to ‘high’ ( Jefferson et al. 2014 ).
Trials and systematic reviews are used by clinicians and policymakers to develop evidence-based guidelines and make decisions about treatment or prevention of health problems. When the evidence base available to clinicians, policymakers or patients is incomplete or distorted, healthcare decisions and recommendations are made on biased evidence.
Vioxx (Rofecoxib), a Cox-2 inhibitor prescribed for osteoarthritis pain, provides an important example of under-reporting and misreporting of data which led to significant patient harm.
The first safety analysis of the largest study of Rofecoxib found a 79% greater risk of death or serious cardiovascular event in one treatment group compared with the other ( Krumholz et al., 2007 ). This information was not disclosed by the manufacturer (Merck), and the trial continued. The cardiovascular risk associated with Rofecoxib was obscured in several ways.
A number of significant conflicts of interest among board members of Merck were undisclosed, and not made public while the trial was in progress or when it was published ( Krumholz et al., 2007 ). Merck now faces legal claims from nearly 30,000 people who experienced adverse cardiovascular events while taking Rofecoxib.
If benefits are over-reported and harms are under-reported, clinicians, patients and the public will have a false sense of security about the safety of treatments. This results in unnecessary suffering and death ( Cowley et al. 1993 ), perpetuates research waste and misguides future research ( Glasziou & Chalmers, 2018 ).
Transparency is the most important action to safeguard health research.
Pre-study: The results of prospectively registered trials are significantly more likely to be published than those of unregistered trials (adjusted OR 4.53, 95% CI 1.12-18.34; Chan et al., 2017 ). Prospective registration of all clinical trials should be required, and encouraged for other study designs, by journal editors, regulators, research ethics committees, funders, and sponsors.
During the study: Open science practices, such as making de-identified data and analytical code publicly available through platforms like GitHub or the Open Science Framework aids reproducibility, prevents duplication, reduces waste, accelerates innovation, identifies errors and prevents reporting biases.
Post-study: Reporting guidelines such as CONSORT can help guide researchers to improve their reporting of randomised trials ( Moher et al., 2010 ).
Other checklists and tools have been developed to assess the risk of reporting biases in studies, including the Cochrane Risk of Bias Tool , GRADE and ORBIT-II ( Page et al., 2018 ).
Catalogue of Bias. Richards GC, Onakpoya IJ. Reporting biases. In: Catalog Of Bias 2019: www.catalogueofbiases.org/reportingbiases
Related biases
- Outcome reporting bias
- Publication bias
- Selection bias
Al-Marzouki et al. The effect of scientific misconduct on the results of clinical trials: a Delphi survey . Contemp Clin Trials. 2005. 26: 331-337.
Dickersin & Chalmers. Recognising, investigating and dealing with incomplete and biased reporting of clinical research: from Francis Bacon to the World Health Organisation . JLL Bulletin: Commentaries on the history of treatment evaluation. 2010.
Higgins & Green . Definitions of some types of reporting biases. Cochrane Handbook for Systematic Reviews of Interventions, v5.1.0 . 2011.
McGauran et al. Reporting bias in medical research – a narrative review . Trials 2010. 11:37.
Jones et al. Comparison of registered and published outcomes in randomized controlled trials: a systematic review . BMC Med. 2015; 13:282.
Saini et al. Selective reporting bias of harm outcomes within studies: findings from a cohort of systematic reviews . BMJ 2014; 349
Scharf & Colevas. Adverse event reporting in publications compared with sponsor database for cancer clinical trials . J Clin Oncol 2006. 24:24 pp 3933-8.
Jefferson et al. Risk of bias in industry-funded oseltamivir trials: comparison of core reports versus full clinical study reports . BMJ Open 2014;4:e005253.
Krumholz et al. What have we learnt from Vioxx? BMJ, 2007. 334(7585): 120-123
Cowley et al. The effect of lorcainide on arrhythmias and survival in patients with acute myocardial infarction: an example of publication bias . Int J Cardiol 1993. 1;40(2): 161-6.
Glasziou & Chalmers. Research waste is still a scandal . The BMJ 2018; 363:k4645.
Chan et al. Association of trial registration with reporting of primary outcomes in protocols and publications . JAMA 2017; 318:17, 1709-1711.
Moher et al. CONSORT 2010 Explanation and Elaboration: updated guidelines for reporting parallel group randomised trials . 2010. 340:c869
PubMed feed
- Ali G Alghamdi. Assessing Medical Student Perceptions of Open-Book Exams for Self-Directed Learning
- Carolina Quintero Arias. Food insecurity in high-risk rural communities before and during the COVID-19 pandemic
- Thi-Phuong-Thao Pham. Comparative efficacy of antioxidant therapies for sepsis and septic shock in the intensive care unit: A frequentist network meta-analysis
- Jie He. miR-29 as diagnostic biomarkers for tuberculosis: a systematic review and meta-analysis
- Domonkos File. The imbalance of self-reported wanting and liking is associated with the degree of attentional bias toward smoking-related stimuli in low nicotine dependence smokers
View more →
Leave a Reply Cancel reply
Your email address will not be published. Required fields are marked *

Research Bias 101: What You Need To Know
By: Derek Jansen (MBA) | Expert Reviewed By: Dr Eunice Rautenbach | September 2022
If you’re new to academic research, research bias (also sometimes called researcher bias) is one of the many things you need to understand to avoid compromising your study. If you’re not careful, research bias can ruin the credibility of your study.
In this post, we’ll unpack the thorny topic of research bias. We’ll explain what it is , look at some common types of research bias and share some tips to help you minimise the potential sources of bias in your research.
Overview: Research Bias 101
- What is research bias (or researcher bias)?
- Bias #1 – Selection bias
- Bias #2 – Analysis bias
- Bias #3 – Procedural (admin) bias
So, what is research bias?
Well, simply put, research bias is when the researcher – that’s you – intentionally or unintentionally skews the process of a systematic inquiry , which then of course skews the outcomes of the study . In other words, research bias is what happens when you affect the results of your research by influencing how you arrive at them.
For example, if you planned to research the effects of remote working arrangements across all levels of an organisation, but your sample consisted mostly of management-level respondents , you’d run into a form of research bias. In this case, excluding input from lower-level staff (in other words, not getting input from all levels of staff) means that the results of the study would be ‘biased’ in favour of a certain perspective – that of management.
Of course, if your research aims and research questions were only interested in the perspectives of managers, this sampling approach wouldn’t be a problem – but that’s not the case here, as there’s a misalignment between the research aims and the sample .
Now, it’s important to remember that research bias isn’t always deliberate or intended. Quite often, it’s just the result of a poorly designed study, or practical challenges in terms of getting a well-rounded, suitable sample. While perfect objectivity is the ideal, some level of bias is generally unavoidable when you’re undertaking a study. That said, as a savvy researcher, it’s your job to reduce potential sources of research bias as much as possible.
To minimize potential bias, you first need to know what to look for . So, next up, we’ll unpack three common types of research bias we see at Grad Coach when reviewing students’ projects . These include selection bias , analysis bias , and procedural bias . Keep in mind that there are many different forms of bias that can creep into your research, so don’t take this as a comprehensive list – it’s just a useful starting point.
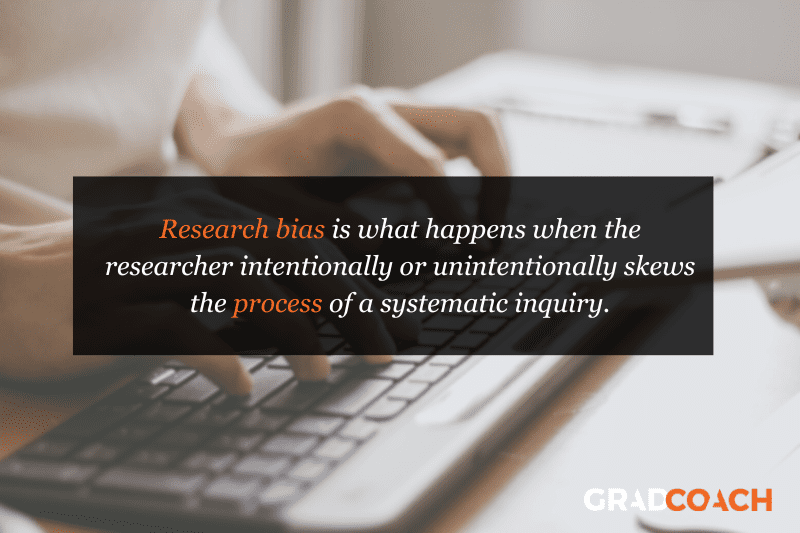
Bias #1 – Selection Bias
First up, we have selection bias . The example we looked at earlier (about only surveying management as opposed to all levels of employees) is a prime example of this type of research bias. In other words, selection bias occurs when your study’s design automatically excludes a relevant group from the research process and, therefore, negatively impacts the quality of the results.
With selection bias, the results of your study will be biased towards the group that it includes or favours, meaning that you’re likely to arrive at prejudiced results . For example, research into government policies that only includes participants who voted for a specific party is going to produce skewed results, as the views of those who voted for other parties will be excluded.
Selection bias commonly occurs in quantitative research , as the sampling strategy adopted can have a major impact on the statistical results . That said, selection bias does of course also come up in qualitative research as there’s still plenty room for skewed samples. So, it’s important to pay close attention to the makeup of your sample and make sure that you adopt a sampling strategy that aligns with your research aims. Of course, you’ll seldom achieve a perfect sample, and that okay. But, you need to be aware of how your sample may be skewed and factor this into your thinking when you analyse the resultant data.
Need a helping hand?
Bias #2 – Analysis Bias
Next up, we have analysis bias . Analysis bias occurs when the analysis itself emphasises or discounts certain data points , so as to favour a particular result (often the researcher’s own expected result or hypothesis). In other words, analysis bias happens when you prioritise the presentation of data that supports a certain idea or hypothesis , rather than presenting all the data indiscriminately .
For example, if your study was looking into consumer perceptions of a specific product, you might present more analysis of data that reflects positive sentiment toward the product, and give less real estate to the analysis that reflects negative sentiment. In other words, you’d cherry-pick the data that suits your desired outcomes and as a result, you’d create a bias in terms of the information conveyed by the study.
Although this kind of bias is common in quantitative research, it can just as easily occur in qualitative studies, given the amount of interpretive power the researcher has. This may not be intentional or even noticed by the researcher, given the inherent subjectivity in qualitative research. As humans, we naturally search for and interpret information in a way that confirms or supports our prior beliefs or values (in psychology, this is called “confirmation bias”). So, don’t make the mistake of thinking that analysis bias is always intentional and you don’t need to worry about it because you’re an honest researcher – it can creep up on anyone .
To reduce the risk of analysis bias, a good starting point is to determine your data analysis strategy in as much detail as possible, before you collect your data . In other words, decide, in advance, how you’ll prepare the data, which analysis method you’ll use, and be aware of how different analysis methods can favour different types of data. Also, take the time to reflect on your own pre-conceived notions and expectations regarding the analysis outcomes (in other words, what do you expect to find in the data), so that you’re fully aware of the potential influence you may have on the analysis – and therefore, hopefully, can minimize it.
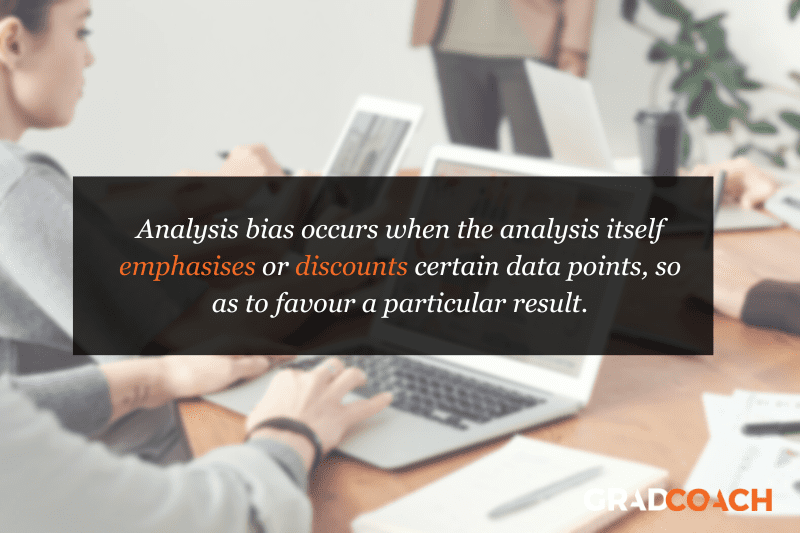
Bias #3 – Procedural Bias
Last but definitely not least, we have procedural bias , which is also sometimes referred to as administration bias . Procedural bias is easy to overlook, so it’s important to understand what it is and how to avoid it. This type of bias occurs when the administration of the study, especially the data collection aspect, has an impact on either who responds or how they respond.
A practical example of procedural bias would be when participants in a study are required to provide information under some form of constraint. For example, participants might be given insufficient time to complete a survey, resulting in incomplete or hastily-filled out forms that don’t necessarily reflect how they really feel. This can happen really easily, if, for example, you innocently ask your participants to fill out a survey during their lunch break.
Another form of procedural bias can happen when you improperly incentivise participation in a study. For example, offering a reward for completing a survey or interview might incline participants to provide false or inaccurate information just to get through the process as fast as possible and collect their reward. It could also potentially attract a particular type of respondent (a freebie seeker), resulting in a skewed sample that doesn’t really reflect your demographic of interest.
The format of your data collection method can also potentially contribute to procedural bias. If, for example, you decide to host your survey or interviews online, this could unintentionally exclude people who are not particularly tech-savvy, don’t have a suitable device or just don’t have a reliable internet connection. On the flip side, some people might find in-person interviews a bit intimidating (compared to online ones, at least), or they might find the physical environment in which they’re interviewed to be uncomfortable or awkward (maybe the boss is peering into the meeting room, for example). Either way, these factors all result in less useful data.
Although procedural bias is more common in qualitative research, it can come up in any form of fieldwork where you’re actively collecting data from study participants. So, it’s important to consider how your data is being collected and how this might impact respondents. Simply put, you need to take the respondent’s viewpoint and think about the challenges they might face, no matter how small or trivial these might seem. So, it’s always a good idea to have an informal discussion with a handful of potential respondents before you start collecting data and ask for their input regarding your proposed plan upfront.
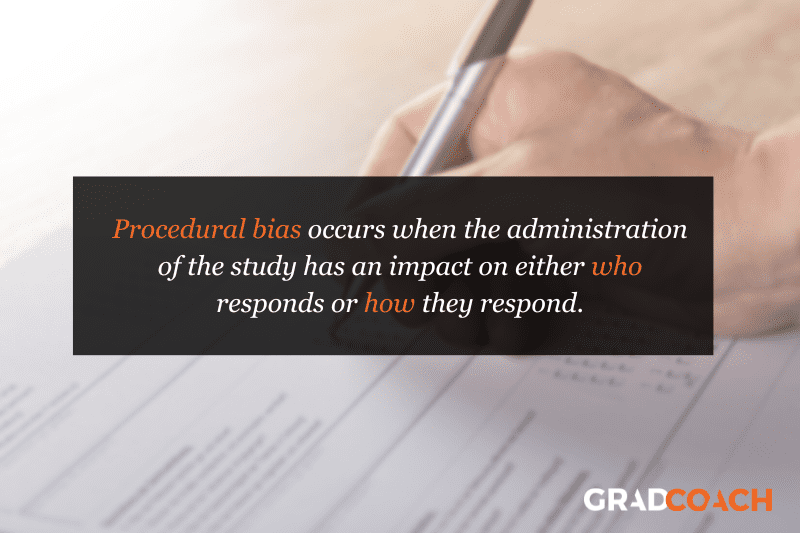
Let’s Recap
Ok, so let’s do a quick recap. Research bias refers to any instance where the researcher, or the research design , negatively influences the quality of a study’s results, whether intentionally or not.
The three common types of research bias we looked at are:
- Selection bias – where a skewed sample leads to skewed results
- Analysis bias – where the analysis method and/or approach leads to biased results – and,
- Procedural bias – where the administration of the study, especially the data collection aspect, has an impact on who responds and how they respond.
As I mentioned, there are many other forms of research bias, but we can only cover a handful here. So, be sure to familiarise yourself with as many potential sources of bias as possible to minimise the risk of research bias in your study.
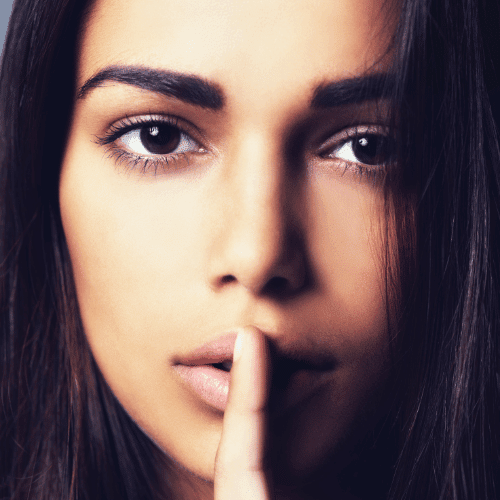
Psst... there’s more!
This post was based on one of our popular Research Bootcamps . If you're working on a research project, you'll definitely want to check this out ...
You Might Also Like:
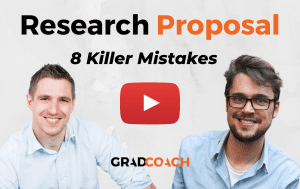
This is really educational and I really like the simplicity of the language in here, but i would like to know if there is also some guidance in regard to the problem statement and what it constitutes.
Do you have a blog or video that differentiates research assumptions, research propositions and research hypothesis?
Submit a Comment Cancel reply
Your email address will not be published. Required fields are marked *
Save my name, email, and website in this browser for the next time I comment.
- Print Friendly
Reporting Biases
- Reference work entry
- First Online: 20 July 2022
- pp 2045–2071
- Cite this reference work entry
- S. Swaroop Vedula 3 ,
- Asbjørn Hróbjartsson 4 &
- Matthew J. Page 5
188 Accesses
Clinical trials are experiments in human beings. Findings from these experiments, either by themselves or within research syntheses, are often meant to evidence-based clinical decision-making. These decisions can be misled when clinical trials are reported in a biased manner. For clinical trials to inform healthcare decisions without bias, their reporting should be complete, timely, transparent, and accessible. Reporting of clinical trials is biased when it is influenced by the nature and direction of its results. Reporting biases in clinical trials may manifest in different ways, including results not being reported at all, reported in part, with delay, or in sources of scientific literature that are harder to access. Biased reporting of clinical trials in turn can introduce bias into research syntheses, with the eventual consequence being misinformed healthcare decisions. Clinical trial registration, access to protocols and statistical analysis plans, and guidelines for transparent and complete reporting are critical to prevent reporting biases.
This is a preview of subscription content, log in via an institution to check access.
Access this chapter
- Available as PDF
- Read on any device
- Instant download
- Own it forever
- Available as EPUB and PDF
- Durable hardcover edition
- Dispatched in 3 to 5 business days
- Free shipping worldwide - see info
Tax calculation will be finalised at checkout
Purchases are for personal use only
Institutional subscriptions
Abraha I, Cherubini A, Cozzolino F, De Florio R, Luchetta ML, Rimland JM, Folletti I, Marchesi M, Germani A, Orso M, Eusebi P, Montedori A (2015) Deviation from intention to treat analysis in randomised trials and treatment effect estimates: meta-epidemiological study. BMJ 350:h2445
Article Google Scholar
Barbour V, Burch D, Godlee F, Heneghan C, Lehman R, Perera R et al (2016) Characterisation of trials where marketing purposes have been influential in study design: a descriptive study. Trials 17:31
Begg CB (1985) A measure to aid in the interpretation of published clinical trials. Stat Med 4(1):1–9
Article MathSciNet Google Scholar
Bekelman JE, Li Y, Gross CP (2003) Scope and impact of financial conflicts of interest in biomedical research: a systematic review. JAMA 289(4):454–465
Bero L (2017) Addressing bias and conflict of interest among biomedical researchers. JAMA 317(17):1723–1724
Bombardier C, Laine L, Reicin A, Shapiro D, Burgos-Vargas R, Davis B et al (2000) Comparison of upper gastrointestinal toxicity of Rofecoxib and Naproxen in patients with rheumatoid arthritis. N Engl J Med 343(21):1520–1528
Boutron I, Ravaud P (2018) Misrepresentation and distortion of research in biomedical literature. Proc Natl Acad Sci 115(11):2613–2619
Boutron I, Dutton S, Ravaud P, Altman DG (2010) Reporting and interpretation of randomized controlled trials with statistically nonsignificant results for primary outcomes. JAMA 303(20):2058–2064
Boutron I, Altman DG, Hopewell S, Vera-Badillo F, Tannock I, Ravaud P (2014) Impact of spin in the abstracts of articles reporting results of randomized controlled trials in the field of cancer: the SPIIN randomized controlled trial. J Clin Oncol 32(36):4120–4126
Boutron I, Page MJ, Higgins JPT, Altman DG, Lundh A, Hróbjartsson A (2019) Chapter 7: considering bias and conflicts of interest among the included studies. In: Higgins JPT, Thomas J, Chandler J, Cumpston M, Li T, Page MJ, Welch VA (eds) Cochrane handbook for systematic reviews of interventions. Version 6.0 (updated July 2019). Available from www.training.cochrane.org/handbook. Cochrane
Brassington I (2017) The ethics of reporting all the results of clinical trials. Br Med Bull 121(1):19–29
Cardiac Arrhythmia Suppression Trial (CAST) Investigators (1989) Preliminary report: effect of encainide and flecainide on mortality in a randomized trial of arrhythmia suppression after myocardial infarction. N Engl J Med 321(6):406–412
Chan AW, Hróbjartsson A (2018) Promoting public access to clinical trial protocols: challenges and recommendations. Trials 19(1):116
Chan AW, Hróbjartsson A, Haahr MT, Gøtzsche PC, Altman DG (2004a) Empirical evidence for selective reporting of outcomes in randomized trials: comparison of protocols to published articles. JAMA 291(20):2457–2465
Chan AW, Krleza-Jerić K, Schmid I, Altman DG (2004b) Outcome reporting bias in randomized trials funded by the Canadian Institutes of Health Research. CMAJ 171(7):735–740
Chan AW, Hróbjartsson A, Jørgensen KJ, Gøtzsche PC, Altman DG (2008) Discrepancies in sample size calculations and data analyses reported in randomised trials: comparison of publications with protocols. BMJ 337:a2299
Chan A-W, Tetzlaff JM, Altman DG, Laupacis A, Gøtzsche PC, Krleža-Jerić K et al (2013) SPIRIT 2013 statement: defining standard protocol items for clinical trials. Ann Intern Med 158(3):200–207
Chan A-W, Song F, Vickers A, Jefferson T, Dickersin K, Gøtzsche PC et al (2014) Increasing value and reducing waste: addressing inaccessible research. Lancet 383(9913):257–266
Chiu K, Grundy Q, Bero L (2017) ‘Spin’ in published biomedical literature: a methodological systematic review. PLoS Biol 15(9):e2002173
Cowley AJ, Skene A, Stainer K, Hampton JR (1993) The effect of lorcainide on arrhythmias and survival in patients with acute myocardial infarction: an example of publication bias. Int J Cardiol 40(2):161–166
Cronin E, Sheldon T (2004) Factors influencing the publication of health research. Int J Technol Assess Health Care 20(3):351–355
de Vries YA, Roest AM, Turner EH, de Jonge P (2019) Hiding negative trials by pooling them: a secondary analysis of pooled-trials publication bias in FDA-registered antidepressant trials. Psychol Med 49(12):2020–2026
Dechartres A, Atal I, Riveros C, Meerpohl J, Ravaud P (2018) Association between publication characteristics and treatment effect estimates: a meta-epidemiologic study. Ann Intern Med 169:385–393
Dickersin K (1990) The existence of publication bias and risk factors for its occurrence. JAMA 263(10):1385–1389
Dickersin K, Chalmers I (2011) Recognizing, investigating and dealing with incomplete and biased reporting of clinical research: from Francis Bacon to the WHO. J R Soc Med 104(12):532–538
Dickersin K, Min YI (1993) NIH clinical trials and publication bias. Online J Curr Clin Trials Doc No 50
Google Scholar
Dickersin K, Chan S, Chalmers TC, Sacks HS, Smith H (1987) Publication bias and clinical trials. Control Clin Trials 8(4):343–353
Dickersin K, Min Y-I, Meinert CL (1992) Factors influencing publication of research results: follow-up of applications submitted to two Institutional Review Boards. JAMA 267(3):374–378
Duyx B, Urlings MJE, Swaen GMH, Bouter LM, Zeegers MP (2017) Scientific citations favor positive results: a systematic review and meta-analysis. J Clin Epidemiol 88:92–101
Dwan K, Gamble C, Williamson PR, Kirkham JJ (2013) Systematic review of the empirical evidence of study publication bias and outcome reporting bias – an updated review. PLoS One 8(7):1–37
Easterbrook PJ, Gopalan R, Berlin JA, Matthews DR (1991) Publication bias in clinical research. Lancet 337(8746):867–872
Egger M, Smith GD, Schneider M, Minder C (1997) Bias in meta-analysis detected by a simple, graphical test. BMJ 315(7109):629–634
Eyding D, Lelgemann M, Grouven U, Härter M, Kromp M, Kaiser T et al (2010) Reboxetine for acute treatment of major depression: systematic review and meta-analysis of published and unpublished placebo and selective serotonin reuptake inhibitor controlled trials. BMJ 341:c4737
Ferguson JM, Mendels J, Schwartz GE (2002) Effects of reboxetine on Hamilton depression rating scale factors from randomized, placebo-controlled trials in major depression. Int Clin Psychopharmacol 17(2):45–51
Frank RA, Sharifabadi AD, Salameh J-P, McGrath TA, Kraaijpoel N, Dang W et al (2019) Citation bias in imaging research: are studies with higher diagnostic accuracy estimates cited more often? Eur Radiol 29(4):1657–1664
Gehr BT, Weiss C, Porzsolt F (2006) The fading of reported effectiveness. A meta-analysis of randomised controlled trials. BMC Med Res Methodol 6(1):25
Glasziou P, Altman DG, Bossuyt P, Boutron I, Clarke M, Julious S et al (2014) Reducing waste from incomplete or unusable reports of biomedical research. Lancet 383(9913):267–276
Gøtzsche PC (1987) Reference bias in reports of drug trials. Br Med J Clin Res Ed 295(6599):654–656
Hahn S, Williamson PR, Hutton JL (2002) Investigation of within-study selective reporting in clinical research: follow-up of applications submitted to a local research ethics committee. J Eval Clin Pract 8(3):353–359
Hall R, de Antueno C, Webber A (2007) Publication bias in the medical literature: a review by a Canadian research ethics board. Can J Anesth 54(5):380–388
Hart B, Lundh A, Bero L (2012) Effect of reporting bias on meta-analyses of drug trials: reanalysis of meta-analyses. BMJ 344:d7202
Hartling L, Craig WR, Russell K, Stevens K, Klassen TP (2004) Factors influencing the publication of randomized controlled trials in child health research. Arch Pediatr Adolesc Med 158(10):983–987
Hartling L, Featherstone R, Nuspl M, Shave K, Dryden D, Vandermeer B (2017) Grey literature in systematic reviews: a cross-sectional study of the contribution of non-English reports, unpublished studies and dissertations to the results of meta-analyses in child-relevant reviews. Syst Rev 17:64
Heres S, Wagenpfeil S, Hamann J, Kissling W, Leucht S (2004) Language bias in neuroscience – is the Tower of Babel located in Germany? Eur Psychiatry 19(4):230–232
Hetherington J, Dickersin K, Chalmers I, Meinert CL (1989) Retrospective and prospective identification of unpublished controlled trials: lessons from a survey of obstetricians and pediatricians. Pediatrics 84(2):374–380
Hill KP, Ross JS, Egilman DS, Krumholz HM (2008) The ADVANTAGE seeding trial: a review of internal documents. Ann Intern Med 149(4):251
Hopewell S, Clarke M, Stewart L, Tierney J (2007) Time to publication for results of clinical trials. Cochrane Database Syst Rev 2007(2):MR000011
Hopewell S, Loudon K, Clarke MJ, Oxman AD, Dickersin K (2009) Publication bias in clinical trials due to statistical significance or direction of trial results. Cochrane Database Syst Rev 1:MR000006
Jefferson T, Doshi P, Boutron I, Golder S, Heneghan C, Hodkinson A et al (2018) When to include clinical study reports and regulatory documents in systematic reviews. BMJ Evid-Based Med 23(6):210–217
Jennions MD, Møller AP (2002) Relationships fade with time: a meta-analysis of temporal trends in publication in ecology and evolution. Proc R Soc Lond B Biol Sci 269(1486):43–48
Jones CW, Keil LG, Holland WC, Caughey MC, Platts-Mills TF (2015) Comparison of registered and published outcomes in randomized controlled trials: a systematic review. BMC Med 13:282
Jüni P, Holenstein F, Sterne J, Bartlett C, Egger M (2002) Direction and impact of language bias in meta-analyses of controlled trials: empirical study. Int J Epidemiol 31(1):115–123
Kicinski M (2014) How does under-reporting of negative and inconclusive results affect the false-positive rate in meta-analysis? A simulation study. BMJ Open 4(8):e004831
Kirkham JJ, Dwan KM, Altman DG, Gamble C, Dodd S, Smyth R, Williamson PR (2010) The impact of outcome reporting bias in randomised controlled trials on a cohort of systematic reviews. BMJ 340:c365
Kirsch I, Deacon BJ, Huedo-Medina TB, Scoboria A, Moore TJ, Johnson BT (2008) Initial severity and antidepressant benefits: a meta-analysis of data submitted to the Food and Drug Administration. PLoS Med 5(2):e45
Kjaergard LL, Gluud C (2002) Citation bias of hepato-biliary randomized clinical trials. J Clin Epidemiol 55(4):407–410
Lee KP, Boyd EA, Holroyd-Leduc JM, Bacchetti P, Bero LA (2006) Predictors of publication: characteristics of submitted manuscripts associated with acceptance at major biomedical journals. Med J Aust 184(12):621–626
Lexchin J, Bero LA, Djulbegovic B, Clark O (2003) Pharmaceutical industry sponsorship and research outcome and quality: systematic review. BMJ 326(7400):1167–1170
Li T, Mayo-Wilson E, Fusco N, Hong H, Dickersin K (2018) Caveat emptor: the combined effects of multiplicity and selective reporting. Trials 19(1):497
Liebeskind DS, Kidwell CS, Sayre JW, Saver JL (2006) Evidence of publication bias in reporting acute stroke clinical trials. Neurology 67(6):973–979
Lundh A, Lexchin J, Mintzes B, Schroll JB, Bero L (2017) Industry sponsorship and research outcome. Cochrane Database Syst Rev 2(2):MR000033
Mahoney MJ (1977) Publication prejudices: an experimental study of confirmatory bias in the peer review system. Cogn Ther Res 1(2):161–175
Malberg JE, Eisch AJ, Nestler EJ, Duman RS (2000) Chronic antidepressant treatment increases neurogenesis in adult rat hippocampus. J Neurosci 20(24):9104–9110
Marks-Anglin A, Chen Y (2020) A historical review of publication bias. Res Synth Methods 11(6):725–742
Matheson A (2017) Marketing trials, marketing tricks – how to spot them and how to stop them. Trials 18:105
Mayo-Wilson E, Li T, Fusco N, Bertizzolo L, Canner JK, Cowley T et al (2017) Cherry-picking by trialists and meta-analysts can drive conclusions about intervention efficacy. J Clin Epidemiol 91(Suppl C):95–110
Mayo-Wilson E, Fusco N, Li T, Hong H, Canner JK, Dickersin K et al (2019) Harms are assessed inconsistently and reported inadequately Part 2: nonsystematic adverse events. J Clin Epidemiol 113:11–19
McCormack JP, Rangno R (2002) Digging for data from the COX-2 trials. CMAJ 166(13):1649–1650
McGauran N, Wieseler B, Kreis J, Schüler Y-B, Kölsch H, Kaiser T (2010) Reporting bias in medical research – a narrative review. Trials 11:37
Melander H, Ahlqvist-Rastad J, Meijer G, Beermann B (2003) Evidence b(i)ased medicine – selective reporting from studies sponsored by pharmaceutical industry: review of studies in new drug applications. BMJ 326(7400):1171–1173
Morrison A, Polisena J, Husereau D, Moulton K, Clark M, Fiander M, Mierzwinski-Urban M, Clifford T, Hutton B, Rabb D (2012) The effect of English-language restriction on systematic review-based meta-analyses: a systematic review of empirical studies. Int J Technol Assess Health Care 28:138–144
Mukherjee D, Nissen SE, Topol EJ (2001) Risk of cardiovascular events associated with selective COX-2 inhibitors. JAMA 286(8):954–959
Olson CM, Rennie D, Cook D, Dickersin K, Flanagin A, Hogan JW et al (2002) Publication bias in editorial decision making. JAMA 287(21):2825–2828
Østengaard L, Lundh A, Tjørnhøj-Thomsen T, Abdi S, Gelle MHA, Stewart LA, Boutron I, Hróbjartsson A (2020) Influence and management of conflicts of interest in randomised clinical trials: qualitative interview study. BMJ 371:m3764
Ottenbacher K, DiFabio RP (1985) Efficacy of spinal manipulation/mobilization therapy. A meta-analysis. Spine 10(9):833–837
Page MJ, McKenzie JE, Higgins JPT (2018) Tools for assessing risk of reporting biases in studies and syntheses of studies: a systematic review. BMJ Open 8(3):e019703
Page MJ, Higgins JPT, Sterne JAC (2019) Chapter 13: assessing risk of bias due to missing results in a synthesis. In: Higgins JPT, Thomas J, Chandler J, Cumpston M, Li T, Page MJ, Welch VA (eds) Cochrane handbook for systematic reviews of interventions. Version 6.0 (updated July 2019). Available from www.training.cochrane.org/handbook . Cochrane
Psaty BM, Kronmal RA (2008) Reporting mortality findings in trials of Rofecoxib for Alzheimer disease or cognitive impairment: a case study based on documents from Rofecoxib litigation. JAMA 299(15):1813–1817
Scherer RW, Ugarte-Gil C, Schmucker C, Meerpohl JJ (2015) Authors report lack of time as main reason for unpublished research presented at biomedical conferences: a systematic review. J Clin Epidemiol 68(7):803–810
Scherer RW, Meerpohl JJ, Pfeifer N, Schmucker C, Schwarzer G, von Elm E (2018) Full publication of results initially presented in abstracts. Cochrane Database Syst Rev 11(11):MR000005
Schmucker C, Schell LK, Portalupi S, Oeller P, Cabrera L, Bassler D, Schwarzer G, Scherer RW, Antes G, von Elm E, Meerpohl JJ (2014) Extent of non-publication in cohorts of studies approved by research ethics committees or included in trial registries. PLoS One 9:e114023
Schmucker CM, Blumle A, Schell LK, Schwarzer G, Oeller P, Cabrera L, von Elm E, Briel M, Meerpohl JJ (2017) Systematic review finds that study data not published in full text articles have unclear impact on meta-analyses results in medical research. PLoS One 12:e0176210
Simes RJ (1986) Publication bias: the case for an international registry of clinical trials. J Clin Oncol 4(10):1529–1541
Sismondo S (2007) Ghost management: how much of the medical literature is shaped behind the scenes by the pharmaceutical industry? PLoS Med 4(9):e286
Sismondo S (2008a) Pharmaceutical company funding and its consequences: a qualitative systematic review. Contemp Clin Trials 29(2):109–113
Sismondo S (2008b) How pharmaceutical industry funding affects trial outcomes: causal structures and responses. Soc Sci Med 66(9):1909–1914
Smith ML (1980) Publication bias and meta-analysis. Eval Educ 4:22–24
Smyth RM, Kirkham JJ, Jacoby A, Altman DG, Gamble C, Williamson PR (2011) Frequency and reasons for outcome reporting bias in clinical trials: interviews with trialists. BMJ 342:c7153
Song F, Parekh S, Hooper L, Loke YK, Ryder J, Sutton AJ, Hing C, Kwok CS, Pang C, Harvey I (2010) Dissemination and publication of research findings: an updated review of related biases. Health Technol Assess 14(8):iii, ix–xi, 1–193
Song F, Loke Y, Hooper L (2014) Why are medical and health-related studies not being published? A systematic review of reasons given by investigators. PLoS One 9(10):e110418
Sterling TD (1959) Publication decisions and their possible effects on inferences drawn from tests of significance – or vice versa. J Am Stat Assoc 54(285):30–34
Stern JM, Simes RJ (1997) Publication bias: evidence of delayed publication in a cohort study of clinical research projects. BMJ 315(7109):640–645
Tendal B, Nüesch E, Higgins JPT, Jüni P, Gøtzsche PC (2011) Multiplicity of data in trial reports and the reliability of meta-analyses: empirical study. BMJ 343:d4829
Turner EH, Matthews AM, Linardatos E, Tell RA, Rosenthal R (2008) Selective publication of antidepressant trials and its influence on apparent efficacy. N Engl J Med 358(3):252–260
van Lent M, Overbeke J, Out HJ (2014) Role of editorial and peer review processes in publication bias: analysis of drug trials submitted to eight medical journals. PLoS One 9(8):e104846
Vandekerckhove P, O’Donovan PA, Lilford RJ, Harada TW (1993) Infertility treatment: from cookery to science. The epidemiology of randomised controlled trials. BJOG Int J Obstet Gynaecol 100(11):1005–1036
Vedula SS, Bero L, Scherer RW, Dickersin K (2009) Outcome reporting in industry-sponsored trials of gabapentin for off-label use. N Engl J Med 361(20):1963–1971
Vedula SS, Goldman PS, Rona IJ, Greene TM, Dickersin K (2012) Implementation of a publication strategy in the context of reporting biases. A case study based on new documents from Neurontin litigation. Trials 13:136
Vedula SS, Li T, Dickersin K (2013) Differences in reporting of analyses in internal company documents versus published trial reports: comparisons in industry-sponsored trials in off-label uses of gabapentin. PLoS Med 10(1):e1001378
Vera Badillo FE, Shapiro R, Ocana A, Amir E, Tannock I (2012) Bias in reporting of endpoints of efficacy and toxicity in randomized clinical trials (RCTs) for women with breast cancer (BC). J Clin Oncol 30(Suppl 15):6043–6043
Vevea JL, Coburn K, Sutton A (2019) Publication bias. In: Cooper H, Hedges LV, Valentine JC (eds) The handbook of research synthesis and meta-analysis. Russell Sage Foundation, pp 383–430, New York, USA
von Elm E, Poglia G, Walder B, Tramèr MR (2004) Different patterns of duplicate publication: an analysis of articles used in systematic reviews. JAMA 291(8):974–980
Wallach JD, Krumholz HM (2019) Not reporting results of a clinical trial is academic misconduct. Ann Intern Med 171(4):293
Wang AT, McCoy CP, Murad MH, Montori VM (2010) Association between industry affiliation and position on cardiovascular risk with rosiglitazone: cross sectional systematic review. BMJ 340:c1344
Download references
Author information
Authors and affiliations.
Malone Center for Engineering in Healthcare, Whiting School of Engineering, The Johns Hopkins University, Baltimore, MD, USA
S. Swaroop Vedula
Cochrane Denmark and Centre for Evidence-Based Medicine Odense, University of Southern Denmark, Odense, Denmark
Asbjørn Hróbjartsson
School of Public Health and Preventive Medicine, Monash University, Melbourne, VIC, Australia
Matthew J. Page
You can also search for this author in PubMed Google Scholar
Corresponding author
Correspondence to S. Swaroop Vedula .
Editor information
Editors and affiliations.
Department of Surgery, Division of Surgical Oncology, Brigham and Women’s Hospital, Harvard Medical School, Boston, MA, USA
Steven Piantadosi
Department of Epidemiology, School of Public Health, Johns Hopkins University, Baltimore, MD, USA
Curtis L. Meinert
Section Editor information
Department of Ophthalmology, University of Colorado Anschutz Medical Campus, Aurora, CO, USA
Tianjing Li
The Johns Hopkins Center for Clinical Trials and Evidence Synthesis, Johns Hopkins School of Public Health, Baltimore, MA, USA
Brigham and Women’s Hospital, Harvard Medical School, Boston, MA, USA
Rights and permissions
Reprints and permissions
Copyright information
© 2022 Springer Nature Switzerland AG
About this entry
Cite this entry.
Vedula, S.S., Hróbjartsson, A., Page, M.J. (2022). Reporting Biases. In: Piantadosi, S., Meinert, C.L. (eds) Principles and Practice of Clinical Trials. Springer, Cham. https://doi.org/10.1007/978-3-319-52636-2_183
Download citation
DOI : https://doi.org/10.1007/978-3-319-52636-2_183
Published : 20 July 2022
Publisher Name : Springer, Cham
Print ISBN : 978-3-319-52635-5
Online ISBN : 978-3-319-52636-2
eBook Packages : Mathematics and Statistics Reference Module Computer Science and Engineering
Share this entry
Anyone you share the following link with will be able to read this content:
Sorry, a shareable link is not currently available for this article.
Provided by the Springer Nature SharedIt content-sharing initiative
- Publish with us
Policies and ethics
- Find a journal
- Track your research
Health Research Reporting Guidelines, Study Execution Manuals, Critical Appraisal, Risk of Bias, & Non-reporting Biases
- Health Research Reporting Guidelines
- Study Execution Manuals
- Critical Appraisal
- Risk of Bias
What are reporting biases?
List of reporting biases, tools to assess non-reporting biases.
- Quality of Evidence
- HSLS Systematic Review LibGuide
For additional information, contact:
Helena VonVille, MLS, MPH
- HSLS Pitt Public Health Liaison
- HSLS Research & Instruction Librarian
- Email me: [email protected]
- Schedule an appointment with me
Cochrane Handbook for Systematic Reviews of Interventions
Chapter 13: Assessing risk of bias due to missing results in a synthesis focuses on the causes of missing evidence, such as studies that aren't published or non-significant outcomes aren't reported. It then describes a framework for describing the assessment of missing results.
The language on this topic has changed over the years.
- PRISMA (2009) referred to this as " risk of bias across studies "
- PRISMA-P (2015; Item 16) refers to this as " meta-biases "
- PRISMA 2020 (Item 14) refers to this as " reporting bias assessment "
- The Cochrane Handbook also refers to these as " non-reporting biases "
While the terminology has changed, the intent is the same:
- Determine what evidence is missing in the final analysis; and
- Determine how that impacts the summary of evidence.
Where are these reported in an SR?
Any systematic deviation that results in a potential skewing away from the truth of the results should be evaluated and reported. The PRISMA reporting guidelines for a protocol and for the manuscript all include items for assessing these types of biases.
- Item #16: Specify any planned assessment of meta-bias(es) (sometimes called non-reporting biases across studies)
- Item #14: Describe any methods used to assess risk of bias due to missing results in a synthesis (arising from reporting biases) (See the grey box at the bottom of page 18 of the PRISMA E&E )
- Item #21: Present assessments of risk of bias due to missing results (arising from reporting biases) for each synthesis assessed.
What are non-reporting biases?
Non-reporting biases encompass a variety of categories. The Catalogue of Bias ( Catalogue of Biases . Richards GC, Onakpoya IJ. Reporting biases. In: Catalog Of Bias 2019) refers to reporting bias as, "A systematic distortion that arises from the selective disclosure or withholding of information by parties involved in the design, conduct, analysis, or dissemination of a study or research findings". It lists the following types of reporting biases:
- Publication bias
- Time-lag bias
- Multiple (duplicate) publication bias
- Location bias
- Citation bias
- Language bias
- Outcome reporting bias
This page will be updated as new tools and resources become available.
Risk of Bias Tools (Cochrane)
- A cribsheet summarizing the tool .
- A template for completing the assessment .
- Full guidance for the updated version is not available yet.
Chiocchia V, Nikolakopoulou A, Higgins JPT, Page MJ, Papakonstantinou T, Cipriani A, Furukawa TA, Siontis GCM, Egger M, Salanti G. ROB-MEN: a tool to assess risk of bias due to missing evidence in network meta-analysis. BMC Med. 2021 Nov 23;19(1):304. doi: 10.1186/s12916-021-02166-3 . PMID: 34809639 ; PMCID: PMC8609747 .
- << Previous: Risk of Bias
- Next: Quality of Evidence >>
- Last Updated: May 23, 2024 2:33 PM
- URL: https://hsls.libguides.com/reporting-study-tools
- Open access
- Published: 13 April 2010
Reporting bias in medical research - a narrative review
- Natalie McGauran 1 ,
- Beate Wieseler 1 ,
- Julia Kreis 1 ,
- Yvonne-Beatrice Schüler 1 ,
- Heike Kölsch 1 &
- Thomas Kaiser 1
Trials volume 11 , Article number: 37 ( 2010 ) Cite this article
100k Accesses
289 Citations
132 Altmetric
Metrics details
Reporting bias represents a major problem in the assessment of health care interventions. Several prominent cases have been described in the literature, for example, in the reporting of trials of antidepressants, Class I anti-arrhythmic drugs, and selective COX-2 inhibitors. The aim of this narrative review is to gain an overview of reporting bias in the medical literature, focussing on publication bias and selective outcome reporting. We explore whether these types of bias have been shown in areas beyond the well-known cases noted above, in order to gain an impression of how widespread the problem is. For this purpose, we screened relevant articles on reporting bias that had previously been obtained by the German Institute for Quality and Efficiency in Health Care in the context of its health technology assessment reports and other research work, together with the reference lists of these articles.
We identified reporting bias in 40 indications comprising around 50 different pharmacological, surgical (e.g. vacuum-assisted closure therapy), diagnostic (e.g. ultrasound), and preventive (e.g. cancer vaccines) interventions. Regarding pharmacological interventions, cases of reporting bias were, for example, identified in the treatment of the following conditions: depression, bipolar disorder, schizophrenia, anxiety disorder, attention-deficit hyperactivity disorder, Alzheimer's disease, pain, migraine, cardiovascular disease, gastric ulcers, irritable bowel syndrome, urinary incontinence, atopic dermatitis, diabetes mellitus type 2, hypercholesterolaemia, thyroid disorders, menopausal symptoms, various types of cancer (e.g. ovarian cancer and melanoma), various types of infections (e.g. HIV, influenza and Hepatitis B), and acute trauma. Many cases involved the withholding of study data by manufacturers and regulatory agencies or the active attempt by manufacturers to suppress publication. The ascertained effects of reporting bias included the overestimation of efficacy and the underestimation of safety risks of interventions.
In conclusion, reporting bias is a widespread phenomenon in the medical literature. Mandatory prospective registration of trials and public access to study data via results databases need to be introduced on a worldwide scale. This will allow for an independent review of research data, help fulfil ethical obligations towards patients, and ensure a basis for fully-informed decision making in the health care system.
Peer Review reports
The reporting of research findings may depend on the nature and direction of results, which is referred to as "reporting bias" [ 1 , 2 ]. For example, studies in which interventions are shown to be ineffective are sometimes not published, meaning that only a subset of the relevant evidence on a topic may be available [ 1 , 2 ]. Various types of reporting bias exist (Table 1 ), including publication bias and outcome reporting bias, which concern bias from missing outcome data on 2 levels: the study level, i.e. "non-publication due to lack of submission or rejection of study reports", and the outcome level, i.e. "the selective non-reporting of outcomes within published studies" [ 3 ].
Reporting bias on a study level
Results of clinical research are largely underreported or reported with delay. Various analyses of research protocols submitted to institutional review boards and research ethics committees in Europe, the United States, and Australia found that on average, only about half of the protocols had been published, with higher publication rates in Anglo-Saxon countries [ 4 – 10 ].
Similar analyses have been performed of trials submitted to regulatory authorities: a cohort study of trials supporting new drugs approved by the Food and Drug Administration (FDA) identified over 900 trials of 90 new drugs in FDA reviews; only 43% of the trials were published [ 11 ]. Wide variations in publication rates have been shown for specific indications [ 12 – 16 ]. The selective submission of clinical trials with positive outcomes to regulatory authorities has also been described [ 17 ]. Even if trials are published, the time lapse until publication may be substantial [ 8 , 18 , 19 ].
There is no simple classification of a clinical trial into "published" or "unpublished", as varying degrees of publication exist. These range from full-text publications in peer-reviewed journals that are easily identifiable through a search in bibliographic databases, to study information entered in trial registries, so-called grey literature (e.g. abstracts and working papers), and data on file in drug companies and regulatory agencies, which may or may not be provided to health technology assessment (HTA) agencies or other researchers after being requested. If such data are transmitted, they may then be fully published or not (e.g. the German Institute for Quality and Efficiency in Health Care [Institut für Qualität und Wirtschaftlichkeit im Gesundheitswesen, IQWiG] publishes all data used in its assessment reports [ 20 ], whereas the UK National Institute for Clinical Excellence [NICE] may accept "commercial in confidence" data [ 21 ]).
Even if studies are presented at meetings, this does not necessarily mean subsequent full publication: an analysis of nearly 30,000 meeting abstracts from various disciplines found a publication rate of 63% for randomized or controlled clinical trials [ 22 ].
Reporting bias on an outcome level
Selective reporting within a study may involve (a) selective reporting of analyses or (b) selective reporting of outcomes. This may include, for example, the reporting of (a) per-protocol (PP) versus intention-to-treat (ITT) analyses or adjusted versus unadjusted analyses, and (b) outcomes from different time points or statistically significant versus non-significant outcomes [ 3 , 23 ].
Various reviews have found extensive selective reporting in study publications [ 3 , 14 , 24 – 28 ]. For example, comparisons of publications with study protocols have shown that primary outcomes had been newly introduced, omitted, or changed in about 40% to 60% of cases [ 3 , 24 ]. Selective reporting particularly concerns the underreporting of adverse events [ 12 , 29 – 32 ]. For example, an analysis of 192 randomized drug trials in various indications showed that only 46% of publications stated the frequency of specific reasons for treatment discontinuation due to toxicity [ 29 ]. Outcomes are not only selectively reported, but negative results are reported in a positive manner and conclusions are often not supported by results data [ 16 , 26 , 33 – 35 ]. For instance, a comparison of study characteristics reported in FDA reviews of New Drug Applications (NDAs) with those reported in publications found that 9 of 99 conclusions had been changed in the publications, all in favour of the new drug [ 26 ].
Factors associated with reporting bias
Characteristics of published studies.
The fact that studies with positive or favourable results are more likely to be published than those with negative or unfavourable results was already addressed in the 1950s [ 36 ], and has since been widely confirmed [ 3 , 6 – 8 , 14 , 37 – 40 ]. Studies with positive or favourable results have been associated with various other factors such as faster publication [ 8 , 18 , 19 , 37 ], publication in higher impact factor journals [ 7 , 41 ], a greater number of publications [ 7 ] (including covert duplicate publications [ 42 ]), more frequent citation [ 43 – 45 ], and more likely publication in English [ 46 ].
Several other factors have been linked to successful publication, for example, methodological quality [ 47 ], study type [ 47 ], sample size [ 5 , 7 , 48 ], multicentre status [ 5 , 6 , 41 ], and non-commercial funding [ 5 , 6 , 49 , 50 ]. However, for some factors, these associations are inconsistent [ 6 , 37 ].
Submission and rejection of studies
One of the main reasons for the non-publication of negative studies seems to be the non-submission of manuscripts by investigators, not the rejection of manuscripts by medical journals. A follow-up of studies approved by US institutional review boards showed that only 6 of 124 unpublished studies had actually been rejected for publication [ 6 ]. A prospective cohort study of 745 manuscripts submitted to JAMA showed no statistically significant difference in publication rates between studies with positive and those with negative results [ 51 ], which has been confirmed by further analyses of other journals [ 47 , 52 ]. Author surveys have shown that the most common reasons for not submitting papers were negative results and a lack of interest, time, or other resources [ 39 – 41 , 53 ].
The role of the pharmaceutical industry
An association has been shown between industry sponsorship or industry affiliation of authors and positive research outcomes and conclusions, both in publications of primary studies and in systematic reviews [ 49 , 54 – 63 ]. For example, in a systematic review of the scope and impact of financial conflicts of interest in biomedical research, an aggregation of the results of 8 analyses of the relation between industry sponsorship and outcomes showed a statistically significant association between industry sponsorship and pro-industry conclusions [ 55 ]. A comparison of the methodological quality and conclusions in Cochrane reviews with those in industry-supported meta-analyses found that the latter were less transparent, less critical of methodological limitations of the included trials, and drew more favourable conclusions [ 57 ]. In addition, publication constraints and active attempts to prevent publication have been identified in industry-sponsored research [ 55 , 64 – 68 ]. Other aspects of industry involvement, such as design bias, are beyond the scope of this paper.
Rationale, aim and procedure
IQWiG produces HTA reports of drug and non-drug interventions for the decision-making body of the statutory health care funds, the Federal Joint Committee. The process of report production includes requesting information on published and unpublished studies from manufacturers; unfortunately, compliance by manufacturers is inconsistent, as recently shown in the attempted concealment of studies on antidepressants [ 69 ]. Reporting bias in antidepressant research has been shown before [ 16 , 70 ]; other well-known cases include Class I anti-arrhythmic drugs [ 71 , 72 ] and selective COX-2 inhibitors [ 73 , 74 ].
The aim of this narrative review was to gain an overview of reporting bias in the medical literature, focussing on publication bias and selective outcome reporting. We wished to explore whether this type of bias has been shown in areas beyond the well-known cases noted above, in order to obtain an impression of how widespread this problem is. The review was based on the screening of full-text publications on reporting bias that had either been obtained by the Institute in the context of its HTA reports and other research work or were identified by the screening of the reference lists of the on-site publications. The retrieved examples were organized according to indications and interventions. We also discuss the effects of reporting bias, as well as the measures that have been implemented to solve this problem.
The term "reporting bias" traditionally refers to the reporting of clinical trials and other types of studies; if one extends this term beyond experimental settings, for example, to the withholding of information on any beneficial medical innovation, then an early example of reporting bias was noted by Rosenberg in his article "Secrecy in medical research", which describes the invention of the obstetrical forceps. This device was developed by the Chamberlen brothers in Europe in the 17th century; however, it was kept secret for commercial reasons for 3 generations and as a result, many women and neonates died during childbirth [ 75 ]. In the context of our paper, we also considered this extended definition of reporting bias.
We identified reporting bias in 40 indications comprising around 50 different interventions. Examples were found in various sources, e.g. journal articles of published versus unpublished data, reviews of reporting bias, editorials, letters to the editor, newspaper reports, expert and government reports, books, and online sources. The following text summarizes the information presented in these examples. More details and references to the background literature are included in Additional file 1 : Table S2.
Mental and behavioural disorders
Reporting bias is common in psychiatric research (see below). This also includes industry-sponsorship bias [ 76 – 82 ].
Turner et al compared FDA reviews of antidepressant trials including over 12,000 patients with the matching publications and found that 37 out of 38 trials viewed as positive by the FDA were published [ 16 ]. Of the 36 trials having negative or questionable results according to the FDA, 22 were unpublished and 11 of the 14 published studies conveyed a positive outcome. According to the publications, 94% of the trials had positive results, which was in contrast to the proportion reported by the FDA (51%). The overall increase in effect size in the published trials was 32%. In a meta-analysis of data from antidepressant trials submitted to the FDA, Kirsch et al requested data on 6 antidepressants from the FDA under the Freedom of Information Act. However, the FDA did not disclose relevant data from 9 of 47 trials, all of which failed to show a statistically significant benefit over placebo. Data from 4 of these trials were available on the GlaxoSmithKline (GSK) website. In total, the missing data represented 38% of patients in sertraline trials and 23% of patients in citalopram trials. The analysis of trials investigating the 4 remaining antidepressants showed that drug-placebo differences in antidepressant efficacy were relatively small, even for severely depressed patients [ 83 ].
Selective serotonin reuptake inhibitors (SSRIs)
One of the biggest controversies surrounding unpublished data was the withholding of efficacy and safety data from SSRI trials. In a lawsuit launched by the Attorney General of the State of New York it was alleged that GSK had published positive information about the paediatric use of paroxetine in major depressive disorder (MDD), but had concealed negative safety and efficacy data [ 84 ]. The company had conducted at least 5 trials on the off-label use of paroxetine in children and adolescents but published only one, which showed mixed results for efficacy. The results of the other trials, which did not demonstrate efficacy and suggested a possible increased risk of suicidality, were suppressed [ 84 ]. As part of a legal settlement, GSK agreed to establish an online clinical trials registry containing results summaries for all GSK-sponsored studies conducted after a set date [ 85 , 86 ].
Whittington et al performed a systematic review of published versus unpublished data on SSRIs in childhood depression. While published data indicated a favourable risk-benefit profile for some SSRIs, the inclusion of unpublished data indicated a potentially unfavourable risk-benefit profile for all SSRIs investigated except fluoxetine [ 70 ].
Newer antidepressants
IQWiG published the preliminary results of an HTA report on reboxetine, a selective norepinephrine reuptake inhibitor, and other antidepressants. At least 4600 patients had participated in 16 reboxetine trials, but the majority of data were unpublished. Despite a request for information the manufacturer Pfizer refused to provide these data. Only data on about 1600 patients were analysable and IQWiG concluded that due to the risk of publication bias, no statement on the benefit or harm of reboxetine could be made [ 69 , 87 ]. The preliminary HTA report mentioned above also included an assessment of mirtazapine, a noradrenergic and specific serotonergic antidepressant. Four potentially relevant trials were identified in addition to 27 trials included in the assessment, but the manufacturer Essex Pharma did not provide the study reports. Regarding the other trials, the manufacturer did not send the complete study reports, so the full analyses were not available. IQWiG concluded that the results of the assessment of mirtazapine may have been biased by unpublished data [ 69 , 87 ]. After the behaviour of Pfizer and Essex Pharma had been widely publicized, the companies provided the majority of study reports for the final HTA report. The preliminary report's conclusion on the effects of mirtazapine was not affected by the additional data. For reboxetine, the analysis of the published and unpublished data changed the conclusion from "no statement possible" to "no benefit proven" [ 88 ].
Bipolar disorder
Lamotrigine.
A review by Nassir Ghaemi et al of data on lamotrigine in bipolar disorder provided on the GSK website showed that data from negative trials were available on the website but that the studies had not been published in detail or publications emphasized positive secondary outcomes instead of negative primary ones. Outside of the primary area of efficacy (prophylaxis of mood episodes), the drug showed very limited efficacy in indications such as acute bipolar depression, for which clinicians were supporting its use [ 35 ].
Gabapentin, a GABA analogue, was approved by the FDA in 1993 for a certain type of epilepsy, and in 2002 for postherpetic neuralgia. As of February 1996, 83% of gabapentin use was for epilepsy, and 17% for off-label indications (see the expert report by Abramson [ 89 ]). As the result of a comprehensive marketing campaign by Pfizer, the number of patients in the US taking gabapentin rose from about 430,000 to nearly 6 million between 1996 and 2001; this increase was solely due to off-label use for indications, including bipolar disorder. As of September 2001, 93.5% of gabapentin use was for off-label indications [ 89 ]. In a further expert report, Dickersin noted "extensive evidence of reporting bias" [ 34 ], which she further analysed in a recent publication with Vedula et al [ 90 ]. Concerning the trials of gabapentin for bipolar disorders, 2 of the 3 trials (all published) were negative for the primary outcome. However, these publications showed "extensive spin and misrepresentation of data" [ 34 ].
Schizophrenia
The Washington Post reported that a trial on quetiapine, an atypical antipsychotic, was "silenced" in 1997, the same year it was approved by the FDA to treat schizophrenia. The study ("Study 15") was not published. Patients taking quetiapine had shown high rates of treatment discontinuations and had experienced significant weight increases. However, data presented by the manufacturer AstraZeneca in 1999 at European and US meetings actually indicated that the drug helped psychotic patients lose weight [ 91 ].
Panic disorder
Turner described an example of reporting bias in the treatment of panic disorder: according to a review article, 3 "well designed studies" had apparently shown that the controlled-release formulation of paroxetine had been effective in patients with this condition. However, according to the corresponding FDA statistical review, only one study was strongly positive, the second study was non-significant regarding the primary outcome (and marginally significant for a secondary outcome), and the third study was clearly negative [ 92 ].
Further examples of reporting bias in research on mental and behavioural disorders are included in Additional file 1 : Table S2.
Disorders of the nervous system
Alzheimer's disease.
Internal company analyses and information provided by the manufacturer Merck & Co to the FDA on rofecoxib, a selective COX-2 inhibitor, were released during litigation procedures. The documents referred to trials investigating the effects of rofecoxib on the occurrence or progression of Alzheimer's disease. Psaty and Kronmal performed a review of these documents and 2 trial publications and showed that, although presenting mortality data, the publications had not included analyses or statistical tests of these data and both had concluded that regarding safety, rofecoxib was "well tolerated". In contrast, in April 2001, Merck's internal ITT analyses of pooled data from these 2 trials showed a significant increase in total mortality. However, this information was neither disclosed to the FDA nor published in a timely fashion [ 74 ]. Rofecoxib was taken off the market by Merck in 2004 [ 93 ], among allegations that the company had been aware of the safety risks since 2000 [ 73 ].
In their article "An untold story?", Lenzer and Brownlee reported the case of valdecoxib, another selective COX-2 inhibitor withdrawn from the market due to cardiovascular concerns [ 94 , 95 ]. In 2001, the manufacturer Pfizer had applied for approval in 4 indications, including acute pain. The application for acute pain was rejected and some of the information about the corresponding trials removed from the FDA website for confidentiality reasons. Further examples of reporting bias in research on pain are presented in Additional file 1 : Table S2.
According to the expert report by Dickersin, all 3 trials on gabapentin for migraine showed negative results for the primary outcome. Substantial reporting bias was present. One trial was fully published (seemingly with a redefined primary outcome showing positive results in a subgroup of patients), one was unpublished, and preliminary (positive) results were presented for the third trial [ 34 ].
Disorders of the circulatory system
Coronary heart disease (bleeding prophylaxis during bypass surgery).
In his article on observational studies on drug safety, Hiatt reported the case of aprotinin, an antifibrinolytic drug formerly marketed to reduce bleeding during heart bypass graft surgery. In 2006, data from 2 published observational studies indicated serious concerns about the drug's safety [ 96 ]. The FDA subsequently convened an expert meeting in which the safety data presented by the manufacturer Bayer did not reveal any increased risk of fatal or nonfatal cardiovascular events. However, it turned out that Bayer had not presented additional observational data, which, according to an FDA review, indicated that aprotinin may be associated with an increased risk of death and other serious adverse events. In November 2007 Bayer suspended the worldwide marketing of aprotinin, after requests and advice from various drug regulating authorities [ 97 ].
Prevention of arrhythmia
Class i anti-arrhythmic drugs.
In a clinical trial conducted in 1980, 9 out of 49 patients with suspected acute myocardial infarction treated with a class Ic anti-arrhythmic drug (lorcainide) died, versus only one patient in the placebo group; the investigators interpreted this finding as an "effect of chance" [ 71 ]. The development of lorcainide was discontinued for commercial reasons, and the results of the trial were not published until 1993. The investigators then stated that if the trial had been published earlier, it "might have provided an early warning of trouble ahead" [ 71 ]. Instead, during the 1980s, class I drugs were widely used, even though concerns as to their lack of effect were published as early as 1983 [ 98 , 99 ]. Further reviews and trials confirmed this suspicion, as well as an increase in mortality [ 100 – 102 ]. In his book "Deadly Medicine", Moore described the consequences as "America's worst drug disaster", which had "produced a death toll larger than the United States' combat losses in wars such as Korea and Vietnam" [ 72 ]. Further examples of reporting bias in research on disorders of the circulatory system are presented in Additional file 1 : Table S2.
Disorders of the digestive system
Irritable bowel syndrome.
Barbehenn et al compared a published trial on alosetron, a 5-HT 3 antagonist, in women with irritable bowel syndrome with data obtained from the FDA [ 103 ]. She noted that according to the graphics in the publication, which presented relative differences in pain and discomfort scores, the drug seemed effective. However, when plotting the absolute data from the FDA review, the data points were almost superimposable. After discussions with the FDA about potential serious side effects, the drug was withdrawn from the market by the manufacturer in 2000, but reapproved with restrictions in 2002 [ 104 ]. A further example of reporting bias in research on disorders of the digestive system is presented in Additional file 1 : Table S2.
Disorders of the genitourinary system/Perinatal medicine
Urinary incontinence.
Lenzer and Brownlee also reported cases of suicide in a trial investigating the selective serotonin and noradrenalin reuptake inhibitor duloxetine for a new indication, urinary incontinence in women. However, the FDA refused to release data on these cases, citing trade secrecy laws. These laws "permit companies to withhold all information, even deaths, about drugs that do not win approval for a new indication, even when the drug is already on the market for other indications" [ 94 ]. Two examples of reporting bias in perinatal research are presented in Additional file 1 : Table S2.
Disorders of the musculoskeletal system
Osteoarthritis.
In 2000, a trial comparing upper gastrointestinal toxicity of rofecoxib, a selective COX-2 inhibitor, and naproxen in over 8000 patients with rheumatoid arthritis reported that rofecoxib was associated with significantly fewer clinically important upper gastrointestinal events. The significantly lower myocardial infarction rate in the naproxen group was attributed to a cardioprotective effect of naproxen (VIGOR trial, [ 105 ]). Concerns about the risk of selective COX-2-inhibitor-related cardiovascular events were raised as early as 2001 [ 106 ], and in 2002, an analysis including previously unpublished data from FDA reports of the VIGOR trial showed a statistically significant increase of serious cardiovascular thrombotic events in patients using rofecoxib [ 107 ].
In their article on access to pharmaceutical data at the FDA, Lurie and Zieve presented the example of the selective COX-2 inhibitor celecoxib: in a journal publication of a trial investigating the gastrointestinal toxicity with celecoxib versus other pain medications, the study authors concluded that the drug was associated with a lower incidence of gastrointestinal ulcers after 6 months of therapy [ 108 , 109 ]. However, they failed to disclose that at the time of publication they had already received data for the full study period (12 months), which showed no advantage over the comparator drugs for the above outcome [ 109 ].
Disorders of the skin
Atopic dermatitis, evening primrose oil.
In his editorial "Evening primrose oil for atopic dermatitis - Time to say goodnight", Williams reported that he and his colleague, who had performed an individual patient meta-analysis of evening primrose oil for atopic dermatitis commissioned by the UK Department of Health, were not given permission to publish their report, which included 10 previously unpublished studies. After submission of the report to the Department of Health, Searle, the company then responsible for product marketing, required the authors and referees to sign a written statement that the contents of the report had not been leaked. Other research had not shown convincing evidence of a benefit, and in 2002 the UK Medicines Control Agency withdrew marketing authorisation [ 66 ].
Endocrine and metabolic disorders
Diabetes mellitus type 2.
- Rosiglitazone
The US cardiologist Steven Nissen commented on safety issues surrounding rosiglitazone, a thiazolidinedione used to treat type 2 diabetes. After the drug's approval, the FDA was informed in August 2005 by the manufacturer GSK that it had performed a meta-analysis of 42 randomized clinical trials of rosiglitazone, which suggested a 31% increase in the risk of ischaemic cardiovascular complications. GSK posted this finding on its website. However, neither GSK nor the FDA disseminated their findings in a broad way to the scientific community and the public [ 110 ]. The safety concerns were supported by a controversially discussed meta-analysis performed by Nissen and Wolski, who found that treatment with rosiglitazone was associated with a significantly increased risk of myocardial infarction and an increase in the risk of death from cardiovascular causes that had borderline significance [ 111 ]. More examples of reporting bias in diabetes research are presented in Additional file 1 : Table S2.
Hypercholesterolaemia
Ezetimibe and simvastatin.
In his article "Controversies surround heart drug study" Mitka described a trial that compared the 2 anticholesterol drugs ezetimibe and simvastatin versus simvastatin alone in patients with heterozygous familial hypercholesterolaemia [ 112 ]. No statistically significant difference between treatment groups was found for the primary outcome (mean change in the carotid intima-media thickness) after 2 years [ 113 ]. The trial, which was sponsored by Merck & Co. and Schering-Plough, was concluded in April 2006. A delay of almost 2 years in the reporting of results followed amidst allegations that the manufacturers had attempted to change the study endpoints prior to the publication of results [ 112 ]. A further case of reporting bias in research on ezetimibe is included in Additional file 1 : Table S2.
Cerivastatin
Psaty et al conducted a review of the published literature on the statin cerivastatin and also analysed internal company documents that became available during litigation procedures [ 114 ]. In the published literature, cerivastatin was associated with a substantially higher risk of rhabdomyolysis than other statins; this particularly referred to cerivastatin-gemfibrozil combination therapy. Cerivastatin was launched in the US in 1998 by Bayer, and within 3 to 4 months, internal documents indicated there had been multiple cases of cerivastatin-gemfibrozil interactions. However, it took more than 18 months until a contraindication about the concomitant use of the 2 drugs was added to the package insert. The unpublished data available in 1999 also suggested an association between high-dose cerivastatin monotherapy and rhabdomyolysis. In 1999/2000, the company analysed FDA adverse event reporting system data, which suggested that compared with atorvastatin, cerivastatin monotherapy substantially increased the risk of rhabdomyolysis. However, these findings were not disseminated or published. Cerivastatin was removed from the market in August 2001 [ 114 ]. In the same month, the German Ministry of Health accused Bayer of withholding vital information from its federal drug agency [ 115 ].
Thyroid disorders
Levothyroxine.
The Wall Street Journal reported the suppression of the results of a trial comparing the bioavailability of generic and brand-name levothyroxine products in the treatment of hypothyroidism; the investigators had concluded that the products were bioequivalent and in most cases interchangeable [ 116 , 117 ]. The trial was completed in 1990; over the next 7 years, the manufacturer of the brand-name product Synthroid, Boots pharmaceuticals, successfully delayed publication [ 65 ]. The manuscript was finally published in 1997.
Menopausal symptoms
A study investigating tibolone, a synthetic steroid, in breast-cancer patients with climacteric complaints was terminated prematurely after it was shown that this drug significantly increased the risk of cancer recurrence [ 118 ]. According to the German TV programme Frontal 21, the manufacturer (Schering-Plough, formerly NV Organon) informed regulatory authorities and ethics committees, as well as study centres and participants. However, the study results were not published until 1.5 years later [ 119 ].
Oncology is another area in which reporting bias is common [ 40 , 50 , 54 , 120 – 127 ]. A review of over 2000 oncology trials registered in ClinicalTrials.gov showed that less than 20% were available in PubMed, with substantial differences between trials sponsored by clinical trial networks and those sponsored by industry regarding both publication rates (59% vs. 6%) and the proportion of trials with positive results (50% vs. 75%) [ 50 ].
Ovarian cancer
Combination chemotherapy.
In one of the earliest publications measuring the effects of reporting bias, Simes compared published oncology trials and trials identified in cancer registries that investigated the survival impact of initial alkylating agent (AA) therapy versus combination chemotherapy (CC) in advanced ovarian cancer. A meta-analysis of the published trials showed a significant survival advantage for CC; however, no such advantage was shown in the meta-analysis of registered trials [ 121 ].
Multiple myeloma
The above study also investigated the survival impact of AA/prednisone versus CC in multiple myeloma. The meta-analysis of published trials demonstrated a significant survival advantage for CC. A survival benefit was also shown in the registered trials; however, the estimated magnitude of the benefit was reduced [ 121 ]. A further example of reporting bias in cancer research is presented in Additional file 1 : Table S2.
Disorders of the blood
Thalassaemia major, iron-chelation agents.
In his editorial "Thyroid storm", Rennie, among other things, discussed events surrounding a US researcher who had been involved in a trial investigating the effects of an oral iron-chelation agent in patients with thalassaemia major. She had initially published an optimistic article on the effects of this agent. However, further research showed a lack of effectiveness and a potential safety risk. She had signed a confidentiality agreement but, because of her concerns, decided to break confidentiality and report her results at a meeting; the manufacturer unsuccessfully attempted to block her presentation [ 128 ].
Bacterial, fungal, and viral infections
Oseltamivir.
The BMJ and Channel 4 News reported on the difficulties in obtaining data for an updated Cochrane review on neuraminidase inhibitors in influenza [ 129 ]. A previous analysis of oseltamivir, which was used in the prior Cochrane review [ 130 ], was based on 10 industry-sponsored trials of which only 2 had been published in peer-reviewed journals [ 131 ]. The manufacturer Roche initially declined to provide the necessary data to reproduce the analysis and then only provided a selection of files [ 129 ]. The Cochrane authors (Jefferson et al) subsequently concluded that "Evidence on the effects of oseltamivir in complications from lower respiratory tract infections, reported in our 2006 Cochrane review, may be unreliable" [ 132 ]. Roche has since agreed to provide public access to study summaries and password-protected access to the full study reports [ 129 ].
Anti-HIV agents
Ioannidis et al identified several examples of publication bias in trials investigating medications against HIV. At least 13 trials of 6 antiviral agents including at least 3779 patients had remained unpublished for more than 3 years from the time of their meeting presentation or completion. At least 9 of these trials had negative preliminary or final results. For example, 2 large negative isoprinosine trials were unpublished, whilst a positive trial had been published in a high impact journal [ 33 ]. Further examples of reporting bias in research on infections are presented in Additional file 1 : Table S2.
Acute trauma
Acute spinal cord injury, high-dose steroids.
Lenzer and Brownlee described the concerns of neurosurgeons regarding the use of high-dose steroids in patients with acute spinal cord injury. They noted that one neurosurgeon believed that several thousand patients had died as a result of this intervention; 2 surveys showed that many other neurosurgeons shared his concerns. The single available study, which had been funded by the NIH, was potentially flawed and several researchers had unsuccessfully lobbied for the release of the underlying data [ 94 ].
Human albumin infusion
In the UK Health Committee's 2004-2005 report on the influence of the pharmaceutical industry, Chalmers mentioned a systematic review of human albumin solution, which is used in the treatment of shock, e.g. in patients with burns. The results showed no evidence that albumin was helpful and suggested that this intervention may actually be harmful. Although the UK Medicines Control Agency subsequently slightly modified the labelling, it kept confidential the evidence upon which the drug had been re-licensed in 1993 [ 133 , 134 ].
Vaccinations
Hiv-1 vaccine.
McCarthy reported the case of an HIV-1 vaccine study that was terminated early when no difference in efficacy between the vaccine and placebo was found. After the lead investigators refused to include a post-hoc analysis arguing that it had not been part of the study protocol and that invalid statistical methods had been used, the manufacturer, Immune Response, filed an (unsuccessful) claim seeking to prevent publication. After publication, the manufacturer filed a claim against the study's lead investigators and their universities asking for US $7-10 million in damages [ 135 ].
Cancer vaccines
Rosenberg provided various examples of how researchers and companies withheld information on cancer vaccines for competitive reasons; for example, researchers were asked to keep information confidential that might have prevented cancer patients from receiving ineffective or even harmful doses of a new agent [ 75 ].
Other indications
Nocturnal leg cramps.
Man-Song-Hing et al performed a meta-analysis including unpublished individual patient data (IPD) obtained from the FDA on trials investigating quinine for the treatment of nocturnal leg cramps. They showed that the pooling only of published studies overestimated efficacy by more than 100% [ 136 ]. Further examples of reporting bias in other indications are presented in Additional file 1 : Table S2.
Further research areas
Reporting bias has also been shown in other research areas, such as genetics [ 137 , 138 ], effects of passive smoking [ 139 , 140 ] and nicotine [ 141 , 142 ], and effects of air pollution [ 143 ].
The numerous examples identified show that reporting bias concerns not only previously highlighted therapies such as antidepressants, pain medication, or cancer drugs, but affects a wide range of indications and interventions. Many cases involved the withholding of study data by manufacturers and regulatory agencies or the active attempt to suppress publication by manufacturers, which either resulted in substantial delays in publication (time-lag bias) or no publication at all.
Limitations of the review
The review does not provide a complete overview of reporting bias in clinical research. Although our efforts to identify relevant literature went beyond the usual efforts applied in narrative reviews, the review is non-systematic and we emphasized this feature in the title. A substantial amount of relevant literature was available in-house and further relevant literature was obtained by screening reference lists. We dispensed with our initial plan to conduct a systematic review to identify cases of reporting bias, as we noticed that many cases were not identifiable by screening titles and abstracts of citations from bibliographic databases, but were "hidden" in the discussion sections of journal articles or mentioned in other sources such as newspapers, books, government reports or websites. As a search of bibliographic databases and the Internet using keywords related to reporting bias produces thousands of potentially relevant hits, we would therefore have had to obtain and read an excessive amount of full texts in order to ensure that we had not missed any examples. This was not feasible due to resource limitations. However, within the framework of a previous publication [ 144 ] we had conducted a literature search in PubMed, and some of the citations retrieved formed the basis of our literature pool for the current review. In spite of this non-systematic approach, we were able to identify dozens of cases of reporting bias in numerous indications.
Another potential limitation of the review is the validity of the sources describing cases of reporting bias. Although the majority of examples were identified in peer-reviewed journals, several cases were based on information from other sources such as newspaper articles and websites. However, we also regard these sources to be valuable as they provide a broader overview of reporting bias beyond well-known examples and also offer a starting point for more systematic research on the additional examples identified.
Effects of reporting bias
Published evidence tends to overestimate efficacy and underestimate safety risks. The extent of misestimation is often unknown. The few identified comparisons that quantified overestimates of treatment effects in fully published versus unpublished or not fully published data showed wide variations in their results. Comparisons of pooled published versus pooled published and unpublished FDA data showed a greater treatment effect of 11% to 69% for individual antidepressants, 32% for the class of antidepressants [ 16 ], and over 100% for an agent to treat nocturnal leg cramps [ 136 ]. In addition, published studies have shown a 9% to 15% greater treatment effect than grey literature studies [ 145 , 146 ]. Thus, the conclusions of systematic reviews and meta-analyses based on published evidence alone may be misleading [ 5 , 7 , 38 ]. This is a serious concern as these documents are being used increasingly to support decision making in the health care system. Reporting bias may consequently result in inappropriate health care decisions by policy makers and clinicians, which harm patients, waste resources, and misguide future research [ 4 , 5 , 34 ].
Trial registration and public access to study data
There is an ethical obligation to publish research findings [ 120 , 147 – 150 ]. For example, patients who participate in clinical trials do so in the belief that they are contributing to medical progress, and this will only be the case if these trials are published. Deliberate non- or selective reporting represents unethical behaviour and scientific misconduct [ 34 , 147 ]. Public access to study data may also help identify safety problems at an earlier stage, which in the past have in some cases not always been detected by regulatory authorities [ 151 – 153 ]. Two concepts can help solve the issue of reporting bias: firstly, the mandatory and prospective registration of clinical trials, and secondly, the mandatory publication of full study results in results databases after study completion.
Non-industry initiatives
One of the first searchable computerized international registries of clinical trials was introduced in the United States in 1967; since then, several national and international trial registries have been created [ 154 ], such as the US government's trial registry and results database ClinicalTrials.gov (see Tse et al for an update on this registry [ 155 , 156 ]). The various controversies surrounding reporting bias, particularly the non-reporting of safety data, accelerated the movement both for trial registration and the establishment of results databases. Numerous researchers, organizations, regulatory and governmental authorities started various initiatives to achieve these goals [ 148 , 157 – 165 ].
In 2004, the International Committee of Medical Journal Editors (ICMJE) announced that it would make registration of clinical trials in a public registry a condition of consideration for publication [ 158 ]; this statement has since been updated [ 166 , 167 ].
In 2006, the WHO established the International Clinical Trials Registry Platform (ICTRP) in an initiative to bring national trial registries together in a global network providing a single point of access to registered trials [ 157 ]. However, to date no consensus has been found between the parties involved concerning which characteristics must be made publicly available at registration [ 168 ].
Section 801 of the US FDA Amendments Act 2007 (FDAAA, [ 169 ]) requires the registration at inception of all clinical trials involving a drug, biological product, or device regulated by the FDA. Trials must be registered on ClinicalTrials.gov and a defined set of results must be posted in the same registry within 12 months of study completion. Exceptions are phase I drug trials and early feasibility device trials. Non-compliance is sanctioned with monetary fines [ 163 , 170 ].
In 2004, the European Agency for the Evaluation of Medicinal Products (now European Medicines Agency) launched the European clinical trials database EudraCT (eudract.emea.europa.eu) to provide national authorities with a common set of information on clinical trials conducted in the EU. The database was initially supposed to be available only to the responsible authorities of the member states, as well as to the European Commission and the European Medicines Agency [ 171 ]. In 2006, the regulation on medicinal products for paediatric use was published, which required that information about European paediatric clinical trials of investigational medicinal products was to be made publicly available on EudraCT [ 172 , 173 ], and in February 2009, the European Commission published a guideline including the list of data fields to be made public [ 174 ]. On the same date, a similar list was published for all trials [ 175 ]. However, the legal obligation to publish information on trials in adults is not fully clear, and it is also unclear when all relevant information from EudraCT will be made publicly accessible.
With the introduction of the above-mentioned legislation, regulatory agencies are on the one hand helping to solve the problem of reporting bias, but on the other hand, they are also part of the problem: several of the examples identified refer to the non-publication or active withholding of study data by regulatory agencies [ 83 , 94 , 109 , 133 ]. This is partly due to existing confidentiality regulations such as Exemption 4 of the US Freedom of Information Act [ 176 ]. To solve the problems resulting from this situation, current legislation has to be changed to allow for the publication of comprehensive information on study methods and results by regulatory agencies. In his essay "A taxpayer-funded clinical trials registry and results database", Turner called for increased access to the FDA information sources, which would at least enable the assessment of drugs marketed in the USA [ 92 ]. Although the FDA posts selected reviews of NDAs on its website after the approval process following the Electronic Freedom of Information Act [ 177 ], the availability of these reviews is limited [ 92 ]. Moreover, according to the FDAAA, the results of older trials of approved drugs or of drugs that were never approved need not be disclosed [ 170 ], which is why a retrospective registry and results database is needed [ 178 ].
Industry initiatives
In 2002, the US Pharmaceutical Research and Manufacturers Association (PhRMA) member companies committed themselves to the registration of all hypothesis-testing clinical trials at initiation and to the timely disclosure of summary results, regardless of outcome [ 179 , 180 ]. PhRMA also launched the clinical study results database ClinicalStudyResults.org in 2004. In 2005, a similar commitment was made by several pharmaceutical industry associations [ 181 ], which has since been updated [ 182 ]. Following the legal settlement in the paroxetine case, GSK established a trial registry on its website gsk-clinicalstudyregister.com and other large companies have followed. In 2008, the German Association of Research-Based Pharmaceutical Companies (VFA) published a position paper on the issue of publication bias and claimed that, because of the voluntary self-commitment of the pharmaceutical industry and the introduction of legislation for the reporting of study data, publication bias had become a "historical" topic [ 183 ]. However, even after the update of the position paper in January 2009 [ 184 ], in Germany alone further attempts by drug companies to withhold study data have occurred [ 69 ], which shows that voluntary self-commitment is insufficient.
Conclusions
Reporting bias is widespread in the medical literature and has harmed patients in the past. Mandatory prospective registration of trials and public access to study data via results databases need to be introduced on a worldwide level. This would help fulfil ethical obligations towards patients by enabling proactive publication and independent reviews of clinical trial data, and ensure a basis for fully informed decision making in the health care system. Otherwise, clinical decision making based on the "best evidence" will remain an illusion.
Green S, Higgins S, editors: Glossary. Cochrane Handbook for Systematic Reviews of Interventions 4.2.5. Last update May 2005 [accessed 22 Feb 2010], http://www.cochrane.org/resources/handbook/
Sterne J, Egger M, Moher D: Addressing reporting biases. Cochrane handbook for systematic reviews of interventions. Edited by: Higgins JPT, Green S. 2008, Chichester: Wiley, 297-334. full_text.
Google Scholar
Dwan K, Altman DG, Arnaiz JA, Bloom J, Chan AW, Cronin E, Decullier E, Easterbrook PJ, Von Elm E, Gamble C, Ghersi D, Ioannidis JP, Simes J, Williamson PR: Systematic review of the empirical evidence of study publication bias and outcome reporting bias. PLoS ONE. 2008, 3: e3081-10.1371/journal.pone.0003081.
PubMed PubMed Central Google Scholar
Blumle A, Antes G, Schumacher M, Just H, Von Elm E: Clinical research projects at a German medical faculty: follow-up from ethical approval to publication and citation by others. J Med Ethics. 2008, 34: e20-10.1136/jme.2008.024521.
CAS PubMed Google Scholar
Von Elm E, Rollin A, Blumle A, Huwiler K, Witschi M, Egger M: Publication and non-publication of clinical trials: longitudinal study of applications submitted to a research ethics committee. Swiss Med Wkly. 2008, 138: 197-203.
PubMed Google Scholar
Dickersin K, Min YI, Meinert CL: Factors influencing publication of research results: follow-up of applications submitted to two institutional review boards. JAMA. 1992, 267: 374-378. 10.1001/jama.267.3.374.
Easterbrook PJ, Berlin JA, Gopalan R, Matthews DR: Publication bias in clinical research. Lancet. 1991, 337: 867-872. 10.1016/0140-6736(91)90201-Y.
Stern JM, Simes RJ: Publication bias: evidence of delayed publication in a cohort study of clinical research projects. BMJ. 1997, 315: 640-645.
CAS PubMed PubMed Central Google Scholar
Pich J, Carne X, Arnaiz JA, Gomez B, Trilla A, Rodes J: Role of a research ethics committee in follow-up and publication of results. Lancet. 2003, 361: 1015-1016. 10.1016/S0140-6736(03)12799-7.
Decullier E, Lheritier V, Chapuis F: Fate of biomedical research protocols and publication bias in France: retrospective cohort study. BMJ. 2005, 331: 19-24. 10.1136/bmj.38488.385995.8F.
Lee K, Bacchetti P, Sim I: Publication of clinical trials supporting successful new drug applications: a literature analysis. PLoS Med. 2008, 5: e191-10.1371/journal.pmed.0050191.
Hemminki E: Study of information submitted by drug companies to licensing authorities. Br Med J. 1980, 280: 833-836. 10.1136/bmj.280.6217.833.
MacLean CH, Morton SC, Ofman JJ, Roth EA, Shekelle PG: How useful are unpublished data from the Food and Drug Administration in meta-analysis?. J Clin Epidemiol. 2003, 56: 44-51. 10.1016/S0895-4356(02)00520-6.
Melander H, Ahlqvist-Rastad J, Meijer G, Beermann B: Evidence b(i)ased medicine: selective reporting from studies sponsored by pharmaceutical industry; review of studies in new drug applications. BMJ. 2003, 326: 1171-1173. 10.1136/bmj.326.7400.1171.
Benjamin DK, Smith PB, Murphy MD, Roberts R, Mathis L, Avant D, Califf RM, Li JS: Peer-reviewed publication of clinical trials completed for pediatric exclusivity. JAMA. 2006, 296: 1266-1273. 10.1001/jama.296.10.1266.
Turner EH, Matthews AM, Linardatos E, Tell RA, Rosenthal R: Selective publication of antidepressant trials and its influence on apparent efficacy. N Engl J Med. 2008, 358: 252-260. 10.1056/NEJMsa065779.
Bardy AH: Bias in reporting clinical trials. Br J Clin Pharmacol. 1998, 46: 147-150. 10.1046/j.1365-2125.1998.00759.x.
Ioannidis JP: Effect of the statistical significance of results on the time to completion and publication of randomized efficacy trials. JAMA. 1998, 279: 281-286. 10.1001/jama.279.4.281.
Hopewell S, Clarke M, Stewart L, Tierney J: Time to publication for results of clinical trials. Cochrane Database Syst Rev. 2007, MR000011-2
Institute for Quality and Efficiency in Health Care: General methods: version 3.0. Last update 27 May 2008 [accessed 22 Feb 2010], http://www.iqwig.de/download/IQWiG_General_methods_V-3-0.pdf
National Institute for Health and Clinical Excellence: Guide to the methods of technology appraisal. London. 2008
Scherer RW, Langenberg P, Von Elm E: Full publication of results initially presented in abstracts. Cochrane Database Syst Rev. 2007, MR000005-2
Altman D: Outcome reporting bias in meta-analyses. Last update 2007 [accessed 24 Feb 2010], http://www.chalmersresearch.com/bmg/docs/t2p1.pdf
Chan AW, Hrobjartsson A, Haahr MT, Gotzsche PC, Altman DG: Empirical evidence for selective reporting of outcomes in randomized trials: comparison of protocols to published articles. JAMA. 2004, 291: 2457-2465. 10.1001/jama.291.20.2457.
Chan AW, Altman DG: Identifying outcome reporting bias in randomised trials on PubMed: review of publications and survey of authors. BMJ. 2005, 330: 753-10.1136/bmj.38356.424606.8F.
Rising K, Bacchetti P, Bero L: Reporting bias in drug trials submitted to the Food and Drug Administration: review of publication and presentation. PLoS Med. 2008, 5: e217-10.1371/journal.pmed.0050217.
Chan AW, Krleza-Jeric K, Schmid I, Altman DG: Outcome reporting bias in randomized trials funded by the Canadian Institutes of Health Research. CMAJ. 2004, 171: 735-740.
Al-Marzouki S, Roberts I, Evans S, Marshall T: Selective reporting in clinical trials: analysis of trial protocols accepted by The Lancet. Lancet. 2008, 372: 201-10.1016/S0140-6736(08)61060-0.
Ioannidis JP, Lau J: Completeness of safety reporting in randomized trials: an evaluation of 7 medical areas. JAMA. 2001, 285: 437-443. 10.1001/jama.285.4.437.
Hazell L, Shakir SA: Under-reporting of adverse drug reactions: a systematic review. Drug Saf. 2006, 29: 385-396. 10.2165/00002018-200629050-00003.
Bonhoeffer J, Zumbrunn B, Heininger U: Reporting of vaccine safety data in publications: systematic review. Pharmacoepidemiol Drug Saf. 2005, 14: 101-106. 10.1002/pds.979.
Loke YK, Derry S: Reporting of adverse drug reactions in randomised controlled trials: a systematic survey. BMC Clin Pharmacol. 2001, 1: 3-10.1186/1472-6904-1-3.
Ioannidis JP, Cappelleri JC, Sacks HS, Lau J: The relationship between study design, results, and reporting of randomized clinical trials of HIV infection. Control Clin Trials. 1997, 18: 431-444. 10.1016/S0197-2456(97)00097-4.
Dickersin K: Reporting and other biases in studies of Neurontin for migraine, psychiatric/bipolar disorders, nociceptive pain, and neuropathic pain. Last update 10 Aug 2008 [accessed 26 Feb 2010], http://dida.library.ucsf.edu/pdf/oxx18r10
Nassir Ghaemi S, Shirzadi AA, Filkowski M: Publication bias and the pharmaceutical industry: the case of lamotrigine in bipolar disorder. Medscape J Med. 2008, 10: 211-
Sterling T: Publication decisions and their possible effects on inferences drawn from tests of significances. J Am Stat Assoc. 1959, 54: 30-34. 10.2307/2282137.
Hopewell S, Loudon K, Clarke MJ, Oxman AD, Dickersin K: Publication bias in clinical trials due to statistical significance or direction of trial results. Cochrane Database Syst Rev. 2009, MR000006-1
Song F, Eastwood AJ, Gilbody S, Duley L, Sutton AJ: Publication and related biases. Health Technol Assess. 2000, 4: 1-115.
Dickersin K, Chan S, Chalmers TC, Sacks HS, Smith H: Publication bias and clinical trials. Control Clin Trials. 1987, 8: 343-353. 10.1016/0197-2456(87)90155-3.
Krzyzanowska MK, Pintilie M, Tannock IF: Factors associated with failure to publish large randomized trials presented at an oncology meeting. JAMA. 2003, 290: 495-501. 10.1001/jama.290.4.495.
Timmer A, Hilsden RJ, Cole J, Hailey D, Sutherland LR: Publication bias in gastroenterological research: a retrospective cohort study based on abstracts submitted to a scientific meeting. BMC Med Res Methodol. 2002, 2: 7-10.1186/1471-2288-2-7.
Tramer MR, Reynolds DJ, Moore RA, McQuay HJ: Impact of covert duplicate publication on meta-analysis: a case study. BMJ. 1997, 315: 635-640.
Gotzsche PC: Reference bias in reports of drug trials. Br Med J (Clin Res Ed). 1987, 295: 654-656. 10.1136/bmj.295.6599.654.
CAS Google Scholar
Kjaergard LL, Gluud C: Citation bias of hepato-biliary randomized clinical trials. J Clin Epidemiol. 2002, 55: 407-410. 10.1016/S0895-4356(01)00513-3.
Ravnskov U: Quotation bias in reviews of the diet-heart idea. J Clin Epidemiol. 1995, 48: 713-719. 10.1016/0895-4356(94)00222-C.
Egger M, Zellweger-Zahner T, Schneider M, Junker C, Lengeler C, Antes G: Language bias in randomised controlled trials published in English and German. Lancet. 1997, 350: 326-329. 10.1016/S0140-6736(97)02419-7.
Lee KP, Boyd EA, Holroyd-Leduc JM, Bacchetti P, Bero LA: Predictors of publication: characteristics of submitted manuscripts associated with acceptance at major biomedical journals. Med J Aust. 2006, 184: 621-626.
Callaham ML, Wears RL, Weber EJ, Barton C, Young G: Positive-outcome bias and other limitations in the outcome of research abstracts submitted to a scientific meeting. JAMA. 1998, 280: 254-257. 10.1001/jama.280.3.254.
Lexchin J, Bero LA, Djulbegovic B, Clark O: Pharmaceutical industry sponsorship and research outcome and quality: systematic review. BMJ. 2003, 326: 1167-1170. 10.1136/bmj.326.7400.1167.
Ramsey S, Scoggins J: Commentary: practicing on the tip of an information iceberg? Evidence of underpublication of registered clinical trials in oncology. Oncologist. 2008, 13: 925-929. 10.1634/theoncologist.2008-0133.
Olson CM, Rennie D, Cook D, Dickersin K, Flanagin A, Hogan JW, Zhu Q, Reiling J, Pace B: Publication bias in editorial decision making. JAMA. 2002, 287: 2825-2828. 10.1001/jama.287.21.2825.
Okike K, Kocher MS, Mehlman CT, Heckman JD, Bhandari M: Publication bias in orthopaedic research: an analysis of scientific factors associated with publication in the Journal of Bone and Joint Surgery (American Volume). J Bone Joint Surg Am. 2008, 90: 595-601. 10.2106/JBJS.G.00279.
Dickersin K, Min YI: NIH clinical trials and publication bias. Online J Curr Clin Trials. 1993, Doc No 50:[4967 words; 4953 paragraphs].
Hartmann M, Knoth H, Schulz D, Knoth S: Industry-sponsored economic studies in oncology vs studies sponsored by nonprofit organisations. Br J Cancer. 2003, 89: 1405-1408. 10.1038/sj.bjc.6601308.
Bekelman JE, Li Y, Gross CP: Scope and impact of financial conflicts of interest in biomedical research: a systematic review. JAMA. 2003, 289: 454-465. 10.1001/jama.289.4.454.
Sismondo S: Pharmaceutical company funding and its consequences: a qualitative systematic review. Contemp Clin Trials. 2008, 29: 109-113. 10.1016/j.cct.2007.08.001.
Jorgensen AW, Hilden J, Gotzsche PC: Cochrane reviews compared with industry supported meta-analyses and other meta-analyses of the same drugs: systematic review. BMJ. 2006, 333: 782-10.1136/bmj.38973.444699.0B.
Liss H: Publication bias in the pulmonary/allergy literature: effect of pharmaceutical company sponsorship. Isr Med Assoc J. 2006, 8: 451-454.
Ridker PM, Torres J: Reported outcomes in major cardiovascular clinical trials funded by for-profit and not-for-profit organizations: 2000-2005. JAMA. 2006, 295: 2270-2274. 10.1001/jama.295.19.2270.
Als-Nielsen B, Chen W, Gluud C, Kjaergard LL: Association of funding and conclusions in randomized drug trials: a reflection of treatment effect or adverse events?. JAMA. 2003, 290: 921-928. 10.1001/jama.290.7.921.
Perlis CS, Harwood M, Perlis RH: Extent and impact of industry sponsorship conflicts of interest in dermatology research. J Am Acad Dermatol. 2005, 52: 967-971. 10.1016/j.jaad.2005.01.020.
Bhandari M, Busse JW, Jackowski D, Montori VM, Schünemann H, Sprague S, Mears D, Schemitsch EH, Heels-Ansdell D, Devereaux PJ: Association between industry funding and statistically significant pro-industry findings in medical and surgical randomized trials. CMAJ. 2004, 170: 477-480.
Kjaergard LL, Als-Nielsen B: Association between competing interests and authors' conclusions: epidemiological study of randomised clinical trials published in the BMJ. BMJ. 2002, 325: 249-10.1136/bmj.325.7358.249.
Lauritsen K, Havelund T, Laursen LS, Rask-Madsen J: Withholding unfavourable results in drug company sponsored clinical trials. Lancet. 1987, 1: 1091-10.1016/S0140-6736(87)90515-0.
Wise J: Research suppressed for seven years by drug company. BMJ. 1997, 314: 1145-
Williams HC: Evening primrose oil for atopic dermatitis. BMJ. 2003, 327: 1358-1359. 10.1136/bmj.327.7428.1358.
Henry DA, Kerridge IH, Hill SR, McNeill PM, Doran E, Newby DA, Henderson KM, Maguire J, Stokes BJ, Macdonald GJ, Day RO: Medical specialists and pharmaceutical industry-sponsored research: a survey of the Australian experience. Med J Aust. 2005, 182: 557-560.
Gotzsche PC, Hrobjartsson A, Johansen HK, Haahr MT, Altman DG, Chan AW: Constraints on publication rights in industry-initiated clinical trials. JAMA. 2006, 295: 1645-1646. 10.1001/jama.295.14.1645.
Stafford N: German agency refuses to rule on drug's benefits until Pfizer discloses all trial results. BMJ. 2009, 338: b2521-10.1136/bmj.b2521.
Whittington CJ, Kendall T, Fonagy P, Cottrell D, Cotgrove A, Boddington E: Selective serotonin reuptake inhibitors in childhood depression: systematic review of published versus unpublished data. Lancet. 2004, 363: 1341-1345. 10.1016/S0140-6736(04)16043-1.
Cowley AJ, Skene A, Stainer K, Hampton JR: The effect of lorcainide on arrhythmias and survival in patients with acute myocardial infarction: an example of publication bias. Int J Cardiol. 1993, 40: 161-166. 10.1016/0167-5273(93)90279-P.
Moore TJ: Deadly medicine: why tens of thousands of heart patients died in America's worst drug disaster. 1995, New York: Simon & Schuster
Mathews A, Martinez B: E-mails suggest Merck knew Vioxx's dangers at early stage. Wall Street Journal. 2004, A1-
Psaty BM, Kronmal RA: Reporting mortality findings in trials of rofecoxib for Alzheimer disease or cognitive impairment: a case study based on documents from rofecoxib litigation. JAMA. 2008, 299: 1813-1817. 10.1001/jama.299.15.1813.
Rosenberg SA: Secrecy in medical research. N Engl J Med. 1996, 334: 392-394. 10.1056/NEJM199602083340610.
Baker CB, Johnsrud MT, Crismon ML, Rosenheck RA, Woods SW: Quantitative analysis of sponsorship bias in economic studies of antidepressants. Br J Psychiatry. 2003, 183: 498-506. 10.1192/bjp.183.6.498.
Moncrieff J: Clozapine v. conventional antipsychotic drugs for treatment-resistant schizophrenia: a re-examination. Br J Psychiatry. 2003, 183: 161-166. 10.1192/bjp.183.2.161.
Montgomery JH, Byerly M, Carmody T, Li B, Miller DR, Varghese F, Holland R: An analysis of the effect of funding source in randomized clinical trials of second generation antipsychotics for the treatment of schizophrenia. Control Clin Trials. 2004, 25: 598-612. 10.1016/j.cct.2004.09.002.
Procyshyn RM, Chau A, Fortin P, Jenkins W: Prevalence and outcomes of pharmaceutical industry-sponsored clinical trials involving clozapine, risperidone, or olanzapine. Can J Psychiatry. 2004, 49: 601-606.
Perlis RH, Perlis CS, Wu Y, Hwang C, Joseph M, Nierenberg AA: Industry sponsorship and financial conflict of interest in the reporting of clinical trials in psychiatry. Am J Psychiatry. 2005, 162: 1957-1960. 10.1176/appi.ajp.162.10.1957.
Heres S, Davis J, Maino K, Jetzinger E, Kissling W, Leucht S: Why olanzapine beats risperidone, risperidone beats quetiapine, and quetiapine beats olanzapine: an exploratory analysis of head-to-head comparison studies of second-generation antipsychotics. Am J Psychiatry. 2006, 163: 185-194. 10.1176/appi.ajp.163.2.185.
Kelly RE, Cohen LJ, Semple RJ, Bialer P, Lau A, Bodenheimer A, Neustadter E: Relationship between drug company funding and outcomes of clinical psychiatric research. Psychol Med. 2006, 36: 1647-1656. 10.1017/S0033291706008567.
Kirsch I, Deacon BJ, Huedo-Medina TB, Scoboria A, Moore TJ, Johnson BT: Initial severity and antidepressant benefits: a meta-analysis of data submitted to the Food and Drug Administration. PLoS Med. 2008, 5: e45-10.1371/journal.pmed.0050045.
Office of the Attorney General: Major pharmaceutical firm concealed drug information. Last update 02 Jun 2004 [accessed 24 Feb 2010], http://www.oag.state.ny.us/media_center/2004/jun/jun2b_04.html
Office of the Attorney General: Settlement sets new standard for release of drug information. Last update 26 Aug 2004 [accessed 26 Feb 2010], http://www.oag.state.ny.us/media_center/2004/aug/aug26a_04.html
Gibson L: GlaxoSmithKline to publish clinical trials after US lawsuit. BMJ. 2004, 328: 1513-10.1136/bmj.328.7455.1513-a.
Institute for Quality and Efficiency in Health Care: Bupropion, mirtazapine and reboxetine in the treatment of depression: executive summary of preliminary report; commission no A05-20C. Last update 29 May 2009 [accessed 26 Feb 2010], http://www.iqwig.de/download/A05-20C_Executive_summary_Bupropion_mirtazapine_and_reboxetine_in_the_treatment_of_depression.pdf
Institute for Quality and Efficiency in Health Care: Antidepressants: benefit of reboxetine not proven. Last update 24 Nov 2009 [accessed 26 Feb 2010], http://www.iqwig.de/antidepressants-benefit-of-reboxetine-not-proven.981.en.html
Abramson J: Expert report. Last update 11 Aug 2008 [accessed 26 Feb 2010], http://dida.library.ucsf.edu/pdf/oxx18v10
Vedula SS, Bero L, Scherer RW, Dickersin K: Outcome reporting in industry-sponsored trials of gabapentin for off-label use. N Engl J Med. 2009, 361: 1963-1971. 10.1056/NEJMsa0906126.
Vedantam S: A silenced drug study creates an uproar. Washington Post. 2009, A01-
Turner EH: A taxpayer-funded clinical trials registry and results database. PLoS Med. 2004, 1: e60-10.1371/journal.pmed.0010060.
Singh D: Merck withdraws arthritis drug worldwide. BMJ. 2004, 329: 816-10.1136/bmj.329.7470.816-a.
Lenzer J, Brownlee S: An untold story?. BMJ. 2008, 336: 532-534. 10.1136/bmj.39504.662685.0F.
Waknine Y: Bextra withdrawn from market. Medscape Today [Online]. 2005, http://www.medscape.com/viewarticle/502642
Hiatt WR: Observational studies of drug safety--aprotinin and the absence of transparency. N Engl J Med. 2006, 355: 2171-2173. 10.1056/NEJMp068252.
Tuffs A: Bayer withdraws heart surgery drug. BMJ. 2007, 335: 1015-10.1136/bmj.39395.644826.DB.
Furberg CD: Effect of antiarrhythmic drugs on mortality after myocardial infarction. Am J Cardiol. 1983, 52: 32C-36C. 10.1016/0002-9149(83)90629-X.
Antes G: Tödliche Medizin. Unpublizierte Studien - harmlos? [Fatal medicine. Unpublished studies - harmless?]. MMW Fortschr Med. 2006, 148: 8-
Hine LK, Laird N, Hewitt P, Chalmers TC: Meta-analytic evidence against prophylactic use of lidocaine in acute myocardial infarction. Arch Intern Med. 1989, 149: 2694-2698. 10.1001/archinte.149.12.2694.
MacMahon S, Collins R, Peto R, Koster RW, Yusuf S: Effects of prophylactic lidocaine in suspected acute myocardial infarction: an overview of results from the randomized, controlled trials. JAMA. 1988, 260: 1910-1916. 10.1001/jama.260.13.1910.
Preliminary report: effect of encainide and flecainide on mortality in a randomized trial of arrhythmia suppression after myocardial infarction. The Cardiac Arrhythmia Suppression Trial (CAST) Investigators. N Engl J Med. 1989, 321: 406-412.
Barbehenn E, Lurie P, Wolfe SM: Alosetron for irritable bowel syndrome. Lancet. 2000, 356: 2009-2010. 10.1016/S0140-6736(05)72978-0.
Moynihan R: Alosetron: a case study in regulatory capture, or a victory for patients' rights?. BMJ. 2002, 325: 592-595. 10.1136/bmj.325.7364.592.
Bombardier C, Laine L, Reicin A, Shapiro D, Burgos-Vargas R, Davis B, Day R, Ferraz MB, Hawkey CJ, Hochberg MC, Kvien TK, Schnitzer TJ, VIGOR Study Group: Comparison of upper gastrointestinal toxicity of rofecoxib and naproxen in patients with rheumatoid arthritis. N Engl J Med. 2000, 343: 1520-1528. 10.1056/NEJM200011233432103.
Mukherjee D, Nissen SE, Topol EJ: Risk of cardiovascular events associated with selective COX-2 inhibitors. JAMA. 2001, 286: 954-959. 10.1001/jama.286.8.954.
McCormack JP, Rangno R: Digging for data from the COX-2 trials. CMAJ. 2002, 166: 1649-1650.
Silverstein FE, Faich G, Goldstein JL, Simon LS, Pincus T, Whelton A, Makuch R, Eisen G, Agrawal NM, Stenson WF, Burr AM: Gastrointestinal toxicity with celecoxib vs nonsteroidal anti-inflammatory drugs for osteoarthritis and rheumatoid arthritis: the CLASS study: a randomized controlled trial. JAMA. 2000, 284: 1247-1255. 10.1001/jama.284.10.1247.
Lurie P, Zieve A: Sometimes the silence can be like the thunder: access to pharmaceutical data at the FDA. Law Contemp Probl. 2008, 69: 85-97.
Nissen S, Califf R: A conversation about rosiglitazone. Medscape Diabetes & Endocrinology [Online]. 2007, http://www.medscape.com/viewarticle/561666
Nissen SE, Wolski K: Effect of rosiglitazone on the risk of myocardial infarction and death from cardiovascular causes. N Engl J Med. 2007, 356: 2457-2471. 10.1056/NEJMoa072761.
Mitka M: Controversies surround heart drug study: questions about Vytorin and trial sponsors' conduct. JAMA. 2008, 299: 885-887. 10.1001/jama.299.8.885.
Kastelein JJ, Akdim F, Stroes ES, Zwinderman AH, Bots ML, Stalenhoef AF, Visseren FL, Sijbrands EJ, Trip MD, Stein EA, Duivenvoorden R, Veltri EP, Marais AD, de Groot E, ENHANCE Investigators: Simvastatin with or without ezetimibe in familial hypercholesterolemia. N Engl J Med. 2008, 358: 1431-1443. 10.1056/NEJMoa0800742.
Psaty BM, Furberg CD, Ray WA, Weiss NS: Potential for conflict of interest in the evaluation of suspected adverse drug reactions: use of cerivastatin and risk of rhabdomyolysis. JAMA. 2004, 292: 2622-2631. 10.1001/jama.292.21.2622.
Tuffs A: Bayer faces potential fine over cholesterol lowering drug. BMJ. 2001, 323: 415-10.1136/bmj.323.7310.415.
King RT: Bitter pill: how a drug firm paid for university study, then undermined it. Wall Street Journal. 1996, 1: A13-
Dong BJ, Hauck WW, Gambertoglio JG, Gee L, White JR, Bubp JL, Greenspan FS: Bioequivalence of generic and brand-name levothyroxine products in the treatment of hypothyroidism. JAMA. 1997, 277: 1205-1213. 10.1001/jama.277.15.1205.
Kenemans P, Bundred NJ, Foidart JM, Kubista E, von Schoultz B, Sismondi P, Vassilopoulou-Sellin R, Yip CH, Egberts J, Mol-Arts M, Mulder R, van Os S, Beckmann MW, LIBERATE Study Group: Safety and efficacy of tibolone in breast-cancer patients with vasomotor symptoms: a double-blind, randomised, non-inferiority trial. Lancet Oncol. 2009, 10: 135-146. 10.1016/S1470-2045(08)70341-3.
Lippegaus O, Prokscha S, Thimme C: Verharmloste Gefahren. Krebs durch Hormonbehandlung [Trivialised dangers. Cancer caused by hormone therapy]. Last update 2009 [accessed 26 Feb 2010], http://frontal21.zdf.de/ZDFde/inhalt/11/0,1872,7593675,00.html
Doroshow JH: Commentary: publishing cancer clinical trial results: a scientific and ethical imperative. Oncologist. 2008, 13: 930-932. 10.1634/theoncologist.2008-0168.
Simes RJ: Publication bias: the case for an international registry of clinical trials. J Clin Oncol. 1986, 4: 1529-1541.
Takeda A, Loveman E, Harris P, Hartwell D, Welch K: Time to full publication of studies of anti-cancer medicines for breast cancer and the potential for publication bias: a short systematic review. Health Technol Assess. 2008, 12: iii-x. 1-46
Peppercorn J, Blood E, Winer E, Partridge A: Association between pharmaceutical involvement and outcomes in breast cancer clinical trials. Cancer. 2007, 109: 1239-1246. 10.1002/cncr.22528.
Kyzas PA, Loizou KT, Ioannidis JP: Selective reporting biases in cancer prognostic factor studies. J Natl Cancer Inst. 2005, 97: 1043-1055.
Begg CB, Pocock SJ, Freedman L, Zelen M: State of the art in comparative cancer clinical trials. Cancer. 1987, 60: 2811-2815. 10.1002/1097-0142(19871201)60:11<2811::AID-CNCR2820601136>3.0.CO;2-P.
Kyzas PA, Denaxa-Kyza D, Ioannidis JP: Almost all articles on cancer prognostic markers report statistically significant results. Eur J Cancer. 2007, 43: 2559-2579.
Manheimer E, Anderson D: Survey of public information about ongoing clinical trials funded by industry: evaluation of completeness and accessibility. BMJ. 2002, 325: 528-531. 10.1136/bmj.325.7363.528.
Rennie D: Thyroid storm. JAMA. 1997, 277: 1238-1243. 10.1001/jama.277.15.1238.
Godlee F, Clarke M: Why don't we have all the evidence on oseltamivir?. BMJ. 2009, 339: b5351-10.1136/bmj.b5351.
Jefferson TO, Demicheli V, Di Pietrantonj C, Jones M, Rivetti D: Neuraminidase inhibitors for preventing and treating influenza in healthy adults. Cochrane Database Syst Rev. 2006, 3: CD001265-
Kaiser L, Wat C, Mills T, Mahoney P, Ward P, Hayden F: Impact of oseltamivir treatment on influenza-related lower respiratory tract complications and hospitalizations. Arch Intern Med. 2003, 163: 1667-1672. 10.1001/archinte.163.14.1667.
Jefferson T, Jones M, Doshi P, Del Mar C: Neuraminidase inhibitors for preventing and treating influenza in healthy adults: systematic review and meta-analysis. BMJ. 2009, 339: b5106-10.1136/bmj.b5106.
The influence of the pharmaceutical industry; formal minutes, oral and written evidence. 2005, London: Stationery Office, 2: [House of Commons, Health Committee (Series Editor): Report of session 2004-05; vol 4]
Cochrane Injuries Group Albumin Reviewers: Human albumin administration in critically ill patients: systematic review of randomised controlled trials. BMJ. 1998, 317: 235-240.
McCarthy M: Company sought to block paper's publication. Lancet. 2000, 356: 1659-10.1016/S0140-6736(00)03166-4.
Man-Son-Hing M, Wells G, Lau A: Quinine for nocturnal leg cramps: a meta-analysis including unpublished data. J Gen Intern Med. 1998, 13: 600-606. 10.1046/j.1525-1497.1998.00182.x.
Marshall E: Is data-hoarding slowing the assault on pathogens?. Science. 1997, 275: 777-780. 10.1126/science.275.5301.777.
Campbell EG, Clarridge BR, Gokhale M, Birenbaum L, Hilgartner S, Holtzman NA, Blumenthal D: Data withholding in academic genetics: evidence from a national survey. JAMA. 2002, 287: 473-480. 10.1001/jama.287.4.473.
Misakian AL, Bero LA: Publication bias and research on passive smoking: comparison of published and unpublished studies. JAMA. 1998, 280: 250-253. 10.1001/jama.280.3.250.
Barnes DE, Bero LA: Why review articles on the health effects of passive smoking reach different conclusions. JAMA. 1998, 279: 1566-1570. 10.1001/jama.279.19.1566.
Hilts PJ: Philip Morris blocked paper showing addiction, panel finds. New York Times. 1994, A7-
Hilts PJ: Scientists say Philip Morris withheld nicotine findings. New York Times. 1994, A1-A7.
Anderson HR, Atkinson RW, Peacock JL, Sweeting MJ, Marston L: Ambient particulate matter and health effects: publication bias in studies of short-term associations. Epidemiology. 2005, 16: 155-163. 10.1097/01.ede.0000152528.22746.0f.
Peinemann F, McGauran N, Sauerland S, Lange S: Negative pressure wound therapy: potential publication bias caused by lack of access to unpublished study results data. BMC Med Res Methodol. 2008, 8: 4-10.1186/1471-2288-8-4.
McAuley L, Pham B, Tugwell P, Moher D: Does the inclusion of grey literature influence estimates of intervention effectiveness reported in meta-analyses?. Lancet. 2000, 356: 1228-1231. 10.1016/S0140-6736(00)02786-0.
Hopewell S, McDonald S, Clarke M, Egger M: Grey literature in meta-analyses of randomized trials of health care interventions. Cochrane Database Syst Rev. 2007, MR000010-2
Chalmers I: Underreporting research is scientific misconduct. JAMA. 1990, 263: 1405-1408. 10.1001/jama.263.10.1405.
World Medical Association: Declaration of Helsinki: ethical principles for medical research involving human subjects. Last update Oct 2008 [accessed 26 Feb 2010], http://www.wma.net/en/30publications/10policies/b3/index.html
Pearn J: Publication: an ethical imperative. BMJ. 1995, 310: 1313-1315.
The Nuremberg code. Trials of war criminals before the Nuremberg Military Tribunals under Control Council Law no10. 1949, Washington, D.C.: US Government Printing Office, 2: 181-182.
Healy D: Did regulators fail over selective serotonin reuptake inhibitors?. BMJ. 2006, 333: 92-95. 10.1136/bmj.333.7558.92.
Topol EJ: Failing the public health: rofecoxib, Merck, and the FDA. N Engl J Med. 2004, 351: 1707-1709. 10.1056/NEJMp048286.
Rennie D: When evidence isn't: trials, drug companies and the FDA. J Law Policy. 2007, 15: 991-1012.
Dickersin K, Rennie D: Registering clinical trials. JAMA. 2003, 290: 516-523. 10.1001/jama.290.4.516.
Tse T, Williams RJ, Zarin DA: Update on Registration of Clinical Trials in ClinicalTrials.gov. Chest. 2009, 136: 304-305. 10.1378/chest.09-1219.
Tse T, Williams RJ, Zarin DA: Reporting "basic results" in ClinicalTrials.gov. Chest. 2009, 136: 295-303. 10.1378/chest.08-3022.
WHO clinical trials initiative to protect the public. Bull World Health Organ. 2006, 84: 10-11.
De Angelis C, Drazen JM, Frizelle FA, Haug C, Hoey J, Horton R, Kotzin S, Laine C, Marusic A, Overbeke AJ, Schroeder TV, Sox HC, Weyden Van Der MB, International Committee of Medical Journal Editors: Clinical trial registration: a statement from the International Committee of Medical Journal Editors. N Engl J Med. 2004, 351: 1250-1251. 10.1056/NEJMe048225.
Deutsches Cochrane Zentrum, Deutsches Netzwerk Evidenzbasierte Medizin: Stellungnahme [Comment]. Last update 22 Sep 2004 [accessed 26 Feb 2010], http://www.ebm-netzwerk.de/netzwerkarbeit/images/stellungnahme_anhoerung_probandenschutz.pdf
Krleza-Jeric K: International dialogue on the Public Reporting Of Clinical Trial Outcome and Results: PROCTOR meeting. Croat Med J. 2008, 49: 267-268. 10.3325/cmj.2008.2.267.
Krleza-Jeric K, Chan AW, Dickersin K, Sim I, Grimshaw J, Gluud C: Principles for international registration of protocol information and results from human trials of health related interventions: Ottawa statement (part 1). BMJ. 2005, 330: 956-958. 10.1136/bmj.330.7497.956.
European Research Council: ERC Scientific Council guidelines on open access. Last update 17 Dec 2007 [accessed 25 Feb 2010], http://erc.europa.eu/pdf/ScC_Guidelines_Open_Access_revised_Dec07_FINAL.pdf
Groves T: Mandatory disclosure of trial results for drugs and devices. BMJ. 2008, 336: 170-10.1136/bmj.39469.465139.80.
Steinbrook R: Public access to NIH-funded research. N Engl J Med. 2005, 352: 1739-1741. 10.1056/NEJMp058088.
Dickersin K: Report from the Panel on the Case for Registers of Clinical Trials at the Eighth Annual Meeting of the Society for Clinical Trials. Control Clin Trials. 1988, 9: 76-81.
De Angelis CD, Drazen JM, Frizelle FA, Haug C, Hoey J, Horton R, Kotzin S, Laine C: Is this clinical trial fully registered? A statement from the International Committee of Medical Journal Editors. N Engl J Med. 2005, 352: 2436-2438. 10.1056/NEJMe058127.
Laine C, Horton R, DeAngelis CD, Drazen JM, Frizelle FA, Godlee F, Haug C, Hebert PC, Kotzin S, Marusic A, Sahni P, Schroeder TV, Sox HC, Weyden Van der MB, Verheugt FW: Clinical trial registration--looking back and moving ahead. N Engl J Med. 2007, 356: 2734-2736. 10.1056/NEJMe078110.
Krleza-Jeric K: Clinical trial registration: the differing views of industry, the WHO, and the Ottawa Group. PLoS Med. 2005, 2: e378-10.1371/journal.pmed.0020378.
Food and Drug Administration: FDA Amendments Act (FDAAA) of 2007, public law no. 110-85 §801. Last update 2007 [accessed 26 Feb 2010], http://frwebgate.access.gpo.gov/cgi-bin/getdoc.cgi?dbname=110_cong_public_laws%26docid=f:publ085.110.pdf
Wood AJJ: Progress and deficiencies in the registration of clinical trials. N Engl J Med. 2009, 360: 824-830. 10.1056/NEJMsr0806582.
European Medicines Agency: EMEA launches EudraCT database. Last update 06 May 2004 [accessed 25 Feb 2010], http://www.emea.europa.eu/pdfs/general/direct/pr/1258904en.pdf
Smyth RL: Making information about clinical trials publicly available. BMJ. 2009, 338: b2473-10.1136/bmj.b2473.
Regulation (EC) No 1901/2006 of the European Parliament and of the Council of 12 December 2006 on medicinal products for paediatric use and amending regulation (EEC) no 1768/92, directive 2001/20/EC, directive 2001/83/EC and regulation (EC) No 726/2004. Official J Eur Commun. 2006, 49: L378/1-L378/19.
European Commission: List of fields to be made public from EudraCT for paediatric clinical trials in accordance with article 41 of regulation (EC) no 1901/2006 and its implementing guideline 2009/C28/01. Last update 26 Mar 2009 [accessed 26 Feb 2010], http://ec.europa.eu/enterprise/pharmaceuticals/eudralex/vol-10/2009_02_04_guidelines_paed.pdf
European Commission: List of fields contained in the 'EudraCT' clinical trials database to be made public, in accordance with Article 57(2) of Regulation (EC) No 726/2004 and its implementing guideline 2008/c168/021. Last update 04 Feb 2009 [accessed 25 Feb 2010], http://ec.europa.eu/enterprise/pharmaceuticals/eudralex/vol-10/2009_02_04_guideline.pdf
Committee on Government Reform: A citizen's guide on using the Freedom of Information Act and the Privacy Act of 1974 to request government records. Last update 20 Sep 2005 [accessed 26 Feb 2010], http://www.fas.org/sgp/foia/citizen.pdf
Food and Drug Administration: Executive summary of the Food and Drug Administration's consumer roundtable on consumer protection priorities. Last update 2000 [accessed 26 Feb 2010], http://www.fda.gov/ohrms/dockets/dockets/00n_1665/cr00001.pdf
Turner EH: Closing a loophole in the FDA Amendments Act. Science. 2008, 322: 44-46. 10.1126/science.322.5898.44c.
Pharmaceutical Research and Manufacturers of America: PhRMA clinical trial registry proposal. Last update 2010 [accessed 26 Feb 2010], http://www.phrma.org/node/446
Principles on the conduct of clinical trials and communication of clinical trial results. 2002, Washington DC: Pharmaceutical Research and Manufacturers of America
International Federation of Pharmaceutical Manufacturers & Associations: Joint position on the disclosure of clinical trial information via clinical trial registries and databases. Last update 2005 [accessed 24 Feb 2010], http://www.phrma.org/files/attachments/2005-01-06.1113.PDF
International Federation of Pharmaceutical Manufacturers & Associations: Joint position on the disclosure of clinical trial information via clinical trial registries and databases. Last update Nov 2008 [accessed 11 Mar 2010], http://www.ifpma.org/pdf/Revised_Joint_Industry_Position_26Nov08.pdf
Verband Forschender Arzneimittelhersteller: VFA-Positionspapier zum Thema "publication bias" [VFA position paper on the subject of "publication bias"]. 2008, Berlin: VFA
Verband Forschender Arzneimittelhersteller: VFA-Positionspapier zum Thema "publication bias" [VFA position paper on the subject of "publication bias"]. Last update Jan 2009 [accessed 26 Feb 2010], http://www.vfa.de/download/SAVE/de/presse/positionen/pos-publication-bias.html/pos-publication-bias.pdf
Mathew SJ, Charney DS: Publication bias and the efficacy of antidepressants. Am J Psychiatry. 2009, 166: 140-145. 10.1176/appi.ajp.2008.08071102.
Abbott A: British panel bans use of antidepressant to treat children. Nature. 2003, 423: 792-
Mitka M: FDA alert on antidepressants for youth. JAMA. 2003, 290: 2534-10.1001/jama.290.19.2534.
Garland EJ: Facing the evidence: antidepressant treatment in children and adolescents. CMAJ. 2004, 170: 489-491.
Herxheimer A, Mintzes B: Antidepressants and adverse effects in young patients: uncovering the evidence. CMAJ. 2004, 170: 487-489.
Dyer O: GlaxoSmithKline faces US lawsuit over concealment of trial results. BMJ. 2004, 328: 1395-10.1136/bmj.328.7453.1395.
Jureidini JN, McHenry LB, Mansfield PR: Clinical trials and drug promotion: selective reporting of study 329. Int J Risk Safety Med. 2008, 73-81.
Institute for Quality and Efficiency in Health Care: Preliminary report on antidepressants published. Last update 10 Jun 2009 [accessed 26 Feb 2010], http://www.iqwig.de/index.867.en.html
Steinman MA, Bero LA, Chren MM, Landefeld CS: Narrative review: the promotion of gabapentin: an analysis of internal industry documents. Ann Intern Med. 2006, 145: 284-293.
Steinman MA, Harper GM, Chren MM, Landefeld CS, Bero LA: Characteristics and impact of drug detailing for gabapentin. PLoS Med. 2007, 4: e134-10.1371/journal.pmed.0040134.
Landefeld CS, Steinman MA: The Neurontin legacy: marketing through misinformation and manipulation. N Engl J Med. 2009, 360: 103-106. 10.1056/NEJMp0808659.
Mack A: Examination of the evidence for off-label use of gabapentin. J Manag Care Pharm. 2003, 9: 559-568.
Petersen M: Memos cast shadow on drug's promotion. New York Times. 2002, C2-
U.S. Department of Justice: Warner-Lambert to pay $430 million to resolve criminal & civil health care liability relating to off-label promotion. Last update 13 May 2004 [accessed 13 Mar 2010], http://www.usdoj.gov/opa/pr/2004/May/04_civ_322.htm
Feeley J, Cronin Fisk M: AstraZeneca Seroquel studies 'buried,' papers show (update 3). Last update 27 Feb 2009 [accessed 19 Mar 2010], http://www.bloomberg.com/apps/news?pid=20601087%26sid=aS_.NqzMArG8#
Milford P: AstraZeneca may link Seroquel, diabetes, doctor says (update 1). Last update 11 Mar 2009 [accessed 19 Mar 2010], http://www.bloomberg.com/apps/news?pid=newsarchive%26sid=ayzJsK2HlF6s
Whalen J: AstraZeneca chalks up Seroquel dismissal in State Court. Wall Street Journal Health Blog [Online]. 2009, http://blogs.wsj.com/health/2009/06/10/astrazeneca-chalks-up-seroquel-dismissal-in-state-court
Kapczinski F, Lima MS, Souza JS, Schmitt R: Antidepressants for generalized anxiety disorder. Cochrane Database Syst Rev. 2003, CD003592-2
Bang LM, Keating GM: Paroxetine controlled release. CNS Drugs. 2004, 18: 355-364. 10.2165/00023210-200418060-00003.
Lenzer J: NIH secrets: study break. Last update 19.10.2006 [accessed 13 Mar 2010], http://www.ahrp.org/cms/index2.php?option=com_content%26do_pdf=1%26id=398
Ray WA, Stein CM, Daugherty JR, Hall K, Arbogast PG, Griffin MR: COX-2 selective non-steroidal anti-inflammatory drugs and risk of serious coronary heart disease. Lancet. 2002, 360: 1071-1073. 10.1016/S0140-6736(02)11131-7.
Juni P, Nartey L, Reichenbach S, Sterchi R, Dieppe PA, Egger M: Risk of cardiovascular events and rofecoxib: cumulative meta-analysis. Lancet. 2004, 364: 2021-2029. 10.1016/S0140-6736(04)17514-4.
Curfman GD, Morrissey S, Drazen JM: Expression of concern: Bombardier et al., "Comparison of upper gastrointestinal toxicity of rofecoxib and naproxen in patients with rheumatoid arthritis," N Engl J Med 2000;343:1520-8. N Engl J Med. 2005, 353: 2813-2814. 10.1056/NEJMe058314.
Waxman HA: The lessons of Vioxx: drug safety and sales. N Engl J Med. 2005, 352: 2576-2578. 10.1056/NEJMp058136.
Waxman HA: The Marketing of Vioxx to Physicians (Memorandum to Democratic members of the Government Reform Committee). 2005
Krumholz HM, Ross JS, Presler AH, Egilman DS: What have we learnt from Vioxx?. BMJ. 2007, 334: 120-123. 10.1136/bmj.39024.487720.68.
Charatan F: Merck to pay $58 m in settlement over rofecoxib advertising. BMJ. 2008, 336: 1208-1209. 10.1136/bmj.39591.705231.DB.
DeAngelis CD, Fontanarosa PB: Impugning the integrity of medical science: the adverse effects of industry influence. JAMA. 2008, 299: 1833-1835. 10.1001/jama.299.15.1833.
Hill KP, Ross JS, Egilman DS, Krumholz HM: The ADVANTAGE seeding trial: a review of internal documents. Ann Intern Med. 2008, 149: 251-258.
Ross JS, Hill KP, Egilman DS, Krumholz HM: Guest authorship and ghostwriting in publications related to rofecoxib: a case study of industry documents from rofecoxib litigation. JAMA. 2008, 299: 1800-1812. 10.1001/jama.299.15.1800.
Moynihan R: Merck defends Vioxx in court, as publisher apologises for fake journal. BMJ. 2009, 338: b1914-10.1136/bmj.b1914.
West RR, Jones DA: Publication bias in statistical overview of trials: example of psychological rehabilitation following myocardial infarction [Abstract]. Proceedings of the 2nd International Conference on the Scientific Basis of Health Services and 5th Annual Cochrane Colloquium; 1997 Oct 8-12; Amsterdam. Amsterdam. 1999, 17-
Mangano DT, Tudor IC, Dietzel C: The risk associated with aprotinin in cardiac surgery. N Engl J Med. 2006, 354: 353-365. 10.1056/NEJMoa051379.
Karkouti K, Beattie WS, Dattilo KM, McCluskey SA, Ghannam M, Hamdy A, Wijeysundera DN, Fedorko L, Yau TM: A propensity score case-control comparison of aprotinin and tranexamic acid in high-transfusion-risk cardiac surgery. Transfusion (Paris). 2006, 46: 327-338.
Hauser RG, Maron BJ: Lessons from the failure and recall of an implantable cardioverter-defibrillator. Circulation. 2005, 112: 2040-2042. 10.1161/CIRCULATIONAHA.105.580381.
Kesselheim AS, Mello MM: Confidentiality laws and secrecy in medical research: improving public access to data on drug safety. Health Aff (Millwood). 2007, 26: 483-491. 10.1377/hlthaff.26.2.483.
Sackner-Bernstein JD, Kowalski M, Fox M, Aaronson K: Short-term risk of death after treatment with nesiritide for decompensated heart failure: a pooled analysis of randomized controlled trials. JAMA. 2005, 293: 1900-1905. 10.1001/jama.293.15.1900.
Camilleri M, Northcutt AR, Kong S, Dukes GE, McSorley D, Mangel AW: Efficacy and safety of alosetron in women with irritable bowel syndrome: a randomised, placebo-controlled trial. Lancet. 2000, 355: 1035-1040. 10.1016/S0140-6736(00)02033-X.
Horton R: Lotronex and the FDA: a fatal erosion of integrity. Lancet. 2001, 357: 1544-1545. 10.1016/S0140-6736(00)04776-0.
Lenzer J: FDA warns that antidepressants may increase suicidality in adults. BMJ. 2005, 331: 70-10.1136/bmj.331.7508.70-b.
Lenzer J: Drug secrets: what the FDA isn't telling. Slate Magazine. 2005, http://www.slate.com/id/2126918
Saunders MC, Dick JS, Brown IM, McPherson K, Chalmers I: The effects of hospital admission for bed rest on the duration of twin pregnancy: a randomised trial. Lancet. 1985, 2: 793-795. 10.1016/S0140-6736(85)90792-5.
Nissen SE: The DREAM trial. Lancet. 2006, 368: 2049-10.1016/S0140-6736(06)69825-5.
Drazen JM, Morrissey S, Curfman GD: Rosiglitazone: continued uncertainty about safety. N Engl J Med. 2007, 357: 63-64. 10.1056/NEJMe078118.
Home PD, Pocock SJ, Beck-Nielsen H, Gomis R, Hanefeld M, Jones NP, Komajda M, McMurray JJ: Rosiglitazone evaluated for cardiovascular outcomes: an interim analysis. N Engl J Med. 2007, 357: 28-38. 10.1056/NEJMoa073394.
Nathan DM: Rosiglitazone and cardiotoxicity: weighing the evidence. N Engl J Med. 2007, 357: 64-66. 10.1056/NEJMe078117.
Psaty BM, Furberg CD: The record on rosiglitazone and the risk of myocardial infarction. N Engl J Med. 2007, 357: 67-69. 10.1056/NEJMe078116.
Psaty BM, Furberg CD: Rosiglitazone and cardiovascular risk. N Engl J Med. 2007, 356: 2522-2524. 10.1056/NEJMe078099.
Rosen CJ: The rosiglitazone story: lessons from an FDA Advisory Committee meeting. N Engl J Med. 2007, 357: 844-846. 10.1056/NEJMp078167.
Singh S, Loke YK, Furberg CD: Long-term risk of cardiovascular events with rosiglitazone: a meta-analysis. JAMA. 2007, 298: 1189-1195. 10.1001/jama.298.10.1189.
Shuster JJ, Schatz DA: The rosiglitazone meta-analysis: lessons for the future. Diabetes Care. 2008, 31: e10-10.2337/dc07-2147.
Friedrich JO, Beyene J, Adhikari NK: Rosiglitazone: can meta-analysis accurately estimate excess cardiovascular risk given the available data? Re-analysis of randomized trials using various methodologic approaches. BMC Res Notes. 2009, 2: 5-10.1186/1756-0500-2-5.
Home PD, Pocock SJ, Beck-Nielsen H, Curtis PS, Gomis R, Hanefeld M, Jones NP, Komajda M, McMurray JJ: Rosiglitazone evaluated for cardiovascular outcomes in oral agent combination therapy for type 2 diabetes (RECORD): a multicentre, randomised, open-label trial. Lancet. 2009, 373: 2125-2135. 10.1016/S0140-6736(09)60953-3.
Merck/Schering-Plough Pharmaceuticals: Merck/Schering-Plough Pharmaceuticals provides results of the ENHANCE trial. Last update 14 Jan 2008 [accessed 13 Mar 2010], http://www.msppharma.com/msppharma/documents/press_release/ENHANCE_news_release_1-14-08.pdf
Greenland P, Lloyd-Jones D: Critical lessons from the ENHANCE trial. JAMA. 2008, 299: 953-955. 10.1001/jama.299.8.953.
Lenzer J: Unreported cholesterol drug data released by company. BMJ. 2008, 336: 180-181. 10.1136/bmj.39468.610775.DB.
Berenson A: Data about Zetia risks was not fully revealed. New York Times. 2007
Furberg CD, Pitt B: Withdrawal of cerivastatin from the world market. Curr Control Trials Cardiovasc Med. 2001, 2: 205-207. 10.1186/CVM-2-5-205.
Wooltorton E: Bayer pulls cerivastatin (Baycol) from market. CMAJ. 2001, 165: 632-
Marwick C: Bayer is forced to release documents over withdrawal of cerivastatin. BMJ. 2003, 326: 518-10.1136/bmj.326.7388.518/a.
Piorkowski JD: Bayer's response to "potential for conflict of interest in the evaluation of suspected adverse drug reactions: use of cerivastatin and risk of rhabdomyolysis". JAMA. 2004, 292: 2655-2657. 10.1001/jama.292.21.2655.
Zinberg DS: A cautionary tale. Science. 1996, 273: 411-10.1126/science.273.5274.411.
Begg CB, Berlin JA: Publication bias and dissemination of clinical research. J Natl Cancer Inst. 1989, 81: 107-115. 10.1093/jnci/81.2.107.
Nathan DG, Weatherall DJ: Academia and industry: lessons from the unfortunate events in Toronto. Lancet. 1999, 353: 771-772. 10.1016/S0140-6736(99)00072-0.
Harris G: Approval of antibiotic worried safety officials. New York Times. 2006
Ross DB: The FDA and the case of Ketek. N Engl J Med. 2007, 356: 1601-1604. 10.1056/NEJMp078032.
Johansen HK, Gotzsche PC: Problems in the design and reporting of trials of antifungal agents encountered during meta-analysis. JAMA. 1999, 282: 1752-1759. 10.1001/jama.282.18.1752.
McKenzie R, Fried MW, Sallie R, Conjeevaram H, Di Bisceglie AM, Park Y, Savarese B, Kleiner D, Tsokos M, Luciano C: Hepatic failure and lactic acidosis due to fialuridine (FIAU), an investigational nucleoside analogue for chronic hepatitis B. N Engl J Med. 1995, 333: 1099-1105. 10.1056/NEJM199510263331702.
Blumsohn A: Authorship, ghostscience, access to data and control of the pharmaceutical scientific literature: who stands behind the word?. Prof Ethics Rep. 2006, 19: 1-4.
Bracken MB, Shepard MJ, Holford TR, Leo-Summers L, Aldrich EF, Fazl M, Fehlings M, Herr DL, Hitchon PW, Marshall LF, Nockels RP, Pascale V, Perot PL, Piepmeier J, Sonntag VK, Wagner F, Wilberger JE, Winn HR, Young W: Administration of methylprednisolone for 24 or 48 hours or tirilazad mesylate for 48 hours in the treatment of acute spinal cord injury: results of the Third National Acute Spinal Cord Injury Randomized Controlled Trial. JAMA. 1997, 277: 1597-1604. 10.1001/jama.277.20.1597.
Download references
Acknowledgements
The authors thank Dirk Eyding, Daniel Fleer, Elke Hausner, Regine Potthast, Andrea Steinzen, and Siw Waffenschmidt for helping to screen reference lists and Verena Wekemann for formatting citations.
Funding source
This work was supported by the German Institute for Quality and Efficiency in Health Care. All authors are employees of the Institute.
Author information
Authors and affiliations.
Institute for Quality and Efficiency in Health Care, Dillenburger Str 27, 51105, Cologne, Germany
Natalie McGauran, Beate Wieseler, Julia Kreis, Yvonne-Beatrice Schüler, Heike Kölsch & Thomas Kaiser
You can also search for this author in PubMed Google Scholar
Corresponding author
Correspondence to Natalie McGauran .
Additional information
Competing interests.
Non-financial competing interests: All authors are employees of the German Institute for Quality and Efficiency in Health Care. In order to produce unbiased HTA reports, the Institute depends on access to all of the relevant data on the topic under investigation. We therefore support the mandatory worldwide establishment of trial registries and study results databases.
Authors' contributions
NM and BW had the idea for the manuscript. NM, HK, YBS, and JK screened reference lists. JK and YBS reviewed titles and abstracts of potentially relevant citations identified in the screening process. NM extracted relevant examples from the full-text publications. BW and TK checked the extracted examples. NM drafted the first version of the manuscript. The remaining authors contributed important intellectual content to the final version. All authors approved the final version.
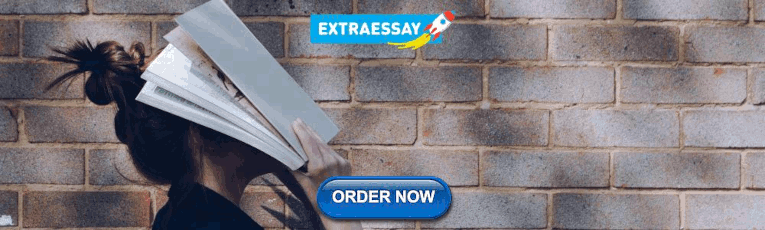
Electronic supplementary material
13063_2009_448_moesm1_esm.doc.
Additional file 1: Table S2: Examples of reporting bias in the medical literature. Extracts from 50 publications presenting examples of reporting bias. (DOC 634 KB)
Rights and permissions
This article is published under license to BioMed Central Ltd. This is an Open Access article distributed under the terms of the Creative Commons Attribution License ( http://creativecommons.org/licenses/by/2.0 ), which permits unrestricted use, distribution, and reproduction in any medium, provided the original work is properly cited.
Reprints and permissions
About this article
Cite this article.
McGauran, N., Wieseler, B., Kreis, J. et al. Reporting bias in medical research - a narrative review. Trials 11 , 37 (2010). https://doi.org/10.1186/1745-6215-11-37
Download citation
Received : 07 November 2009
Accepted : 13 April 2010
Published : 13 April 2010
DOI : https://doi.org/10.1186/1745-6215-11-37
Share this article
Anyone you share the following link with will be able to read this content:
Sorry, a shareable link is not currently available for this article.
Provided by the Springer Nature SharedIt content-sharing initiative
- Irritable Bowel Syndrome
- Atopic Dermatitis
ISSN: 1745-6215
- Submission enquiries: Access here and click Contact Us
- General enquiries: [email protected]
Log in using your username and password
- Search More Search for this keyword Advanced search
- Latest content
- For authors
- Browse by collection
- BMJ Journals More You are viewing from: Google Indexer
You are here
- Volume 8, Issue 3
- Tools for assessing risk of reporting biases in studies and syntheses of studies: a systematic review
- Article Text
- Article info
- Citation Tools
- Rapid Responses
- Article metrics

- Matthew J Page 1 , 2 ,
- Joanne E McKenzie 1 ,
- Julian P T Higgins 2
- 1 School of Public Health and Preventive Medicine , Monash University , Melbourne , Victoria , Australia
- 2 Population Health Sciences , Bristol Medical School, University of Bristol , Bristol , UK
- Correspondence to Dr Matthew J Page; matthew.page{at}monash.edu
Background Several scales, checklists and domain-based tools for assessing risk of reporting biases exist, but it is unclear how much they vary in content and guidance. We conducted a systematic review of the content and measurement properties of such tools.
Methods We searched for potentially relevant articles in Ovid MEDLINE, Ovid Embase, Ovid PsycINFO and Google Scholar from inception to February 2017. One author screened all titles, abstracts and full text articles, and collected data on tool characteristics.
Results We identified 18 tools that include an assessment of the risk of reporting bias. Tools varied in regard to the type of reporting bias assessed (eg, bias due to selective publication, bias due to selective non-reporting), and the level of assessment (eg, for the study as a whole, a particular result within a study or a particular synthesis of studies). Various criteria are used across tools to designate a synthesis as being at ‘high’ risk of bias due to selective publication (eg, evidence of funnel plot asymmetry, use of non-comprehensive searches). However, the relative weight assigned to each criterion in the overall judgement is unclear for most of these tools. Tools for assessing risk of bias due to selective non-reporting guide users to assess a study, or an outcome within a study, as ‘high’ risk of bias if no results are reported for an outcome. However, assessing the corresponding risk of bias in a synthesis that is missing the non-reported outcomes is outside the scope of most of these tools. Inter-rater agreement estimates were available for five tools.
Conclusion There are several limitations of existing tools for assessing risk of reporting biases, in terms of their scope, guidance for reaching risk of bias judgements and measurement properties. Development and evaluation of a new, comprehensive tool could help overcome present limitations.
- publication bias
- bias (epidemiology)
- review literature as topic
This is an Open Access article distributed in accordance with the Creative Commons Attribution Non Commercial (CC BY-NC 4.0) license, which permits others to distribute, remix, adapt, build upon this work non-commercially, and license their derivative works on different terms, provided the original work is properly cited and the use is non-commercial. See: http://creativecommons.org/licenses/by-nc/4.0/
https://doi.org/10.1136/bmjopen-2017-019703
Statistics from Altmetric.com
Request permissions.
If you wish to reuse any or all of this article please use the link below which will take you to the Copyright Clearance Center’s RightsLink service. You will be able to get a quick price and instant permission to reuse the content in many different ways.
Strengths and limitations of this study
Tools for assessing risk of reporting biases, and studies evaluating their measurement properties, were identified by searching several relevant databases using a search string developed in conjunction with an information specialist.
Detailed information on the content and measurement properties of existing tools was collected, providing readers with pertinent information to help decide which tools to use in evidence syntheses.
Screening of articles and data collection were performed by one author only, so it is possible that some relevant articles were missed, or that errors in data collection were made.
The search of grey literature was not comprehensive, so it is possible that there are other tools for assessing risk of reporting biases, and unpublished studies evaluating measurement properties, that were omitted from this review.
Background
The credibility of evidence syntheses can be compromised by reporting biases, which arise when dissemination of research findings is influenced by the nature of the results. 1 For example, there may be bias due to selective publication, where a study is only published if the findings are considered interesting (also known as publication bias). 2 In addition, bias due to selective non-reporting may occur, where findings (eg, estimates of intervention efficacy or an association between exposure and outcome) that are statistically non-significant are not reported or are partially reported in a paper (eg, stating only that ‘P>0.05’). 3 Alternatively, there may be bias in selection of the reported result, where authors perform multiple analyses for a particular outcome/association, yet only report the result which yielded the most favourable effect estimate. 4 Evidence from cohorts of clinical trials followed from inception suggest that biased dissemination is common. Specifically, on average, half of all trials are not published, 1 5 trials with statistically significant results are twice as likely to be published 5 and a third of trials have outcomes that are omitted, added or modified between protocol and publication. 6
Audits of systematic review conduct suggest that most systematic reviewers do not assess risk of reporting biases. 7–10 For example, in a cross-sectional study of 300 systematic reviews indexed in MEDLINE in February 2014, 7 the risk of bias due to selective publication was not considered in 56% of reviews. A common reason for not doing so was that the small number of included studies, or inability to perform a meta-analysis, precluded the use of funnel plots. Only 19% of reviews included a search of a trial registry to identify completed but unpublished trials or prespecified but non-reported outcomes, and only 7% included a search of another source of data disseminated outside of journal articles. The risk of bias due to selective non-reporting in the included studies was assessed in only 24% of reviews. 7 Another study showed that authors of Cochrane reviews routinely record whether any outcomes that were measured were not reported in the included trials, yet rarely consider if such non-reporting could have biased the results of a synthesis. 11
Previous researchers have summarised the characteristics of tools designed to assess various sources of bias in randomised trials, 12–14 non-randomised studies of interventions (NRSI), 14 15 diagnostic test accuracy studies 16 and systematic reviews. 14 17 Others have summarised the performance of statistical methods developed to detect or adjust for reporting biases. 18–20 However, no prior review has focused specifically on tools (ie, structured instruments such as scales, checklists or domain-based tools) for assessing the risk of reporting biases. A particular challenge when assessing risk of reporting biases is that existing tools vary in their level of assessment. For example, tools for assessing risk of bias due to selective publication direct assessments at the level of the synthesis, whereas tools for assessing risk of bias due to selective non-reporting within studies can direct assessments at the level of the individual study, at the level of the synthesis or at both levels. It is unclear how many tools are available to assess different types of reporting bias, and what level they direct assessments at. It is also unclear whether criteria for reaching risk of bias judgements are consistent across existing tools. Therefore, the aim of this research was to conduct a systematic review of the content and measurement properties of such tools.
Methods for this systematic review were prespecified in a protocol which was uploaded to the Open Science Framework in February 2017 ( https://osf.io/9ea22/ ).
Eligibility criteria
Papers were included if the authors described a tool that was designed for use by individuals performing evidence syntheses to assess risk of reporting biases in the included studies or in their synthesis of studies. Tools could assess any type of reporting bias, including bias due to selective publication, bias due to selective non-reporting or bias in selection of the reported result. Tools could assess the risk of reporting biases in any type of study (eg, randomised trial of intervention, diagnostic test accuracy study, observational study estimating prevalence of an exposure) and in any type of result (eg, estimate of intervention efficacy or harm, estimate of diagnostic accuracy, association between exposure and outcome). Eligible tools could take any form, including scales, checklists and domain-based tools. To be considered a scale, each item had to have a numeric score attached to it, so that an overall summary score could be calculated. 12 To be considered a checklist, the tool had to include multiple questions, but the developers’ intention was not to attach a numerical score to each response, or to calculate an overall score. 13 Domain-based tools were those that required users to judge risk of bias or quality within specific domains, and to record the information on which each judgement was based. 21
Tools with a broad scope, for example, to assess multiple sources of bias or the overall quality of the body of evidence, were eligible if one of the items covered risk of reporting bias. Multidimensional tools with a statistical component were also eligible (eg, those that require users to respond to a set of questions about the comprehensiveness of the search, as well as to perform statistical tests for funnel plot asymmetry). In addition, any studies that evaluated the measurement properties of existing tools (eg, construct validity, inter-rater agreement, time taken to complete assessments) were eligible for inclusion. Papers were eligible regardless of the date or format of publication, but were limited to those written in English.
The following were ineligible:
articles or book chapters providing guidance on how to address reporting biases, but which do not include a structured tool that can be applied by users (eg, the 2011 Cochrane Handbook chapter on reporting biases 22 );
tools developed or modified for use in one particular systematic review;
tools designed to appraise published systematic reviews, such as the Risk Of Bias In Systematic reviews (ROBIS) tool 23 or A MeaSurement Tool to Assess systematic Reviews (AMSTAR) 24 ;
articles that focus on the development or evaluation of statistical methods to detect or adjust for reporting biases, as these have been reviewed elsewhere. 18–20
Search methods
On 9 February 2017, one author (MJP) searched for potentially relevant records in Ovid MEDLINE (January 1946 to February 2017), Ovid Embase (January 1980 to February 2017) and Ovid PsycINFO (January 1806 to February 2017). The search strategies included terms relating to reporting bias which were combined with a search string used previously by Whiting et al to identify risk of bias/quality assessment tools 17 (see full Boolean search strategies in online supplementary table S1 ).
Supplementary file 1
To capture any tools not published by formal academic publishers, we searched Google Scholar using the phrase ‘reporting bias tool OR risk of bias’. One author (MJP) screened the titles of the first 300 records, as recommended by Haddaway et al . 25 To capture any papers that may have been missed by all searches, one author (MJP) screened the references of included articles. In April 2017, the same author emailed the list of included tools to 15 individuals with expertise in reporting biases and risk of bias assessment, and asked if they were aware of any other tools we had not identified.
Study selection and data collection
One author (MJP) screened all titles and abstracts retrieved by the searches. The same author screened any full-text articles retrieved. One author (MJP) collected data from included papers using a standardised data-collection form. The following data on included tools were collected:
type of tool (scale, checklist or domain-based tool);
types of reporting bias addressed by the tool;
level of assessment (ie, whether users direct assessments at the synthesis or at the individual studies included in the synthesis);
whether the tool is designed for general use (generic) or targets specific study designs or topic areas (specific);
items included in the tool;
how items within the tool are rated;
methods used to develop the tool (eg, Delphi study, expert consensus meeting);
availability of guidance to assist with completion of the tool (eg, guidance manual).
The following data from studies evaluating measurement properties of an included tool were collected:
tool evaluated
measurement properties evaluated (eg, inter-rater agreement)
number of syntheses/studies evaluated
publication year of syntheses/studies evaluated
areas of healthcare addressed by syntheses/studies evaluated
number of assessors
estimate (and precision) of psychometric statistics (eg, weighted kappa; κ).
Data analysis
We summarised the characteristics of included tools in tables. We calculated the median (IQR) number of items across all tools, and tabulated the frequency of different criteria used in tools to denote a judgement of ‘high’ risk of reporting bias. We summarised estimates of psychometric statistics, such as weighted κ to estimate inter-rater agreement, 26 by reporting the range of values across studies. For studies reporting weighted κ, we categorised agreement according to the system proposed by Landis and Koch, 27 as poor (0.00), slight (0.01–0.20), fair (0.21–0.40), moderate (0.41–0.60), substantial (0.61–0.80) or almost perfect (0.81–1.00).
In total, 5554 records were identified from the searches, of which we retrieved 165 for full-text screening ( figure 1 ). The inclusion criteria were met by 42 reports summarising 18 tools ( table 1 ) and 17 studies evaluating the measurement properties of tools. 3 4 21 28–66 A list of excluded papers is presented in online supplementary table S2 . No additional tools were identified by the 15 experts contacted.
Supplementary file 2
- View inline
List of included tools
- Download figure
- Open in new tab
- Download powerpoint
Flow diagram of identification, screening and inclusion of studies. a Records identified from Ovid MEDLINE, Ovid Embase, Ovid PsycINFO and Google Scholar. b Records identified from screening references of included articles. SR, systematic review.
General characteristics of included tools
Nearly all of the included tools (16/18; 89%) were domain-based, where users judge risk of bias or quality within specific domains ( table 2 ; individual characteristics of each tool are presented in online supplementary table S3 ). All tools were designed for generic rather than specific use. Five tools focused solely on the risk of reporting biases 3 28 29 47 48 ; the remainder addressed reporting biases and other sources of bias/methodological quality (eg, problems with randomisation, lack of blinding). Half of the tools (9/18; 50%) addressed only one type of reporting bias (eg, bias due to selective non-reporting only). Tools varied in regard to the study design that they assessed (ie, randomised trial, non-randomised study of an intervention, laboratory animal experiment). The publication year of the tools ranged from 1998 to 2016 (the earliest was the Downs-Black tool, 31 a 27-item tool assessing multiple sources of bias, one of which focuses on risk of bias in the selection of the reported result).
Supplementary file 3
Summary of general characteristics of included tools
Assessments for half of the tools (9/18; 50%) are directed at an individual study (eg, tool is used to assess whether any outcomes in a study were not reported). In 5/18 (28%) tools, assessments are directed at a specific outcome or result within a study (eg, tool is used to assess whether a particular outcome in a study , such as pain, was not reported). In a few tools (4/18; 22%), assessments are directed at a specific synthesis (eg, tool is used to assess whether a particular synthesis , such as a meta-analysis of studies examining pain as an outcome, is missing unpublished studies).
The content of the included tools was informed by various sources of data. The most common included a literature review of items used in existing tools or a literature review of empirical evidence of bias (9/18; 50%), ideas generated at an expert consensus meeting (8/18; 44%) and pilot feedback on a preliminary version of the tool (7/18; 39%). The most common type of guidance available for the tools was a brief annotation per item/response option (9/18; 50%). A detailed guidance manual is available for four (22%) tools.
Tool content
Four tools include items for assessing risk of bias due to both selective publication and selective non-reporting. 29 33 45 49 One of these tools (the AHRQ tool for evaluating the risk of reporting bias 29 ) directs users to assess a particular synthesis, where a single risk of bias judgement is made based on information about unpublished studies and under-reported outcomes. In the other three tools (the GRADE framework, and two others which are based on GRADE), 33 45 49 the different sources of reporting bias are assessed in separate domains (bias due to selective non-reporting is considered in a ‘study limitations (risk of bias)’ domain, while bias due to selective publication is considered in a ‘publication bias’ domain).
Five tools 21 28 43 44 47 guide users to assess risk of bias due to both selective non-reporting and selection of the reported result (ie, problems with outcomes/results that are not reported and those that are reported, respectively). Four of these tools, which include the Cochrane risk of bias tool for randomised trials 21 and three others which are based on the Cochrane tool, 43 44 47 direct assessments at the study level. That is, a whole study is rated at ‘high’ risk of reporting bias if any outcome/result in the study has been omitted, or fully reported, on the basis of the findings.
Some of the tools designed to assess the risk of bias due to selective non-reporting ask users to assess, for particular outcomes of interest, whether the outcome was not reported or only partially reported in the study on the basis of its results (eg, Outcome Reporting Bias In Trials (ORBIT) tools, 3 48 the AHRQ outcome reporting bias framework, 28 and GRADE. 34 This allows users to perform multiple outcome-level assessments of the risk of reporting bias (rather than one assessment for the study as a whole). In total, 15 tools include a mechanism for assessing risk of bias due to selective non-reporting in studies, but assessing the corresponding risk of bias in a synthesis that is missing the non-reported outcomes is not within the scope of 11 of these tools. 3 21 28 30 38 43 44 47 48 51 52
A variety of criteria are used in existing tools to inform a judgement of ‘high’ risk of bias due to selective publication ( table 3 ), selective non-reporting ( table 4 ), and selection of the reported result ( table 5 ; more detail is provided in online supplementary table S4 ). In the four tools with an assessment of risk of bias due to selective publication, ‘high’ risk criteria include evidence of funnel plot asymmetry, discrepancies between published and unpublished studies, use of non-comprehensive searches and presence of small, ‘positive’ studies with for-profit interest ( table 3 ). However, not all of these criteria appear in all tools (only evidence of funnel plot asymmetry does), and the relative weight assigned to each criterion in the overall risk of reporting bias judgement is clear for only one tool (the Semi-Automated Quality Assessment Tool; SAQAT). 45 46
Supplementary file 4
Criteria used in existing tools to inform a judgement of ‘high’ risk of bias due to selective publication
Criteria used in existing tools to inform a judgement of ‘high’ risk of bias due to selective non-reporting
Criteria used in existing tools to inform a judgement of ‘high’ risk of bias in selection of the reported result
All 15 tools with an assessment of the risk of bias due to selective non-reporting suggest that the risk of bias is ‘high’ when it is clear that an outcome was measured but no results were reported ( table 4 ). Fewer of these tools (n=8; 53%) also recommend a ‘high’ risk judgement when results for an outcome are partially reported (eg, it is stated that the result was non-significant, but no effect estimate or summary statistics are presented).
The eight tools that include an assessment of the risk of bias in selection of the reported result recommend various criteria for a ‘high’ risk judgement ( table 5 ). These include when some outcomes that were not prespecified are added post hoc (in 4 (50%) tools), or when it is likely that the reported result for a particular outcome has been selected, on the basis of the findings, from among multiple outcome measurements or analyses within the outcome domain (in 2 (25%) tools).
General characteristics of studies evaluating measurement properties of included tools
Despite identifying 17 studies that evaluated measurement properties of an included tool, psychometric statistics for the risk of reporting bias component were available only from 12 studies 43 44 54–60 62 64 66 (the other five studies include only data on properties of the multidimensional tool as a whole 31 53 61 63 65 ; online supplementary table S5 ). Nearly all 12 studies (11; 92%) evaluated inter-rater agreement between two assessors; eight of these studies reported weighted κ values, but only two described the weighting scheme. 55 62 Eleven studies 43 44 54–60 64 66 evaluated the measurement properties of tools for assessing risk of bias in a study due to selective non-reporting or risk of bias in selection of the reported result; in these 11 studies, a median of 40 (IQR 32–109) studies were assessed. One study 62 evaluated a tool for assessing risk of bias in a synthesis due to selective publication, in which 44 syntheses were assessed. In the studies evaluating inter-rater agreement, all involved two assessors.
Supplementary file 5
Results of evaluation studies.
Five studies 54 56–58 60 included data on the inter-rater agreement of assessments of risk of bias due to selective non-reporting using the Cochrane risk of bias tool for randomised trials 21 ( table 6 ). Weighted κ values in four studies 54 56–58 ranged from 0.13 to 0.50 (sample size ranged from 87 to 163 studies), suggesting slight to moderate agreement. 27 In the other study, 60 the per cent agreement in selective non-reporting assessments in trials that were included in two different Cochrane reviews was low (43% of judgements were in agreement). Two other studies found that inter-rater agreement of selective non-reporting assessments were substantial for SYRCLE’s RoB tool (κ=0.62, n=32), 43 but poor for the RoBANS tool (κ=0, n=39). 44 There was substantial agreement between raters in the assessment of risk of bias due to selective publication using the SAQAT (κ=0.63, n=29). 62 The inter-rater agreement of assessments of risk of bias in selection of the reported result using the ROBINS-I tool 4 was moderate for NRSI included in a review of the effect of cyclooxygenase-2 inhibitors on cardiovascular events (κ=0.45, n=21), and substantial for NRSI included in a review of the effect of thiazolidinediones on cardiovascular events (κ=0.78, n=16). 55
Reported measurement properties of tools with an assessment of the risk of reporting bias
From a systematic search of the literature, we identified 18 tools designed for use by individuals performing evidence syntheses to assess risk of reporting biases in the included studies or in their synthesis of studies. The tools varied with regard to the type of reporting bias assessed (eg, bias due to selective publication, bias due to selective non-reporting), and the level of assessment (eg, for the study as a whole, a particular outcome within a study or a particular synthesis of studies). Various criteria are used across tools to designate a synthesis as being at ‘high’ risk of bias due to selective publication (eg, evidence of funnel plot asymmetry, use of non-comprehensive searches). However, the relative weight assigned to each criterion in the overall judgement is not clear for most of these tools. Tools for assessing risk of bias due to selective non-reporting guide users to assess a study, or an outcome within a study, as ‘high’ risk of bias if no results are reported for an outcome. However, assessing the corresponding risk of bias in a synthesis that is missing the non-reported outcomes is outside the scope of most of these tools. Inter-rater agreement estimates were available for five tools, 4 21 43 44 62 and ranged from poor to substantial; however, the sample sizes of most evaluations were small, and few described the weighting scheme used to calculate κ.
Strengths and limitations
There are several strengths of this research. Methods were conducted in accordance with a systematic review protocol ( https://osf.io/9ea22/ ). Published articles were identified by searching several relevant databases using a search string developed in conjunction with an information specialist, 17 and by contacting experts to identify tools missed by the search. Detailed information on the content and measurement properties of existing tools was collected, providing readers with pertinent information to help decide which tools to use in future reviews. However, the findings need to be considered in light of some limitations. Screening of articles and data collection were performed by one author only. It is therefore possible that some relevant articles were missed, or that errors in data collection were made. The search for unpublished tools was not comprehensive (only Google Scholar was searched), so it is possible that other tools for assessing risk of reporting biases exist. Further, restricting the search to articles in English was done to expedite the review process, but may have resulted in loss of information about tools written in other languages, and additional evidence on measurement properties of tools.
Comparison with other studies
Other systematic reviews of risk of bias tools 12–17 have restricted inclusion to tools developed for particular study designs (eg, randomised trials, diagnostic test accuracy studies), where the authors recorded all the sources of bias addressed. A different approach was taken in the current review, where all tools (regardless of study design) that address a particular source of bias were examined. By focusing on one source of bias only, the analysis of included items and criteria for risk of bias judgements was more detailed than that recorded previously. Some of the existing reviews of tools 15 considered tools that were developed or modified in the context of a specific systematic review. However, such tools were excluded from the current review as they are unlikely to have been developed systematically, 15 67 and are difficult to find (all systematic reviews conducted during a particular period would need to have been examined for the search to be considered exhaustive).
Explanations and implications
Of the 18 tools identified, only four (22%) included a mechanism for assessing risk of bias due to selective publication, which is the type of reporting bias that has been investigated by methodologists most often. 2 This is perhaps unsurprising given that hundreds of statistical methods to ‘detect’ or ‘adjust’ for bias due to selective publication have been developed. 18 These statistical methods may be considered by methodologists and systematic reviewers as the tools of choice for assessing this type of bias. However, application of these statistical methods without considering other factors (eg, existence of registered but unpublished studies, conflicts of interest that may influence investigators to not disseminate studies with unfavourable results) is not sufficiently comprehensive, and could lead to incorrect conclusions about the risk of bias due to selective publication. Further, there are many limitations of these statistical approaches, in terms of their underlying assumptions, statistical power, which is often low because most meta-analyses include few studies, 7 and the need for specialist statistical software to apply them. 19 68 These factors may have limited their use in practice and potentially explain why a large number of systematic reviewers currently ignore the risk of bias due to selective publication. 7–9 69
Our analysis suggests that the factors that need to be considered to assess risk of reporting biases adequately (eg, comprehensiveness of the search, amount of data missing from the synthesis due to unpublished studies and under-reported outcomes) are fragmented. A similar problem was occurring a decade ago with the assessment of risk of bias in randomised trials. Some authors assessed only problems with randomisation, while others focused on whether trials were not ‘double blinded’ or had any missing participant data. 70 It was not until all the important bias domains were brought together into a structured, domain-based tool to assess the risk of bias in randomised trials, 21 that systematic reviewers started to consider risk of bias in trials comprehensively. A similar initiative to link all the components needed to judge the risk of reporting biases into a comprehensive new tool may improve the credibility of evidence syntheses.
In particular, there is an emergent need for a new tool to assess the risk that a synthesis is affected by reporting biases. This tool could guide users to consider risk of bias in a synthesis due to both selective publication and selective non-reporting, given that both practices lead to the same consequence: evidence missing from the synthesis. 11 Such a tool would complement recently developed tools for assessing risk of bias within studies (RoB 2.0 41 and ROBINS-I 4 which include a domain for assessing the risk of bias in selection of the reported result, but no mechanism to assess risk of bias due to selective non-reporting). Careful thought would need to be given as to how to weigh up various pieces of information underpinning the risk of bias judgement. For example, users will need guidance on how evidence of known, unpublished studies (as identified from trial registries, protocols or regulatory documents) should be considered alongside evidence that is more speculative (eg, funnel plots suggesting that studies may be missing). Further, guidance for the tool will need to emphasise the value of seeking documents other than published journal articles (eg, protocols) to inform risk of bias judgements. Preparation of a detailed guidance manual may enhance the usability of the tool, minimise misinterpretation and increase reliability in assessments. Once developed, evaluations of the measurement properties of the tool, such as inter-rater agreement and construct validity, should be conducted to explore whether modifications to the tool are necessary.
Conclusions
There are several limitations of existing tools for assessing risk of reporting biases in studies or syntheses of studies, in terms of their scope, guidance for reaching risk of bias judgements and measurement properties. Development and evaluation of a new, comprehensive tool could help overcome present limitations.
- Vickers A , et al
- Hooper L , et al
- Kirkham JJ ,
- Altman DG , et al
- Sterne JA ,
- Hernán MA ,
- Reeves BC , et al
- Schmucker C ,
- Schell LK ,
- Portalupi S , et al
- Holland WC , et al
- Shamseer L ,
- Koletsi D ,
- Fleming PS , et al
- Umberham BA ,
- Detweiler BN , et al
- Chan AW , et al
- Nichol G , et al
- Macedo LG ,
- Gadotti IC , et al
- Shukla VK ,
- Bak G , et al
- Sanderson S ,
- Whiting P ,
- Rutjes AW ,
- Dinnes J , et al
- Savovic J , et al
- Mueller KF ,
- Meerpohl JJ ,
- Briel M , et al
- Sutton AJ ,
- Ioannidis JP , et al
- Higgins JP ,
- Altman DG ,
- Gøtzsche PC , et al
- Sterne JAC ,
- Savović J ,
- Higgins JP , et al
- Grimshaw JM ,
- Wells GA , et al
- Haddaway NR ,
- Collins AM ,
- Coughlin D , et al
- Landis JR ,
- Balshem H ,
- Stevens A ,
- Ansari M , et al
- Berkman ND ,
- Downes MJ ,
- Brennan ML ,
- Williams HC , et al
- Kolamunnage-Dona R , et al
- Guyatt GH ,
- Vist GE , et al
- Vist G , et al
- Montori V , et al
- Schünemann H ,
- Guyatt G , et al
- Santesso N ,
- Carrasco-Labra A ,
- Langendam M , et al
- Hayden JA ,
- van der Windt DA ,
- Cartwright JL , et al
- Higgins JPT ,
- Page MJ , et al
- Savović J , et al
- Hooijmans CR ,
- Rovers MM ,
- de Vries RB , et al
- Lee YJ , et al
- Llewellyn A , et al
- Stewart GB ,
- Schünemann H , et al
- Tejani AM ,
- Huan LN , et al
- Gamble C , et al
- Salanti G ,
- Del Giovane C ,
- Chaimani A , et al
- Viswanathan M ,
- Dryden DM , et al
- Armijo-Olivo S ,
- Stiles CR ,
- Hagen NA , et al
- da Costa BR , et al
- Bilandzic A ,
- Fitzpatrick T ,
- Rosella L , et al
- Hartling L ,
- Liang Y , et al
- Vandermeer B , et al
- Milne A , et al
- Jordan VM ,
- Lensen SF ,
- Farquhar CM
- Miladinovic B ,
- Guyatt GH , et al
- Llewellyn A ,
- Whittington C ,
- Stewart G , et al
- Mustafa RA ,
- Brozek J , et al
- Norris SL ,
- Holmer HK ,
- Ogden LA , et al
- O’Connor SR ,
- Ryan B , et al
- Tierney JF ,
- Whiting PF ,
- Westwood ME , et al
- Moher D , et al
- Gøtzsche PC
Contributors MJP conceived and designed the study, collected data, analysed the data and wrote the first draft of the article. JEM and JPTH provided input on the study design and contributed to revisions of the article. All authors approved the final version of the submitted article.
Funding MJP is supported by an Australian National Health and Medical Research Council (NHMRC) Early Career Fellowship (1088535). JEM is supported by an NHMRC Australian Public Health Fellowship (1072366). JPTH is funded in part by Cancer Research UK Programme Grant C18281/A19169; is a member of the MRC Integrative Epidemiology Unit at the University of Bristol, which is supported by the UK Medical Research Council and the University of Bristol (grant MC_UU_12013/9); and is a member of the MRC ConDuCT-II Hub (Collaboration and innovation for Difficult and Complex randomised controlled Trials In Invasive procedures; grant MR/K025643/1).
Competing interests JPTH led or participated in the development of four of the included tools (the current Cochrane risk of bias tool for randomised trials, the RoB 2.0 tool for assessing risk of bias in randomised trials, the ROBINS-I tool for assessing risk of bias in non-randomised studies of interventions and the framework for assessing quality of evidence from a network meta-analysis). MJP participated in the development of one of the included tools (the RoB 2.0 tool for assessing risk of bias in randomised trials). All authors are participating in the development of a new tool for assessing risk of reporting biases in systematic reviews.
Patient consent Not required.
Provenance and peer review Not commissioned; externally peer reviewed.
Data sharing statement The study protocol, data collection form, and the raw data and statistical analysis code for this study are available on the Open Science Framework: https://osf.io/3jdaa/
Read the full text or download the PDF:
Causes of reporting bias: a theoretical framework
Affiliations.
- 1 Department of Public Health and Primary Care, Leiden University Medical Center, Hippocratespad 21, Gebouw 3, Leiden, 2300 RC Leiden, The Netherlands.
- 2 Department of Primary and Community Care, Radboud university medical center, Geert Grooteplein Noord 21, 6500 HB Nijmegen, The Netherlands.
- 3 ACHIEVE Centre for Applied Research, Amsterdam University of Applied Sciences, Tafelbergweg 51, Amsterdam, 1105 BD Amsterdam, The Netherlands.
- 4 Department of Cardiology, Amsterdam University Medical Center (location Meibergdreef), University of Amsterdam, Meibergdreef 9, 1105 AZ Amsterdam, The Netherlands.
- 5 Apotheek Boekel, Kerkstraat 35, Boekel, 5427 BB, The Netherlands.
- 6 Department of Epidemiology and Biostatistics, Amsterdam University Medical Centers, location VUmc, Van der Boechorststraat 7, 1081 BT Amsterdam, The Netherlands.
- 7 Department of Philosophy, Faculty of Humanities, Vrije Universiteit Amsterdam, De Boelelaan 1105, 1081 HV Amsterdam, The Netherlands.
- PMID: 31497290
- PMCID: PMC6713068
- DOI: 10.12688/f1000research.18310.2
Reporting of research findings is often selective. This threatens the validity of the published body of knowledge if the decision to report depends on the nature of the results. The evidence derived from studies on causes and mechanisms underlying selective reporting may help to avoid or reduce reporting bias. Such research should be guided by a theoretical framework of possible causal pathways that lead to reporting bias. We build upon a classification of determinants of selective reporting that we recently developed in a systematic review of the topic. The resulting theoretical framework features four clusters of causes. There are two clusters of necessary causes: (A) motivations (e.g. a preference for particular findings) and (B) means (e.g. a flexible study design). These two combined represent a sufficient cause for reporting bias to occur. The framework also features two clusters of component causes: (C) conflicts and balancing of interests referring to the individual or the team, and (D) pressures from science and society. The component causes may modify the effect of the necessary causes or may lead to reporting bias mediated through the necessary causes. Our theoretical framework is meant to inspire further research and to create awareness among researchers and end-users of research about reporting bias and its causes.
Keywords: Causality; publication bias; questionable research practice; reporting bias; research design; selective reporting.
Publication types
- Research Support, Non-U.S. Gov't
- Systematic Review
- Research Design*
Grants and funding
Thank you for visiting nature.com. You are using a browser version with limited support for CSS. To obtain the best experience, we recommend you use a more up to date browser (or turn off compatibility mode in Internet Explorer). In the meantime, to ensure continued support, we are displaying the site without styles and JavaScript.
- View all journals
- My Account Login
- Explore content
- About the journal
- Publish with us
- Sign up for alerts
- Open access
- Published: 22 May 2024
Uncovering the essence of diverse media biases from the semantic embedding space
- Hong Huang 1 , 2 , 3 , 4 , 5 ,
- Hua Zhu 1 , 2 , 3 , 4 , 5 ,
- Wenshi Liu 4 , 5 ,
- Hua Gao 5 ,
- Hai Jin 1 , 2 , 3 , 4 , 5 &
- Bang Liu 6
Humanities and Social Sciences Communications volume 11 , Article number: 656 ( 2024 ) Cite this article
178 Accesses
Metrics details
- Cultural and media studies
Media bias widely exists in the articles published by news media, influencing their readers’ perceptions, and bringing prejudice or injustice to society. However, current analysis methods usually rely on human efforts or only focus on a specific type of bias, which cannot capture the varying magnitudes, connections, and dynamics of multiple biases, thus remaining insufficient to provide a deep insight into media bias. Inspired by the Cognitive Miser and Semantic Differential theories in psychology, and leveraging embedding techniques in the field of natural language processing, this study proposes a general media bias analysis framework that can uncover biased information in the semantic embedding space on a large scale and objectively quantify it on diverse topics. More than 8 million event records and 1.2 million news articles are collected to conduct this study. The findings indicate that media bias is highly regional and sensitive to popular events at the time, such as the Russia-Ukraine conflict. Furthermore, the results reveal some notable phenomena of media bias among multiple U.S. news outlets. While they exhibit diverse biases on different topics, some stereotypes are common, such as gender bias. This framework will be instrumental in helping people have a clearer insight into media bias and then fight against it to create a more fair and objective news environment.
Similar content being viewed by others
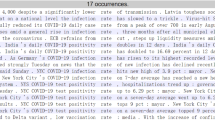
What is newsworthy about Covid-19? A corpus linguistic analysis of news values in reports by China Daily and The New York Times
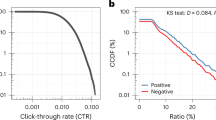
Negativity drives online news consumption
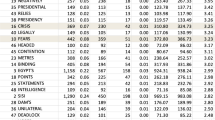
Media bias through collocations: a corpus-based study of Egyptian and Ethiopian news coverage of the Grand Ethiopian Renaissance Dam
Introduction.
In the era of information explosion, news media play a crucial role in delivering information to people and shaping their minds. Unfortunately, media bias, also called slanted news coverage, can heavily influence readers’ perceptions of news and result in a skewing of public opinion (Gentzkow et al. 2015 ; Puglisi and Snyder Jr, 2015b ; Sunstein, 2002 ). This influence can potentially lead to severe societal problems. For example, a report from FAIR has shown that Verizon management is more than twice as vocal as worker representatives in news reports about the Verizon workers’ strike in 2016 Footnote 1 , putting workers at a disadvantage in the news and contradicting the principles of fair and objective journalism. Unfortunately, this is just the tip of the media bias iceberg.
Media bias can be defined as the bias of journalists and news producers within the mass media in selecting and covering numerous events and stories (Gentzkow et al. 2015 ). This bias can manifest in various forms, such as event selection, tone, framing, and word choice (Hamborg et al. 2019 ; Puglisi and Snyder Jr, 2015b ). Given the vast number of events happening in the world at any given moment, even the most powerful media must be selective in what they choose to report instead of covering all available facts in detail (Downs, 1957 ). This selectivity can result in the perception of bias in the news coverage, whether intentional or unintentional. Academics in journalism studies attempt to explain the news selection process by developing taxonomies of news values (Galtung and Ruge, 1965 ; Harcup and O’neill, 2001 , 2017 ), which refer to certain criteria and principles that news editors and journalists consider when selecting, editing, and reporting the news. These values help determine which stories should be considered news and the significance of these stories in news reporting. However, different news organizations and journalists may emphasize different news values based on their specific objectives and audience. Consequently, a media outlet may be very keen on reporting events about specific topics while turning a blind eye to others. For example, news coverage often ignores women-related events and issues with the implicit assumption that they are less critical than men-related contents (Haraldsson and Wängnerud, 2019 ; Lühiste and Banducci, 2016 ; Ross and Carter, 2011 ). Once events are selected, the media must consider how to organize and write their news articles. At that time, the choice of tone, framing, and word is highly subjective and can introduce bias. Specifically, the words used by the authors to refer to different entities may not be neutral but instead imply various associations and value judgments (Puglisi and Snyder Jr, 2015b ). As shown in Fig. 1 , the same topic can be expressed in entirely different ways, depending on a media outlet’s standpoint Footnote 2 . For example, certain “right-wing” media outlets tend to support legal abortion, while some “left-wing” ones oppose it.
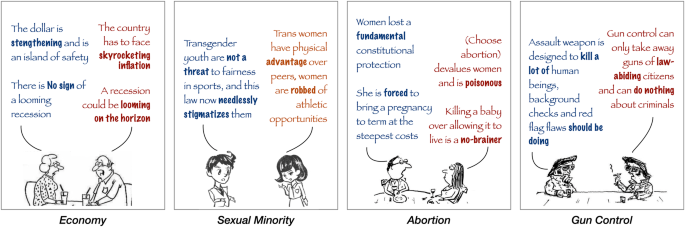
The blue and red fonts represent the views of some “left-wing” and “right-wing” media outlets, respectively.
In fact, media bias is influenced by many factors: explicit factors such as geographic location, media position, editorial guideline, topic setting, and so on; obscure factors such as political ideology (Groseclose and Milyo, 2005 ; MacGregor, 1997 ; Merloe, 2015 ), business reason (Groseclose and Milyo, 2005 ; Paul and Elder, 2004 ), and personal career (Baron, 2006 ), etc. Besides, some studies also summarize these factors related to bias as supply-side and demand-side ones (Gentzkow et al. 2015 ; Puglisi and Snyder Jr, 2015b ). The influence of these complex factors makes the emergence of media bias inevitable. However, media bias may hinder readers from forming objective judgments about the real world, lead to skewed public opinion, and even exacerbate social prejudices and unfairness. For example, the New York Times supports Iranian women’s saying no to hijabs in defense of women’s rights Footnote 3 while criticizing the Chinese government’s initiative to encourage Uyghur women to remove hijabs and veils Footnote 4 . Besides, the influence of news coverage on voter behavior is a subject of ongoing debate. While some studies indicate that slanted news coverage can influence voters and election outcomes (Bovet and Makse, 2019 ; DellaVigna and Kaplan, 2008 ; Grossmann and Hopkins, 2016 ), others suggest that this influence is limited in certain circumstances (Stroud, 2010 ). Fortunately, research on media bias has drawn attention from multiple disciplines.
In social science, the study of media bias has a long tradition dating back to the 1950s (White, 1950 ). So far, most of the analyses in social science have been qualitative, aiming to analyze media opinions expressed in the editorial section (e.g., endorsements (Ansolabehere et al. 2006 ), editorials (Ho et al. 2008 ), ballot propositions (Puglisi and Snyder Jr, 2015a )) or find out biased instances in news articles by human annotations (Niven, 2002 ; Papacharissi and de Fatima Oliveira, 2008 ; Vaismoradi et al. 2013 ). Some researchers also conduct quantitative analysis, which primarily involves counting the frequency of specific keywords or articles related to certain issues (D’Alessio and Allen, 2000 ; Harwood and Garry, 2003 ; Larcinese et al. 2011 ). In particular, there are some attempts to estimate media bias using automatic tools (Groseclose and Milyo, 2005 ), and they commonly rely on text similarity and sentiment computation (Gentzkow and Shapiro, 2010 ; Gentzkow et al. 2006 ; Lott Jr and Hassett, 2014 ). In summary, social science research on media bias has yielded extensive and effective methodologies. These methodologies interpret media bias from diverse perspectives, marking significant progress in the realm of media studies. However, these methods usually rely on manual annotation and analysis of the texts, which requires significant manual effort and expertise (Park et al. 2009 ), thus might be inefficient and subjective. For example, in a quantitative analysis, researchers might devise a codebook with detailed definitions and rules for annotating texts, and then ask coders to read and annotate the corresponding texts (Hamborg et al. 2019 ). Developing a codebook demands substantial expertise. Moreover, the standardization process for text annotation is subjective, as different coders may interpret the same text differently, thus leading to varied annotations.
In computer science, research on social media is extensive (Lazaridou et al. 2020 ; Liu et al. 2021b ; Tahmasbi et al. 2021 ), but few methods are specifically designed to study media bias (Hamborg et al. 2019 ). Some techniques that specialize in the study of media bias focus exclusively on one type of bias (Huang et al. 2021 ; Liu et al. 2021b ; Zhang et al. 2017 ), thus not general enough. In natural language processing (NLP), research on the bias of pre-trained models or language models has attracted much attention (Qiang et al. 2023 ), aiming to identify and reduce the potential impact of bias in pre-trained models on downstream tasks (Huang et al. 2020 ; Liu et al. 2021a ; Wang et al. 2020 ). In particular, some studies on pre-trained word embedding models show that they have captured rich human knowledge and biases (Caliskan et al. 2017 ; Grand et al. 2022 ; Zeng et al. 2023 ). However, such works mainly focus on pre-trained models rather than media bias directly, which limits their applicability to media bias analysis.
A major challenge in studying media bias is that the evaluation of media bias is highly subjective because individuals have varying evaluation criteria for bias. Take political bias as an example, a story that one person views as neutral may appear to be left-leaning or right-leaning by someone else. To address this challenge, we develop an objective and comprehensive media bias analysis framework. We study media bias from two distinct but highly relevant perspectives: the macro level and the micro level. From the macro perspective, we focus on the event selection bias of each media, i.e., the types of events each media tends to report on. From the micro perspective, we focus on the bias introduced by media in the choice of words and sentence construction when composing news articles about the selected events.
In news articles, media outlets convey their attitudes towards a subject through the contexts surrounding it. However, the language used by the media to describe and refer to entities may not be purely neutral descriptors but rather imply various associations and value judgments. According to the cognitive miser theory in psychology, the human mind is considered a cognitive miser who tends to think and solve problems in simpler and less effortful ways to avoid cognitive effort (Fiske and Taylor, 1991 ; Stanovich, 2009 ). Therefore, faced with endless news information, ordinary readers will tend to summarize and remember the news content simply, i.e., labeling the things involved in news reports. Frequent association of certain words with a particular entity or subject in news reports can influence a media outlet’s loyal readers to adopt these words as labels for the corresponding item in their cognition due to the cognitive miser effect. Unfortunately, such a cognitive approach is inadequate and susceptible to various biases. For instance, if a media outlet predominantly focuses on male scientists while neglecting their female counterparts, some naive readers may perceive scientists to be mostly male, leading to a recognition bias in their perception of the scientist and even forming stereotypes unconsciously over time. According to the “distributional hypothesis” in modern linguistics (Firth, 1957 ; Harris, 1954 ; Sahlgren, 2008 ), a word’s meaning is characterized by the words occurring in the same context as it. Here, we simplify the complex associations between different words (or entities/subjects) and their respective context words into co-occurrence relationships. An effective technique to capture word semantics based on co-occurrence information is neural network-based word embedding models (Kenton and Toutanova, 2019 ; Le and Mikolov, 2014 ; Mikolov et al. 2013 ).
Word embedding models represent each word in the vocabulary as a vector (i.e., word embedding) within the word embedding space. In this space, words that frequently co-occur in similar contexts are positioned close to each other. For instance, if a media outlet predominantly features male scientists, the word “scientist” and related male-centric terms, such as “man” and “he” will frequently co-occur. Consequently, these words will cluster near the word “scientist” in the embedding space, while female-related words occupy more distant positions. This enables us to evaluate the media outlet’s gender bias concerning the term “scientist” by comparing the embedding distances between “scientist” and words associated with both males and females. This approach aligns closely with the Semantic Differential theory in psychology (Osgood et al. 1957 ), which gauges an individual’s attitudes toward various concepts, objects, and events using bipolar scales constructed from adjectives with opposing semantics. In this study, to identify media bias from news articles, we first define two sets of words with opposite semantics for each topic to develop media bias evaluation scales. Then, we quantify media bias on each topic by calculating the embedding distance difference between a target word (e.g., scientist) and these two sets of words (e.g., female-related words and male-related words) in the word embedding space.
Compared with the bias in news articles, event selection bias is more obscure, as only events of interest to the media are reported in the final articles, while events deliberately ignored by the media remain invisible to the public. Similar to the co-occurrence relationship between words mentioned earlier, two media outlets that frequently select and report on the same events should exhibit similar biases in event selection, as two words that occur frequently in the same contexts have similar semantics. Therefore, we refer to Latent Semantic Analysis (LSA (Deerwester et al. 1990 )) and generate vector representation (i.e., media embedding) for each media via truncated singular value decomposition (Truncated SVD (Halko et al. 2011 )). Essentially, a media embedding encodes the distribution of the events that a media outlet tends to report on. Therefore, in the media embedding space, media outlets that often select and report on the same events will be close to each other due to similar distributions of the selected events. If a media outlet shows significant differences in such a distribution compared to other media outlets, we can conclude that it is biased in event selection. Inspired by this, we conduct clustering on the media embeddings to study how different media outlets differ in the distribution of selected events, i.e., the so-called event selection bias.
These two methodologies, designed for micro-level and macro-level analysis, share a fundamental similarity: both leverage data-driven embedding models to represent each word or media outlet as a distinctive vector within the embedding space and conduct further analysis based on these vectors. Therefore, in this study, we integrate both methodologies into a unified framework for media bias analysis. We aim to uncover media bias on a large scale and quantify it objectively on diverse topics. Our experiment results show that: (1) Different media outlets have different preferences for various news events, and those from the same country or organization tend to share more similar tastes. Besides, the occurrence of international hot events will lead to the convergence of different media outlets’ event selection. (2) Despite differences in media bias, some stereotypes, such as gender bias, are common among various media outlets. These findings align well with our empirical understanding, thus validating the effectiveness of our proposed framework.
Data and methods
The first dataset is the GDELT Mention Table, a product of the Google Jigsaw-backed GDELT project Footnote 5 . This project aims to monitor news reports from all over the world, including print, broadcast, and online sources, in over 100 languages. Each time an event is mentioned in a news report, a new row is added to the Mention Table (See Supplementary Information Tab. S1 for details). Given that different media outlets may report on the same event at varying times, the same event can appear in multiple rows of the table. While the fields GlobalEventID and EventTimeDate are globally unique attributes for each event, MentionSourceName and MentionTimeDate may differ. Based on the GlobalEventID and MentionSourceName fields in the Mention Table, we can count the number of times each media outlet has reported on each event, ultimately constructing a “media-event” matrix. In this matrix, the element at ( i , j ) denotes the number of times that media outlet j has reported on the event i in past reports.
As a global event database, GDELT collects a vast amount of global events and topics, encompassing news coverage worldwide. However, despite its widespread usage in many studies, there are still some noteworthy issues. Here, we highlight some of the issues to remind readers to use it more cautiously. Above all, while GDELT provides a vast amount of data from various sources, it cannot capture every event accurately. It relies on automated data collection methods, and this could result in certain events being missed. Furthermore, its algorithms for event extraction and categorization cannot always perfectly capture the nuanced context and meaning of each event, which might lead to potential misinterpretations.
The second dataset is built on MediaCloud Footnote 6 , an open-source platform for research on media ecosystems. MediaCloud’s API enables the querying of news article URLs for a given media outlet, which can then be retrieved using a web crawler. In this study, we have collected more than 1.2 million news articles from 12 mainstream US media outlets in 2016-2021 via MediaCloud’s API (See Supplementary Information Tab. S2 for details).
Media bias estimation by media embedding
Latent Semantic Analysis (LSA (Deerwester et al. 1990 )) is a well-established technique for uncovering the topic-based semantic relationships between text documents and words. By performing truncated singular value decomposition (Truncated SVD (Halko et al. 2011 )) on a “document-word” matrix, LSA can effectively capture the topics discussed in a corpus of text documents. This is accomplished by representing documents and words as vectors in a high-dimensional embedding space, where the similarity between vectors reflects the similarity of the topics they represent. In this study, we apply this idea to media bias analysis by likening media and events to documents and words, respectively. By constructing a “media-event” matrix and performing Truncated SVD, we can uncover the underlying topics driving the media coverage of specific events. Our hypothesis posits that media outlets mentioning certain events more frequently are more likely to exhibit a biased focus on the topics related to those events. Therefore, media outlets sharing similar topic tastes during event selection will be close to each other in the embedding space, which provides a good opportunity to shed light on the media’s selection bias.
The generation procedures for media embeddings are shown in Supplementary Information Fig. S1 . First, a “media-event” matrix denoted as A m × n is constructed based on the GDELT Mention Table, where m and n represent the total number of media outlets and events, respectively. Each entry A i , j represents the number of times that media i has reported on event j . Subsequently, Truncated SVD is performed on the matrix A m × n , which results in three matrices: U m × k , Σ k × k and \({V}_{n\times k}^{T}\) . The product of Σ k × k and \({V}_{n\times k}^{T}\) is represented by E k × n . Each column of E k × n corresponds to a k -dimensional vector representation for a specific media outlet, i.e., a media embedding. Specifically, the decomposition of matrix A m × n can be formulated as follows:
Equation( 1 ) defines the complete singular value decomposition of A m × n . Both \({U}_{m\times m}^{0}\) and \({({V}_{n\times n}^{0})}^{T}\) are orthogonal matrices. \({{{\Sigma }}}_{m\times n}^{0}\) is a m × n diagonal matrix whose diagonal elements are non-negative singular values of the matrix A m × n in descending order. Equation( 2 ) defines the truncated singular value decomposition (i.e., Truncated SVD) of A m × n . Based on the result of complete singular value decomposition, the part corresponding to the largest k singular values is equivalent to the result of Truncated SVD. Specifically, U m × k comprises the first k columns of the matrix \({U}_{m\times m}^{0}\) , while \({V}_{n\times k}^{T}\) comprises the first k rows of the matrix \({({V}_{n\times n}^{0})}^{T}\) . Additionally, the diagonal matrix Σ k × k is composed of the first k diagonal elements of \({{{\Sigma }}}_{m\times n}^{0}\) , representing the largest k singular values of A m × n . In particular, the media embedding model is defined as the product of the matrices Σ k × k and \({V}_{n\times k}^{T}\) , which has n k -dimensional media embeddings as follows:
To measure the similarity between two media embedding sets, we refer to Word Mover Distance (WMD (Kusner et al. 2015 )). WMD is designed to measure the dissimilarity between two text documents based on word embedding. Here, we subtract the optimal value of the original WMD objective function from 1 to convert the dissimilarity value into a normalized similarity score that ranges from 0 to 1. Specifically, the similarity between two media embedding sets is formulated as follows:
Let n denote the total number of media outlets, and s be an n -dimensional vector corresponding to the first media embedding set. For each i , the weight of media i in the embedding set is given by \({s}_{i}=\frac{1}{\sum_{k = 1}^{n}{t}_{i}}\) , where t i = 1 if media i is in the embedding set, and t i = 0 otherwise. Similarly, \({s}^{{\prime} }\) is another n -dimensional vector corresponding to the second media embedding set. The distance between media i and j is calculated using c ( i , j ) = ∥ e i − e j ∥ 2 , where e i and e j are the embedding representations of media i and j , respectively. The flow matrix T ∈ R n × n is used to determine how much media i in s travels to media j in \({s}^{{\prime} }\) . Specifically, T i , j ≥ 0 denotes the amount of flow from media i to media j .
Media bias estimation by word embedding
Word embedding models (Kenton and Toutanova, 2019 ; Le and Mikolov, 2014 ; Mikolov et al. 2013 ) are widely used in text-related tasks due to their ability to capture rich semantics of natural language. In this study, we regard media bias in news articles as a special type of semantic and capture it using Word2Vec (Le and Mikolov, 2014 ; Mikolov et al. 2013 ).
Supplementary Information Fig. S2 presents the process of building media corpora and training word embedding models to capture media bias. First, we reorganize the corpus for each media outlet by up-sampling to ensure that each media corpus contains the same number of news articles. The advantage of up-sampling is that it makes full use of the existing media corpus data, as opposed to discarding part of the data like down-sampling does. Second, we superimpose all 12 media corpora to construct a large base corpus and pre-train a Word2Vec model denoted as W b a s e based on it. Third, we fine-tune the same pre-trained model W b a s e using the specific corpus of each media outlet separately and get 12 fine-tuned models denoted as \({W}^{{m}_{i}}\) ( i = 1, 2, . . . 12).
In particular, the main objective of reorganizing the original corpora is to ensure that each corpus equivalently contributes to the pre-training process, in case a large corpus from certain media dominates the pre-trained model. As shown in Supplementary Information Tab. S2 , the largest corpus in 2016-2021 is from USA Today, which contains 295,518 news articles. Therefore, we can reorganize the other 11 media corpora by up-sampling to ensure that each of the 12 corpora has 295,518 articles. For example, as for NPR’s initial corpus, which has 14,654 news articles, we first repeatedly superimpose 295, 518//14, 654 = 20 times to get 293,080 articles and then randomly sample 295, 518%14, 654 = 2, 438 from the initial 14,654 articles as a supplement. Finally, we can get a reorganized NPR corpus with 295,518 articles.
Semantic Differential is a psychological technique proposed by (Osgood et al. 1957 ) to measure people’s psychological attitudes toward a given conceptual object. In the Semantic Differential theory, a given object’s semantic attributes can be evaluated in multiple dimensions. Each dimension consists of two poles corresponding to a pair of adjectives with opposite semantics (i.e., antonym pairs). The position interval between the poles of each dimension is divided into seven equally-sized parts. Then, given the object, respondents are asked to choose one of the seven parts in each dimension. The closer the position is to a pole, the closer the respondent believes the object is semantically related to the corresponding adjective. Supplementary Information Fig. S3 provides an example of Semantic Differential.
Constructing evaluation dimensions using antonym pairs in Semantic Differential is a reliable idea that aligns with how people generally evaluate things. For example, when imagining the gender-related characteristics of an occupation (e.g., nurse), individuals usually weigh between “man” and “woman”, both of which are antonyms regarding gender. Likewise, when it comes to giving an impression of the income level of the Asian race, people tend to weigh between “rich” (high income) and “poor” (low income), which are antonyms related to income. Based on such consistency, we can naturally apply Semantic Differential to measure a media outlet’s attitudes towards different entities and concepts, i.e., media bias.
Specifically, given a media m , a topic T (e.g., gender) and two semantically opposite topic word sets \(P={\{{p}_{i}\}}_{i = 1}^{{K}_{1}}\) and \(\neg P={\{\neg {p}_{i}\}}_{i = 1}^{{K}_{2}}\) about topic T , media m ’s bias towards the target x can be defined as:
Here, K 1 and K 2 denote the number of words in topic word sets P and ¬ P , respectively. W m represents the word embedding model obtained by fine-tuning W b a s e using the specific corpus of media m . \(\overrightarrow{{W}_{x}^{m}}\) is the embedding representation of the word x in W m . S i m is a similarity function used to measure the similarity between two vectors (i.e., word embeddings). In practice, we employ the cosine similarity function, which is commonly used in the field of natural language processing. In particular, equation( 5 ) calculates the difference of average similarities between the target word x and two semantically opposite topic word sets, namely P and ¬ P . Similar to the antonym pairs in Semantic Differential, such two topic word sets are used to construct the evaluation scale of media bias. In practice, to ensure the stability of the results, we have repeated this experiment five times, each time with a different random seed for up-sampling. Therefore, the final results shown in Fig. 4 are the average bias values for each topic.
The idea of recovering media bias by embedding methods
We first analyzed media bias from the aspect of event selection to study which topics a media outlet tends to focus on or ignore. Based on the GDELT database, we constructed a large “media-event" matrix that records the times each media outlet mentioned each event in news reports from February to April 2022. To extract media bias information, we referred to the idea of Latent Semantic Analysis (Deerwester et al. 1990 ) and performed Truncated SVD (Halko et al. 2011 ) on this matrix to generate vector representation (i.e., media embedding) for each media outlet (See Methods for details). Specifically, outlets with similar event selection bias (i.e., outlets that often report on events of similar topics) will have similar media embeddings. Such a bias encoded in the vector representation of each outlet is exactly the first type of media bias we aim to study.
Then, we analyzed media bias in news articles to investigate the value judgments and attitudes conveyed by media through their news articles. We collected more than 1.2 million news articles from 12 mainstream US news outlets, spanning from January 2016 to December 2021, via MediaCloud’s API. To identify media bias from each outlet’s corpus, we performed three sequential steps: (1) Pre-train a Word2Vec word embedding model based on all outlets’ corpora. (2) Fine-tune the pre-trained model by using the specific corpus of each outlet separately and obtain 12 fine-tuned models corresponding to the 12 outlets. (3) Quantify each outlet’s bias based on the corresponding fine-tuned model, combined with the idea of Semantic Differential, i.e., measuring the embedding similarities between the target words and two sets of topic words with opposite semantics (See Methods for details). An example of using Semantic Differential (Osgood et al. 1957 ) to quantify media bias is shown in Supplementary Information Fig. S4 .
Media show significant clustering due to their regions and organizations
In this experiment, we aimed to capture and analyze the event selection bias of different media outlets based on the proposed media embedding methodology. To achieve a comprehensive analysis, we selected 247 media outlets from 8 countries ( Supplementary Information Tab. S6) , including the United States, the United Kingdom, Canada, Australia, Ireland, and New Zealand-six English-speaking nations with India and China, two populous countries. For each country, we chose media outlets that were the most active during February-April 2022, with media activity measured by the quantity of news reports. We then generated embedding representations for each media outlet via Truncated SVD and performed K-means clustering (Lloyd, 1982 ; MacQueen, 1967 ) on the obtained media embedding representations (with K = 10) for further analysis. Details of the experiment are presented in the first section of the supplementary Information. Figure 2 visualizes the clustering results.
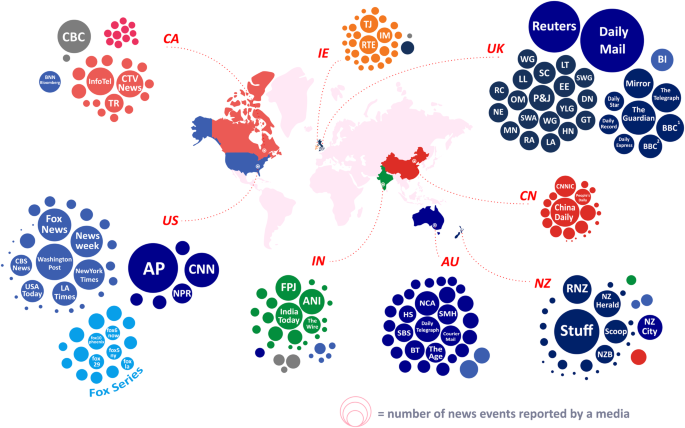
There are 247 media outlets from 8 countries: Canada (CA), Ireland (IE), United Kingdom (UK), China (CN), United States (US), India (IN), Australia (AU), and New Zealand (NZ). Each circle in the visualization represents a media outlet, with its color indicating the cluster it belongs to, and its diameter proportional to the number of events reported by the outlet between February and April 2022. The text in each circle represents the name or abbreviation of a media outlet (See Supplementary Information Tab. S6 for details). The results indicate that media outlets from the same country tend to be grouped together in clusters. Moreover, the majority of media outlets in the Fox series form a distinct cluster, indicating a high degree of similarity in their event selection bias.
First, we find that media outlets from different countries tend to form distinct clusters, signifying the regional nature of media bias. Specifically, we can interpret Fig. 2 from two different perspectives, and both come to this conclusion. On the one hand, most media outlets from the same country tend to appear in a limited number of clusters, which suggests that they share similar event selection bias. On the other hand, as we can see, media outlets in the same cluster mostly come from the same country, indicating that media exhibiting similar event selection bias tends to be from the same country. In our view, differences in geographical location lead to diverse initial event information accessibility for media outlets from different regions, thus shaping the content they choose to report.
Besides, we observe an intriguing pattern where the Associated Press (AP) and Reuters, despite their geographical separation, share similar event selection biases as they are clustered together. This abnormal phenomenon could be attributed to their status as international media outlets, which enables them to cover various global events, thus leading to extensive overlapping news coverage. In addition, 16 out of the 21 Fox series media outlets form a distinct cluster on their own, suggesting that a media outlet’s bias is strongly associated with the organization it belongs to. After all, media outlets within the same organization often tend to prioritize or overlook specific events due to shared positions, interests, and other influencing factors.
International hot topics drive media bias to converge
Previous results have revealed a significant correlation between media bias and the location of a media outlet. Therefore, we conducted an experiment to further investigate the event selection biases of media outlets from 25 different countries. To achieve this, we gathered GDELT data spanning from February to April 2022 and created three “media-event” matrices on a monthly basis. We then subjected each month’s “media-event” matrix to the same processing steps: (1) generating an embedding representation for each media outlet through matrix decomposition, (2) obtaining the embedding representation of each media outlet that belongs to each country to construct a media embedding set, and (3) calculating the similarity between every two countries (i.e., each two media embedding sets) using Word Mover Distance (WMD (Kusner et al. 2015 )) as the similarity metric (See Methods for details). Figure 3 presents the changes in event selection bias similarity amongst media outlets from different countries between February and April 2022.
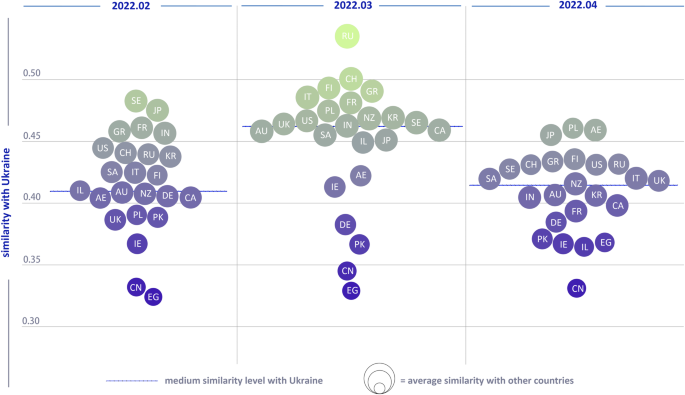
The horizontal axis in this figure represents the time axis, measured in months. Meanwhile, the vertical axis indicates the event selection similarity between Ukrainian media and media from other countries. Each circle represents a country, with the font inside it representing the corresponding country’s abbreviation (see details in Supplementary Information Tab. S3) . The size of a circle corresponds to the average event selection similarity between the media of a specific country and the media of all other countries. The color of the circle corresponds to the vertical axis scale. The blue dotted line’s ordinate represents the median similarity to Ukrainian media.
We find that the similarities between Ukraine and other countries peaked significantly in March 2022. This result aligns with the timeline of the Russia-Ukraine conflict: the conflict broke out around February 22, attracting media attention worldwide. In March, the conflict escalated, and the regional situation became increasingly tense, leading to even more media coverage worldwide. By April, the prolonged conflict had made the international media accustomed to it, resulting in a decline in media interest. Furthermore, we observed that the event selection biases of media outlets in both EG (Egypt) and CN (China) differed significantly from those of other countries. Given that both countries are not predominantly English-speaking, their English-language media outlets may have specific objectives such as promoting their national image and culture, which could influence and constrain the topics that a media outlet tends to cover.
Additionally, we observe that in March 2022, the country with the highest similarity to Ukraine was Russia, and in April, it was Poland. This change can be attributed to the evolving regional situation. In March, when the conflict broke out, media reports primarily focused on the warring parties, namely Russia and Ukraine. As the war continued, the impact of the war on Ukraine gradually became the focus of media coverage. For instance, the war led to the migration of a large number of Ukrainian citizens to nearby countries, among which Poland received the most citizens of Ukraine at that time.
Media shows diverse biases on different topics
In this experiment, we took 12 mainstream US news outlets as examples and conducted a quantitative media bias analysis on three typical topics (Fan and Gardent, 2022 ; Puglisi and Snyder Jr, 2015b ; Sun and Peng, 2021 ): Gender bias (about occupation); Political bias (about the American state); Income bias (about race & ethnicity). The topic words for each topic are listed in Supplementary Information Tab. S4 . These topic words are sourced from related literature (Caliskan et al. 2017 ), and search engines, along with the authors’ intuitive assessments.
Gender bias in terms of Occupation
In news coverage, media outlets may intentionally or unintentionally associate an occupation with a particular gender (e.g., stereotypes like police-man, nurse-woman). Such gender bias can subtly affect people’s attitudes towards different occupations and even impact employment fairness. To analyze gender biases in news coverage towards 8 common occupations (note that more occupations can be studied using the same methodology), we examined 12 mainstream US media outlets. As shown in Fig. 4 a, all these outlets tend to associate “teacher” and “nurse” with women. In contrast, when reporting on “police,” “driver,” “lawyer,” and “scientist,” most outlets show bias towards men. As for “director” and “photographer,” only slightly more than half of the outlets show bias towards men. Supplementary Information Tab. S5 shows the proportion of women in the eight occupations in America according to U.S. Bureau of Labor Statistics Footnote 7 . Women’s proportions in “teacher” and “nurse” dominate, while men’s in “police,” “driver,” and “lawyer” are significantly higher. Besides, among “directors,” “scientists,” and “photographers,” the proportions of women and men are about the same. Comparing the experiment results with USCB’s statistics, we find that these media outlets’ gender bias towards an occupation is highly consistent with the actual women (or men) ratio in the occupation. Such a phenomenon highlights the potential for media outlets to perpetuate and reinforce existing gender bias in society, emphasizing the need for increased awareness and attention to media bias. Note that we reorganized the corpus of each media outlet by up-sampling during the data preprocessing process, which introduced some randomness to the experiment results (See Methods for details). Therefore, we set five different random seeds for up-sampling and repeated the experiment mentioned above five times. A two-tailed t-test on the difference between the results shown in Fig. 4 a and the results of current repeated experiments showed no significant difference ( Supplementary Information Fig. S6) .
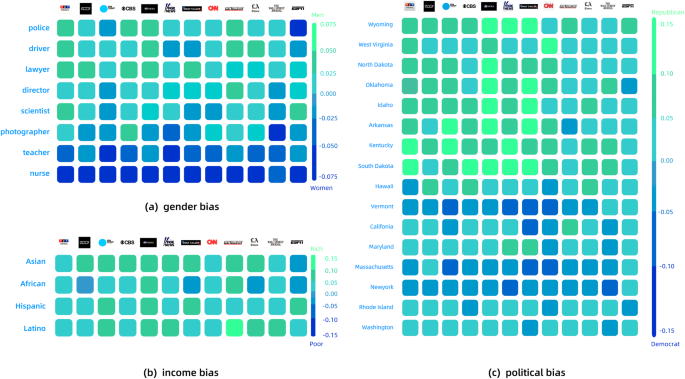
Each column corresponds to a media outlet, and each row corresponds to a target word which usually means an entity or concept in the news text. The color bar on the right describes the value range of the bias value, with each interval of the bias value corresponding to a different color. As the bias value changes from negative to positive, the corresponding color changes from purple to yellow. Because the range of bias values differs across each topic, the color bar of different topics can also vary. The color of each heatmap square corresponds to an interval in the color bar. Specifically, the square located in row i and column j represents the bias of media j when reporting on target i. a Gender bias about eight common occupations. b Income bias about four races or ethnicities. c Political bias about the top-10 “red state” (Wyoming, West Virginia, North Dakota, Oklahoma, Idaho, Arkansas, Kentucky, South Dakota, Alabama, Texas) and the top-10 “blue state” (Hawaii, Vermont, California, Maryland, Massachusetts, New York, Rhode Island, Washington, Connecticut, Illinois) according to the CPVI ranking (Ardehaly and Culotta, 2017 ). Limited by the page layout, only the top-8 results are shown here. Please refer to Supplementary Information Fig. S5 for the complete results.
Income bias in terms of Race and Ethnicity
Media coverage often discusses the income of different groups of people, including many races and ethnicities. Here, we aim to investigate whether the media outlets are biased in their income coverage, such as associating a specific race or ethnicity with rich or poor. To this end, we selected four US racial and ethnic groups as research subjects: Asian, African, Hispanic, and Latino. In line with previous studies (Grieco and Cassidy, 2015 ; Nerenz et al. 2009 ; Perez and Hirschman, 2009 ), we considered Asian and African as racial categories and Hispanic and Latino as ethnic categories. Referring to the income statistics from USCB Footnote 8 , we do not strictly distinguish these concepts and compare them together. As shown in Fig. 4 b, for the majority of media outlets, Asian is most frequently associated with the rich, with ESPN being the only exception. This anomalous finding may be attributed to ESPN’s position as a sports media, with a primary emphasis on sports that are particularly popular with Hispanic, Latino, and African-American audiences, such as soccer, basketball, and golf. Additionally, there is a significant disparity in the media’s coverage of income bias toward Africans, Hispanics, and Latinos. Specifically, the biases towards Hispanic and Latino populations are generally comparable, with both groups being portrayed as richer than African Americans in most media coverage. Referring to the aforementioned income statistics of the U.S. population, the income rankings of different races and ethnicities have remained stable from 1950 to 2020: Asians have consistently had the highest income, followed by Hispanics with the second-highest income, and African Americans with the lowest income (the income of Black Americans is used as an approximation for African Americans). It is worth noting that USCB considers Hispanic and Latino to be the same ethnicity, although there are some controversies surrounding this practice (Mora, 2014 ; Rodriguez, 2000 ). However, these controversies are not the concern of this work, so we use Hispanic income as an approximation of Latino income following USCB. Comparing our experiment results with USCB’s income statistics, we find that the media outlets’ income bias towards different races and ethnicities is roughly consistent with their actual income levels. A two-tailed t-test on the difference between the results shown in Fig. 4 b and the results of repeated experiments showed no significant difference ( Supplementary Information Fig. S7) .
Political bias in terms of Region
Numerous studies have shown that media outlets tend to publish politically biased news articles that support the political parties they favor while criticizing those they oppose (Lazaridou et al. 2020 ; Puglisi, 2011 ). For example, a report from the Red State described liberals as regressive leftists with mental health issues. Conversely, a story from Right Wing News reported that Obama’s administration was terrible (Lazaridou et al. 2020 ). Such political inclinations will hinder readers’ objective judgment of political events and affect their attitudes toward different political parties. Therefore, we analyzed the political biases of 12 mainstream US media outlets when talking about different US states, aiming to increase public awareness of such biases in news coverage. As shown in Fig. 4 c, in the reports of these media outlets, most red states lean Republican, while most blue states lean Democrat. In particular, some blue states also show a leaning toward Republicans, such as Hawaii and Maryland. Such an abnormal phenomenon can be attributed to the source of the corpus data used in this study. The corpus data, which was used to train word embedding models, spans from January 2016 to December 2021. During this period, the Republican Party was in power, with Trump serving as president from January 2017 to January 2021. Thus, the majority of the data was collected during the Republican administration. We suggest that Trump’s presidency resulted in increased media coverage of the Republican Party, thus causing some blue states to be associated more frequently with Republicans in news reports. A two-tailed t-test on the difference between the results shown in Fig. 4 c and the results of repeated experiments showed no significant difference ( Supplementary Information Fig. S8 and Fig. S9) .
Media logic and news evaluation are two important concepts in social science. The former refers to the rules, conventions, and strategies that the media follow in the production, dissemination, and reception of information, reflecting the media’s organizational structure, commercial interests, and socio-cultural background (Altheide, 2015 ). The latter refers to the systematic analysis of the quality, effectiveness, and impact of news reports, involving multiple criteria and dimensions such as truthfulness, accuracy, fairness, balance, objectivity, diversity, etc. When studying media bias issues, media logic provides a framework for understanding the rules and patterns of media operations, while news evaluation helps identify and analyze potential biases in media reports. For example, to study media’s political bias, (D’heer, 2018 ; Esser and Strömbäck, 2014 ) compare the frameworks, languages, and perspectives used by traditional news media and social media in reporting political elections, so as to understand the impact of these differences on voters’ attitudes and behaviors. However, in spite of the progress, these methods often rely on manual observation and interpretation, thus inefficient and susceptible to human bias and errors.
In this work, we propose an automated media bias analysis framework that enables us to uncover media bias on a large scale. To carry out this study, we amassed an extensive dataset, comprising over 8 million event records and 1.2 million news articles from a diverse range of media outlets (see details of the data collection process in Methods). Our research delves into media bias from two distinct yet highly pertinent perspectives. From the macro perspective, we aim to uncover the event selection bias of each media outlet, i.e., which types of events a media outlet tends to report on. From the micro perspective, our goal is to quantify the bias of each media outlet in wording and sentence construction when composing news articles about the selected events. The experimental results align well with our existing knowledge and relevant statistical data, indicating the effectiveness of embedding methods in capturing the characteristics of media bias. The methodology we employed is unified and intuitive and follows a basic idea. First, we train embedding models using real-world data to capture and encode media bias. At this step, based on the characteristics of different types of media bias, we choose appropriate embedding methods to model them respectively (Deerwester et al. 1990 ; Le and Mikolov, 2014 ; Mikolov et al. 2013 ). Then, we utilize various methods, including cluster analysis (Lloyd, 1982 ; MacQueen, 1967 ), similarity calculation (Kusner et al. 2015 ), and semantic differential (Osgood et al. 1957 ), to extract media bias information from the obtained embedding models.
To capture the event selection biases of different media outlets, we employ Truncated SVD (Halko et al. 2011 ) on the “media-event” matrix to generate media embeddings. Truncated SVD is a widely used technique in NLP. In particular, LSA (Deerwester et al. 1990 ) applies Truncated SVD to the “document-word” matrix to capture the underlying topic-based semantic relationships between text documents and words. LSA assumes that a document tends to use relevant words when it talks about a particular topic and obtains the vector representation for each document in a latent topic space, where documents talking about similar topics are located near each other. By analogizing media outlets and events with documents and words, we can naturally apply Truncate SVD to explore media bias in the event selection process. Specifically, we assume that there are underlying topics when considering a media outlet’s event selection bias. If a media focuses on a topic, it will tend to report events related to that topic and otherwise ignore them. Therefore, media outlets sharing similar event selection biases (i.e., tend to report events about similar topics) will be close to each other in the latent topic space, which provides a good opportunity for us to study media bias (See Methods and Results for details).
When describing something, relevant contexts must be considered. For instance, positive and negative impressions are conveyed through the use of context words such as “diligent” and “lazy”, respectively. Similarly, a media outlet’s attitude towards something is reflected in the news context in which it is presented. Here, we study the association between each target and its news contexts based on the co-occurrence relationship between words. Our underlying assumption is that frequently co-occurring words are strongly associated, which aligns with the idea of word embedding models (Kenton and Toutanova, 2019 ; Le and Mikolov, 2014 ; Mikolov et al. 2013 ), where the embeddings of frequently co-occurring words are relatively similar. For example, suppose that in the corpus of media M, the word “scientist” often co-occurs with female-related words (e.g., “woman” and “she”, etc.) but rarely with those male-related words. Then, the semantic similarities of “scientist” with female-related words should be much higher than those of male-related words in the word embedding model. Therefore, we can conclude that media M’s reports on scientists are biased towards women.
According to the theory of Semantic Differential (Osgood et al. 1957 ), the difference in semantic similarities between “scientist” and female-related words versus male-related words can serve as an estimation of media M’s gender bias. Since we have kept all settings (e.g., corpus size, starting point for model fine-tuning, etc.) the same when training word embedding models for different media outlets, the estimated bias values can be interpreted as absolute ones within the same reference system. In other words, the estimated bias values for different media outlets are directly comparable in this study, with a value of 0 denoting unbiased and a value closer to 1 or -1 indicating a more pronounced bias.
We notice that there has been literature investigating the choice of events/topics and words/frames to measure media bias, such as partisan and ideological biases (Gentzkow et al. 2015 ; Puglisi and Snyder Jr, 2015b ). However, our approach not only considers bias related to the selective reporting of events (using event embedding) but also studies biased wording in news texts (using word embedding). While the former focuses on the macro level, the latter examines the micro level. These two perspectives are distinct yet highly relevant, but previous studies often only consider one of them. For the choice of events/topics, our approach allows us to explore how they change over time. For example, we can analyze the time-changing similarities between media outlets from different countries, as shown in Fig. 3 . For the choice of words/frames, prior work has either analyzed specific biases based on the frequency of particular words (Gentzkow and Shapiro, 2010 ; Gentzkow et al. 2006 ), which fails to capture deeper semantics in media language or analyzed specific biases by merely aggregating the analysis results for every single article in the corpus (e.g., calculating the sentiment (Gentzkow et al. 2006 ; Lott Jr and Hassett, 2014 ; Soroka, 2012 ) of each article or its similarity with certain authorship (Gentzkow and Shapiro, 2010 ; Groseclose and Milyo, 2005 ), then summing them up as the final bias value), without considering the relationships between different articles, thus lacking a holistic nature. In contrast, our method, based on word embeddings (Le and Mikolov, 2014 ; Mikolov et al. 2013 ), directly models the semantic associations between all words and entities in the corpus with a neural network, offering advantages in capturing both semantic meaning and holistic nature. Specially, we not only utilize word embedding techniques but also integrate them with appropriate psychological/sociological theories, such as the Semantic Differential theory and the Cognitive Miser theory. These theories endow our approach with better interpretability. In addition, the method we propose is a generalizable framework for studying media bias using embedding techniques. While this study has focused on validating its effectiveness with specific types of media bias, it can actually be applied to a broader range of media bias research. We will expand the application of this framework in future work.
As mentioned above, our proposed framework examines media bias from two distinct but highly relevant perspectives. Here, taking the significant Russia-Ukraine conflict event as an example, we will demonstrate how these two perspectives contribute to providing researchers and the public with a more comprehensive and objective assessment of media bias. For instance, we can gather relevant news articles and event reporting records about the ongoing Russia-Ukraine conflict from various media outlets worldwide and generate media and word embedding models. Then, according to the embedding similarities of different media outlets, we can judge which types of events each media outlet tends to report and select some media that tend to report on different events. By synthesizing the news reports of the selected media, we can gain a more comprehensive understanding of the conflict instead of being limited to the information selectively provided by a few media. Besides, based on the word embedding model and the bias estimation method based on Semantic Differential, we can objectively judge each media’s attitude towards Russia and Ukraine (e.g., whether a media tends to use positive or negative words to describe either party). Once a news outlet is detected as apparently biased, we should read its articles more carefully to avoid being misled.
In the end, despite the advantages of our framework, there are still some shortcomings that need improvement. First, while the media embeddings generated based on matrix decomposition have successfully captured media bias in the event selection process, interpreting these continuous numerical vectors directly can be challenging. We hope that future work will enable the media embedding to directly explain what a topic exactly means and which topics a media outlet is most interested in, thus helping us understand media bias better. Second, since there is no absolute, independent ground truth on which events have occurred and should have been covered, the aforementioned media selection bias, strictly speaking, should be understood as relative topic coverage, which is a narrower notion. Third, for topics involving more complex semantic relationships, estimating media bias using scales based on antonym pairs and the Semantic Differential theory may not be feasible, which needs further investigation in the future.
Data availability
The data that support the findings of this study are available at https://github.com/CGCL-codes/media-bias .
Code availability
The code that supports the findings of this study is also available at https://github.com/CGCL-codes/media-bias .
https://fair.org/home/when-both-sides-are-covered-in-verizon-strike-bosses-side-is-heard-more/ .
These views were extracted from reports by some mainstream US media outlets in 2022 when the Democratic Party (left-wing) was in power.
https://www.nytimes.com/2022/09/26/world/middleeast/women-iran-protests-hijab.html .
https://www.nytimes.com/2014/08/08/world/asia/uighurs-veils-a-protest-against-chinas-curbs.html .
https://www.gdeltproject.org/ .
https://mediacloud.org/ .
https://www.bls.gov/cps/cpsaat11.htm .
https://www.census.gov/content/dam/Census/library/publications/2021/demo/p60-273.pdf .
Altheide, DL (2015) Media logic. The international encyclopedia of political communication, pages 1–6
Ansolabehere S, Lessem R, Snyder Jr JM (2006) The orientation of newspaper endorsements in us elections, 1940–2002. Quarterly Journal of political science 1(4):393
Article Google Scholar
Ardehaly, EM, Culotta, A (2017) Mining the demographics of political sentiment from twitter using learning from label proportions. In 2017 IEEE international conference on data mining (ICDM), pages 733–738. IEEE
Baron DP (2006) Persistent media bias. Journal of Public Economics 90(1-2):1–36
Article ADS Google Scholar
Bovet A, Makse HA (2019) Influence of fake news in twitter during the 2016 us presidential election. Nature communications 10(1):1–14
Caliskan A, Bryson JJ, Narayanan A (2017) Semantics derived automatically from language corpora contain human-like biases. Science 356(6334):183–186
Article ADS CAS PubMed Google Scholar
D’Alessio D, Allen M (2000) Media bias in presidential elections: A meta-analysis. Journal of communication 50(4):133–156
Deerwester S, Dumais ST, Furnas GW, Landauer TK, Harshman R (1990) Indexing by latent semantic analysis. Journal of the American society for information science 41(6):391–407
DellaVigna S, Kaplan E (2008) The political impact of media bias. Information and Public Choice, page 79
Downs A (1957) An economic theory of political action in a democracy. Journal of political economy 65(2):135–150
D’heer E (2018) Media logic revisited. the concept of social media logic as alternative framework to study politicians’ usage of social media during election times. Media logic (s) revisited: Modelling the interplay between media institutions, media technology and societal change, pages 173–194
Esser F, Strömbäck J (2014) Mediatization of politics: Understanding the transformation of Western democracies. Springer
Fan A, Gardent, C (2022) Generating biographies on Wikipedia: The impact of gender bias on the retrieval-based generation of women biographies. In Proceedings of the Conference of the 60th Annual Meeting of the Association for Computational Linguistics (ACL)
Firth, JR (1957) A synopsis of linguistic theory, 1930–1955. Studies in linguistic analysis
Fiske ST, Taylor SE (1991) Social cognition. Mcgraw-Hill Book Company
Galtung J, Ruge MariHolmboe (1965) The structure of foreign news: The presentation of the congo, cuba and cyprus crises in four norwegian newspapers. Journal of peace research 2(1):64–90
Gentzkow M, Shapiro JM (2010) What drives media slant? evidence from us daily newspapers. Econometrica 78(1):35–71
Article MathSciNet Google Scholar
Gentzkow M, Glaeser EL, Goldin C (2006) The rise of the fourth estate. how newspapers became informative and why it mattered. In Corruption and reform: Lessons from America’s economic history, pages 187–230. University of Chicago Press
Gentzkow M, Shapiro JM, Stone DF (2015) Media bias in the marketplace: Theory. In Handbook of Media Economics, volume 1, pages 623–645. Elsevier
Grand G, Blank IdanAsher, Pereira F, Fedorenko E (2022) Semantic projection recovers rich human knowledge of multiple object features from word embeddings. Nature Human Behaviour 6(7):975–987
Article PubMed PubMed Central Google Scholar
Grieco EM, Cassidy RC (2015) Overview of race and hispanic origin: Census 2000 brief. In ’Mixed Race’Studies, pages 225–243. Routledge
Groseclose T, Milyo J (2005) A measure of media bias. The Quarterly Journal of Economics 120(4):1191–1237
Grossmann, Matt and Hopkins, David A (2016) Asymmetric politics: Ideological Republicans and group interest Democrats . Oxford University Press
Halko N, Martinsson Per-Gunnar, Tropp JA (2011) Finding structure with randomness: Probabilistic algorithms for constructing approximate matrix decompositions. SIAM review 53(2):217–288
Hamborg F, Donnay K, Gipp B (2019) Automated identification of media bias in news articles: an interdisciplinary literature review. International Journal on Digital Libraries 20(4):391–415
Haraldsson A, Wängnerud L (2019) The effect of media sexism on women’s political ambition: evidence from a worldwide study. Feminist media studies 19(4):525–541
Harcup T, O’neill D (2001) What is news? galtung and ruge revisited. Journalism studies 2(2):261–280
Harcup T, O’neill D (2017) What is news? news values revisited (again). Journalism studies 18(12):1470–1488
Harris ZS (1954) Distributional structure. Word 10(2-3):146–162
Harwood TG, Garry T (2003) An overview of content analysis. The marketing review 3(4):479–498
Ho DE, Quinn KM et al. (2008) Measuring explicit political positions of media. Quarterly Journal of Political Science 3(4):353–377
Huang H, Chen Z, Shi X, Wang C, He Z, Jin H, Zhang M, Li Z (2021) China in the eyes of news media: a case study under covid-19 epidemic. Frontiers of Information Technology & Electronic Engineering 22(11):1443–1457
Huang P-S, Zhang H, Jiang R, Stanforth R, Welbl J, Rae J, Maini V, Yogatama D, Kohli P (2020) Reducing sentiment bias in language models via counterfactual evaluation. In Findings of the Association for Computational Linguistics: EMNLP 2020, pages 65–83
Devlin J, Chang MW, Lee K, Toutanova K. Bert: Pre-training of deep bidirectional transformers for language understanding. In Proceedings of NAACL-HLT, pages 4171–4186, (2019)
Kusner M, Sun Y, Kolkin N, Weinberger K. From word embeddings to document distances. In International conference on machine learning, pages 957–966. PMLR, (2015)
Larcinese V, Puglisi R, Snyder Jr JM (2011) Partisan bias in economic news: Evidence on the agenda-setting behavior of us newspapers. Journal of public Economics 95(9–10):1178–1189
Lazaridou K, Löser A, Mestre M, Naumann F (2020) Discovering biased news articles leveraging multiple human annotations. In Proceedings of the 12th Language Resources and Evaluation Conference, pages 1268–1277
Le, Q, Mikolov, T (2014) Distributed representations of sentences and documents. In International conference on machine learning, pages 1188–1196. PMLR
Liu, R, Jia, C, Wei, J, Xu, G, Wang, L, Vosoughi, S (2021) Mitigating political bias in language models through reinforced calibration. In Proceedings of the AAAI Conference on Artificial Intelligence, volume 35, pages 14857–14866
Liu R, Wang L, Jia, C, Vosoughi, S (2021) Political depolarization of news articles using attribute-aware word embeddings. In Proceedings of the 15th International AAAI Conference on Web and Social Media (ICWSM 2021)
Lloyd S (1982) Least squares quantization in pcm. IEEE transactions on information theory 28(2):129–137
Lott Jr JR, Hassett KA (2014) Is newspaper coverage of economic events politically biased? Public Choice 160(1–2):65–108
Lühiste M, Banducci S (2016) Invisible women? comparing candidates’ news coverage in Europe. Politics & Gender 12(2):223–253
MacGregor, B (1997) Live, direct and biased?: Making television news in the satellite age
MacQueen, J (1967) Classification and analysis of multivariate observations. In 5th Berkeley Symp. Math. Statist. Probability, pages 281–297
Merloe P (2015) Authoritarianism goes global: Election monitoring vs. disinformation. Journal of Democracy 26(3):79–93
Mikolov T, Chen K, Corrado GS, Dean J (2013) Efficient estimation of word representations in vector space. In International Conference on Learning Representations
Mora, GC (2014) Making Hispanics: How activists, bureaucrats, and media constructed a new American. University of Chicago Press
Nerenz DR, McFadden B, Ulmer C et al. (2009) Race, ethnicity, and language data: standardization for health care quality improvement
Niven, David (2002). Tilt?: The search for media bias. Greenwood Publishing Group
Osgood, Charles Egerton, Suci, George J and Tannenbaum, Percy H (1957) The measurement of meaning. Number 47. University of Illinois Press
Papacharissi Z, de Fatima Oliveira M (2008) News frames terrorism: A comparative analysis of frames employed in terrorism coverage in US and UK newspapers. The international journal of press/politics 13(1):52–74
Park S, Kang S, Chung, S, Song, J (2009) Newscube: delivering multiple aspects of news to mitigate media bias. In Proceedings of the SIGCHI conference on human factors in computing systems, pages 443–452
Paul R, Elder L (2004) The thinkers guide for conscientious citizens on how to detect media bias & propaganda in national and world news: Based on critical thinking concepts & tools
Perez AnthonyDaniel, Hirschman C (2009) The changing racial and ethnic composition of the US population: Emerging American identities. Population and development review 35(1):1–51
Puglisi, R (2011) Being the New York times: the political behaviour of a newspaper. The BE journal of economic analysis & policy 11(1)
Puglisi R, Snyder Jr JM (2015a) The balanced US press. Journal of the European Economic Association 13(2):240–264
Puglisi, Riccardo and Snyder Jr, James M (2015b) Empirical studies of media bias. In Handbook of media economics, volume 1, pages 647–667. Elsevier
Qiang J, Zhang F, Li Y, Yuan Y, Zhu Y, Wu X (2023) Unsupervised statistical text simplification using pre-trained language modeling for initialization. Frontiers of Computer Science 17(1):171303
Rodriguez, CE (2000) Changing race: Latinos, the census, and the history of ethnicity in the United States, volume 41. NYU Press
Ross K, Carter C (2011) Women and news: A long and winding road. Media, Culture & Society 33(8):1148–1165
Sahlgren M (2008) The distributional hypothesis. Italian Journal of Disability Studies 20:33–53
Google Scholar
Soroka SN (2012) The gatekeeping function: distributions of information in media and the real world. The Journal of Politics 74(2):514–528
Stanovich KE (2009) What intelligence tests miss: The psychology of rational thought. Yale University Press
Stroud NatalieJomini (2010) Polarization and partisan selective exposure. Journal of Communication 60(3):556–576
Sun J, Peng N (2021) Men are elected, women are married: Events gender bias on wikipedia. In Proceedings of the Conference of the 59th Annual Meeting of the Association for Computational Linguistics (ACL)
Sunstein C (2002) The law of group polarization. Journal of Political Philosophy 10:175–195
Tahmasbi F, Schild L, Ling C, Blackburn J, Stringhini G, Zhang Y, Zannettou S (2021) “go eat a bat, chang!”: On the emergence of sinophobic behavior on web communities in the face of covid-19. In Proceedings of the Web Conference, pages 1122–1133
Vaismoradi M, Turunen H, Bondas T (2013) Content analysis and thematic analysis: Implications for conducting a qualitative descriptive study. Nursing & health sciences 15(3):398–405
Wang T, Lin XV, Rajani NF, McCann B, Ordonez V, Xiong, C (2020). Double-hard debias: Tailoring word embeddings for gender bias mitigation. In Proceedings of the 58th Annual Meeting of the Association for Computational Linguistics, pages 5443–5453
White DavidManning (1950) The “gate keeper”: a case study in the selection of news. Journalism Quarterly 27(4):383–390
Zeng Y, Li Z, Chen Z, Ma H (2023) Aspect-level sentiment analysis based on semantic heterogeneous graph convolutional network. Frontiers of Computer Science 17(6):176340
Zhang Y, Wang H, Yin G, Wang T, Yu Y (2017) Social media in github: the role of@-mention in assisting software development. Science China Information Sciences 60(3):1–18
Download references
Acknowledgements
The work is supported by the National Natural Science Foundation of China (No. 62127808).
Author information
Authors and affiliations.
National Engineering Research Center for Big Data Technology and System, Wuhan, China
Hong Huang, Hua Zhu & Hai Jin
Services Computing Technology and System Lab, Wuhan, China
Cluster and Grid Computing Lab, Wuhan, China
School of Computer Science and Technology, Wuhan, China
Hong Huang, Hua Zhu, Wenshi Liu & Hai Jin
Huazhong University of Science and Technology, Wuhan, China
Hong Huang, Hua Zhu, Wenshi Liu, Hua Gao & Hai Jin
DIRO, Université de Montréal & Mila & Canada CIFAR AI Chair, Montreal, Canada
You can also search for this author in PubMed Google Scholar
Contributions
HH: conceptualization, writing-review & editing, supervision; HZ: software, writing-original draft, data curation; WSL: software; HG and HJ: resources; BL: methodology, writing-review & editing, supervision.
Corresponding author
Correspondence to Hong Huang .
Ethics declarations
Competing interests.
The authors declare no competing interests.
Ethical approval
Ethical approval is not required as the study does not involve human participants.
Informed consent
This article does not contain any studies with human participants performed by any of the authors.
Additional information
Publisher’s note Springer Nature remains neutral with regard to jurisdictional claims in published maps and institutional affiliations.
Supplementary information
Supplementary material, rights and permissions.
Open Access This article is licensed under a Creative Commons Attribution 4.0 International License, which permits use, sharing, adaptation, distribution and reproduction in any medium or format, as long as you give appropriate credit to the original author(s) and the source, provide a link to the Creative Commons licence, and indicate if changes were made. The images or other third party material in this article are included in the article’s Creative Commons licence, unless indicated otherwise in a credit line to the material. If material is not included in the article’s Creative Commons licence and your intended use is not permitted by statutory regulation or exceeds the permitted use, you will need to obtain permission directly from the copyright holder. To view a copy of this licence, visit http://creativecommons.org/licenses/by/4.0/ .
Reprints and permissions
About this article
Cite this article.
Huang, H., Zhu, H., Liu, W. et al. Uncovering the essence of diverse media biases from the semantic embedding space. Humanit Soc Sci Commun 11 , 656 (2024). https://doi.org/10.1057/s41599-024-03143-w
Download citation
Received : 26 February 2023
Accepted : 07 May 2024
Published : 22 May 2024
DOI : https://doi.org/10.1057/s41599-024-03143-w
Share this article
Anyone you share the following link with will be able to read this content:
Sorry, a shareable link is not currently available for this article.
Provided by the Springer Nature SharedIt content-sharing initiative
Quick links
- Explore articles by subject
- Guide to authors
- Editorial policies

Log in using your username and password
- Search More Search for this keyword Advanced search
- Latest content
- Current issue
- BMJ Journals More You are viewing from: Google Indexer
You are here
- Online First
- Pilot study on large language models for risk-of-bias assessments in systematic reviews: A(I) new type of bias?
- Article Text
- Article info
- Citation Tools
- Rapid Responses
- Article metrics

- http://orcid.org/0000-0001-7989-6994 Joseph Barsby 1 , 2 ,
- http://orcid.org/0000-0003-4417-0370 Samuel Hume 1 , 3 ,
- http://orcid.org/0000-0002-7679-9125 Hamish AL Lemmey 1 , 4 ,
- http://orcid.org/0000-0002-2867-7393 Joseph Cutteridge 5 , 6 ,
- http://orcid.org/0000-0001-8621-5165 Regent Lee 7 ,
- http://orcid.org/0000-0003-3795-6762 Katarzyna D Bera 3 , 7
- 1 Oxford Medical School , Oxford , UK
- 2 Newcastle Upon Tyne Hospitals NHS Foundation Trust , Newcastle Upon Tyne , UK
- 3 University of Oxford St Anne's College , Oxford , UK
- 4 University of Oxford Magdalen College , Oxford , UK
- 5 York and Scarborough Teaching Hospitals NHS Foundation Trust , York , UK
- 6 Hull University Teaching Hospitals NHS Trust , Hull , UK
- 7 Nuffield Department of Surgical Sciences , University of Oxford , Oxford , UK
- Correspondence to Dr Katarzyna D Bera, University of Oxford Nuffield Department of Surgical Sciences, Oxford, UK; katarzyna.bera{at}st-annes.ox.ac.uk
https://doi.org/10.1136/bmjebm-2024-112990
Statistics from Altmetric.com
Request permissions.
If you wish to reuse any or all of this article please use the link below which will take you to the Copyright Clearance Center’s RightsLink service. You will be able to get a quick price and instant permission to reuse the content in many different ways.
- Systematic Reviews as Topic
Risk-of-bias (RoB) assessment is used to assess randomised control trials for systematic errors. Developed by Cochrane, it is considered the gold standard of assessing RoB for studies included within systematic reviews, representing a key part of Preferred Reporting Items for Systematic Reviews and Meta-Analyses guidelines. 1 The RoB tool comprises six domains that may signify bias: random sequence generation, allocation concealment, blinding of participants and personnel, attrition bias, reporting bias and other potential biases. 2 This assessment is an integral component of evaluating the quality of evidence; however, it is a time-consuming and labour-intensive process.
Large language models (LLMs) are a form of generative artificial intelligence (AI) trained on large volumes of data. ChatGPT is an LLM developed by OpenAI, capable of generating a wide variety of responses in response to user prompts. Concerns exist around the application of such AI tools in research, including ethical, copyright, plagiarism and cybersecurity risks. 3 However, LLMs are increasingly popular with investigators seeking to streamline analyses. Studies have begun investigating the potential role of LLMs in the RoB assessment process. 4 5 Given the flexibility and rapidly evolving nature of LLMs, our goal was to explore whether ChatGPT could be used to automate the RoB assessment process without sacrificing accuracy. This study offers an assessment of the applicability of LLMs in SRs as of December 2023.
This study sits within an SR (PROSPERO CRD420212479050). Two reviewers (SH and HALL) implemented RoB across n=15 full-length papers in portable document format (PDF) format ( table 1 ). Six domains were assessed independently alongside an added ‘overall assessment’ domain ranking each as high, low or unclear RoB. Alongside RoB assessment, reviewers recorded author name, DOI and publication year using a Microsoft Office form. Any conflicts were resolved by discussion, with a third reviewer (KDB) available for arbitration, although this was not required.
- View inline
List of included papers (n=15), numbered 1–15
In parallel, a fourth reviewer (JB) uploaded the same PDF files to ChatPDF.com, a plug-in powered by ChatGPT3.5 that facilitates the upload of PDF files for analysis by ChatGPT3.5. ChatGPT3.5 was prompted to assess the RoB within each paper through prompts pertaining to each of the six domains. Responses were recorded in a separate, but identical, Microsoft Office form. Reviewer 4 (JB) was blinded to the assessment of reviewers 1 and 2 throughout. JB then repeated this process using ChatGPT4.
Responses from decisions across all domains were compared considering percentage of concurrent decisions, opposite decisions and indeterminable decisions. All data were analysed and stored in Microsoft Excel. Gemini was trialled but was unable to perform a RoB assessment in its current form.
In total, n=105 decisions were undertaken by GPT3.5, GPT4 and human reviewers. ChatGPT3.5 was concurrent with human (gold standard) assessment in 41/105 (39.0%) decisions and disagreed on 10/105 (9.5%). ChatGPT4 agreed with human assessment on 34/105 (34.3%) decisions and disagreed on 15/105 (14.3%). Both ChatGPT3.5 and ChatGPT4 delivered an indeterminate response on 54/105 (51.4%) decisions. ChatGPT3.5 outperformed or matched the performance of ChatGPT4 in 6/7 (85.6%) domains (aside from selective reporting), with ChatGPT3.5 performing best in assessing sequence generation and completeness of data, with 8/15 (53.3%) concurrent with human assessment. ChatGPT4 performed best in assessing selective reporting, with 14/15 (93.3%) correct decisions. Results by domain are summarised in table 2 . Notably, GPT4 performed superiorly in one domain (selective reporting), returning a correct decision in 14/15 (93.3%) cases, while GPT3.5 was correct in 10/15 (66.7%) decisions.
Summary of risk-of-bias assessment outcome per domain assessed by ChatGPT3.5 and ChatGPT4 compared with human assessment
When assessing Karanikolas (2011) and Mann (1983), ChatGPT3.5 returned an assessment as ‘moderate’, and ‘low to moderate’, in the overall domain. Both were recorded as unclear as substitute. On one occasion, ChatGPT identified an author as ‘P. C. Weaver’, who is not an author on any included papers. When discussing Lastoria (2006), ChatGPT responded initially in Portuguese.
We explored the potential for ChatGPT as a tool for assessing RoB. Overall, ChatGPT demonstrated moderate agreement, and minor disagreement with gold standard (human) assessment. While encouraging, this suboptimal performance precludes us from recommending ChatGPT be used in real-world RoB assessment.
To emulate end-users, prompts were not standardised, and some questions were repeated to ensure accuracy. When responses were ‘moderate’, ChatGPT was prompted to reassess. Similarly, ChatGPT often declined to perform assessments, which required further prompting with alternate question structure or wording. When prompted to assess allocation concealment for Choksy (2006), ChatGPT summarised the process as follows: ‘randomisation was performed using a random number table and randomisation details were placed in sealed envelopes which were opened in the operating theatre’. Human assessment ranked this as low RoB, whereas ChatGPT ranked this as unclear stating ‘it [was] unclear whether these envelopes were opaque’, demonstrating a literal interpretation of the literature not seen in human assessment.
In this analysis, ChatGPT4 did not offer improvement on ChatGPT3.5 in any domain, aside from selective reporting. For selective reporting, ChatGPT4 returned a correct decision in 14/15 (93.3%) cases, and ChatGPT3.5 in 10/15 (66.7%). However, human assessment returned a decision of unclear on n=14/15 of these assessments. ChatGPT4’s inability to give a definitive assessment is perhaps best outlined by ChatGPT4’s response: ‘To make a definitive assessment, one would ideally compare the outcomes reported in the paper with those specified in the study’s protocol registration’. This could represent an improvement through appreciation of the wider context of a given paper.
Our findings have limitations: the sample size of included papers in this study was small and fairly homogenous. Additionally, we chose not to use standardised prompts. Prompt variability has been demonstrated to introduce output variability. 5 Future research could benefit from a larger and more diverse dataset, with standardised prompts to assess and improve consistency of responses.
Supplemental material
Ethics statements, patient consent for publication.
Not applicable.
Ethics approval
- McKenzie JE ,
- Bossuyt PM , et al
- Higgins JPT ,
- Altman DG ,
- Gøtzsche PC , et al
- Arshad HB ,
- Khan SU , et al
- Talukdar JR
Supplementary materials
Supplementary data.
This web only file has been produced by the BMJ Publishing Group from an electronic file supplied by the author(s) and has not been edited for content.
- Data supplement 1
X @scullingmonkey
Contributors Conception of study: JB and KDB. Data collection: JB, JC, KDB, SH, HALL. Data analysis: JB, JC and KDB. Data interpretation: JB, KDB and LR. Drafting of paper: JB, JC and KDB. Approval of final version: all authors. ChatGPT is the subject of this study and was only used as described in methods and results. ChatGPT was not used in analysing, drafting or rewriting of the paper.
Funding The authors have not declared a specific grant for this research from any funding agency in the public, commercial or not-for-profit sectors.
Competing interests None declared.
Provenance and peer review Not commissioned; externally peer reviewed.
Supplemental material This content has been supplied by the author(s). It has not been vetted by BMJ Publishing Group Limited (BMJ) and may not have been peer-reviewed. Any opinions or recommendations discussed are solely those of the author(s) and are not endorsed by BMJ. BMJ disclaims all liability and responsibility arising from any reliance placed on the content. Where the content includes any translated material, BMJ does not warrant the accuracy and reliability of the translations (including but not limited to local regulations, clinical guidelines, terminology, drug names and drug dosages), and is not responsible for any error and/or omissions arising from translation and adaptation or otherwise.
Read the full text or download the PDF:
Submitting a Bias Report
The University considers bias incidents antithetical to the Penn State Values . The online bias incident report form provides the Penn State community a mechanism for reporting incidents involving acts of bias due to:
- actual or perceived age
- race or color
- ancestry or national origin
- sexual orientation
- gender identity
- physical or mental disability
- religion or creed
- veteran status or service in the uniformed services (as defined in state and federal law)
- marital or family status,
- pregnancy or pregnancy-related conditions
- genetic information
- political ideas
Considerations
Students may use the form to report any act of bias that they have experienced themselves or observed directed against anyone else.
Faculty and staff should only use the form to report acts they observe that are directed against students. If no student is involved, faculty and staff should contact the Office of Equal Opportunity and Access at 814-863-0471 .
You may submit this report anonymously. However, please be advised that, without your contact information, we are unable to follow up with you. Additionally, anonymous submissions may limit our ability to address the reported incident.
Report a Bias Incident
What to expect after submitting a bias incident report
- Immediately upon submission, you will receive an auto-confirmation from the secure online system notifying you that your report was received.
- Within 1-2 working days, you will receive an email from the Bias Response Coordinator to let you know when the Bias Response Network will review your report and to provide any needed resources.
- The Bias Response Network will review your report and determine the appropriate referral.
- The Bias Response Coordinator will refer your report to the appropriate party.
- The Bias Response Coordinator will email you to let you know to whom your report was referred.
- If you requested a meeting to discuss the reported incident, the person to whom your report was referred will contact you to ensure that your perspective is heard, gather any additional details you may wish to provide, and provide additional resources if appropriate.
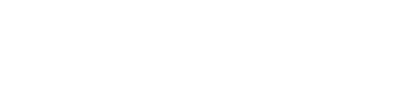
Along with Stanford news and stories, show me:
- Student information
- Faculty/Staff information
We want to provide announcements, events, leadership messages and resources that are relevant to you. Your selection is stored in a browser cookie which you can remove at any time using “Clear all personalization” below.
We all know that unpleasant feeling when we’re talking about something interesting and halfway through our sentence we’re interup – “Wait, what’s for dinner?” – pted.
Stanford scholar Katherine Hilton surveyed 5,000 American English speakers to better understand what affects people’s perceptions of conversational interruptions (Image credit: L.A. Cicero)
But was that really an interruption? Whether or not one person interrupted another depends on whom you ask, according to new research from Stanford’s Katherine Hilton, a doctoral candidate in linguistics.
“What people perceive as an interruption varies systematically across different speakers and speech acts,” said Hilton, who is also a Geballe Dissertation Prize Fellow at the Stanford Humanities Center . “Listeners’ own conversational styles influence whether they interpret simultaneous, overlapping talk as interruptive or cooperative. We all have different opinions about how a good conversation is supposed to go.”
Using a set of carefully controlled scripted audio clips, Hilton surveyed 5,000 American English speakers to better understand what affects people’s perceptions of interruptions. She had participants listen to audio clips and then answer questions about whether the speakers seemed to be friendly and engaged, listening to one another, or trying to interrupt.
Hilton found that American English speakers have different conversational styles. She identified two distinct groups: high and low intensity speakers. High intensity speakers are generally uncomfortable with moments of silence in conversation and consider talking at the same time a sign of engagement. Low intensity speakers find simultaneous chatter to be rude and prefer people speak one at a time in conversation.
Hilton also found a gender disparity among survey participants. Male listeners were more likely to view women who interrupted another speaker in the audio clips as ruder, less friendly and less intelligent than men who interrupted.
Conversational styles and gender bias
Previous research on interruptions has primarily focused on analyzing recordings of natural conversations. But their results could not be generalizable because every study defines an interruption differently, said Penny Eckert , a Stanford professor of linguistics.
“Katherine’s experiment really pulls apart the whole notion of interruption,” Eckert said.
Go to the web site to view the video.
Stanford doctoral candidate Katherine Hilton examined how people perceive interruptions in conversation. She found that those perceptions are affected by the listener’s own conversational style as well as gender.
For example, when a woman asserts herself in a conversation with a man, is that speaking up or interrupting? While other studies have shown that women tend to be seen more negatively than men if they speak up or interrupt, no one has measured those perceptions quantitatively before, Eckert said.
“Gendered ways of talking and interpreting matter, and they have many consequences, including political ones,” said Rob Podesva , an assistant professor of linguistics and Hilton’s adviser. “Katherine’s research shows that there are systematic gender disparities in how we interpret interruptions. Being aware of these disparities may be the first step in figuring out how to address them in the future.”
The differences in conversational styles became evident when participants listened to audio clips in which two people spoke at the same time but were agreeing with each other and stayed on topic, Hilton said.
The high intensity group reported that conversations where people spoke simultaneously when expressing agreement were not interruptive but engaged and friendlier than the conversations with pauses in between speaking turns. In contrast, the low intensity group perceived any amount of simultaneous chitchat as a rude interruption, regardless of what the speakers were saying.
“However, these two categories represent extremes on a spectrum,” Hilton said. “In other words, most people are likely to be somewhere in between the two conversational styles.”
Hilton also found a significant gender bias among study participants when they listened to audio clips considered highly interruptive by almost all participants. In those clips, interrupters did not just talk at the same time as another speaker, but they also changed the subject or raised their voices.
Male listeners were more likely to view a female speaker who interrupted as ruder, less friendly and less intelligent than if the interrupter were male, although both male and female speakers were performing identical scripts in the audio clips, Hilton said.
However, female listeners did not show a significant bias in favor of female or male speakers.
“Finding this gender bias wasn’t as surprising as the extent of it and the fact that it altered perceptions of a female speaker’s intelligence, which we don’t think of as related to interruptions,” Hilton said.
Understanding human interaction
Hilton was curious about how conversations work well before she started studying linguistics. She described her family as incredibly talkative, so growing up, she got used to a high-intensity conversational style.
“During my family gatherings everyone would be talking all at once, and it could get pretty chaotic,” Hilton said. “I had to figure out how to navigate that, and I was always interested in who gets to speak when. Who gets to tell their stories and opinions? And who doesn’t? It fascinates me how people can manage all of this complexity without any explicit rules for conversation.”
Hilton said she is excited to contribute research that helps shed light on the cognitive, social and cultural aspects of human interaction.
“People care about being interrupted, and those small interruptions can have a massive effect on overall communication down the line,” Hilton said. “Breaking apart what an interruption means is essential if we want to understand how humans interact with each other.”
Media Contacts
Alex Shashkevich, Stanford News Service: (650) 497-4419, [email protected]

An official website of the United States government
The .gov means it’s official. Federal government websites often end in .gov or .mil. Before sharing sensitive information, make sure you’re on a federal government site.
The site is secure. The https:// ensures that you are connecting to the official website and that any information you provide is encrypted and transmitted securely.
- Publications
- Account settings
Preview improvements coming to the PMC website in October 2024. Learn More or Try it out now .
- Advanced Search
- Journal List
- HHS Author Manuscripts

Identifying and Avoiding Bias in Research
This narrative review provides an overview on the topic of bias as part of Plastic and Reconstructive Surgery 's series of articles on evidence-based medicine. Bias can occur in the planning, data collection, analysis, and publication phases of research. Understanding research bias allows readers to critically and independently review the scientific literature and avoid treatments which are suboptimal or potentially harmful. A thorough understanding of bias and how it affects study results is essential for the practice of evidence-based medicine.
The British Medical Journal recently called evidence-based medicine (EBM) one of the fifteen most important milestones since the journal's inception 1 . The concept of EBM was created in the early 1980's as clinical practice became more data-driven and literature based 1 , 2 . EBM is now an essential part of medical school curriculum 3 . For plastic surgeons, the ability to practice EBM is limited. Too frequently, published research in plastic surgery demonstrates poor methodologic quality, although a gradual trend toward higher level study designs has been noted over the past ten years 4 , 5 . In order for EBM to be an effective tool, plastic surgeons must critically interpret study results and must also evaluate the rigor of study design and identify study biases. As the leadership of Plastic and Reconstructive Surgery seeks to provide higher quality science to enhance patient safety and outcomes, a discussion of the topic of bias is essential for the journal's readers. In this paper, we will define bias and identify potential sources of bias which occur during study design, study implementation, and during data analysis and publication. We will also make recommendations on avoiding bias before, during, and after a clinical trial.
I. Definition and scope of bias
Bias is defined as any tendency which prevents unprejudiced consideration of a question 6 . In research, bias occurs when “systematic error [is] introduced into sampling or testing by selecting or encouraging one outcome or answer over others” 7 . Bias can occur at any phase of research, including study design or data collection, as well as in the process of data analysis and publication ( Figure 1 ). Bias is not a dichotomous variable. Interpretation of bias cannot be limited to a simple inquisition: is bias present or not? Instead, reviewers of the literature must consider the degree to which bias was prevented by proper study design and implementation. As some degree of bias is nearly always present in a published study, readers must also consider how bias might influence a study's conclusions 8 . Table 1 provides a summary of different types of bias, when they occur, and how they might be avoided.

Major Sources of Bias in Clinical Research
Tips to avoid different types of bias during a trial.
Chance and confounding can be quantified and/or eliminated through proper study design and data analysis. However, only the most rigorously conducted trials can completely exclude bias as an alternate explanation for an association. Unlike random error, which results from sampling variability and which decreases as sample size increases, bias is independent of both sample size and statistical significance. Bias can cause estimates of association to be either larger or smaller than the true association. In extreme cases, bias can cause a perceived association which is directly opposite of the true association. For example, prior to 1998, multiple observational studies demonstrated that hormone replacement therapy (HRT) decreased risk of heart disease among post-menopausal women 8 , 9 . However, more recent studies, rigorously designed to minimize bias, have found the opposite effect (i.e., an increased risk of heart disease with HRT) 10 , 11 .
II. Pre-trial bias
Sources of pre-trial bias include errors in study design and in patient recruitment. These errors can cause fatal flaws in the data which cannot be compensated during data analysis. In this section, we will discuss the importance of clearly defining both risk and outcome, the necessity of standardized protocols for data collection, and the concepts of selection and channeling bias.
Bias during study design
The definition of risk and outcome should be clearly defined prior to study implementation. Subjective measures, such as the Baker grade of capsular contracture, can have high inter-rater variability and the arbitrary cutoffs may make distinguishing between groups difficult 12 . This can inflate the observed variance seen with statistical analysis, making a statistically significant result less likely. Objective, validated risk stratification models such as those published by Caprini 13 and Davison 14 for venous thromboembolism, or standardized outcomes measures such as the Breast-Q 15 should have lower inter-rater variability and are more appropriate for use. When risk or exposure is retrospectively identified via medical chart review, it is prudent to crossreference data sources for confirmation. For example, a chart reviewer should confirm a patient-reported history of sacral pressure ulcer closure with physical exam findings and by review of an operative report; this will decrease discrepancies when compared to using a single data source.
Data collection methods may include questionnaires, structured interviews, physical exam, laboratory or imaging data, or medical chart review. Standardized protocols for data collection, including training of study personnel, can minimize inter-observer variability when multiple individuals are gathering and entering data. Blinding of study personnel to the patient's exposure and outcome status, or if not possible, having different examiners measure the outcome than those who evaluated the exposure, can also decrease bias. Due to the presence of scars, patients and those directly examining them cannot be blinded to whether or not an operation was received. For comparisons of functional or aesthetic outcomes in surgical procedures, an independent examiner can be blinded to the type of surgery performed. For example, a hand surgery study comparing lag screw versus plate and screw fixation of metacarpal fractures could standardize the surgical approach (and thus the surgical scar) and have functional outcomes assessed by a blinded examiner who had not viewed the operative notes or x-rays. Blinded examiners can also review imaging and confirm diagnoses without examining patients 16 , 17 .
Selection bias
Selection bias may occur during identification of the study population. The ideal study population is clearly defined, accessible, reliable, and at increased risk to develop the outcome of interest. When a study population is identified, selection bias occurs when the criteria used to recruit and enroll patients into separate study cohorts are inherently different. This can be a particular problem with case-control and retrospective cohort studies where exposure and outcome have already occurred at the time individuals are selected for study inclusion 18 . Prospective studies (particularly randomized, controlled trials) where the outcome is unknown at time of enrollment are less prone to selection bias.
Channeling bias
Channeling bias occurs when patient prognostic factors or degree of illness dictates the study cohort into which patients are placed. This bias is more likely in non-randomized trials when patient assignment to groups is performed by medical personnel. Channeling bias is commonly seen in pharmaceutical trials comparing old and new drugs to one another 19 . In surgical studies, channeling bias can occur if one intervention carries a greater inherent risk 20 . For example, hand surgeons managing fractures may be more aggressive with operative intervention in young, healthy individuals with low perioperative risk. Similarly, surgeons might tolerate imperfect reduction in the elderly, a group at higher risk for perioperative complications and with decreased need for perfect hand function. Thus, a selection bias exists for operative intervention in young patients. Now imagine a retrospective study of operative versus non-operative management of hand fractures. In this study, young patients would be channeled into the operative study cohort and the elderly would be channeled into the nonoperative study cohort.
III. Bias during the clinical trial
Information bias is a blanket classification of error in which bias occurs in the measurement of an exposure or outcome. Thus, the information obtained and recorded from patients in different study groups is unequal in some way 18 . Many subtypes of information bias can occur, including interviewer bias, chronology bias, recall bias, patient loss to follow-up, bias from misclassification of patients, and performance bias.
Interviewer bias
Interviewer bias refers to a systematic difference between how information is solicited, recorded, or interpreted 18 , 21 . Interviewer bias is more likely when disease status is known to interviewer. An example of this would be a patient with Buerger's disease enrolled in a case control study which attempts to retrospectively identify risk factors. If the interviewer is aware that the patient has Buerger's disease, he/she may probe for risk factors, such as smoking, more extensively (“Are you sure you've never smoked? Never? Not even once?”) than in control patients. Interviewer bias can be minimized or eliminated if the interviewer is blinded to the outcome of interest or if the outcome of interest has not yet occurred, as in a prospective trial.
Chronology bias
Chronology bias occurs when historic controls are used as a comparison group for patients undergoing an intervention. Secular trends within the medical system could affect how disease is diagnosed, how treatments are administered, or how preferred outcome measures are obtained 20 . Each of these differences could act as a source of inequality between the historic controls and intervention groups. For example, many microsurgeons currently use preoperative imaging to guide perforator flap dissection. Imaging has been shown to significantly reduce operative time 40 . A retrospective study of flap dissection time might conclude that dissection time decreases as surgeon experience improves. More likely, the use of preoperative imaging caused a notable reduction in dissection time. Thus, chronology bias is present. Chronology bias can be minimized by conducting prospective cohort or randomized control trials, or by using historic controls from only the very recent past.
Recall bias
Recall bias refers to the phenomenon in which the outcomes of treatment (good or bad) may color subjects' recollections of events prior to or during the treatment process. One common example is the perceived association between autism and the MMR vaccine. This vaccine is given to children during a prominent period of language and social development. As a result, parents of children with autism are more likely to recall immunization administration during this developmental regression, and a causal relationship may be perceived 22 . Recall bias is most likely when exposure and disease status are both known at time of study, and can also be problematic when patient interviews (or subjective assessments) are used as a primary data sources. When patient-report data are used, some investigators recommend that the trial design masks the intent of questions in structured interviews or surveys and/or uses only validated scales for data acquisition 23 .
Transfer bias
In almost all clinical studies, subjects are lost to follow-up. In these instances, investigators must consider whether these patients are fundamentally different than those retained in the study. Researchers must also consider how to treat patients lost to follow-up in their analysis. Well designed trials usually have protocols in place to attempt telephone or mail contact for patients who miss clinic appointments. Transfer bias can occur when study cohorts have unequal losses to follow-up. This is particularly relevant in surgical trials when study cohorts are expected to require different follow-up regimens. Consider a study evaluating outcomes in inferior pedicle Wise pattern versus vertical scar breast reductions. Because the Wise pattern patients often have fewer contour problems in the immediate postoperative period, they may be less likely to return for long-term follow-up. By contrast, patient concerns over resolving skin redundancies in the vertical reduction group may make these individuals more likely to return for postoperative evaluations by their surgeons. Some authors suggest that patient loss to follow-up can be minimized by offering convenient office hours, personalized patient contact via phone or email, and physician visits to the patient's home 20 , 24 .
Bias from misclassification of exposure or outcome
Misclassification of exposure can occur if the exposure itself is poorly defined or if proxies of exposure are utilized. For example, this might occur in a study evaluating efficacy of becaplermin (Regranex, Systagenix Wound Management) versus saline dressings for management of diabetic foot ulcers. Significantly different results might be obtained if the becaplermin cohort of patients included those prescribed the medication, rather than patients directly observed to be applying the medication. Similarly, misclassification of outcome can occur if non-objective measures are used. For example, clinical signs and symptoms are notoriously unreliable indicators of venous thromboembolism. Patients are accurately diagnosed by physical exam less than 50% of the time 25 . Thus, using Homan's sign (calf pain elicited by extreme dorsi-flexion) or pleuritic chest pain as study measures for deep venous thrombosis or pulmonary embolus would be inappropriate. Venous thromboembolism is appropriately diagnosed using objective tests with high sensitivity and specificity, such as duplex ultrasound or spiral CT scan 26 - 28 .
Performance bias
In surgical trials, performance bias may complicate efforts to establish a cause-effect relationship between procedures and outcomes. As plastic surgeons, we are all aware that surgery is rarely standardized and that technical variability occurs between surgeons and among a single surgeon's cases. Variations by surgeon commonly occur in surgical plan, flow of operation, and technical maneuvers used to achieve the desired result. The surgeon's experience may have a significant effect on the outcome. To minimize or avoid performance bias, investigators can consider cluster stratification of patients, in which all patients having an operation by one surgeon or at one hospital are placed into the same study group, as opposed to placing individual patients into groups. This will minimize performance variability within groups and decrease performance bias. Cluster stratification of patients may allow surgeons to perform only the surgery with which they are most comfortable or experienced, providing a more valid assessment of the procedures being evaluated. If the operation in question has a steep learning curve, cluster stratification may make generalization of study results to the everyday plastic surgeon difficult.
IV. Bias after a trial
Bias after a trial's conclusion can occur during data analysis or publication. In this section, we will discuss citation bias, evaluate the role of confounding in data analysis, and provide a brief discussion of internal and external validity.
Citation bias
Citation bias refers to the fact that researchers and trial sponsors may be unwilling to publish unfavorable results, believing that such findings may negatively reflect on their personal abilities or on the efficacy of their product. Thus, positive results are more likely to be submitted for publication than negative results. Additionally, existing inequalities in the medical literature may sway clinicians' opinions of the expected trial results before or during a trial. In recognition of citation bias, the International Committee of Medical Journal Editors(ICMJE) released a consensus statement in 2004 29 which required all randomized control trials to be pre-registered with an approved clinical trials registry. In 2007, a second consensus statement 30 required that all prospective trials not deemed purely observational be registered with a central clinical trials registry prior to patient enrollment. ICMJE member journals will not publish studies which are not registered in advance with one of five accepted registries. Despite these measures, citation bias has not been completely eliminated. While centralized documentation provides medical researchers with information about unpublished trials, investigators may be left to only speculate as to the results of these studies.
Confounding
Confounding occurs when an observed association is due to three factors: the exposure, the outcome of interest, and a third factor which is independently associated with both the outcome of interest and the exposure 18 . Examples of confounders include observed associations between coffee drinking and heart attack (confounded by smoking) and the association between income and health status (confounded by access to care). Pre-trial study design is the preferred method to control for confounding. Prior to the study, matching patients for demographics (such as age or gender) and risk factors (such as body mass index or smoking) can create similar cohorts among identified confounders. However, the effect of unmeasured or unknown confounders may only be controlled by true randomization in a study with a large sample size. After a study's conclusion, identified confounders can be controlled by analyzing for an association between exposure and outcome only in cohorts similar for the identified confounding factor. For example, in a study comparing outcomes for various breast reconstruction options, the results might be confounded by the timing of the reconstruction (i.e., immediate versus delayed procedures). In other words, procedure type and timing may have both have significant and independent effects on breast reconstruction outcomes. One approach to this confounding would be to compare outcomes by procedure type separately for immediate and delayed reconstruction patients. This maneuver is commonly termed a “stratified” analysis. Stratified analyses are limited if multiple confounders are present or if sample size is small. Multi-variable regression analysis can also be used to control for identified confounders during data analysis. The role of unidentified confounders cannot be controlled using statistical analysis.
Internal vs. External Validity
Internal validity refers to the reliability or accuracy of the study results. A study's internal validity reflects the author's and reviewer's confidence that study design, implementation, and data analysis have minimized or eliminated bias and that the findings are representative of the true association between exposure and outcome. When evaluating studies, careful review of study methodology for sources of bias discussed above enables the reader to evaluate internal validity. Studies with high internal validity are often explanatory trials, those designed to test efficacy of a specific intervention under idealized conditions in a highly selected population. However, high internal validity often comes at the expense of ability to be generalized. For example, although supra-microsurgery techniques, defined as anastamosis of vessels less than 0.5mm-0.8mm in diameter, have been shown to be technically possible in high volume microsurgery centers 31 - 33 (high internal validity), it is unlikely that the majority of plastic surgeons could perform this operation with an acceptable rate of flap loss.
External validity of research design deals with the degree to which findings are able to be generalized to other groups or populations. In contrast with explanatory trials, pragmatic trials are designed to assess the benefits of interventions under real clinical conditions. These studies usually include study populations generated using minimal exclusion criteria, making them very similar to the general population. While pragmatic trials have high external validity, loose inclusion criteria may compromise the study's internal validity. When reviewing scientific literature, readers should assess whether the research methods preclude generalization of the study's findings to other patient populations. In making this decision, readers must consider differences between the source population (population from which the study population originated) and the study population (those included in the study). Additionally, it is important to distinguish limited ability to be generalized due to a selective patient population from true bias 8 .
When designing trials, achieving balance between internal and external validity is difficult. An ideal trial design would randomize patients and blind those collecting and analyzing data (high internal validity), while keeping exclusion criteria to a minimum, thus making study and source populations closely related and allowing generalization of results (high external validity) 34 . For those evaluating the literature, objective models exist to quantify both external and internal validity. Conceptual models to assess a study's ability to be generalized have been developed 35 . Additionally, qualitative checklists can be used to assess the external validity of clinical trials. These can be utilized by investigators to improve study design and also by those reading published studies 36 .
The importance of internal validity is reflected in the existing concept of “levels of evidence” 5 , where more rigorously designed trials produce higher levels of evidence. Such high-level studies can be evaluated using the Jadad scoring system, an established, rigorous means of assessing the methodological quality and internal validity of clinical trials 37 . Even so-called “gold-standard” RCT's can be undermined by poor study design. Like all studies, RCT's must be rigorously evaluated. Descriptions of study methods should include details on the randomization process, method(s) of blinding, treatment of incomplete outcome data, funding source(s), and include data on statistically insignificant outcomes 38 . Authors who provide incomplete trial information can create additional bias after a trial ends; readers are not able to evaluate the trial's internal and external validity 20 . The CONSORT statement 39 provides a concise 22-point checklist for authors reporting the results of RCT's. Manuscripts that conform to the CONSORT checklist will provide adequate information for readers to understand the study's methodology. As a result, readers can make independent judgments on the trial's internal and external validity.
Bias can occur in the planning, data collection, analysis, and publication phases of research. Understanding research bias allows readers to critically and independently review the scientific literature and avoid treatments which are suboptimal or potentially harmful. A thorough understanding of bias and how it affects study results is essential for the practice of evidence-based medicine.
Acknowledgments
Dr. Pannucci receives salary support from the NIH T32 grant program (T32 GM-08616).
Meeting disclosure:
This work was has not been previously presented.
None of the authors has a financial interest in any of the products, devices, or drugs mentioned in this manuscript.
This is a PDF file of an unedited manuscript that has been accepted for publication. As a service to our customers we are providing this early version of the manuscript. The manuscript will undergo copyediting, typesetting, and review of the resulting proof before it is published in its final citable form. Please note that during the production process errors may be discovered which could affect the content, and all legal disclaimers that apply to the journal pertain.
- Subscribe Now
Swing State Project: May 23, 2024 Toplines
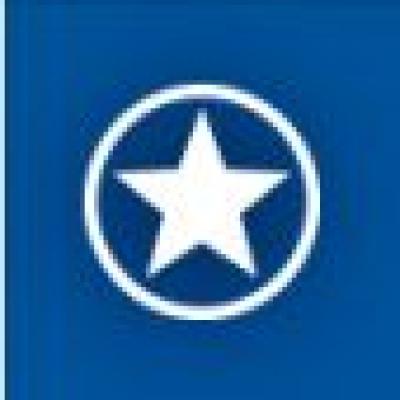
The Swing State Project is a collaboration between The Cook Political Report and two pollsters, BSG and GS Strategy Group. Below are the toplines from the May 2024 poll. In total, 3,969 likely voters across seven swing states (Arizona, Georgia, Michigan, North Carolina, Nevada, Pennsylvania, and Wisconsin) completed the survey.
In the seven states combined, Trump leads Biden in a head-to-head matchup 47% to 44%. In a five-way ballot test that includes Robert F. Kennedy, Jr., Green Party candidate Jill Stein, and independent Cornel West, Trump opened a larger lead—43% to 38% for Biden and 8% for Kennedy, Jr.
View the crosstabs (CPR subscribers only)
Contact us with questions.
More from the Cook Political Report
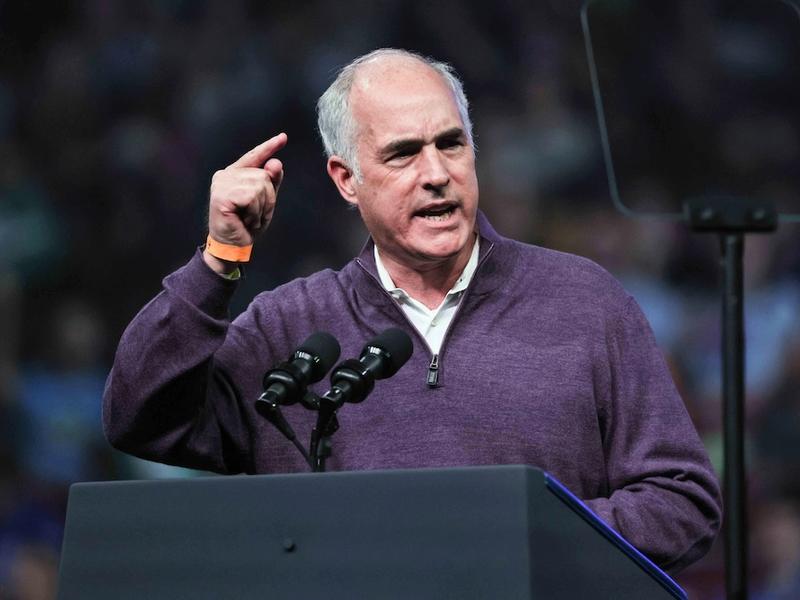
Why Senate Democrats Are Running Strong, Even as Biden Is Struggling

Tale of Two Electorates: Trump Is Excelling With Lower-Engagement Voters, but Will They Show Up?

Can the Domenici Dynasty Put New Mexico Back on the Map?

Tony Gonzales Narrowly Survives Runoff Against Far-Right Opponent

North Carolina Governor Moves From Lean Democrat To Toss Up

Montana House Primary Preview: Former Big Sky Congressman’s Comeback Bid Up in the Air
Subscribe today.
Our subscribers have first access to individual race pages for each House, Senate and Governors race, which will include race ratings (each race is rated on a seven-point scale) and a narrative analysis pertaining to that race.
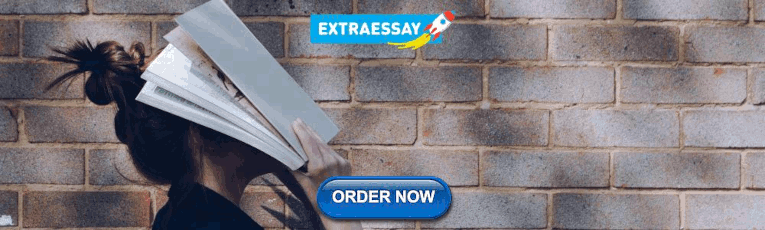
IMAGES
VIDEO
COMMENTS
The problem of selective reporting and research on reporting bias. Selective reporting of research findings presents a large-scale problem in science, substantially affecting the validity of the published body of knowledge ( Bouter et al., 2016; Dwan et al., 2014; van den Bogert et al., 2017).Reporting bias (publication bias or outcome reporting bias) occurs when the decision to report depends ...
Information bias occurs during the data collection step and is common in research studies that involve self-reporting and retrospective data collection. It can also result from poor interviewing techniques or differing levels of recall from participants. The main types of information bias are: Recall bias. Observer bias.
Reporting bias. In epidemiology, reporting bias is defined as "selective revealing or suppression of information" by subjects (for example about past medical history, smoking, sexual experiences). [1] In artificial intelligence research, the term reporting bias is used to refer to people's tendency to under-report all the information available.
Reporting bias is a type of selection bias that occurs when only certain observations are reported or published. Reporting bias can greatly impact the accuracy of results, and it is important to consider reporting bias when conducting research. In this article, we will discuss reporting bias, the types, and the examples. ...
Self-reporting bias. Self-reporting is a common approach for gathering data in epidemiologic and medical research. This method requires participants to respond to the researcher's questions without his/her interference. Examples of self-reporting include questionnaires, surveys, or interviews.
Our definition of reporting biases is a distortion of presented information from research due to the selective disclosure or withholding of information by parties involved with regards to the topic selected for study and the design, conduct, analysis, or dissemination of study methods, findings or both. Researchers have previously described ...
Channeling bias is a type of selection bias noted in observational studies. It occurs most frequently when patient characteristics, such as age or severity of illness, affect cohort assignment. This can occur, for example, in surgical studies where different interventions carry different levels of risk.
Research bias refers to any instance where the researcher, or the research design, negatively influences the quality of a study's results, whether intentionally or not. The three common types of research bias we looked at are: Selection bias - where a skewed sample leads to skewed results. Analysis bias - where the analysis method and/or ...
Clinical trials are experiments in human beings. Findings from these experiments, either by themselves or within research syntheses, are often meant to evidence-based clinical decision-making. These decisions can be misled when clinical trials are reported in a biased manner. For clinical trials to inform healthcare decisions without bias ...
Reporting biases. In: Catalog Of Bias 2019) refers to reporting bias as, "A systematic distortion that arises from the selective disclosure or withholding of information by parties involved in the design, conduct, analysis, or dissemination of a study or research findings". It lists the following types of reporting biases:
The reporting of research findings may depend on the nature and direction of results, which is referred to as "reporting bias" [1, 2].For example, studies in which interventions are shown to be ineffective are sometimes not published, meaning that only a subset of the relevant evidence on a topic may be available [1, 2].Various types of reporting bias exist (Table 1), including publication ...
Reporting bias means that only a selection of results are included in any analysis, which typically covers only a fraction of relevant evidence. This can lead to inappropriate decisions (for example, prescribing ineffective or harmful drugs), resource waste and misguided future research. The umbrella term "reporting bias" covers a wide ...
Outcome reporting bias - where the results of negative clinical trials are cherry-picked or distorted to improve the overall findings; ... Selective reporting bias, FFP, and other examples of research misconduct, all contribute to a culture of mistrust in science and academia. However, journal editors can play a role in helping change this ...
Background The credibility of evidence syntheses can be compromised by reporting biases, which arise when dissemination of research findings is influenced by the nature of the results.1 For example, there may be bias due to selective publication, where a study is only published if the findings are considered interesting (also known as publication bias).2 In addition, bias due to selective non ...
Abstract. The subject of this column is the nature of bias in both quantitative and qualitative research. To that end, bias will be defined and then both the processes by which it enters into research will be entertained along with discussions on how to ameliorate this problem. Clinicians, who are in practice, frequently are called upon to make ...
Reporting of research findings is often selective. This threatens the validity of the published body of knowledge if the decision to report depends on the nature of the results. ... The evidence derived from studies on causes and mechanisms underlying selective reporting may help to avoid or reduce reporting bias. Such research should be guided ...
Recognizing and understanding research bias is crucial for determining the utility of study results and an essential aspect of evidence-based decision-making in the health professions. ... Seeing "bias" as a problem to be managed during the process and reporting of qualitative research may be a way of trying to establish a firmer footing on ...
In conclusion, reporting bias is a widespread phenomenon in the medical literature. Mandatory prospective registration of trials and public access to study data via results databases need to be introduced on a worldwide scale. This will allow for an independent review of research data, help fulfil ethical obligations towards patients, and ...
Bias in research Joanna Smith,1 Helen Noble2 The aim of this article is to outline types of 'bias' across research designs, and consider strategies to minimise bias. Evidence-based nursing, defined as the "process by ... research, studies are more likely to be published if reporting statistically significant
Selective reporting bias occurs when researchers choose to present a subset of results from their study by omitting certain data or complete outcomes. Consequently, the reported estimate or range of outcomes from the study may be biased. How to detect selective reporting bias. Most research generates large amounts of data that can be analyzed ...
Media bias widely exists in the articles published by news media, influencing their readers' perceptions, and bringing prejudice or injustice to society. However, current analysis methods ...
Researcher bias refers to the preconceptions and subjective viewpoints that a researcher may bring to the research process, which can influence how data is collected, interpreted, and presented ...
Risk-of-bias (RoB) assessment is used to assess randomised control trials for systematic errors. Developed by Cochrane, it is considered the gold standard of assessing RoB for studies included within systematic reviews, representing a key part of Preferred Reporting Items for Systematic Reviews and Meta-Analyses guidelines.1 The RoB tool comprises six domains that may signify bias: random ...
Selection bias is the bias introduced by the selection of individuals, groups, or data for analysis in such a way that proper randomization is not achieved, thereby failing to ensure that the sample obtained is representative of the population intended to be analyzed. It is sometimes referred to as the selection effect.The phrase "selection bias" most often refers to the distortion of a ...
Faculty and staff should only use the form to report acts they observe that are directed against students. If no student is involved, faculty and staff should contact the Office of Equal Opportunity and Access at 814-863-0471. You may submit this report anonymously. However, please be advised that, without your contact information, we are ...
Definition of bias. Bias is any trend or deviation from the truth in data collection, data analysis, interpretation and publication which can cause false conclusions. Bias can occur either intentionally or unintentionally ( 1 ). Intention to introduce bias into someone's research is immoral.
"Finding this gender bias wasn't as surprising as the extent of it and the fact that it altered perceptions of a female speaker's intelligence, which we don't think of as related to ...
Accuracy of maternal recall varied greatly in relation to the type of pregnancy event, and recall was inaccurate for many specific events. For the control sample only, maternal recall of the total number of complications corresponded closely to chart information. Contrary to the study hypothesis, mothers of offspring with psychosis report fewer ...
Abstract. This narrative review provides an overview on the topic of bias as part of Plastic and Reconstructive Surgery 's series of articles on evidence-based medicine. Bias can occur in the planning, data collection, analysis, and publication phases of research. Understanding research bias allows readers to critically and independently review ...
The Swing State Project is a collaboration between The Cook Political Report and two pollsters, BSG and GS Strategy Group. Below are the toplines from the May 2024 poll. In total, 3,969 likely voters across seven swing states (Arizona, Georgia, Michigan, North Carolina, Nevada, Pennsylvania, and Wisconsin) completed the survey.