What Is a Testable Hypothesis?
- Scientific Method
- Chemical Laws
- Periodic Table
- Projects & Experiments
- Biochemistry
- Physical Chemistry
- Medical Chemistry
- Chemistry In Everyday Life
- Famous Chemists
- Activities for Kids
- Abbreviations & Acronyms
- Weather & Climate
- Ph.D., Biomedical Sciences, University of Tennessee at Knoxville
- B.A., Physics and Mathematics, Hastings College
A hypothesis is a tentative answer to a scientific question. A testable hypothesis is a hypothesis that can be proved or disproved as a result of testing, data collection, or experience. Only testable hypotheses can be used to conceive and perform an experiment using the scientific method .
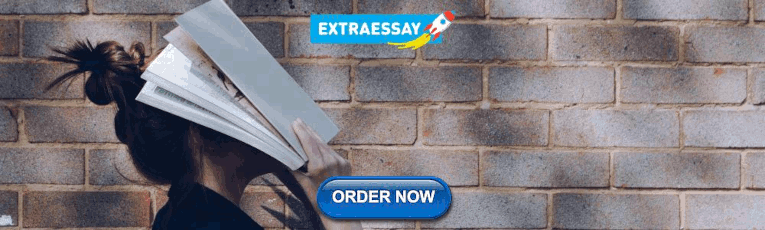
Requirements for a Testable Hypothesis
In order to be considered testable, two criteria must be met:
- It must be possible to prove that the hypothesis is true.
- It must be possible to prove that the hypothesis is false.
- It must be possible to reproduce the results of the hypothesis.
Examples of a Testable Hypothesis
All the following hypotheses are testable. It's important, however, to note that while it's possible to say that the hypothesis is correct, much more research would be required to answer the question " why is this hypothesis correct?"
- Students who attend class have higher grades than students who skip class. This is testable because it is possible to compare the grades of students who do and do not skip class and then analyze the resulting data. Another person could conduct the same research and come up with the same results.
- People exposed to high levels of ultraviolet light have a higher incidence of cancer than the norm. This is testable because it is possible to find a group of people who have been exposed to high levels of ultraviolet light and compare their cancer rates to the average.
- If you put people in a dark room, then they will be unable to tell when an infrared light turns on. This hypothesis is testable because it is possible to put a group of people into a dark room, turn on an infrared light, and ask the people in the room whether or not an infrared light has been turned on.
Examples of a Hypothesis Not Written in a Testable Form
- It doesn't matter whether or not you skip class. This hypothesis can't be tested because it doesn't make any actual claim regarding the outcome of skipping class. "It doesn't matter" doesn't have any specific meaning, so it can't be tested.
- Ultraviolet light could cause cancer. The word "could" makes a hypothesis extremely difficult to test because it is very vague. There "could," for example, be UFOs watching us at every moment, even though it's impossible to prove that they are there!
- Goldfish make better pets than guinea pigs. This is not a hypothesis; it's a matter of opinion. There is no agreed-upon definition of what a "better" pet is, so while it is possible to argue the point, there is no way to prove it.
How to Propose a Testable Hypothesis
Now that you know what a testable hypothesis is, here are tips for proposing one.
- Try to write the hypothesis as an if-then statement. If you take an action, then a certain outcome is expected.
- Identify the independent and dependent variable in the hypothesis. The independent variable is what you are controlling or changing. You measure the effect this has on the dependent variable.
- Write the hypothesis in such a way that you can prove or disprove it. For example, a person has skin cancer, you can't prove they got it from being out in the sun. However, you can demonstrate a relationship between exposure to ultraviolet light and increased risk of skin cancer.
- Make sure you are proposing a hypothesis you can test with reproducible results. If your face breaks out, you can't prove the breakout was caused by the french fries you had for dinner last night. However, you can measure whether or not eating french fries is associated with breaking out. It's a matter of gathering enough data to be able to reproduce results and draw a conclusion.
- Null Hypothesis Examples
- Examples of Independent and Dependent Variables
- What Is the Visible Light Spectrum?
- What Glows Under Black Light?
- Difference Between Independent and Dependent Variables
- What Are Examples of a Hypothesis?
- What Is a Black Light?
- What Is a Hypothesis? (Science)
- What Are the Elements of a Good Hypothesis?
- How To Design a Science Fair Experiment
- Understanding Simple vs Controlled Experiments
- Scientific Method Vocabulary Terms
- Hypothesis, Model, Theory, and Law
- Theory Definition in Science
- Null Hypothesis Definition and Examples
- What 'Fail to Reject' Means in a Hypothesis Test
- Bipolar Disorder
- Therapy Center
- When To See a Therapist
- Types of Therapy
- Best Online Therapy
- Best Couples Therapy
- Best Family Therapy
- Managing Stress
- Sleep and Dreaming
- Understanding Emotions
- Self-Improvement
- Healthy Relationships
- Student Resources
- Personality Types
- Guided Meditations
- Verywell Mind Insights
- 2024 Verywell Mind 25
- Mental Health in the Classroom
- Editorial Process
- Meet Our Review Board
- Crisis Support
How to Write a Great Hypothesis
Hypothesis Definition, Format, Examples, and Tips
Kendra Cherry, MS, is a psychosocial rehabilitation specialist, psychology educator, and author of the "Everything Psychology Book."
:max_bytes(150000):strip_icc():format(webp)/IMG_9791-89504ab694d54b66bbd72cb84ffb860e.jpg)
Amy Morin, LCSW, is a psychotherapist and international bestselling author. Her books, including "13 Things Mentally Strong People Don't Do," have been translated into more than 40 languages. Her TEDx talk, "The Secret of Becoming Mentally Strong," is one of the most viewed talks of all time.
:max_bytes(150000):strip_icc():format(webp)/VW-MIND-Amy-2b338105f1ee493f94d7e333e410fa76.jpg)
Verywell / Alex Dos Diaz
- The Scientific Method
Hypothesis Format
Falsifiability of a hypothesis.
- Operationalization
Hypothesis Types
Hypotheses examples.
- Collecting Data
A hypothesis is a tentative statement about the relationship between two or more variables. It is a specific, testable prediction about what you expect to happen in a study. It is a preliminary answer to your question that helps guide the research process.
Consider a study designed to examine the relationship between sleep deprivation and test performance. The hypothesis might be: "This study is designed to assess the hypothesis that sleep-deprived people will perform worse on a test than individuals who are not sleep-deprived."
At a Glance
A hypothesis is crucial to scientific research because it offers a clear direction for what the researchers are looking to find. This allows them to design experiments to test their predictions and add to our scientific knowledge about the world. This article explores how a hypothesis is used in psychology research, how to write a good hypothesis, and the different types of hypotheses you might use.
The Hypothesis in the Scientific Method
In the scientific method , whether it involves research in psychology, biology, or some other area, a hypothesis represents what the researchers think will happen in an experiment. The scientific method involves the following steps:
- Forming a question
- Performing background research
- Creating a hypothesis
- Designing an experiment
- Collecting data
- Analyzing the results
- Drawing conclusions
- Communicating the results
The hypothesis is a prediction, but it involves more than a guess. Most of the time, the hypothesis begins with a question which is then explored through background research. At this point, researchers then begin to develop a testable hypothesis.
Unless you are creating an exploratory study, your hypothesis should always explain what you expect to happen.
In a study exploring the effects of a particular drug, the hypothesis might be that researchers expect the drug to have some type of effect on the symptoms of a specific illness. In psychology, the hypothesis might focus on how a certain aspect of the environment might influence a particular behavior.
Remember, a hypothesis does not have to be correct. While the hypothesis predicts what the researchers expect to see, the goal of the research is to determine whether this guess is right or wrong. When conducting an experiment, researchers might explore numerous factors to determine which ones might contribute to the ultimate outcome.
In many cases, researchers may find that the results of an experiment do not support the original hypothesis. When writing up these results, the researchers might suggest other options that should be explored in future studies.
In many cases, researchers might draw a hypothesis from a specific theory or build on previous research. For example, prior research has shown that stress can impact the immune system. So a researcher might hypothesize: "People with high-stress levels will be more likely to contract a common cold after being exposed to the virus than people who have low-stress levels."
In other instances, researchers might look at commonly held beliefs or folk wisdom. "Birds of a feather flock together" is one example of folk adage that a psychologist might try to investigate. The researcher might pose a specific hypothesis that "People tend to select romantic partners who are similar to them in interests and educational level."
Elements of a Good Hypothesis
So how do you write a good hypothesis? When trying to come up with a hypothesis for your research or experiments, ask yourself the following questions:
- Is your hypothesis based on your research on a topic?
- Can your hypothesis be tested?
- Does your hypothesis include independent and dependent variables?
Before you come up with a specific hypothesis, spend some time doing background research. Once you have completed a literature review, start thinking about potential questions you still have. Pay attention to the discussion section in the journal articles you read . Many authors will suggest questions that still need to be explored.
How to Formulate a Good Hypothesis
To form a hypothesis, you should take these steps:
- Collect as many observations about a topic or problem as you can.
- Evaluate these observations and look for possible causes of the problem.
- Create a list of possible explanations that you might want to explore.
- After you have developed some possible hypotheses, think of ways that you could confirm or disprove each hypothesis through experimentation. This is known as falsifiability.
In the scientific method , falsifiability is an important part of any valid hypothesis. In order to test a claim scientifically, it must be possible that the claim could be proven false.
Students sometimes confuse the idea of falsifiability with the idea that it means that something is false, which is not the case. What falsifiability means is that if something was false, then it is possible to demonstrate that it is false.
One of the hallmarks of pseudoscience is that it makes claims that cannot be refuted or proven false.
The Importance of Operational Definitions
A variable is a factor or element that can be changed and manipulated in ways that are observable and measurable. However, the researcher must also define how the variable will be manipulated and measured in the study.
Operational definitions are specific definitions for all relevant factors in a study. This process helps make vague or ambiguous concepts detailed and measurable.
For example, a researcher might operationally define the variable " test anxiety " as the results of a self-report measure of anxiety experienced during an exam. A "study habits" variable might be defined by the amount of studying that actually occurs as measured by time.
These precise descriptions are important because many things can be measured in various ways. Clearly defining these variables and how they are measured helps ensure that other researchers can replicate your results.
Replicability
One of the basic principles of any type of scientific research is that the results must be replicable.
Replication means repeating an experiment in the same way to produce the same results. By clearly detailing the specifics of how the variables were measured and manipulated, other researchers can better understand the results and repeat the study if needed.
Some variables are more difficult than others to define. For example, how would you operationally define a variable such as aggression ? For obvious ethical reasons, researchers cannot create a situation in which a person behaves aggressively toward others.
To measure this variable, the researcher must devise a measurement that assesses aggressive behavior without harming others. The researcher might utilize a simulated task to measure aggressiveness in this situation.
Hypothesis Checklist
- Does your hypothesis focus on something that you can actually test?
- Does your hypothesis include both an independent and dependent variable?
- Can you manipulate the variables?
- Can your hypothesis be tested without violating ethical standards?
The hypothesis you use will depend on what you are investigating and hoping to find. Some of the main types of hypotheses that you might use include:
- Simple hypothesis : This type of hypothesis suggests there is a relationship between one independent variable and one dependent variable.
- Complex hypothesis : This type suggests a relationship between three or more variables, such as two independent and dependent variables.
- Null hypothesis : This hypothesis suggests no relationship exists between two or more variables.
- Alternative hypothesis : This hypothesis states the opposite of the null hypothesis.
- Statistical hypothesis : This hypothesis uses statistical analysis to evaluate a representative population sample and then generalizes the findings to the larger group.
- Logical hypothesis : This hypothesis assumes a relationship between variables without collecting data or evidence.
A hypothesis often follows a basic format of "If {this happens} then {this will happen}." One way to structure your hypothesis is to describe what will happen to the dependent variable if you change the independent variable .
The basic format might be: "If {these changes are made to a certain independent variable}, then we will observe {a change in a specific dependent variable}."
A few examples of simple hypotheses:
- "Students who eat breakfast will perform better on a math exam than students who do not eat breakfast."
- "Students who experience test anxiety before an English exam will get lower scores than students who do not experience test anxiety."
- "Motorists who talk on the phone while driving will be more likely to make errors on a driving course than those who do not talk on the phone."
- "Children who receive a new reading intervention will have higher reading scores than students who do not receive the intervention."
Examples of a complex hypothesis include:
- "People with high-sugar diets and sedentary activity levels are more likely to develop depression."
- "Younger people who are regularly exposed to green, outdoor areas have better subjective well-being than older adults who have limited exposure to green spaces."
Examples of a null hypothesis include:
- "There is no difference in anxiety levels between people who take St. John's wort supplements and those who do not."
- "There is no difference in scores on a memory recall task between children and adults."
- "There is no difference in aggression levels between children who play first-person shooter games and those who do not."
Examples of an alternative hypothesis:
- "People who take St. John's wort supplements will have less anxiety than those who do not."
- "Adults will perform better on a memory task than children."
- "Children who play first-person shooter games will show higher levels of aggression than children who do not."
Collecting Data on Your Hypothesis
Once a researcher has formed a testable hypothesis, the next step is to select a research design and start collecting data. The research method depends largely on exactly what they are studying. There are two basic types of research methods: descriptive research and experimental research.
Descriptive Research Methods
Descriptive research such as case studies , naturalistic observations , and surveys are often used when conducting an experiment is difficult or impossible. These methods are best used to describe different aspects of a behavior or psychological phenomenon.
Once a researcher has collected data using descriptive methods, a correlational study can examine how the variables are related. This research method might be used to investigate a hypothesis that is difficult to test experimentally.
Experimental Research Methods
Experimental methods are used to demonstrate causal relationships between variables. In an experiment, the researcher systematically manipulates a variable of interest (known as the independent variable) and measures the effect on another variable (known as the dependent variable).
Unlike correlational studies, which can only be used to determine if there is a relationship between two variables, experimental methods can be used to determine the actual nature of the relationship—whether changes in one variable actually cause another to change.
The hypothesis is a critical part of any scientific exploration. It represents what researchers expect to find in a study or experiment. In situations where the hypothesis is unsupported by the research, the research still has value. Such research helps us better understand how different aspects of the natural world relate to one another. It also helps us develop new hypotheses that can then be tested in the future.
Thompson WH, Skau S. On the scope of scientific hypotheses . R Soc Open Sci . 2023;10(8):230607. doi:10.1098/rsos.230607
Taran S, Adhikari NKJ, Fan E. Falsifiability in medicine: what clinicians can learn from Karl Popper [published correction appears in Intensive Care Med. 2021 Jun 17;:]. Intensive Care Med . 2021;47(9):1054-1056. doi:10.1007/s00134-021-06432-z
Eyler AA. Research Methods for Public Health . 1st ed. Springer Publishing Company; 2020. doi:10.1891/9780826182067.0004
Nosek BA, Errington TM. What is replication ? PLoS Biol . 2020;18(3):e3000691. doi:10.1371/journal.pbio.3000691
Aggarwal R, Ranganathan P. Study designs: Part 2 - Descriptive studies . Perspect Clin Res . 2019;10(1):34-36. doi:10.4103/picr.PICR_154_18
Nevid J. Psychology: Concepts and Applications. Wadworth, 2013.
By Kendra Cherry, MSEd Kendra Cherry, MS, is a psychosocial rehabilitation specialist, psychology educator, and author of the "Everything Psychology Book."
Research Hypothesis In Psychology: Types, & Examples
Saul Mcleod, PhD
Editor-in-Chief for Simply Psychology
BSc (Hons) Psychology, MRes, PhD, University of Manchester
Saul Mcleod, PhD., is a qualified psychology teacher with over 18 years of experience in further and higher education. He has been published in peer-reviewed journals, including the Journal of Clinical Psychology.
Learn about our Editorial Process
Olivia Guy-Evans, MSc
Associate Editor for Simply Psychology
BSc (Hons) Psychology, MSc Psychology of Education
Olivia Guy-Evans is a writer and associate editor for Simply Psychology. She has previously worked in healthcare and educational sectors.
On This Page:
A research hypothesis, in its plural form “hypotheses,” is a specific, testable prediction about the anticipated results of a study, established at its outset. It is a key component of the scientific method .
Hypotheses connect theory to data and guide the research process towards expanding scientific understanding
Some key points about hypotheses:
- A hypothesis expresses an expected pattern or relationship. It connects the variables under investigation.
- It is stated in clear, precise terms before any data collection or analysis occurs. This makes the hypothesis testable.
- A hypothesis must be falsifiable. It should be possible, even if unlikely in practice, to collect data that disconfirms rather than supports the hypothesis.
- Hypotheses guide research. Scientists design studies to explicitly evaluate hypotheses about how nature works.
- For a hypothesis to be valid, it must be testable against empirical evidence. The evidence can then confirm or disprove the testable predictions.
- Hypotheses are informed by background knowledge and observation, but go beyond what is already known to propose an explanation of how or why something occurs.
Predictions typically arise from a thorough knowledge of the research literature, curiosity about real-world problems or implications, and integrating this to advance theory. They build on existing literature while providing new insight.
Types of Research Hypotheses
Alternative hypothesis.
The research hypothesis is often called the alternative or experimental hypothesis in experimental research.
It typically suggests a potential relationship between two key variables: the independent variable, which the researcher manipulates, and the dependent variable, which is measured based on those changes.
The alternative hypothesis states a relationship exists between the two variables being studied (one variable affects the other).
A hypothesis is a testable statement or prediction about the relationship between two or more variables. It is a key component of the scientific method. Some key points about hypotheses:
- Important hypotheses lead to predictions that can be tested empirically. The evidence can then confirm or disprove the testable predictions.
In summary, a hypothesis is a precise, testable statement of what researchers expect to happen in a study and why. Hypotheses connect theory to data and guide the research process towards expanding scientific understanding.
An experimental hypothesis predicts what change(s) will occur in the dependent variable when the independent variable is manipulated.
It states that the results are not due to chance and are significant in supporting the theory being investigated.
The alternative hypothesis can be directional, indicating a specific direction of the effect, or non-directional, suggesting a difference without specifying its nature. It’s what researchers aim to support or demonstrate through their study.
Null Hypothesis
The null hypothesis states no relationship exists between the two variables being studied (one variable does not affect the other). There will be no changes in the dependent variable due to manipulating the independent variable.
It states results are due to chance and are not significant in supporting the idea being investigated.
The null hypothesis, positing no effect or relationship, is a foundational contrast to the research hypothesis in scientific inquiry. It establishes a baseline for statistical testing, promoting objectivity by initiating research from a neutral stance.
Many statistical methods are tailored to test the null hypothesis, determining the likelihood of observed results if no true effect exists.
This dual-hypothesis approach provides clarity, ensuring that research intentions are explicit, and fosters consistency across scientific studies, enhancing the standardization and interpretability of research outcomes.
Nondirectional Hypothesis
A non-directional hypothesis, also known as a two-tailed hypothesis, predicts that there is a difference or relationship between two variables but does not specify the direction of this relationship.
It merely indicates that a change or effect will occur without predicting which group will have higher or lower values.
For example, “There is a difference in performance between Group A and Group B” is a non-directional hypothesis.
Directional Hypothesis
A directional (one-tailed) hypothesis predicts the nature of the effect of the independent variable on the dependent variable. It predicts in which direction the change will take place. (i.e., greater, smaller, less, more)
It specifies whether one variable is greater, lesser, or different from another, rather than just indicating that there’s a difference without specifying its nature.
For example, “Exercise increases weight loss” is a directional hypothesis.
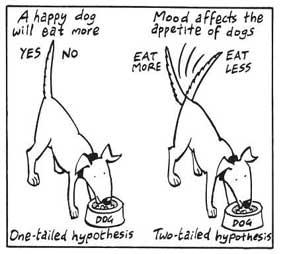
Falsifiability
The Falsification Principle, proposed by Karl Popper , is a way of demarcating science from non-science. It suggests that for a theory or hypothesis to be considered scientific, it must be testable and irrefutable.
Falsifiability emphasizes that scientific claims shouldn’t just be confirmable but should also have the potential to be proven wrong.
It means that there should exist some potential evidence or experiment that could prove the proposition false.
However many confirming instances exist for a theory, it only takes one counter observation to falsify it. For example, the hypothesis that “all swans are white,” can be falsified by observing a black swan.
For Popper, science should attempt to disprove a theory rather than attempt to continually provide evidence to support a research hypothesis.
Can a Hypothesis be Proven?
Hypotheses make probabilistic predictions. They state the expected outcome if a particular relationship exists. However, a study result supporting a hypothesis does not definitively prove it is true.
All studies have limitations. There may be unknown confounding factors or issues that limit the certainty of conclusions. Additional studies may yield different results.
In science, hypotheses can realistically only be supported with some degree of confidence, not proven. The process of science is to incrementally accumulate evidence for and against hypothesized relationships in an ongoing pursuit of better models and explanations that best fit the empirical data. But hypotheses remain open to revision and rejection if that is where the evidence leads.
- Disproving a hypothesis is definitive. Solid disconfirmatory evidence will falsify a hypothesis and require altering or discarding it based on the evidence.
- However, confirming evidence is always open to revision. Other explanations may account for the same results, and additional or contradictory evidence may emerge over time.
We can never 100% prove the alternative hypothesis. Instead, we see if we can disprove, or reject the null hypothesis.
If we reject the null hypothesis, this doesn’t mean that our alternative hypothesis is correct but does support the alternative/experimental hypothesis.
Upon analysis of the results, an alternative hypothesis can be rejected or supported, but it can never be proven to be correct. We must avoid any reference to results proving a theory as this implies 100% certainty, and there is always a chance that evidence may exist which could refute a theory.
How to Write a Hypothesis
- Identify variables . The researcher manipulates the independent variable and the dependent variable is the measured outcome.
- Operationalized the variables being investigated . Operationalization of a hypothesis refers to the process of making the variables physically measurable or testable, e.g. if you are about to study aggression, you might count the number of punches given by participants.
- Decide on a direction for your prediction . If there is evidence in the literature to support a specific effect of the independent variable on the dependent variable, write a directional (one-tailed) hypothesis. If there are limited or ambiguous findings in the literature regarding the effect of the independent variable on the dependent variable, write a non-directional (two-tailed) hypothesis.
- Make it Testable : Ensure your hypothesis can be tested through experimentation or observation. It should be possible to prove it false (principle of falsifiability).
- Clear & concise language . A strong hypothesis is concise (typically one to two sentences long), and formulated using clear and straightforward language, ensuring it’s easily understood and testable.
Consider a hypothesis many teachers might subscribe to: students work better on Monday morning than on Friday afternoon (IV=Day, DV= Standard of work).
Now, if we decide to study this by giving the same group of students a lesson on a Monday morning and a Friday afternoon and then measuring their immediate recall of the material covered in each session, we would end up with the following:
- The alternative hypothesis states that students will recall significantly more information on a Monday morning than on a Friday afternoon.
- The null hypothesis states that there will be no significant difference in the amount recalled on a Monday morning compared to a Friday afternoon. Any difference will be due to chance or confounding factors.
More Examples
- Memory : Participants exposed to classical music during study sessions will recall more items from a list than those who studied in silence.
- Social Psychology : Individuals who frequently engage in social media use will report higher levels of perceived social isolation compared to those who use it infrequently.
- Developmental Psychology : Children who engage in regular imaginative play have better problem-solving skills than those who don’t.
- Clinical Psychology : Cognitive-behavioral therapy will be more effective in reducing symptoms of anxiety over a 6-month period compared to traditional talk therapy.
- Cognitive Psychology : Individuals who multitask between various electronic devices will have shorter attention spans on focused tasks than those who single-task.
- Health Psychology : Patients who practice mindfulness meditation will experience lower levels of chronic pain compared to those who don’t meditate.
- Organizational Psychology : Employees in open-plan offices will report higher levels of stress than those in private offices.
- Behavioral Psychology : Rats rewarded with food after pressing a lever will press it more frequently than rats who receive no reward.

Related Articles
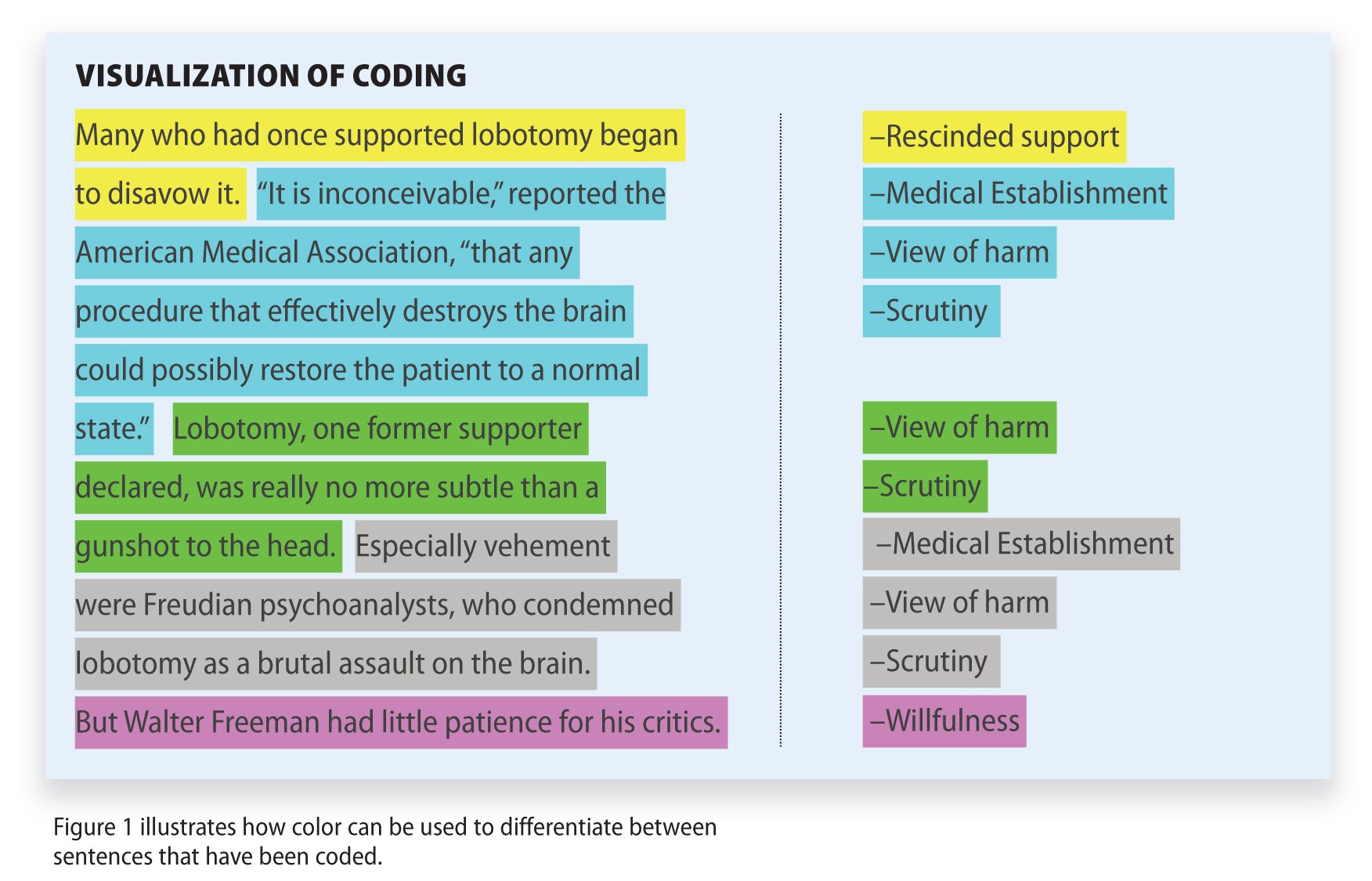
Research Methodology
Qualitative Data Coding
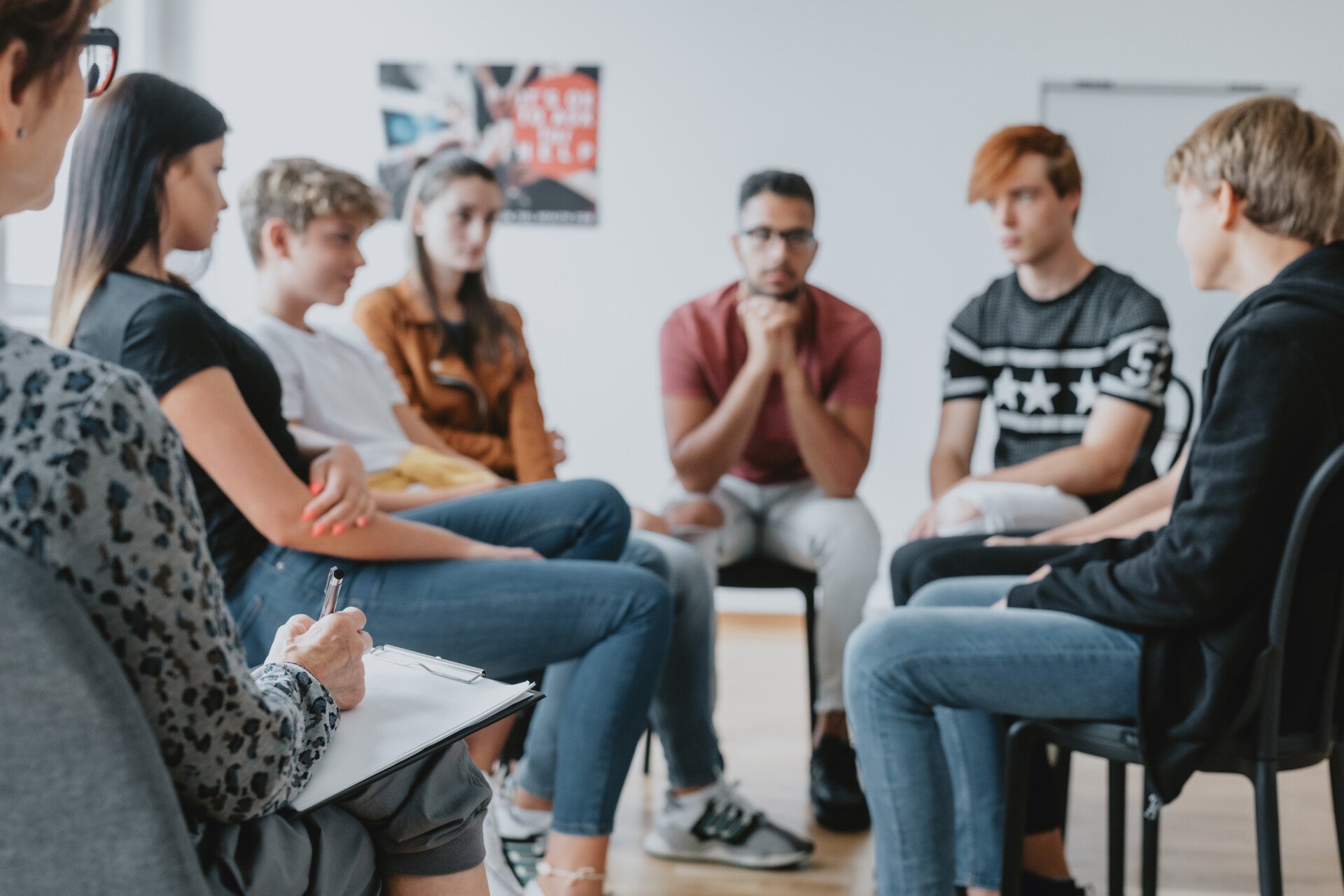
What Is a Focus Group?
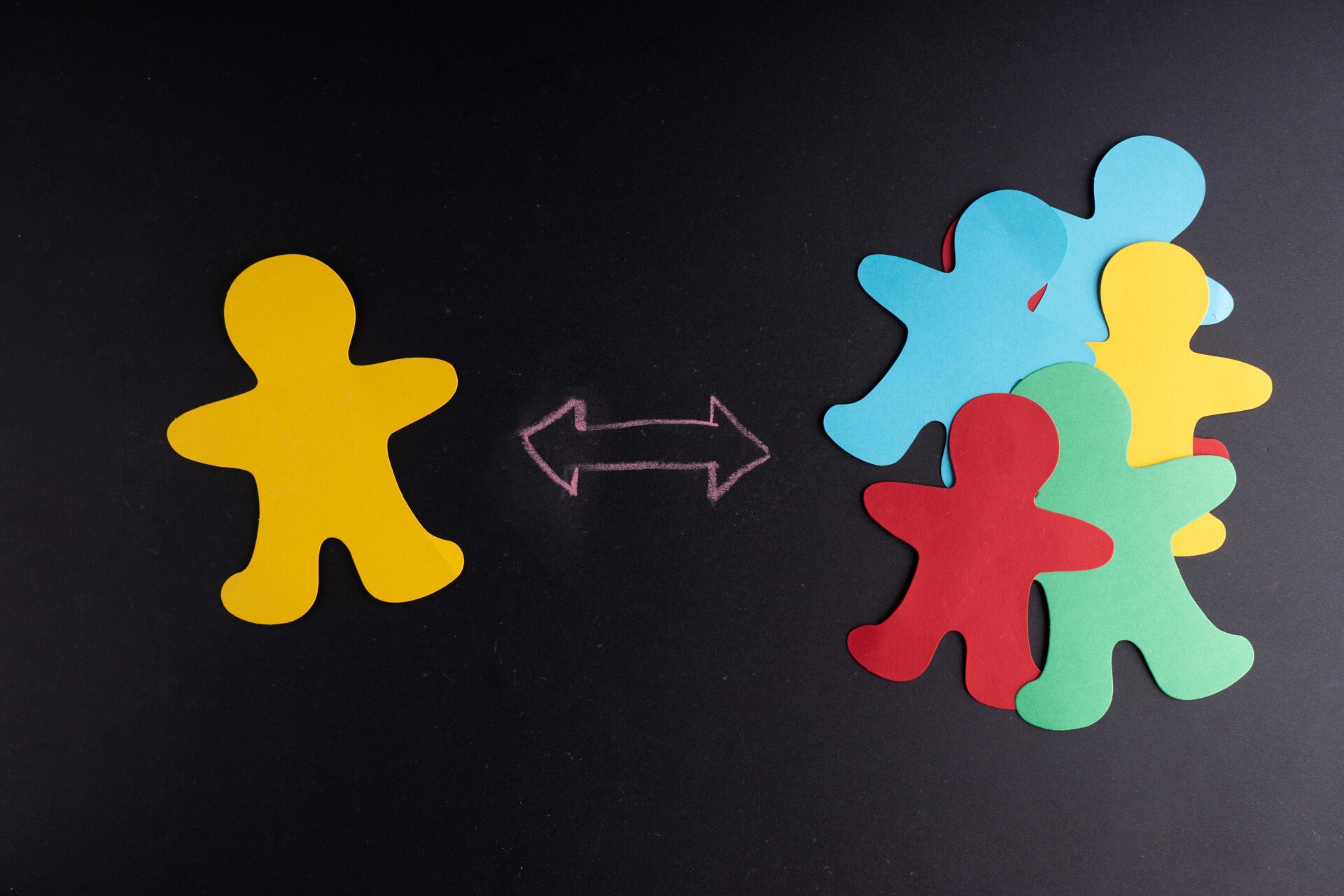
Cross-Cultural Research Methodology In Psychology
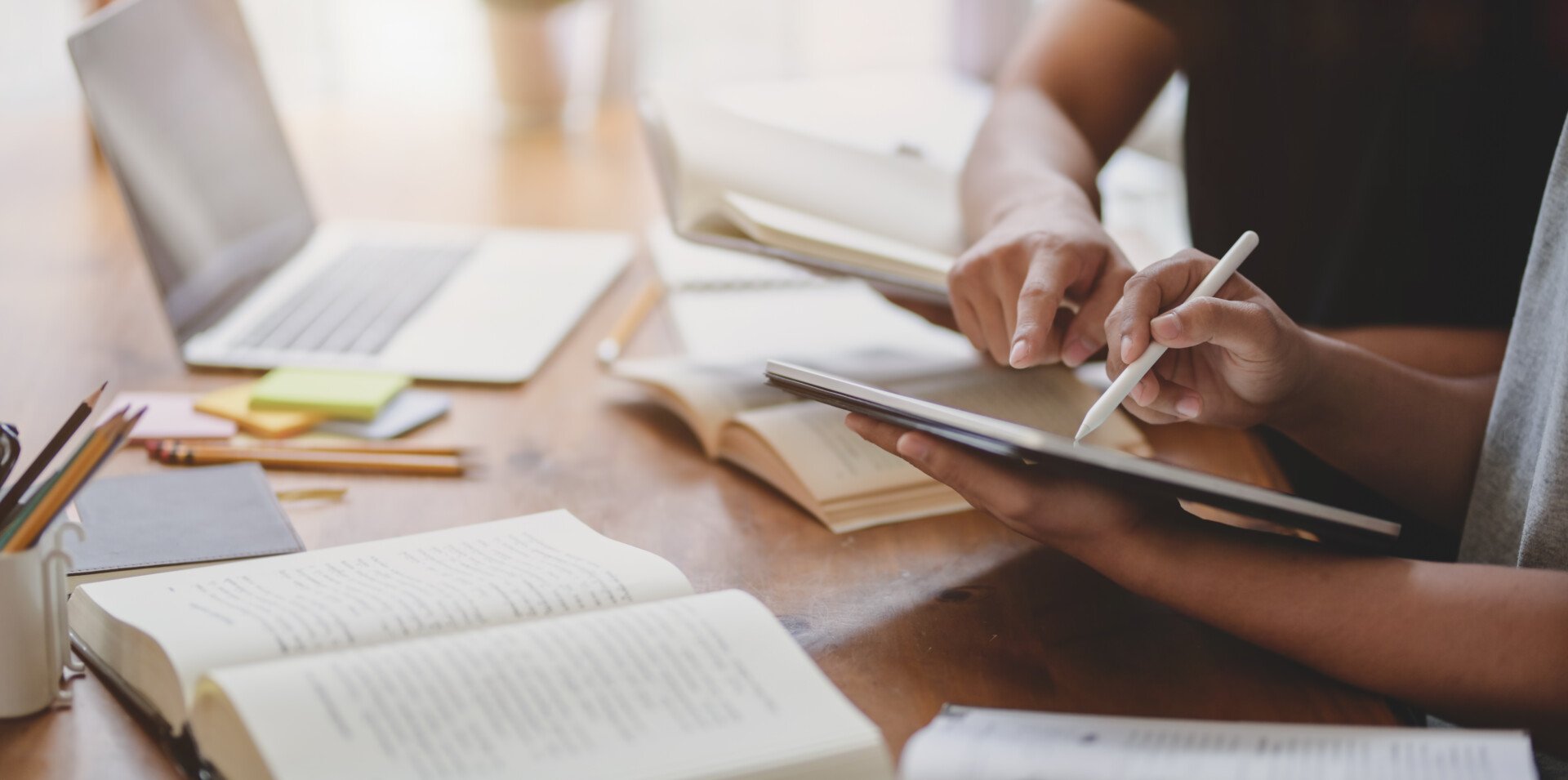
What Is Internal Validity In Research?
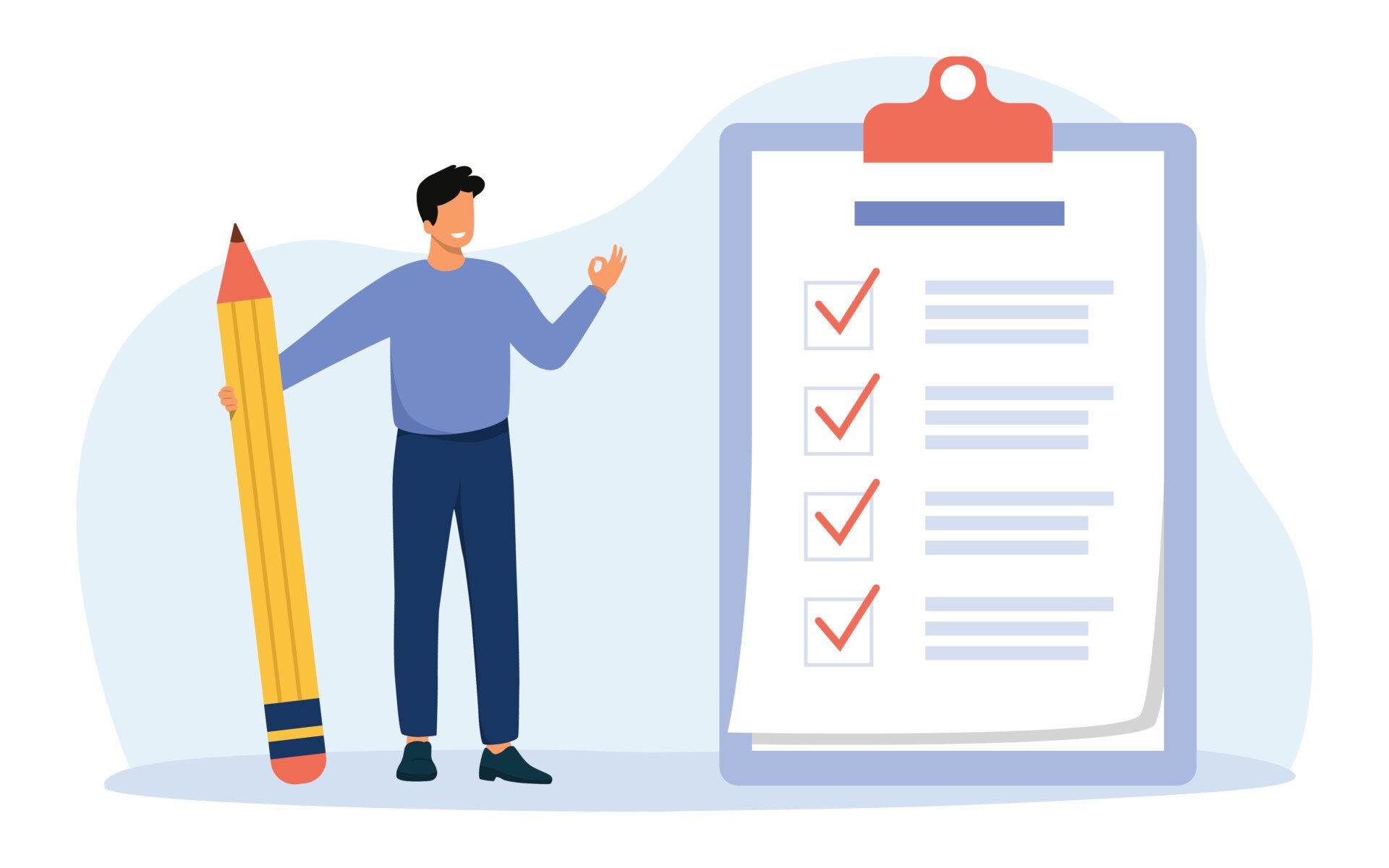
Research Methodology , Statistics
What Is Face Validity In Research? Importance & How To Measure
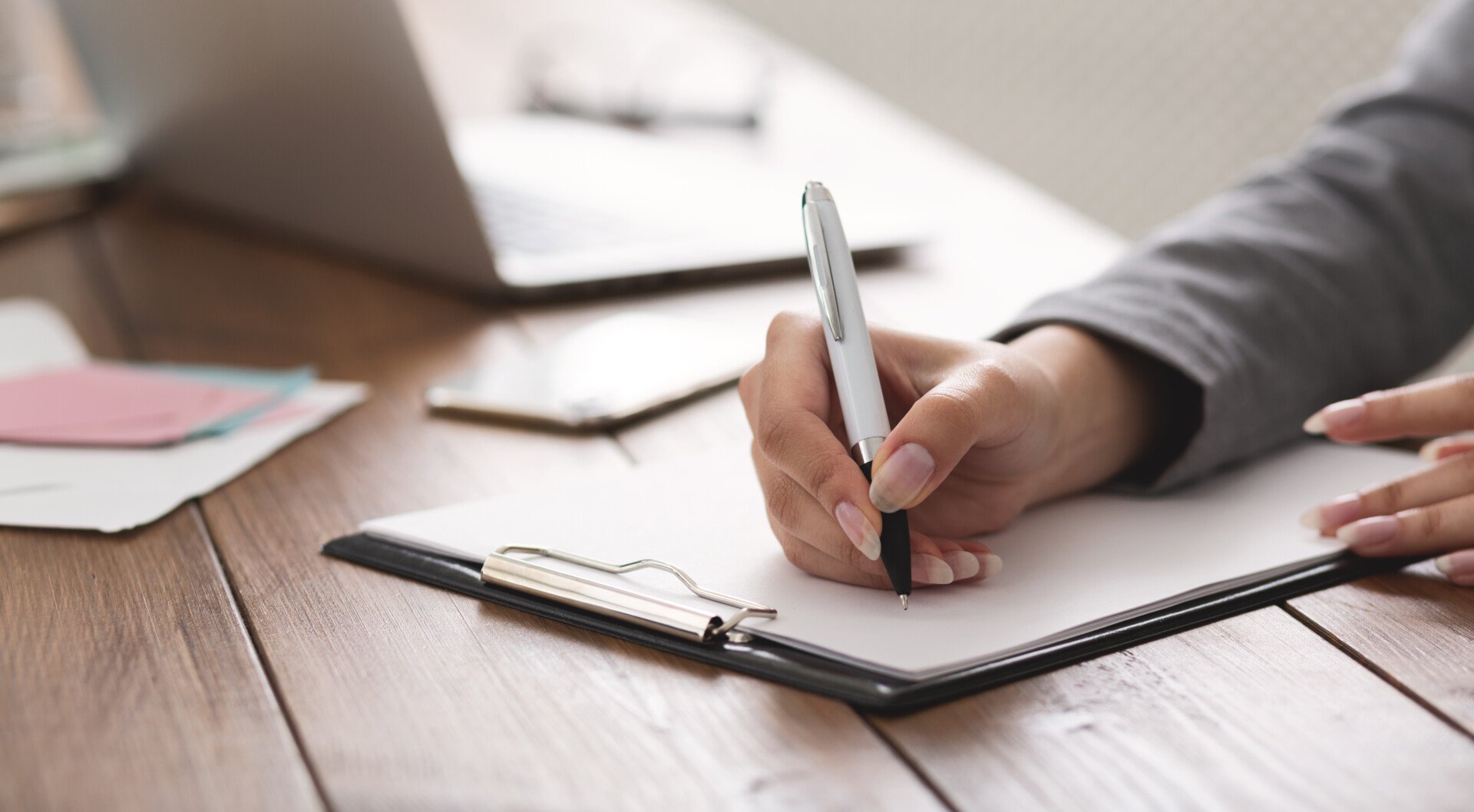
Criterion Validity: Definition & Examples
Have a language expert improve your writing
Run a free plagiarism check in 10 minutes, automatically generate references for free.
- Knowledge Base
- Methodology
- How to Write a Strong Hypothesis | Guide & Examples
How to Write a Strong Hypothesis | Guide & Examples
Published on 6 May 2022 by Shona McCombes .
A hypothesis is a statement that can be tested by scientific research. If you want to test a relationship between two or more variables, you need to write hypotheses before you start your experiment or data collection.
Table of contents
What is a hypothesis, developing a hypothesis (with example), hypothesis examples, frequently asked questions about writing hypotheses.
A hypothesis states your predictions about what your research will find. It is a tentative answer to your research question that has not yet been tested. For some research projects, you might have to write several hypotheses that address different aspects of your research question.
A hypothesis is not just a guess – it should be based on existing theories and knowledge. It also has to be testable, which means you can support or refute it through scientific research methods (such as experiments, observations, and statistical analysis of data).
Variables in hypotheses
Hypotheses propose a relationship between two or more variables . An independent variable is something the researcher changes or controls. A dependent variable is something the researcher observes and measures.
In this example, the independent variable is exposure to the sun – the assumed cause . The dependent variable is the level of happiness – the assumed effect .
Prevent plagiarism, run a free check.
Step 1: ask a question.
Writing a hypothesis begins with a research question that you want to answer. The question should be focused, specific, and researchable within the constraints of your project.
Step 2: Do some preliminary research
Your initial answer to the question should be based on what is already known about the topic. Look for theories and previous studies to help you form educated assumptions about what your research will find.
At this stage, you might construct a conceptual framework to identify which variables you will study and what you think the relationships are between them. Sometimes, you’ll have to operationalise more complex constructs.
Step 3: Formulate your hypothesis
Now you should have some idea of what you expect to find. Write your initial answer to the question in a clear, concise sentence.
Step 4: Refine your hypothesis
You need to make sure your hypothesis is specific and testable. There are various ways of phrasing a hypothesis, but all the terms you use should have clear definitions, and the hypothesis should contain:
- The relevant variables
- The specific group being studied
- The predicted outcome of the experiment or analysis
Step 5: Phrase your hypothesis in three ways
To identify the variables, you can write a simple prediction in if … then form. The first part of the sentence states the independent variable and the second part states the dependent variable.
In academic research, hypotheses are more commonly phrased in terms of correlations or effects, where you directly state the predicted relationship between variables.
If you are comparing two groups, the hypothesis can state what difference you expect to find between them.
Step 6. Write a null hypothesis
If your research involves statistical hypothesis testing , you will also have to write a null hypothesis. The null hypothesis is the default position that there is no association between the variables. The null hypothesis is written as H 0 , while the alternative hypothesis is H 1 or H a .
Hypothesis testing is a formal procedure for investigating our ideas about the world using statistics. It is used by scientists to test specific predictions, called hypotheses , by calculating how likely it is that a pattern or relationship between variables could have arisen by chance.
A hypothesis is not just a guess. It should be based on existing theories and knowledge. It also has to be testable, which means you can support or refute it through scientific research methods (such as experiments, observations, and statistical analysis of data).
A research hypothesis is your proposed answer to your research question. The research hypothesis usually includes an explanation (‘ x affects y because …’).
A statistical hypothesis, on the other hand, is a mathematical statement about a population parameter. Statistical hypotheses always come in pairs: the null and alternative hypotheses. In a well-designed study , the statistical hypotheses correspond logically to the research hypothesis.
Cite this Scribbr article
If you want to cite this source, you can copy and paste the citation or click the ‘Cite this Scribbr article’ button to automatically add the citation to our free Reference Generator.
McCombes, S. (2022, May 06). How to Write a Strong Hypothesis | Guide & Examples. Scribbr. Retrieved 3 June 2024, from https://www.scribbr.co.uk/research-methods/hypothesis-writing/
Is this article helpful?
Shona McCombes
Other students also liked, operationalisation | a guide with examples, pros & cons, what is a conceptual framework | tips & examples, a quick guide to experimental design | 5 steps & examples.
- Privacy Policy

Home » What is a Hypothesis – Types, Examples and Writing Guide
What is a Hypothesis – Types, Examples and Writing Guide
Table of Contents

Definition:
Hypothesis is an educated guess or proposed explanation for a phenomenon, based on some initial observations or data. It is a tentative statement that can be tested and potentially proven or disproven through further investigation and experimentation.
Hypothesis is often used in scientific research to guide the design of experiments and the collection and analysis of data. It is an essential element of the scientific method, as it allows researchers to make predictions about the outcome of their experiments and to test those predictions to determine their accuracy.
Types of Hypothesis
Types of Hypothesis are as follows:
Research Hypothesis
A research hypothesis is a statement that predicts a relationship between variables. It is usually formulated as a specific statement that can be tested through research, and it is often used in scientific research to guide the design of experiments.
Null Hypothesis
The null hypothesis is a statement that assumes there is no significant difference or relationship between variables. It is often used as a starting point for testing the research hypothesis, and if the results of the study reject the null hypothesis, it suggests that there is a significant difference or relationship between variables.
Alternative Hypothesis
An alternative hypothesis is a statement that assumes there is a significant difference or relationship between variables. It is often used as an alternative to the null hypothesis and is tested against the null hypothesis to determine which statement is more accurate.
Directional Hypothesis
A directional hypothesis is a statement that predicts the direction of the relationship between variables. For example, a researcher might predict that increasing the amount of exercise will result in a decrease in body weight.
Non-directional Hypothesis
A non-directional hypothesis is a statement that predicts the relationship between variables but does not specify the direction. For example, a researcher might predict that there is a relationship between the amount of exercise and body weight, but they do not specify whether increasing or decreasing exercise will affect body weight.
Statistical Hypothesis
A statistical hypothesis is a statement that assumes a particular statistical model or distribution for the data. It is often used in statistical analysis to test the significance of a particular result.
Composite Hypothesis
A composite hypothesis is a statement that assumes more than one condition or outcome. It can be divided into several sub-hypotheses, each of which represents a different possible outcome.
Empirical Hypothesis
An empirical hypothesis is a statement that is based on observed phenomena or data. It is often used in scientific research to develop theories or models that explain the observed phenomena.
Simple Hypothesis
A simple hypothesis is a statement that assumes only one outcome or condition. It is often used in scientific research to test a single variable or factor.
Complex Hypothesis
A complex hypothesis is a statement that assumes multiple outcomes or conditions. It is often used in scientific research to test the effects of multiple variables or factors on a particular outcome.
Applications of Hypothesis
Hypotheses are used in various fields to guide research and make predictions about the outcomes of experiments or observations. Here are some examples of how hypotheses are applied in different fields:
- Science : In scientific research, hypotheses are used to test the validity of theories and models that explain natural phenomena. For example, a hypothesis might be formulated to test the effects of a particular variable on a natural system, such as the effects of climate change on an ecosystem.
- Medicine : In medical research, hypotheses are used to test the effectiveness of treatments and therapies for specific conditions. For example, a hypothesis might be formulated to test the effects of a new drug on a particular disease.
- Psychology : In psychology, hypotheses are used to test theories and models of human behavior and cognition. For example, a hypothesis might be formulated to test the effects of a particular stimulus on the brain or behavior.
- Sociology : In sociology, hypotheses are used to test theories and models of social phenomena, such as the effects of social structures or institutions on human behavior. For example, a hypothesis might be formulated to test the effects of income inequality on crime rates.
- Business : In business research, hypotheses are used to test the validity of theories and models that explain business phenomena, such as consumer behavior or market trends. For example, a hypothesis might be formulated to test the effects of a new marketing campaign on consumer buying behavior.
- Engineering : In engineering, hypotheses are used to test the effectiveness of new technologies or designs. For example, a hypothesis might be formulated to test the efficiency of a new solar panel design.
How to write a Hypothesis
Here are the steps to follow when writing a hypothesis:
Identify the Research Question
The first step is to identify the research question that you want to answer through your study. This question should be clear, specific, and focused. It should be something that can be investigated empirically and that has some relevance or significance in the field.
Conduct a Literature Review
Before writing your hypothesis, it’s essential to conduct a thorough literature review to understand what is already known about the topic. This will help you to identify the research gap and formulate a hypothesis that builds on existing knowledge.
Determine the Variables
The next step is to identify the variables involved in the research question. A variable is any characteristic or factor that can vary or change. There are two types of variables: independent and dependent. The independent variable is the one that is manipulated or changed by the researcher, while the dependent variable is the one that is measured or observed as a result of the independent variable.
Formulate the Hypothesis
Based on the research question and the variables involved, you can now formulate your hypothesis. A hypothesis should be a clear and concise statement that predicts the relationship between the variables. It should be testable through empirical research and based on existing theory or evidence.
Write the Null Hypothesis
The null hypothesis is the opposite of the alternative hypothesis, which is the hypothesis that you are testing. The null hypothesis states that there is no significant difference or relationship between the variables. It is important to write the null hypothesis because it allows you to compare your results with what would be expected by chance.
Refine the Hypothesis
After formulating the hypothesis, it’s important to refine it and make it more precise. This may involve clarifying the variables, specifying the direction of the relationship, or making the hypothesis more testable.
Examples of Hypothesis
Here are a few examples of hypotheses in different fields:
- Psychology : “Increased exposure to violent video games leads to increased aggressive behavior in adolescents.”
- Biology : “Higher levels of carbon dioxide in the atmosphere will lead to increased plant growth.”
- Sociology : “Individuals who grow up in households with higher socioeconomic status will have higher levels of education and income as adults.”
- Education : “Implementing a new teaching method will result in higher student achievement scores.”
- Marketing : “Customers who receive a personalized email will be more likely to make a purchase than those who receive a generic email.”
- Physics : “An increase in temperature will cause an increase in the volume of a gas, assuming all other variables remain constant.”
- Medicine : “Consuming a diet high in saturated fats will increase the risk of developing heart disease.”
Purpose of Hypothesis
The purpose of a hypothesis is to provide a testable explanation for an observed phenomenon or a prediction of a future outcome based on existing knowledge or theories. A hypothesis is an essential part of the scientific method and helps to guide the research process by providing a clear focus for investigation. It enables scientists to design experiments or studies to gather evidence and data that can support or refute the proposed explanation or prediction.
The formulation of a hypothesis is based on existing knowledge, observations, and theories, and it should be specific, testable, and falsifiable. A specific hypothesis helps to define the research question, which is important in the research process as it guides the selection of an appropriate research design and methodology. Testability of the hypothesis means that it can be proven or disproven through empirical data collection and analysis. Falsifiability means that the hypothesis should be formulated in such a way that it can be proven wrong if it is incorrect.
In addition to guiding the research process, the testing of hypotheses can lead to new discoveries and advancements in scientific knowledge. When a hypothesis is supported by the data, it can be used to develop new theories or models to explain the observed phenomenon. When a hypothesis is not supported by the data, it can help to refine existing theories or prompt the development of new hypotheses to explain the phenomenon.
When to use Hypothesis
Here are some common situations in which hypotheses are used:
- In scientific research , hypotheses are used to guide the design of experiments and to help researchers make predictions about the outcomes of those experiments.
- In social science research , hypotheses are used to test theories about human behavior, social relationships, and other phenomena.
- I n business , hypotheses can be used to guide decisions about marketing, product development, and other areas. For example, a hypothesis might be that a new product will sell well in a particular market, and this hypothesis can be tested through market research.
Characteristics of Hypothesis
Here are some common characteristics of a hypothesis:
- Testable : A hypothesis must be able to be tested through observation or experimentation. This means that it must be possible to collect data that will either support or refute the hypothesis.
- Falsifiable : A hypothesis must be able to be proven false if it is not supported by the data. If a hypothesis cannot be falsified, then it is not a scientific hypothesis.
- Clear and concise : A hypothesis should be stated in a clear and concise manner so that it can be easily understood and tested.
- Based on existing knowledge : A hypothesis should be based on existing knowledge and research in the field. It should not be based on personal beliefs or opinions.
- Specific : A hypothesis should be specific in terms of the variables being tested and the predicted outcome. This will help to ensure that the research is focused and well-designed.
- Tentative: A hypothesis is a tentative statement or assumption that requires further testing and evidence to be confirmed or refuted. It is not a final conclusion or assertion.
- Relevant : A hypothesis should be relevant to the research question or problem being studied. It should address a gap in knowledge or provide a new perspective on the issue.
Advantages of Hypothesis
Hypotheses have several advantages in scientific research and experimentation:
- Guides research: A hypothesis provides a clear and specific direction for research. It helps to focus the research question, select appropriate methods and variables, and interpret the results.
- Predictive powe r: A hypothesis makes predictions about the outcome of research, which can be tested through experimentation. This allows researchers to evaluate the validity of the hypothesis and make new discoveries.
- Facilitates communication: A hypothesis provides a common language and framework for scientists to communicate with one another about their research. This helps to facilitate the exchange of ideas and promotes collaboration.
- Efficient use of resources: A hypothesis helps researchers to use their time, resources, and funding efficiently by directing them towards specific research questions and methods that are most likely to yield results.
- Provides a basis for further research: A hypothesis that is supported by data provides a basis for further research and exploration. It can lead to new hypotheses, theories, and discoveries.
- Increases objectivity: A hypothesis can help to increase objectivity in research by providing a clear and specific framework for testing and interpreting results. This can reduce bias and increase the reliability of research findings.
Limitations of Hypothesis
Some Limitations of the Hypothesis are as follows:
- Limited to observable phenomena: Hypotheses are limited to observable phenomena and cannot account for unobservable or intangible factors. This means that some research questions may not be amenable to hypothesis testing.
- May be inaccurate or incomplete: Hypotheses are based on existing knowledge and research, which may be incomplete or inaccurate. This can lead to flawed hypotheses and erroneous conclusions.
- May be biased: Hypotheses may be biased by the researcher’s own beliefs, values, or assumptions. This can lead to selective interpretation of data and a lack of objectivity in research.
- Cannot prove causation: A hypothesis can only show a correlation between variables, but it cannot prove causation. This requires further experimentation and analysis.
- Limited to specific contexts: Hypotheses are limited to specific contexts and may not be generalizable to other situations or populations. This means that results may not be applicable in other contexts or may require further testing.
- May be affected by chance : Hypotheses may be affected by chance or random variation, which can obscure or distort the true relationship between variables.
About the author
Muhammad Hassan
Researcher, Academic Writer, Web developer
You may also like

Research Process – Steps, Examples and Tips

Informed Consent in Research – Types, Templates...

Research Summary – Structure, Examples and...

Data Analysis – Process, Methods and Types

Dissertation Methodology – Structure, Example...

Data Collection – Methods Types and Examples
What is a scientific hypothesis?
It's the initial building block in the scientific method.
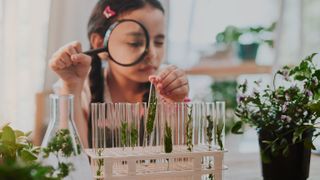
Hypothesis basics
What makes a hypothesis testable.
- Types of hypotheses
- Hypothesis versus theory
Additional resources
Bibliography.
A scientific hypothesis is a tentative, testable explanation for a phenomenon in the natural world. It's the initial building block in the scientific method . Many describe it as an "educated guess" based on prior knowledge and observation. While this is true, a hypothesis is more informed than a guess. While an "educated guess" suggests a random prediction based on a person's expertise, developing a hypothesis requires active observation and background research.
The basic idea of a hypothesis is that there is no predetermined outcome. For a solution to be termed a scientific hypothesis, it has to be an idea that can be supported or refuted through carefully crafted experimentation or observation. This concept, called falsifiability and testability, was advanced in the mid-20th century by Austrian-British philosopher Karl Popper in his famous book "The Logic of Scientific Discovery" (Routledge, 1959).
A key function of a hypothesis is to derive predictions about the results of future experiments and then perform those experiments to see whether they support the predictions.
A hypothesis is usually written in the form of an if-then statement, which gives a possibility (if) and explains what may happen because of the possibility (then). The statement could also include "may," according to California State University, Bakersfield .
Here are some examples of hypothesis statements:
- If garlic repels fleas, then a dog that is given garlic every day will not get fleas.
- If sugar causes cavities, then people who eat a lot of candy may be more prone to cavities.
- If ultraviolet light can damage the eyes, then maybe this light can cause blindness.
A useful hypothesis should be testable and falsifiable. That means that it should be possible to prove it wrong. A theory that can't be proved wrong is nonscientific, according to Karl Popper's 1963 book " Conjectures and Refutations ."
An example of an untestable statement is, "Dogs are better than cats." That's because the definition of "better" is vague and subjective. However, an untestable statement can be reworded to make it testable. For example, the previous statement could be changed to this: "Owning a dog is associated with higher levels of physical fitness than owning a cat." With this statement, the researcher can take measures of physical fitness from dog and cat owners and compare the two.
Types of scientific hypotheses
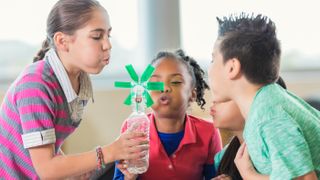
In an experiment, researchers generally state their hypotheses in two ways. The null hypothesis predicts that there will be no relationship between the variables tested, or no difference between the experimental groups. The alternative hypothesis predicts the opposite: that there will be a difference between the experimental groups. This is usually the hypothesis scientists are most interested in, according to the University of Miami .
For example, a null hypothesis might state, "There will be no difference in the rate of muscle growth between people who take a protein supplement and people who don't." The alternative hypothesis would state, "There will be a difference in the rate of muscle growth between people who take a protein supplement and people who don't."
If the results of the experiment show a relationship between the variables, then the null hypothesis has been rejected in favor of the alternative hypothesis, according to the book " Research Methods in Psychology " (BCcampus, 2015).
There are other ways to describe an alternative hypothesis. The alternative hypothesis above does not specify a direction of the effect, only that there will be a difference between the two groups. That type of prediction is called a two-tailed hypothesis. If a hypothesis specifies a certain direction — for example, that people who take a protein supplement will gain more muscle than people who don't — it is called a one-tailed hypothesis, according to William M. K. Trochim , a professor of Policy Analysis and Management at Cornell University.
Sometimes, errors take place during an experiment. These errors can happen in one of two ways. A type I error is when the null hypothesis is rejected when it is true. This is also known as a false positive. A type II error occurs when the null hypothesis is not rejected when it is false. This is also known as a false negative, according to the University of California, Berkeley .
A hypothesis can be rejected or modified, but it can never be proved correct 100% of the time. For example, a scientist can form a hypothesis stating that if a certain type of tomato has a gene for red pigment, that type of tomato will be red. During research, the scientist then finds that each tomato of this type is red. Though the findings confirm the hypothesis, there may be a tomato of that type somewhere in the world that isn't red. Thus, the hypothesis is true, but it may not be true 100% of the time.
Scientific theory vs. scientific hypothesis
The best hypotheses are simple. They deal with a relatively narrow set of phenomena. But theories are broader; they generally combine multiple hypotheses into a general explanation for a wide range of phenomena, according to the University of California, Berkeley . For example, a hypothesis might state, "If animals adapt to suit their environments, then birds that live on islands with lots of seeds to eat will have differently shaped beaks than birds that live on islands with lots of insects to eat." After testing many hypotheses like these, Charles Darwin formulated an overarching theory: the theory of evolution by natural selection.
"Theories are the ways that we make sense of what we observe in the natural world," Tanner said. "Theories are structures of ideas that explain and interpret facts."
- Read more about writing a hypothesis, from the American Medical Writers Association.
- Find out why a hypothesis isn't always necessary in science, from The American Biology Teacher.
- Learn about null and alternative hypotheses, from Prof. Essa on YouTube .
Encyclopedia Britannica. Scientific Hypothesis. Jan. 13, 2022. https://www.britannica.com/science/scientific-hypothesis
Karl Popper, "The Logic of Scientific Discovery," Routledge, 1959.
California State University, Bakersfield, "Formatting a testable hypothesis." https://www.csub.edu/~ddodenhoff/Bio100/Bio100sp04/formattingahypothesis.htm
Karl Popper, "Conjectures and Refutations," Routledge, 1963.
Price, P., Jhangiani, R., & Chiang, I., "Research Methods of Psychology — 2nd Canadian Edition," BCcampus, 2015.
University of Miami, "The Scientific Method" http://www.bio.miami.edu/dana/161/evolution/161app1_scimethod.pdf
William M.K. Trochim, "Research Methods Knowledge Base," https://conjointly.com/kb/hypotheses-explained/
University of California, Berkeley, "Multiple Hypothesis Testing and False Discovery Rate" https://www.stat.berkeley.edu/~hhuang/STAT141/Lecture-FDR.pdf
University of California, Berkeley, "Science at multiple levels" https://undsci.berkeley.edu/article/0_0_0/howscienceworks_19
Sign up for the Live Science daily newsletter now
Get the world’s most fascinating discoveries delivered straight to your inbox.
Cutting pollution from the shipping industry accidentally increased global warming, study suggests
The 165-year reign of oil is coming to an end. But will we ever be able to live without it?
32 unusual medical cases
Most Popular
- 2 Elusive 'octopus squid' with world's largest biological lights attacks camera in striking new video
- 3 3,500-year-old rock art of wild sheep and double-humped camels revealed in Kazakhstan
- 4 Solar power generated enough heat to power a steel furnace
- 5 Wreck of WWII 'Hit 'Em Harder' submarine, which sank with 79 crew on board, discovered in South China Sea
- 2 3,500-year-old rock art of wild sheep and double-humped camels revealed in Kazakhstan
- 3 Cutting pollution from the shipping industry accidentally increased global warming, study suggests
- 4 Wreck of WWII 'Hit 'Em Harder' submarine, which sank with 79 crew on board, discovered in South China Sea
- 5 Eye of the Sahara: Mauritania's giant rock dome that towers over the desert

An official website of the United States government
The .gov means it’s official. Federal government websites often end in .gov or .mil. Before sharing sensitive information, make sure you’re on a federal government site.
The site is secure. The https:// ensures that you are connecting to the official website and that any information you provide is encrypted and transmitted securely.
- Publications
- Account settings
Preview improvements coming to the PMC website in October 2024. Learn More or Try it out now .
- Advanced Search
- Journal List
- J Korean Med Sci
- v.36(50); 2021 Dec 27

Formulating Hypotheses for Different Study Designs
Durga prasanna misra.
1 Department of Clinical Immunology and Rheumatology, Sanjay Gandhi Postgraduate Institute of Medical Sciences, Lucknow, India.
Armen Yuri Gasparyan
2 Departments of Rheumatology and Research and Development, Dudley Group NHS Foundation Trust (Teaching Trust of the University of Birmingham, UK), Russells Hall Hospital, Dudley, UK.
Olena Zimba
3 Department of Internal Medicine #2, Danylo Halytsky Lviv National Medical University, Lviv, Ukraine.
Marlen Yessirkepov
4 Department of Biology and Biochemistry, South Kazakhstan Medical Academy, Shymkent, Kazakhstan.
Vikas Agarwal
George d. kitas.
5 Centre for Epidemiology versus Arthritis, University of Manchester, Manchester, UK.
Generating a testable working hypothesis is the first step towards conducting original research. Such research may prove or disprove the proposed hypothesis. Case reports, case series, online surveys and other observational studies, clinical trials, and narrative reviews help to generate hypotheses. Observational and interventional studies help to test hypotheses. A good hypothesis is usually based on previous evidence-based reports. Hypotheses without evidence-based justification and a priori ideas are not received favourably by the scientific community. Original research to test a hypothesis should be carefully planned to ensure appropriate methodology and adequate statistical power. While hypotheses can challenge conventional thinking and may be controversial, they should not be destructive. A hypothesis should be tested by ethically sound experiments with meaningful ethical and clinical implications. The coronavirus disease 2019 pandemic has brought into sharp focus numerous hypotheses, some of which were proven (e.g. effectiveness of corticosteroids in those with hypoxia) while others were disproven (e.g. ineffectiveness of hydroxychloroquine and ivermectin).
Graphical Abstract

DEFINING WORKING AND STANDALONE SCIENTIFIC HYPOTHESES
Science is the systematized description of natural truths and facts. Routine observations of existing life phenomena lead to the creative thinking and generation of ideas about mechanisms of such phenomena and related human interventions. Such ideas presented in a structured format can be viewed as hypotheses. After generating a hypothesis, it is necessary to test it to prove its validity. Thus, hypothesis can be defined as a proposed mechanism of a naturally occurring event or a proposed outcome of an intervention. 1 , 2
Hypothesis testing requires choosing the most appropriate methodology and adequately powering statistically the study to be able to “prove” or “disprove” it within predetermined and widely accepted levels of certainty. This entails sample size calculation that often takes into account previously published observations and pilot studies. 2 , 3 In the era of digitization, hypothesis generation and testing may benefit from the availability of numerous platforms for data dissemination, social networking, and expert validation. Related expert evaluations may reveal strengths and limitations of proposed ideas at early stages of post-publication promotion, preventing the implementation of unsupported controversial points. 4
Thus, hypothesis generation is an important initial step in the research workflow, reflecting accumulating evidence and experts' stance. In this article, we overview the genesis and importance of scientific hypotheses and their relevance in the era of the coronavirus disease 2019 (COVID-19) pandemic.
DO WE NEED HYPOTHESES FOR ALL STUDY DESIGNS?
Broadly, research can be categorized as primary or secondary. In the context of medicine, primary research may include real-life observations of disease presentations and outcomes. Single case descriptions, which often lead to new ideas and hypotheses, serve as important starting points or justifications for case series and cohort studies. The importance of case descriptions is particularly evident in the context of the COVID-19 pandemic when unique, educational case reports have heralded a new era in clinical medicine. 5
Case series serve similar purpose to single case reports, but are based on a slightly larger quantum of information. Observational studies, including online surveys, describe the existing phenomena at a larger scale, often involving various control groups. Observational studies include variable-scale epidemiological investigations at different time points. Interventional studies detail the results of therapeutic interventions.
Secondary research is based on already published literature and does not directly involve human or animal subjects. Review articles are generated by secondary research. These could be systematic reviews which follow methods akin to primary research but with the unit of study being published papers rather than humans or animals. Systematic reviews have a rigid structure with a mandatory search strategy encompassing multiple databases, systematic screening of search results against pre-defined inclusion and exclusion criteria, critical appraisal of study quality and an optional component of collating results across studies quantitatively to derive summary estimates (meta-analysis). 6 Narrative reviews, on the other hand, have a more flexible structure. Systematic literature searches to minimise bias in selection of articles are highly recommended but not mandatory. 7 Narrative reviews are influenced by the authors' viewpoint who may preferentially analyse selected sets of articles. 8
In relation to primary research, case studies and case series are generally not driven by a working hypothesis. Rather, they serve as a basis to generate a hypothesis. Observational or interventional studies should have a hypothesis for choosing research design and sample size. The results of observational and interventional studies further lead to the generation of new hypotheses, testing of which forms the basis of future studies. Review articles, on the other hand, may not be hypothesis-driven, but form fertile ground to generate future hypotheses for evaluation. Fig. 1 summarizes which type of studies are hypothesis-driven and which lead on to hypothesis generation.

STANDARDS OF WORKING AND SCIENTIFIC HYPOTHESES
A review of the published literature did not enable the identification of clearly defined standards for working and scientific hypotheses. It is essential to distinguish influential versus not influential hypotheses, evidence-based hypotheses versus a priori statements and ideas, ethical versus unethical, or potentially harmful ideas. The following points are proposed for consideration while generating working and scientific hypotheses. 1 , 2 Table 1 summarizes these points.
Evidence-based data
A scientific hypothesis should have a sound basis on previously published literature as well as the scientist's observations. Randomly generated (a priori) hypotheses are unlikely to be proven. A thorough literature search should form the basis of a hypothesis based on published evidence. 7
Unless a scientific hypothesis can be tested, it can neither be proven nor be disproven. Therefore, a scientific hypothesis should be amenable to testing with the available technologies and the present understanding of science.
Supported by pilot studies
If a hypothesis is based purely on a novel observation by the scientist in question, it should be grounded on some preliminary studies to support it. For example, if a drug that targets a specific cell population is hypothesized to be useful in a particular disease setting, then there must be some preliminary evidence that the specific cell population plays a role in driving that disease process.
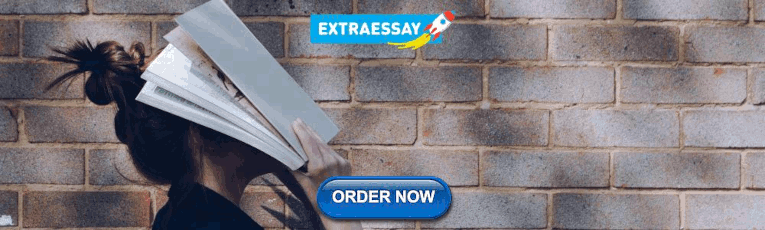
Testable by ethical studies
The hypothesis should be testable by experiments that are ethically acceptable. 9 For example, a hypothesis that parachutes reduce mortality from falls from an airplane cannot be tested using a randomized controlled trial. 10 This is because it is obvious that all those jumping from a flying plane without a parachute would likely die. Similarly, the hypothesis that smoking tobacco causes lung cancer cannot be tested by a clinical trial that makes people take up smoking (since there is considerable evidence for the health hazards associated with smoking). Instead, long-term observational studies comparing outcomes in those who smoke and those who do not, as was performed in the landmark epidemiological case control study by Doll and Hill, 11 are more ethical and practical.
Balance between scientific temper and controversy
Novel findings, including novel hypotheses, particularly those that challenge established norms, are bound to face resistance for their wider acceptance. Such resistance is inevitable until the time such findings are proven with appropriate scientific rigor. However, hypotheses that generate controversy are generally unwelcome. For example, at the time the pandemic of human immunodeficiency virus (HIV) and AIDS was taking foot, there were numerous deniers that refused to believe that HIV caused AIDS. 12 , 13 Similarly, at a time when climate change is causing catastrophic changes to weather patterns worldwide, denial that climate change is occurring and consequent attempts to block climate change are certainly unwelcome. 14 The denialism and misinformation during the COVID-19 pandemic, including unfortunate examples of vaccine hesitancy, are more recent examples of controversial hypotheses not backed by science. 15 , 16 An example of a controversial hypothesis that was a revolutionary scientific breakthrough was the hypothesis put forth by Warren and Marshall that Helicobacter pylori causes peptic ulcers. Initially, the hypothesis that a microorganism could cause gastritis and gastric ulcers faced immense resistance. When the scientists that proposed the hypothesis themselves ingested H. pylori to induce gastritis in themselves, only then could they convince the wider world about their hypothesis. Such was the impact of the hypothesis was that Barry Marshall and Robin Warren were awarded the Nobel Prize in Physiology or Medicine in 2005 for this discovery. 17 , 18
DISTINGUISHING THE MOST INFLUENTIAL HYPOTHESES
Influential hypotheses are those that have stood the test of time. An archetype of an influential hypothesis is that proposed by Edward Jenner in the eighteenth century that cowpox infection protects against smallpox. While this observation had been reported for nearly a century before this time, it had not been suitably tested and publicised until Jenner conducted his experiments on a young boy by demonstrating protection against smallpox after inoculation with cowpox. 19 These experiments were the basis for widespread smallpox immunization strategies worldwide in the 20th century which resulted in the elimination of smallpox as a human disease today. 20
Other influential hypotheses are those which have been read and cited widely. An example of this is the hygiene hypothesis proposing an inverse relationship between infections in early life and allergies or autoimmunity in adulthood. An analysis reported that this hypothesis had been cited more than 3,000 times on Scopus. 1
LESSONS LEARNED FROM HYPOTHESES AMIDST THE COVID-19 PANDEMIC
The COVID-19 pandemic devastated the world like no other in recent memory. During this period, various hypotheses emerged, understandably so considering the public health emergency situation with innumerable deaths and suffering for humanity. Within weeks of the first reports of COVID-19, aberrant immune system activation was identified as a key driver of organ dysfunction and mortality in this disease. 21 Consequently, numerous drugs that suppress the immune system or abrogate the activation of the immune system were hypothesized to have a role in COVID-19. 22 One of the earliest drugs hypothesized to have a benefit was hydroxychloroquine. Hydroxychloroquine was proposed to interfere with Toll-like receptor activation and consequently ameliorate the aberrant immune system activation leading to pathology in COVID-19. 22 The drug was also hypothesized to have a prophylactic role in preventing infection or disease severity in COVID-19. It was also touted as a wonder drug for the disease by many prominent international figures. However, later studies which were well-designed randomized controlled trials failed to demonstrate any benefit of hydroxychloroquine in COVID-19. 23 , 24 , 25 , 26 Subsequently, azithromycin 27 , 28 and ivermectin 29 were hypothesized as potential therapies for COVID-19, but were not supported by evidence from randomized controlled trials. The role of vitamin D in preventing disease severity was also proposed, but has not been proven definitively until now. 30 , 31 On the other hand, randomized controlled trials identified the evidence supporting dexamethasone 32 and interleukin-6 pathway blockade with tocilizumab as effective therapies for COVID-19 in specific situations such as at the onset of hypoxia. 33 , 34 Clues towards the apparent effectiveness of various drugs against severe acute respiratory syndrome coronavirus 2 in vitro but their ineffectiveness in vivo have recently been identified. Many of these drugs are weak, lipophilic bases and some others induce phospholipidosis which results in apparent in vitro effectiveness due to non-specific off-target effects that are not replicated inside living systems. 35 , 36
Another hypothesis proposed was the association of the routine policy of vaccination with Bacillus Calmette-Guerin (BCG) with lower deaths due to COVID-19. This hypothesis emerged in the middle of 2020 when COVID-19 was still taking foot in many parts of the world. 37 , 38 Subsequently, many countries which had lower deaths at that time point went on to have higher numbers of mortality, comparable to other areas of the world. Furthermore, the hypothesis that BCG vaccination reduced COVID-19 mortality was a classic example of ecological fallacy. Associations between population level events (ecological studies; in this case, BCG vaccination and COVID-19 mortality) cannot be directly extrapolated to the individual level. Furthermore, such associations cannot per se be attributed as causal in nature, and can only serve to generate hypotheses that need to be tested at the individual level. 39
IS TRADITIONAL PEER REVIEW EFFICIENT FOR EVALUATION OF WORKING AND SCIENTIFIC HYPOTHESES?
Traditionally, publication after peer review has been considered the gold standard before any new idea finds acceptability amongst the scientific community. Getting a work (including a working or scientific hypothesis) reviewed by experts in the field before experiments are conducted to prove or disprove it helps to refine the idea further as well as improve the experiments planned to test the hypothesis. 40 A route towards this has been the emergence of journals dedicated to publishing hypotheses such as the Central Asian Journal of Medical Hypotheses and Ethics. 41 Another means of publishing hypotheses is through registered research protocols detailing the background, hypothesis, and methodology of a particular study. If such protocols are published after peer review, then the journal commits to publishing the completed study irrespective of whether the study hypothesis is proven or disproven. 42 In the post-pandemic world, online research methods such as online surveys powered via social media channels such as Twitter and Instagram might serve as critical tools to generate as well as to preliminarily test the appropriateness of hypotheses for further evaluation. 43 , 44
Some radical hypotheses might be difficult to publish after traditional peer review. These hypotheses might only be acceptable by the scientific community after they are tested in research studies. Preprints might be a way to disseminate such controversial and ground-breaking hypotheses. 45 However, scientists might prefer to keep their hypotheses confidential for the fear of plagiarism of ideas, avoiding online posting and publishing until they have tested the hypotheses.
SUGGESTIONS ON GENERATING AND PUBLISHING HYPOTHESES
Publication of hypotheses is important, however, a balance is required between scientific temper and controversy. Journal editors and reviewers might keep in mind these specific points, summarized in Table 2 and detailed hereafter, while judging the merit of hypotheses for publication. Keeping in mind the ethical principle of primum non nocere, a hypothesis should be published only if it is testable in a manner that is ethically appropriate. 46 Such hypotheses should be grounded in reality and lend themselves to further testing to either prove or disprove them. It must be considered that subsequent experiments to prove or disprove a hypothesis have an equal chance of failing or succeeding, akin to tossing a coin. A pre-conceived belief that a hypothesis is unlikely to be proven correct should not form the basis of rejection of such a hypothesis for publication. In this context, hypotheses generated after a thorough literature search to identify knowledge gaps or based on concrete clinical observations on a considerable number of patients (as opposed to random observations on a few patients) are more likely to be acceptable for publication by peer-reviewed journals. Also, hypotheses should be considered for publication or rejection based on their implications for science at large rather than whether the subsequent experiments to test them end up with results in favour of or against the original hypothesis.
Hypotheses form an important part of the scientific literature. The COVID-19 pandemic has reiterated the importance and relevance of hypotheses for dealing with public health emergencies and highlighted the need for evidence-based and ethical hypotheses. A good hypothesis is testable in a relevant study design, backed by preliminary evidence, and has positive ethical and clinical implications. General medical journals might consider publishing hypotheses as a specific article type to enable more rapid advancement of science.
Disclosure: The authors have no potential conflicts of interest to disclose.
Author Contributions:
- Data curation: Gasparyan AY, Misra DP, Zimba O, Yessirkepov M, Agarwal V, Kitas GD.
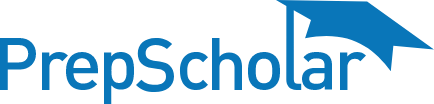
Choose Your Test
Sat / act prep online guides and tips, what is a hypothesis and how do i write one.
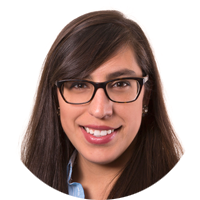
General Education
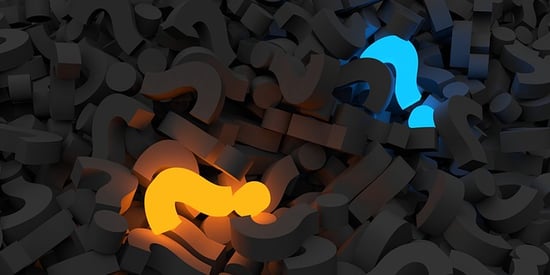
Think about something strange and unexplainable in your life. Maybe you get a headache right before it rains, or maybe you think your favorite sports team wins when you wear a certain color. If you wanted to see whether these are just coincidences or scientific fact, you would form a hypothesis, then create an experiment to see whether that hypothesis is true or not.
But what is a hypothesis, anyway? If you’re not sure about what a hypothesis is--or how to test for one!--you’re in the right place. This article will teach you everything you need to know about hypotheses, including:
- Defining the term “hypothesis”
- Providing hypothesis examples
- Giving you tips for how to write your own hypothesis
So let’s get started!
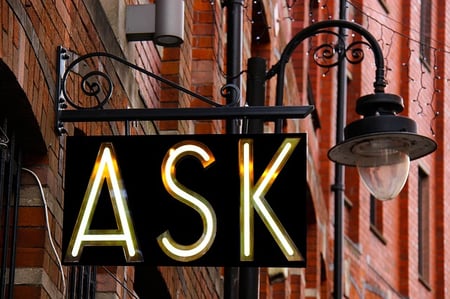
What Is a Hypothesis?
Merriam Webster defines a hypothesis as “an assumption or concession made for the sake of argument.” In other words, a hypothesis is an educated guess . Scientists make a reasonable assumption--or a hypothesis--then design an experiment to test whether it’s true or not. Keep in mind that in science, a hypothesis should be testable. You have to be able to design an experiment that tests your hypothesis in order for it to be valid.
As you could assume from that statement, it’s easy to make a bad hypothesis. But when you’re holding an experiment, it’s even more important that your guesses be good...after all, you’re spending time (and maybe money!) to figure out more about your observation. That’s why we refer to a hypothesis as an educated guess--good hypotheses are based on existing data and research to make them as sound as possible.
Hypotheses are one part of what’s called the scientific method . Every (good) experiment or study is based in the scientific method. The scientific method gives order and structure to experiments and ensures that interference from scientists or outside influences does not skew the results. It’s important that you understand the concepts of the scientific method before holding your own experiment. Though it may vary among scientists, the scientific method is generally made up of six steps (in order):
- Observation
- Asking questions
- Forming a hypothesis
- Analyze the data
- Communicate your results
You’ll notice that the hypothesis comes pretty early on when conducting an experiment. That’s because experiments work best when they’re trying to answer one specific question. And you can’t conduct an experiment until you know what you’re trying to prove!
Independent and Dependent Variables
After doing your research, you’re ready for another important step in forming your hypothesis: identifying variables. Variables are basically any factor that could influence the outcome of your experiment . Variables have to be measurable and related to the topic being studied.
There are two types of variables: independent variables and dependent variables. I ndependent variables remain constant . For example, age is an independent variable; it will stay the same, and researchers can look at different ages to see if it has an effect on the dependent variable.
Speaking of dependent variables... dependent variables are subject to the influence of the independent variable , meaning that they are not constant. Let’s say you want to test whether a person’s age affects how much sleep they need. In that case, the independent variable is age (like we mentioned above), and the dependent variable is how much sleep a person gets.
Variables will be crucial in writing your hypothesis. You need to be able to identify which variable is which, as both the independent and dependent variables will be written into your hypothesis. For instance, in a study about exercise, the independent variable might be the speed at which the respondents walk for thirty minutes, and the dependent variable would be their heart rate. In your study and in your hypothesis, you’re trying to understand the relationship between the two variables.
Elements of a Good Hypothesis
The best hypotheses start by asking the right questions . For instance, if you’ve observed that the grass is greener when it rains twice a week, you could ask what kind of grass it is, what elevation it’s at, and if the grass across the street responds to rain in the same way. Any of these questions could become the backbone of experiments to test why the grass gets greener when it rains fairly frequently.
As you’re asking more questions about your first observation, make sure you’re also making more observations . If it doesn’t rain for two weeks and the grass still looks green, that’s an important observation that could influence your hypothesis. You'll continue observing all throughout your experiment, but until the hypothesis is finalized, every observation should be noted.
Finally, you should consult secondary research before writing your hypothesis . Secondary research is comprised of results found and published by other people. You can usually find this information online or at your library. Additionally, m ake sure the research you find is credible and related to your topic. If you’re studying the correlation between rain and grass growth, it would help you to research rain patterns over the past twenty years for your county, published by a local agricultural association. You should also research the types of grass common in your area, the type of grass in your lawn, and whether anyone else has conducted experiments about your hypothesis. Also be sure you’re checking the quality of your research . Research done by a middle school student about what minerals can be found in rainwater would be less useful than an article published by a local university.
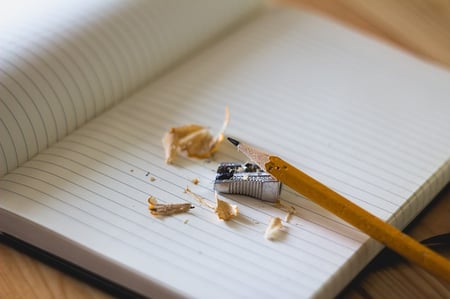
Writing Your Hypothesis
Once you’ve considered all of the factors above, you’re ready to start writing your hypothesis. Hypotheses usually take a certain form when they’re written out in a research report.
When you boil down your hypothesis statement, you are writing down your best guess and not the question at hand . This means that your statement should be written as if it is fact already, even though you are simply testing it.
The reason for this is that, after you have completed your study, you'll either accept or reject your if-then or your null hypothesis. All hypothesis testing examples should be measurable and able to be confirmed or denied. You cannot confirm a question, only a statement!
In fact, you come up with hypothesis examples all the time! For instance, when you guess on the outcome of a basketball game, you don’t say, “Will the Miami Heat beat the Boston Celtics?” but instead, “I think the Miami Heat will beat the Boston Celtics.” You state it as if it is already true, even if it turns out you’re wrong. You do the same thing when writing your hypothesis.
Additionally, keep in mind that hypotheses can range from very specific to very broad. These hypotheses can be specific, but if your hypothesis testing examples involve a broad range of causes and effects, your hypothesis can also be broad.
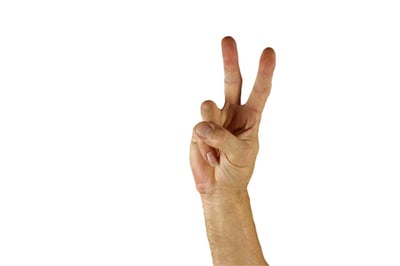
The Two Types of Hypotheses
Now that you understand what goes into a hypothesis, it’s time to look more closely at the two most common types of hypothesis: the if-then hypothesis and the null hypothesis.
#1: If-Then Hypotheses
First of all, if-then hypotheses typically follow this formula:
If ____ happens, then ____ will happen.
The goal of this type of hypothesis is to test the causal relationship between the independent and dependent variable. It’s fairly simple, and each hypothesis can vary in how detailed it can be. We create if-then hypotheses all the time with our daily predictions. Here are some examples of hypotheses that use an if-then structure from daily life:
- If I get enough sleep, I’ll be able to get more work done tomorrow.
- If the bus is on time, I can make it to my friend’s birthday party.
- If I study every night this week, I’ll get a better grade on my exam.
In each of these situations, you’re making a guess on how an independent variable (sleep, time, or studying) will affect a dependent variable (the amount of work you can do, making it to a party on time, or getting better grades).
You may still be asking, “What is an example of a hypothesis used in scientific research?” Take one of the hypothesis examples from a real-world study on whether using technology before bed affects children’s sleep patterns. The hypothesis read s:
“We hypothesized that increased hours of tablet- and phone-based screen time at bedtime would be inversely correlated with sleep quality and child attention.”
It might not look like it, but this is an if-then statement. The researchers basically said, “If children have more screen usage at bedtime, then their quality of sleep and attention will be worse.” The sleep quality and attention are the dependent variables and the screen usage is the independent variable. (Usually, the independent variable comes after the “if” and the dependent variable comes after the “then,” as it is the independent variable that affects the dependent variable.) This is an excellent example of how flexible hypothesis statements can be, as long as the general idea of “if-then” and the independent and dependent variables are present.
#2: Null Hypotheses
Your if-then hypothesis is not the only one needed to complete a successful experiment, however. You also need a null hypothesis to test it against. In its most basic form, the null hypothesis is the opposite of your if-then hypothesis . When you write your null hypothesis, you are writing a hypothesis that suggests that your guess is not true, and that the independent and dependent variables have no relationship .
One null hypothesis for the cell phone and sleep study from the last section might say:
“If children have more screen usage at bedtime, their quality of sleep and attention will not be worse.”
In this case, this is a null hypothesis because it’s asking the opposite of the original thesis!
Conversely, if your if-then hypothesis suggests that your two variables have no relationship, then your null hypothesis would suggest that there is one. So, pretend that there is a study that is asking the question, “Does the amount of followers on Instagram influence how long people spend on the app?” The independent variable is the amount of followers, and the dependent variable is the time spent. But if you, as the researcher, don’t think there is a relationship between the number of followers and time spent, you might write an if-then hypothesis that reads:
“If people have many followers on Instagram, they will not spend more time on the app than people who have less.”
In this case, the if-then suggests there isn’t a relationship between the variables. In that case, one of the null hypothesis examples might say:
“If people have many followers on Instagram, they will spend more time on the app than people who have less.”
You then test both the if-then and the null hypothesis to gauge if there is a relationship between the variables, and if so, how much of a relationship.
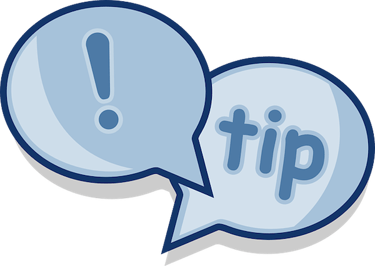
4 Tips to Write the Best Hypothesis
If you’re going to take the time to hold an experiment, whether in school or by yourself, you’re also going to want to take the time to make sure your hypothesis is a good one. The best hypotheses have four major elements in common: plausibility, defined concepts, observability, and general explanation.
#1: Plausibility
At first glance, this quality of a hypothesis might seem obvious. When your hypothesis is plausible, that means it’s possible given what we know about science and general common sense. However, improbable hypotheses are more common than you might think.
Imagine you’re studying weight gain and television watching habits. If you hypothesize that people who watch more than twenty hours of television a week will gain two hundred pounds or more over the course of a year, this might be improbable (though it’s potentially possible). Consequently, c ommon sense can tell us the results of the study before the study even begins.
Improbable hypotheses generally go against science, as well. Take this hypothesis example:
“If a person smokes one cigarette a day, then they will have lungs just as healthy as the average person’s.”
This hypothesis is obviously untrue, as studies have shown again and again that cigarettes negatively affect lung health. You must be careful that your hypotheses do not reflect your own personal opinion more than they do scientifically-supported findings. This plausibility points to the necessity of research before the hypothesis is written to make sure that your hypothesis has not already been disproven.
#2: Defined Concepts
The more advanced you are in your studies, the more likely that the terms you’re using in your hypothesis are specific to a limited set of knowledge. One of the hypothesis testing examples might include the readability of printed text in newspapers, where you might use words like “kerning” and “x-height.” Unless your readers have a background in graphic design, it’s likely that they won’t know what you mean by these terms. Thus, it’s important to either write what they mean in the hypothesis itself or in the report before the hypothesis.
Here’s what we mean. Which of the following sentences makes more sense to the common person?
If the kerning is greater than average, more words will be read per minute.
If the space between letters is greater than average, more words will be read per minute.
For people reading your report that are not experts in typography, simply adding a few more words will be helpful in clarifying exactly what the experiment is all about. It’s always a good idea to make your research and findings as accessible as possible.
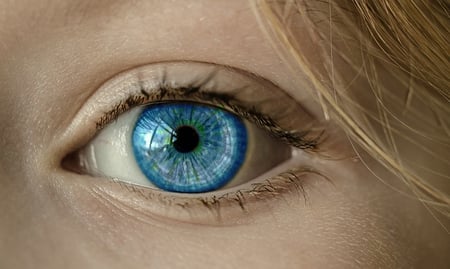
Good hypotheses ensure that you can observe the results.
#3: Observability
In order to measure the truth or falsity of your hypothesis, you must be able to see your variables and the way they interact. For instance, if your hypothesis is that the flight patterns of satellites affect the strength of certain television signals, yet you don’t have a telescope to view the satellites or a television to monitor the signal strength, you cannot properly observe your hypothesis and thus cannot continue your study.
Some variables may seem easy to observe, but if you do not have a system of measurement in place, you cannot observe your hypothesis properly. Here’s an example: if you’re experimenting on the effect of healthy food on overall happiness, but you don’t have a way to monitor and measure what “overall happiness” means, your results will not reflect the truth. Monitoring how often someone smiles for a whole day is not reasonably observable, but having the participants state how happy they feel on a scale of one to ten is more observable.
In writing your hypothesis, always keep in mind how you'll execute the experiment.
#4: Generalizability
Perhaps you’d like to study what color your best friend wears the most often by observing and documenting the colors she wears each day of the week. This might be fun information for her and you to know, but beyond you two, there aren’t many people who could benefit from this experiment. When you start an experiment, you should note how generalizable your findings may be if they are confirmed. Generalizability is basically how common a particular phenomenon is to other people’s everyday life.
Let’s say you’re asking a question about the health benefits of eating an apple for one day only, you need to realize that the experiment may be too specific to be helpful. It does not help to explain a phenomenon that many people experience. If you find yourself with too specific of a hypothesis, go back to asking the big question: what is it that you want to know, and what do you think will happen between your two variables?
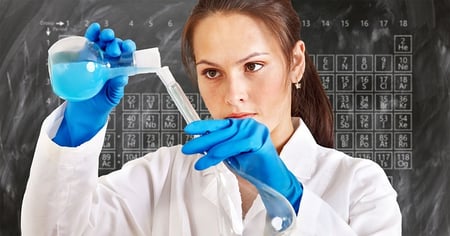
Hypothesis Testing Examples
We know it can be hard to write a good hypothesis unless you’ve seen some good hypothesis examples. We’ve included four hypothesis examples based on some made-up experiments. Use these as templates or launch pads for coming up with your own hypotheses.
Experiment #1: Students Studying Outside (Writing a Hypothesis)
You are a student at PrepScholar University. When you walk around campus, you notice that, when the temperature is above 60 degrees, more students study in the quad. You want to know when your fellow students are more likely to study outside. With this information, how do you make the best hypothesis possible?
You must remember to make additional observations and do secondary research before writing your hypothesis. In doing so, you notice that no one studies outside when it’s 75 degrees and raining, so this should be included in your experiment. Also, studies done on the topic beforehand suggested that students are more likely to study in temperatures less than 85 degrees. With this in mind, you feel confident that you can identify your variables and write your hypotheses:
If-then: “If the temperature in Fahrenheit is less than 60 degrees, significantly fewer students will study outside.”
Null: “If the temperature in Fahrenheit is less than 60 degrees, the same number of students will study outside as when it is more than 60 degrees.”
These hypotheses are plausible, as the temperatures are reasonably within the bounds of what is possible. The number of people in the quad is also easily observable. It is also not a phenomenon specific to only one person or at one time, but instead can explain a phenomenon for a broader group of people.
To complete this experiment, you pick the month of October to observe the quad. Every day (except on the days where it’s raining)from 3 to 4 PM, when most classes have released for the day, you observe how many people are on the quad. You measure how many people come and how many leave. You also write down the temperature on the hour.
After writing down all of your observations and putting them on a graph, you find that the most students study on the quad when it is 70 degrees outside, and that the number of students drops a lot once the temperature reaches 60 degrees or below. In this case, your research report would state that you accept or “failed to reject” your first hypothesis with your findings.
Experiment #2: The Cupcake Store (Forming a Simple Experiment)
Let’s say that you work at a bakery. You specialize in cupcakes, and you make only two colors of frosting: yellow and purple. You want to know what kind of customers are more likely to buy what kind of cupcake, so you set up an experiment. Your independent variable is the customer’s gender, and the dependent variable is the color of the frosting. What is an example of a hypothesis that might answer the question of this study?
Here’s what your hypotheses might look like:
If-then: “If customers’ gender is female, then they will buy more yellow cupcakes than purple cupcakes.”
Null: “If customers’ gender is female, then they will be just as likely to buy purple cupcakes as yellow cupcakes.”
This is a pretty simple experiment! It passes the test of plausibility (there could easily be a difference), defined concepts (there’s nothing complicated about cupcakes!), observability (both color and gender can be easily observed), and general explanation ( this would potentially help you make better business decisions ).
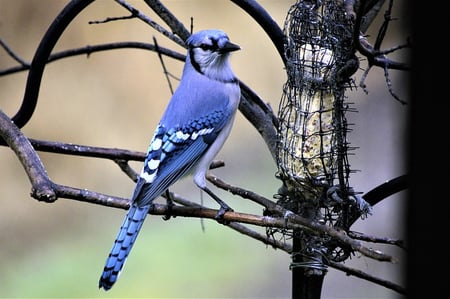
Experiment #3: Backyard Bird Feeders (Integrating Multiple Variables and Rejecting the If-Then Hypothesis)
While watching your backyard bird feeder, you realized that different birds come on the days when you change the types of seeds. You decide that you want to see more cardinals in your backyard, so you decide to see what type of food they like the best and set up an experiment.
However, one morning, you notice that, while some cardinals are present, blue jays are eating out of your backyard feeder filled with millet. You decide that, of all of the other birds, you would like to see the blue jays the least. This means you'll have more than one variable in your hypothesis. Your new hypotheses might look like this:
If-then: “If sunflower seeds are placed in the bird feeders, then more cardinals will come than blue jays. If millet is placed in the bird feeders, then more blue jays will come than cardinals.”
Null: “If either sunflower seeds or millet are placed in the bird, equal numbers of cardinals and blue jays will come.”
Through simple observation, you actually find that cardinals come as often as blue jays when sunflower seeds or millet is in the bird feeder. In this case, you would reject your “if-then” hypothesis and “fail to reject” your null hypothesis . You cannot accept your first hypothesis, because it’s clearly not true. Instead you found that there was actually no relation between your different variables. Consequently, you would need to run more experiments with different variables to see if the new variables impact the results.
Experiment #4: In-Class Survey (Including an Alternative Hypothesis)
You’re about to give a speech in one of your classes about the importance of paying attention. You want to take this opportunity to test a hypothesis you’ve had for a while:
If-then: If students sit in the first two rows of the classroom, then they will listen better than students who do not.
Null: If students sit in the first two rows of the classroom, then they will not listen better or worse than students who do not.
You give your speech and then ask your teacher if you can hand out a short survey to the class. On the survey, you’ve included questions about some of the topics you talked about. When you get back the results, you’re surprised to see that not only do the students in the first two rows not pay better attention, but they also scored worse than students in other parts of the classroom! Here, both your if-then and your null hypotheses are not representative of your findings. What do you do?
This is when you reject both your if-then and null hypotheses and instead create an alternative hypothesis . This type of hypothesis is used in the rare circumstance that neither of your hypotheses is able to capture your findings . Now you can use what you’ve learned to draft new hypotheses and test again!
Key Takeaways: Hypothesis Writing
The more comfortable you become with writing hypotheses, the better they will become. The structure of hypotheses is flexible and may need to be changed depending on what topic you are studying. The most important thing to remember is the purpose of your hypothesis and the difference between the if-then and the null . From there, in forming your hypothesis, you should constantly be asking questions, making observations, doing secondary research, and considering your variables. After you have written your hypothesis, be sure to edit it so that it is plausible, clearly defined, observable, and helpful in explaining a general phenomenon.
Writing a hypothesis is something that everyone, from elementary school children competing in a science fair to professional scientists in a lab, needs to know how to do. Hypotheses are vital in experiments and in properly executing the scientific method . When done correctly, hypotheses will set up your studies for success and help you to understand the world a little better, one experiment at a time.
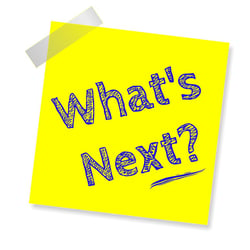
What’s Next?
If you’re studying for the science portion of the ACT, there’s definitely a lot you need to know. We’ve got the tools to help, though! Start by checking out our ultimate study guide for the ACT Science subject test. Once you read through that, be sure to download our recommended ACT Science practice tests , since they’re one of the most foolproof ways to improve your score. (And don’t forget to check out our expert guide book , too.)
If you love science and want to major in a scientific field, you should start preparing in high school . Here are the science classes you should take to set yourself up for success.
If you’re trying to think of science experiments you can do for class (or for a science fair!), here’s a list of 37 awesome science experiments you can do at home
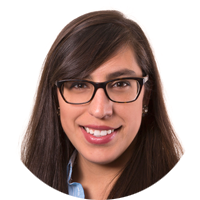
Ashley Sufflé Robinson has a Ph.D. in 19th Century English Literature. As a content writer for PrepScholar, Ashley is passionate about giving college-bound students the in-depth information they need to get into the school of their dreams.
Ask a Question Below
Have any questions about this article or other topics? Ask below and we'll reply!
Improve With Our Famous Guides
- For All Students
The 5 Strategies You Must Be Using to Improve 160+ SAT Points
How to Get a Perfect 1600, by a Perfect Scorer
Series: How to Get 800 on Each SAT Section:
Score 800 on SAT Math
Score 800 on SAT Reading
Score 800 on SAT Writing
Series: How to Get to 600 on Each SAT Section:
Score 600 on SAT Math
Score 600 on SAT Reading
Score 600 on SAT Writing
Free Complete Official SAT Practice Tests
What SAT Target Score Should You Be Aiming For?
15 Strategies to Improve Your SAT Essay
The 5 Strategies You Must Be Using to Improve 4+ ACT Points
How to Get a Perfect 36 ACT, by a Perfect Scorer
Series: How to Get 36 on Each ACT Section:
36 on ACT English
36 on ACT Math
36 on ACT Reading
36 on ACT Science
Series: How to Get to 24 on Each ACT Section:
24 on ACT English
24 on ACT Math
24 on ACT Reading
24 on ACT Science
What ACT target score should you be aiming for?
ACT Vocabulary You Must Know
ACT Writing: 15 Tips to Raise Your Essay Score
How to Get Into Harvard and the Ivy League
How to Get a Perfect 4.0 GPA
How to Write an Amazing College Essay
What Exactly Are Colleges Looking For?
Is the ACT easier than the SAT? A Comprehensive Guide
Should you retake your SAT or ACT?
When should you take the SAT or ACT?
Stay Informed

Get the latest articles and test prep tips!
Looking for Graduate School Test Prep?
Check out our top-rated graduate blogs here:
GRE Online Prep Blog
GMAT Online Prep Blog
TOEFL Online Prep Blog
Holly R. "I am absolutely overjoyed and cannot thank you enough for helping me!”
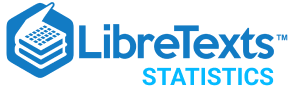
- school Campus Bookshelves
- menu_book Bookshelves
- perm_media Learning Objects
- login Login
- how_to_reg Request Instructor Account
- hub Instructor Commons
Margin Size
- Download Page (PDF)
- Download Full Book (PDF)
- Periodic Table
- Physics Constants
- Scientific Calculator
- Reference & Cite
- Tools expand_more
- Readability
selected template will load here
This action is not available.
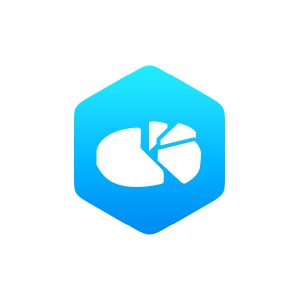
9.1: Introduction to Hypothesis Testing
- Last updated
- Save as PDF
- Page ID 10211
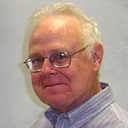
- Kyle Siegrist
- University of Alabama in Huntsville via Random Services
\( \newcommand{\vecs}[1]{\overset { \scriptstyle \rightharpoonup} {\mathbf{#1}} } \)
\( \newcommand{\vecd}[1]{\overset{-\!-\!\rightharpoonup}{\vphantom{a}\smash {#1}}} \)
\( \newcommand{\id}{\mathrm{id}}\) \( \newcommand{\Span}{\mathrm{span}}\)
( \newcommand{\kernel}{\mathrm{null}\,}\) \( \newcommand{\range}{\mathrm{range}\,}\)
\( \newcommand{\RealPart}{\mathrm{Re}}\) \( \newcommand{\ImaginaryPart}{\mathrm{Im}}\)
\( \newcommand{\Argument}{\mathrm{Arg}}\) \( \newcommand{\norm}[1]{\| #1 \|}\)
\( \newcommand{\inner}[2]{\langle #1, #2 \rangle}\)
\( \newcommand{\Span}{\mathrm{span}}\)
\( \newcommand{\id}{\mathrm{id}}\)
\( \newcommand{\kernel}{\mathrm{null}\,}\)
\( \newcommand{\range}{\mathrm{range}\,}\)
\( \newcommand{\RealPart}{\mathrm{Re}}\)
\( \newcommand{\ImaginaryPart}{\mathrm{Im}}\)
\( \newcommand{\Argument}{\mathrm{Arg}}\)
\( \newcommand{\norm}[1]{\| #1 \|}\)
\( \newcommand{\Span}{\mathrm{span}}\) \( \newcommand{\AA}{\unicode[.8,0]{x212B}}\)
\( \newcommand{\vectorA}[1]{\vec{#1}} % arrow\)
\( \newcommand{\vectorAt}[1]{\vec{\text{#1}}} % arrow\)
\( \newcommand{\vectorB}[1]{\overset { \scriptstyle \rightharpoonup} {\mathbf{#1}} } \)
\( \newcommand{\vectorC}[1]{\textbf{#1}} \)
\( \newcommand{\vectorD}[1]{\overrightarrow{#1}} \)
\( \newcommand{\vectorDt}[1]{\overrightarrow{\text{#1}}} \)
\( \newcommand{\vectE}[1]{\overset{-\!-\!\rightharpoonup}{\vphantom{a}\smash{\mathbf {#1}}}} \)
Basic Theory
Preliminaries.
As usual, our starting point is a random experiment with an underlying sample space and a probability measure \(\P\). In the basic statistical model, we have an observable random variable \(\bs{X}\) taking values in a set \(S\). In general, \(\bs{X}\) can have quite a complicated structure. For example, if the experiment is to sample \(n\) objects from a population and record various measurements of interest, then \[ \bs{X} = (X_1, X_2, \ldots, X_n) \] where \(X_i\) is the vector of measurements for the \(i\)th object. The most important special case occurs when \((X_1, X_2, \ldots, X_n)\) are independent and identically distributed. In this case, we have a random sample of size \(n\) from the common distribution.
The purpose of this section is to define and discuss the basic concepts of statistical hypothesis testing . Collectively, these concepts are sometimes referred to as the Neyman-Pearson framework, in honor of Jerzy Neyman and Egon Pearson, who first formalized them.
A statistical hypothesis is a statement about the distribution of \(\bs{X}\). Equivalently, a statistical hypothesis specifies a set of possible distributions of \(\bs{X}\): the set of distributions for which the statement is true. A hypothesis that specifies a single distribution for \(\bs{X}\) is called simple ; a hypothesis that specifies more than one distribution for \(\bs{X}\) is called composite .
In hypothesis testing , the goal is to see if there is sufficient statistical evidence to reject a presumed null hypothesis in favor of a conjectured alternative hypothesis . The null hypothesis is usually denoted \(H_0\) while the alternative hypothesis is usually denoted \(H_1\).
An hypothesis test is a statistical decision ; the conclusion will either be to reject the null hypothesis in favor of the alternative, or to fail to reject the null hypothesis. The decision that we make must, of course, be based on the observed value \(\bs{x}\) of the data vector \(\bs{X}\). Thus, we will find an appropriate subset \(R\) of the sample space \(S\) and reject \(H_0\) if and only if \(\bs{x} \in R\). The set \(R\) is known as the rejection region or the critical region . Note the asymmetry between the null and alternative hypotheses. This asymmetry is due to the fact that we assume the null hypothesis, in a sense, and then see if there is sufficient evidence in \(\bs{x}\) to overturn this assumption in favor of the alternative.
An hypothesis test is a statistical analogy to proof by contradiction, in a sense. Suppose for a moment that \(H_1\) is a statement in a mathematical theory and that \(H_0\) is its negation. One way that we can prove \(H_1\) is to assume \(H_0\) and work our way logically to a contradiction. In an hypothesis test, we don't prove anything of course, but there are similarities. We assume \(H_0\) and then see if the data \(\bs{x}\) are sufficiently at odds with that assumption that we feel justified in rejecting \(H_0\) in favor of \(H_1\).
Often, the critical region is defined in terms of a statistic \(w(\bs{X})\), known as a test statistic , where \(w\) is a function from \(S\) into another set \(T\). We find an appropriate rejection region \(R_T \subseteq T\) and reject \(H_0\) when the observed value \(w(\bs{x}) \in R_T\). Thus, the rejection region in \(S\) is then \(R = w^{-1}(R_T) = \left\{\bs{x} \in S: w(\bs{x}) \in R_T\right\}\). As usual, the use of a statistic often allows significant data reduction when the dimension of the test statistic is much smaller than the dimension of the data vector.
The ultimate decision may be correct or may be in error. There are two types of errors, depending on which of the hypotheses is actually true.
Types of errors:
- A type 1 error is rejecting the null hypothesis \(H_0\) when \(H_0\) is true.
- A type 2 error is failing to reject the null hypothesis \(H_0\) when the alternative hypothesis \(H_1\) is true.
Similarly, there are two ways to make a correct decision: we could reject \(H_0\) when \(H_1\) is true or we could fail to reject \(H_0\) when \(H_0\) is true. The possibilities are summarized in the following table:
Of course, when we observe \(\bs{X} = \bs{x}\) and make our decision, either we will have made the correct decision or we will have committed an error, and usually we will never know which of these events has occurred. Prior to gathering the data, however, we can consider the probabilities of the various errors.
If \(H_0\) is true (that is, the distribution of \(\bs{X}\) is specified by \(H_0\)), then \(\P(\bs{X} \in R)\) is the probability of a type 1 error for this distribution. If \(H_0\) is composite, then \(H_0\) specifies a variety of different distributions for \(\bs{X}\) and thus there is a set of type 1 error probabilities.
The maximum probability of a type 1 error, over the set of distributions specified by \( H_0 \), is the significance level of the test or the size of the critical region.
The significance level is often denoted by \(\alpha\). Usually, the rejection region is constructed so that the significance level is a prescribed, small value (typically 0.1, 0.05, 0.01).
If \(H_1\) is true (that is, the distribution of \(\bs{X}\) is specified by \(H_1\)), then \(\P(\bs{X} \notin R)\) is the probability of a type 2 error for this distribution. Again, if \(H_1\) is composite then \(H_1\) specifies a variety of different distributions for \(\bs{X}\), and thus there will be a set of type 2 error probabilities. Generally, there is a tradeoff between the type 1 and type 2 error probabilities. If we reduce the probability of a type 1 error, by making the rejection region \(R\) smaller, we necessarily increase the probability of a type 2 error because the complementary region \(S \setminus R\) is larger.
The extreme cases can give us some insight. First consider the decision rule in which we never reject \(H_0\), regardless of the evidence \(\bs{x}\). This corresponds to the rejection region \(R = \emptyset\). A type 1 error is impossible, so the significance level is 0. On the other hand, the probability of a type 2 error is 1 for any distribution defined by \(H_1\). At the other extreme, consider the decision rule in which we always rejects \(H_0\) regardless of the evidence \(\bs{x}\). This corresponds to the rejection region \(R = S\). A type 2 error is impossible, but now the probability of a type 1 error is 1 for any distribution defined by \(H_0\). In between these two worthless tests are meaningful tests that take the evidence \(\bs{x}\) into account.
If \(H_1\) is true, so that the distribution of \(\bs{X}\) is specified by \(H_1\), then \(\P(\bs{X} \in R)\), the probability of rejecting \(H_0\) is the power of the test for that distribution.
Thus the power of the test for a distribution specified by \( H_1 \) is the probability of making the correct decision.
Suppose that we have two tests, corresponding to rejection regions \(R_1\) and \(R_2\), respectively, each having significance level \(\alpha\). The test with region \(R_1\) is uniformly more powerful than the test with region \(R_2\) if \[ \P(\bs{X} \in R_1) \ge \P(\bs{X} \in R_2) \text{ for every distribution of } \bs{X} \text{ specified by } H_1 \]
Naturally, in this case, we would prefer the first test. Often, however, two tests will not be uniformly ordered; one test will be more powerful for some distributions specified by \(H_1\) while the other test will be more powerful for other distributions specified by \(H_1\).
If a test has significance level \(\alpha\) and is uniformly more powerful than any other test with significance level \(\alpha\), then the test is said to be a uniformly most powerful test at level \(\alpha\).
Clearly a uniformly most powerful test is the best we can do.
\(P\)-value
In most cases, we have a general procedure that allows us to construct a test (that is, a rejection region \(R_\alpha\)) for any given significance level \(\alpha \in (0, 1)\). Typically, \(R_\alpha\) decreases (in the subset sense) as \(\alpha\) decreases.
The \(P\)-value of the observed value \(\bs{x}\) of \(\bs{X}\), denoted \(P(\bs{x})\), is defined to be the smallest \(\alpha\) for which \(\bs{x} \in R_\alpha\); that is, the smallest significance level for which \(H_0\) is rejected, given \(\bs{X} = \bs{x}\).
Knowing \(P(\bs{x})\) allows us to test \(H_0\) at any significance level for the given data \(\bs{x}\): If \(P(\bs{x}) \le \alpha\) then we would reject \(H_0\) at significance level \(\alpha\); if \(P(\bs{x}) \gt \alpha\) then we fail to reject \(H_0\) at significance level \(\alpha\). Note that \(P(\bs{X})\) is a statistic . Informally, \(P(\bs{x})\) can often be thought of as the probability of an outcome as or more extreme than the observed value \(\bs{x}\), where extreme is interpreted relative to the null hypothesis \(H_0\).
Analogy with Justice Systems
There is a helpful analogy between statistical hypothesis testing and the criminal justice system in the US and various other countries. Consider a person charged with a crime. The presumed null hypothesis is that the person is innocent of the crime; the conjectured alternative hypothesis is that the person is guilty of the crime. The test of the hypotheses is a trial with evidence presented by both sides playing the role of the data. After considering the evidence, the jury delivers the decision as either not guilty or guilty . Note that innocent is not a possible verdict of the jury, because it is not the point of the trial to prove the person innocent. Rather, the point of the trial is to see whether there is sufficient evidence to overturn the null hypothesis that the person is innocent in favor of the alternative hypothesis of that the person is guilty. A type 1 error is convicting a person who is innocent; a type 2 error is acquitting a person who is guilty. Generally, a type 1 error is considered the more serious of the two possible errors, so in an attempt to hold the chance of a type 1 error to a very low level, the standard for conviction in serious criminal cases is beyond a reasonable doubt .
Tests of an Unknown Parameter
Hypothesis testing is a very general concept, but an important special class occurs when the distribution of the data variable \(\bs{X}\) depends on a parameter \(\theta\) taking values in a parameter space \(\Theta\). The parameter may be vector-valued, so that \(\bs{\theta} = (\theta_1, \theta_2, \ldots, \theta_n)\) and \(\Theta \subseteq \R^k\) for some \(k \in \N_+\). The hypotheses generally take the form \[ H_0: \theta \in \Theta_0 \text{ versus } H_1: \theta \notin \Theta_0 \] where \(\Theta_0\) is a prescribed subset of the parameter space \(\Theta\). In this setting, the probabilities of making an error or a correct decision depend on the true value of \(\theta\). If \(R\) is the rejection region, then the power function \( Q \) is given by \[ Q(\theta) = \P_\theta(\bs{X} \in R), \quad \theta \in \Theta \] The power function gives a lot of information about the test.
The power function satisfies the following properties:
- \(Q(\theta)\) is the probability of a type 1 error when \(\theta \in \Theta_0\).
- \(\max\left\{Q(\theta): \theta \in \Theta_0\right\}\) is the significance level of the test.
- \(1 - Q(\theta)\) is the probability of a type 2 error when \(\theta \notin \Theta_0\).
- \(Q(\theta)\) is the power of the test when \(\theta \notin \Theta_0\).
If we have two tests, we can compare them by means of their power functions.
Suppose that we have two tests, corresponding to rejection regions \(R_1\) and \(R_2\), respectively, each having significance level \(\alpha\). The test with rejection region \(R_1\) is uniformly more powerful than the test with rejection region \(R_2\) if \( Q_1(\theta) \ge Q_2(\theta)\) for all \( \theta \notin \Theta_0 \).
Most hypothesis tests of an unknown real parameter \(\theta\) fall into three special cases:
Suppose that \( \theta \) is a real parameter and \( \theta_0 \in \Theta \) a specified value. The tests below are respectively the two-sided test , the left-tailed test , and the right-tailed test .
- \(H_0: \theta = \theta_0\) versus \(H_1: \theta \ne \theta_0\)
- \(H_0: \theta \ge \theta_0\) versus \(H_1: \theta \lt \theta_0\)
- \(H_0: \theta \le \theta_0\) versus \(H_1: \theta \gt \theta_0\)
Thus the tests are named after the conjectured alternative. Of course, there may be other unknown parameters besides \(\theta\) (known as nuisance parameters ).
Equivalence Between Hypothesis Test and Confidence Sets
There is an equivalence between hypothesis tests and confidence sets for a parameter \(\theta\).
Suppose that \(C(\bs{x})\) is a \(1 - \alpha\) level confidence set for \(\theta\). The following test has significance level \(\alpha\) for the hypothesis \( H_0: \theta = \theta_0 \) versus \( H_1: \theta \ne \theta_0 \): Reject \(H_0\) if and only if \(\theta_0 \notin C(\bs{x})\)
By definition, \(\P[\theta \in C(\bs{X})] = 1 - \alpha\). Hence if \(H_0\) is true so that \(\theta = \theta_0\), then the probability of a type 1 error is \(P[\theta \notin C(\bs{X})] = \alpha\).
Equivalently, we fail to reject \(H_0\) at significance level \(\alpha\) if and only if \(\theta_0\) is in the corresponding \(1 - \alpha\) level confidence set. In particular, this equivalence applies to interval estimates of a real parameter \(\theta\) and the common tests for \(\theta\) given above .
In each case below, the confidence interval has confidence level \(1 - \alpha\) and the test has significance level \(\alpha\).
- Suppose that \(\left[L(\bs{X}, U(\bs{X})\right]\) is a two-sided confidence interval for \(\theta\). Reject \(H_0: \theta = \theta_0\) versus \(H_1: \theta \ne \theta_0\) if and only if \(\theta_0 \lt L(\bs{X})\) or \(\theta_0 \gt U(\bs{X})\).
- Suppose that \(L(\bs{X})\) is a confidence lower bound for \(\theta\). Reject \(H_0: \theta \le \theta_0\) versus \(H_1: \theta \gt \theta_0\) if and only if \(\theta_0 \lt L(\bs{X})\).
- Suppose that \(U(\bs{X})\) is a confidence upper bound for \(\theta\). Reject \(H_0: \theta \ge \theta_0\) versus \(H_1: \theta \lt \theta_0\) if and only if \(\theta_0 \gt U(\bs{X})\).
Pivot Variables and Test Statistics
Recall that confidence sets of an unknown parameter \(\theta\) are often constructed through a pivot variable , that is, a random variable \(W(\bs{X}, \theta)\) that depends on the data vector \(\bs{X}\) and the parameter \(\theta\), but whose distribution does not depend on \(\theta\) and is known. In this case, a natural test statistic for the basic tests given above is \(W(\bs{X}, \theta_0)\).

- Science Notes Posts
- Contact Science Notes
- Todd Helmenstine Biography
- Anne Helmenstine Biography
- Free Printable Periodic Tables (PDF and PNG)
- Periodic Table Wallpapers
- Interactive Periodic Table
- Periodic Table Posters
- How to Grow Crystals
- Chemistry Projects
- Fire and Flames Projects
- Holiday Science
- Chemistry Problems With Answers
- Physics Problems
- Unit Conversion Example Problems
- Chemistry Worksheets
- Biology Worksheets
- Periodic Table Worksheets
- Physical Science Worksheets
- Science Lab Worksheets
- My Amazon Books
Hypothesis Examples

A hypothesis is a prediction of the outcome of a test. It forms the basis for designing an experiment in the scientific method . A good hypothesis is testable, meaning it makes a prediction you can check with observation or experimentation. Here are different hypothesis examples.
Null Hypothesis Examples
The null hypothesis (H 0 ) is also known as the zero-difference or no-difference hypothesis. It predicts that changing one variable ( independent variable ) will have no effect on the variable being measured ( dependent variable ). Here are null hypothesis examples:
- Plant growth is unaffected by temperature.
- If you increase temperature, then solubility of salt will increase.
- Incidence of skin cancer is unrelated to ultraviolet light exposure.
- All brands of light bulb last equally long.
- Cats have no preference for the color of cat food.
- All daisies have the same number of petals.
Sometimes the null hypothesis shows there is a suspected correlation between two variables. For example, if you think plant growth is affected by temperature, you state the null hypothesis: “Plant growth is not affected by temperature.” Why do you do this, rather than say “If you change temperature, plant growth will be affected”? The answer is because it’s easier applying a statistical test that shows, with a high level of confidence, a null hypothesis is correct or incorrect.
Research Hypothesis Examples
A research hypothesis (H 1 ) is a type of hypothesis used to design an experiment. This type of hypothesis is often written as an if-then statement because it’s easy identifying the independent and dependent variables and seeing how one affects the other. If-then statements explore cause and effect. In other cases, the hypothesis shows a correlation between two variables. Here are some research hypothesis examples:
- If you leave the lights on, then it takes longer for people to fall asleep.
- If you refrigerate apples, they last longer before going bad.
- If you keep the curtains closed, then you need less electricity to heat or cool the house (the electric bill is lower).
- If you leave a bucket of water uncovered, then it evaporates more quickly.
- Goldfish lose their color if they are not exposed to light.
- Workers who take vacations are more productive than those who never take time off.
Is It Okay to Disprove a Hypothesis?
Yes! You may even choose to write your hypothesis in such a way that it can be disproved because it’s easier to prove a statement is wrong than to prove it is right. In other cases, if your prediction is incorrect, that doesn’t mean the science is bad. Revising a hypothesis is common. It demonstrates you learned something you did not know before you conducted the experiment.
Test yourself with a Scientific Method Quiz .
- Mellenbergh, G.J. (2008). Chapter 8: Research designs: Testing of research hypotheses. In H.J. Adèr & G.J. Mellenbergh (eds.), Advising on Research Methods: A Consultant’s Companion . Huizen, The Netherlands: Johannes van Kessel Publishing.
- Popper, Karl R. (1959). The Logic of Scientific Discovery . Hutchinson & Co. ISBN 3-1614-8410-X.
- Schick, Theodore; Vaughn, Lewis (2002). How to think about weird things: critical thinking for a New Age . Boston: McGraw-Hill Higher Education. ISBN 0-7674-2048-9.
- Tobi, Hilde; Kampen, Jarl K. (2018). “Research design: the methodology for interdisciplinary research framework”. Quality & Quantity . 52 (3): 1209–1225. doi: 10.1007/s11135-017-0513-8
Related Posts

Writing a Strong Hypothesis Statement
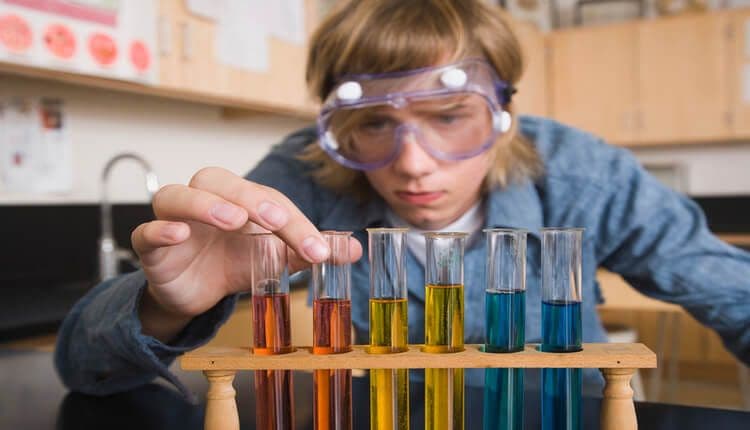
All good theses begins with a good thesis question. However, all great theses begins with a great hypothesis statement. One of the most important steps for writing a thesis is to create a strong hypothesis statement.
What is a hypothesis statement?
A hypothesis statement must be testable. If it cannot be tested, then there is no research to be done.
Simply put, a hypothesis statement posits the relationship between two or more variables. It is a prediction of what you think will happen in a research study. A hypothesis statement must be testable. If it cannot be tested, then there is no research to be done. If your thesis question is whether wildfires have effects on the weather, “wildfires create tornadoes” would be your hypothesis. However, a hypothesis needs to have several key elements in order to meet the criteria for a good hypothesis.
In this article, we will learn about what distinguishes a weak hypothesis from a strong one. We will also learn how to phrase your thesis question and frame your variables so that you are able to write a strong hypothesis statement and great thesis.
What is a hypothesis?
A hypothesis statement posits, or considers, a relationship between two variables.
As we mentioned above, a hypothesis statement posits or considers a relationship between two variables. In our hypothesis statement example above, the two variables are wildfires and tornadoes, and our assumed relationship between the two is a causal one (wildfires cause tornadoes). It is clear from our example above what we will be investigating: the relationship between wildfires and tornadoes.
A strong hypothesis statement should be:
- A prediction of the relationship between two or more variables
A hypothesis is not just a blind guess. It should build upon existing theories and knowledge . Tornadoes are often observed near wildfires once the fires reach a certain size. In addition, tornadoes are not a normal weather event in many areas; they have been spotted together with wildfires. This existing knowledge has informed the formulation of our hypothesis.
Depending on the thesis question, your research paper might have multiple hypothesis statements. What is important is that your hypothesis statement or statements are testable through data analysis, observation, experiments, or other methodologies.
Formulating your hypothesis
One of the best ways to form a hypothesis is to think about “if...then” statements.
Now that we know what a hypothesis statement is, let’s walk through how to formulate a strong one. First, you will need a thesis question. Your thesis question should be narrow in scope, answerable, and focused. Once you have your thesis question, it is time to start thinking about your hypothesis statement. You will need to clearly identify the variables involved before you can begin thinking about their relationship.
One of the best ways to form a hypothesis is to think about “if...then” statements . This can also help you easily identify the variables you are working with and refine your hypothesis statement. Let’s take a few examples.
If teenagers are given comprehensive sex education, there will be fewer teen pregnancies .
In this example, the independent variable is whether or not teenagers receive comprehensive sex education (the cause), and the dependent variable is the number of teen pregnancies (the effect).
If a cat is fed a vegan diet, it will die .
Here, our independent variable is the diet of the cat (the cause), and the dependent variable is the cat’s health (the thing impacted by the cause).
If children drink 8oz of milk per day, they will grow taller than children who do not drink any milk .
What are the variables in this hypothesis? If you identified drinking milk as the independent variable and growth as the dependent variable, you are correct. This is because we are guessing that drinking milk causes increased growth in the height of children.
Refining your hypothesis
Do not be afraid to refine your hypothesis throughout the process of formulation.
Do not be afraid to refine your hypothesis throughout the process of formulation. A strong hypothesis statement is clear, testable, and involves a prediction. While “testable” means verifiable or falsifiable, it also means that you are able to perform the necessary experiments without violating any ethical standards. Perhaps once you think about the ethics of possibly harming some cats by testing a vegan diet on them you might abandon the idea of that experiment altogether. However, if you think it is really important to research the relationship between a cat’s diet and a cat’s health, perhaps you could refine your hypothesis to something like this:
If 50% of a cat’s meals are vegan, the cat will not be able to meet its nutritional needs .
Another feature of a strong hypothesis statement is that it can easily be tested with the resources that you have readily available. While it might not be feasible to measure the growth of a cohort of children throughout their whole lives, you may be able to do so for a year. Then, you can adjust your hypothesis to something like this:
I f children aged 8 drink 8oz of milk per day for one year, they will grow taller during that year than children who do not drink any milk .
As you work to narrow down and refine your hypothesis to reflect a realistic potential research scope, don’t be afraid to talk to your supervisor about any concerns or questions you might have about what is truly possible to research.
What makes a hypothesis weak?
We noted above that a strong hypothesis statement is clear, is a prediction of a relationship between two or more variables, and is testable. We also clarified that statements, which are too general or specific are not strong hypotheses. We have looked at some examples of hypotheses that meet the criteria for a strong hypothesis, but before we go any further, let’s look at weak or bad hypothesis statement examples so that you can really see the difference.
Bad hypothesis 1: Diabetes is caused by witchcraft .
While this is fun to think about, it cannot be tested or proven one way or the other with clear evidence, data analysis, or experiments. This bad hypothesis fails to meet the testability requirement.
Bad hypothesis 2: If I change the amount of food I eat, my energy levels will change .
This is quite vague. Am I increasing or decreasing my food intake? What do I expect exactly will happen to my energy levels and why? How am I defining energy level? This bad hypothesis statement fails the clarity requirement.
Bad hypothesis 3: Japanese food is disgusting because Japanese people don’t like tourists .
This hypothesis is unclear about the posited relationship between variables. Are we positing the relationship between the deliciousness of Japanese food and the desire for tourists to visit? or the relationship between the deliciousness of Japanese food and the amount that Japanese people like tourists? There is also the problematic subjectivity of the assessment that Japanese food is “disgusting.” The problems are numerous.
The null hypothesis and the alternative hypothesis
The null hypothesis, quite simply, posits that there is no relationship between the variables.
What is the null hypothesis?
The hypothesis posits a relationship between two or more variables. The null hypothesis, quite simply, posits that there is no relationship between the variables. It is often indicated as H 0 , which is read as “h-oh” or “h-null.” The alternative hypothesis is the opposite of the null hypothesis as it posits that there is some relationship between the variables. The alternative hypothesis is written as H a or H 1 .
Let’s take our previous hypothesis statement examples discussed at the start and look at their corresponding null hypothesis.
H a : If teenagers are given comprehensive sex education, there will be fewer teen pregnancies .
H 0 : If teenagers are given comprehensive sex education, there will be no change in the number of teen pregnancies .
The null hypothesis assumes that comprehensive sex education will not affect how many teenagers get pregnant. It should be carefully noted that the null hypothesis is not always the opposite of the alternative hypothesis. For example:
If teenagers are given comprehensive sex education, there will be more teen pregnancies .
These are opposing statements that assume an opposite relationship between the variables: comprehensive sex education increases or decreases the number of teen pregnancies. In fact, these are both alternative hypotheses. This is because they both still assume that there is a relationship between the variables . In other words, both hypothesis statements assume that there is some kind of relationship between sex education and teen pregnancy rates. The alternative hypothesis is also the researcher’s actual predicted outcome, which is why calling it “alternative” can be confusing! However, you can think of it this way: our default assumption is the null hypothesis, and so any possible relationship is an alternative to the default.
Step-by-step sample hypothesis statements
Now that we’ve covered what makes a hypothesis statement strong, how to go about formulating a hypothesis statement, refining your hypothesis statement, and the null hypothesis, let’s put it all together with some examples. The table below shows a breakdown of how we can take a thesis question, identify the variables, create a null hypothesis, and finally create a strong alternative hypothesis.
Once you have formulated a solid thesis question and written a strong hypothesis statement, you are ready to begin your thesis in earnest. Check out our site for more tips on writing a great thesis and information on thesis proofreading and editing services.
Editor’s pick
Get free updates.
Subscribe to our newsletter for regular insights from the research and publishing industry!
Review Checklist
Start with a clear thesis question
Think about “if-then” statements to identify your variables and the relationship between them
Create a null hypothesis
Formulate an alternative hypothesis using the variables you have identified
Make sure your hypothesis clearly posits a relationship between variables
Make sure your hypothesis is testable considering your available time and resources
What makes a hypothesis strong? +
A hypothesis is strong when it is testable, clear, and identifies a potential relationship between two or more variables.
What makes a hypothesis weak? +
A hypothesis is weak when it is too specific or too general, or does not identify a clear relationship between two or more variables.
What is the null hypothesis? +
The null hypothesis posits that the variables you have identified have no relationship.
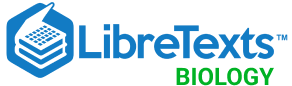
- school Campus Bookshelves
- menu_book Bookshelves
- perm_media Learning Objects
- login Login
- how_to_reg Request Instructor Account
- hub Instructor Commons
Margin Size
- Download Page (PDF)
- Download Full Book (PDF)
- Periodic Table
- Physics Constants
- Scientific Calculator
- Reference & Cite
- Tools expand_more
- Readability
selected template will load here
This action is not available.
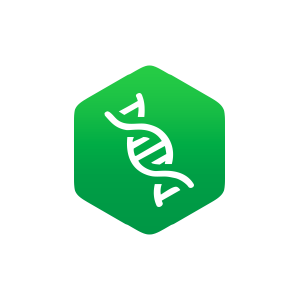
3.14: Experiments and Hypotheses
- Last updated
- Save as PDF
- Page ID 43504
\( \newcommand{\vecs}[1]{\overset { \scriptstyle \rightharpoonup} {\mathbf{#1}} } \)
\( \newcommand{\vecd}[1]{\overset{-\!-\!\rightharpoonup}{\vphantom{a}\smash {#1}}} \)
\( \newcommand{\id}{\mathrm{id}}\) \( \newcommand{\Span}{\mathrm{span}}\)
( \newcommand{\kernel}{\mathrm{null}\,}\) \( \newcommand{\range}{\mathrm{range}\,}\)
\( \newcommand{\RealPart}{\mathrm{Re}}\) \( \newcommand{\ImaginaryPart}{\mathrm{Im}}\)
\( \newcommand{\Argument}{\mathrm{Arg}}\) \( \newcommand{\norm}[1]{\| #1 \|}\)
\( \newcommand{\inner}[2]{\langle #1, #2 \rangle}\)
\( \newcommand{\Span}{\mathrm{span}}\)
\( \newcommand{\id}{\mathrm{id}}\)
\( \newcommand{\kernel}{\mathrm{null}\,}\)
\( \newcommand{\range}{\mathrm{range}\,}\)
\( \newcommand{\RealPart}{\mathrm{Re}}\)
\( \newcommand{\ImaginaryPart}{\mathrm{Im}}\)
\( \newcommand{\Argument}{\mathrm{Arg}}\)
\( \newcommand{\norm}[1]{\| #1 \|}\)
\( \newcommand{\Span}{\mathrm{span}}\) \( \newcommand{\AA}{\unicode[.8,0]{x212B}}\)
\( \newcommand{\vectorA}[1]{\vec{#1}} % arrow\)
\( \newcommand{\vectorAt}[1]{\vec{\text{#1}}} % arrow\)
\( \newcommand{\vectorB}[1]{\overset { \scriptstyle \rightharpoonup} {\mathbf{#1}} } \)
\( \newcommand{\vectorC}[1]{\textbf{#1}} \)
\( \newcommand{\vectorD}[1]{\overrightarrow{#1}} \)
\( \newcommand{\vectorDt}[1]{\overrightarrow{\text{#1}}} \)
\( \newcommand{\vectE}[1]{\overset{-\!-\!\rightharpoonup}{\vphantom{a}\smash{\mathbf {#1}}}} \)
Now we’ll focus on the methods of scientific inquiry. Science often involves making observations and developing hypotheses. Experiments and further observations are often used to test the hypotheses.
A scientific experiment is a carefully organized procedure in which the scientist intervenes in a system to change something, then observes the result of the change. Scientific inquiry often involves doing experiments, though not always. For example, a scientist studying the mating behaviors of ladybugs might begin with detailed observations of ladybugs mating in their natural habitats. While this research may not be experimental, it is scientific: it involves careful and verifiable observation of the natural world. The same scientist might then treat some of the ladybugs with a hormone hypothesized to trigger mating and observe whether these ladybugs mated sooner or more often than untreated ones. This would qualify as an experiment because the scientist is now making a change in the system and observing the effects.
Forming a Hypothesis
When conducting scientific experiments, researchers develop hypotheses to guide experimental design. A hypothesis is a suggested explanation that is both testable and falsifiable. You must be able to test your hypothesis, and it must be possible to prove your hypothesis true or false.
For example, Michael observes that maple trees lose their leaves in the fall. He might then propose a possible explanation for this observation: “cold weather causes maple trees to lose their leaves in the fall.” This statement is testable. He could grow maple trees in a warm enclosed environment such as a greenhouse and see if their leaves still dropped in the fall. The hypothesis is also falsifiable. If the leaves still dropped in the warm environment, then clearly temperature was not the main factor in causing maple leaves to drop in autumn.
In the Try It below, you can practice recognizing scientific hypotheses. As you consider each statement, try to think as a scientist would: can I test this hypothesis with observations or experiments? Is the statement falsifiable? If the answer to either of these questions is “no,” the statement is not a valid scientific hypothesis.
Practice Questions
Determine whether each following statement is a scientific hypothesis.
Air pollution from automobile exhaust can trigger symptoms in people with asthma.
- No. This statement is not testable or falsifiable.
- No. This statement is not testable.
- No. This statement is not falsifiable.
- Yes. This statement is testable and falsifiable.
[reveal-answer q=”429550″] Show Answer [/reveal-answer] [hidden-answer a=”429550″]d: Yes. This statement is testable and falsifiable. This could be tested with a number of different kinds of observations and experiments, and it is possible to gather evidence that indicates that air pollution is not linked with asthma.
[/hidden-answer]
Natural disasters, such as tornadoes, are punishments for bad thoughts and behaviors.
[reveal-answer q=”74245″]Show Answer[/reveal-answer] [hidden-answer a=”74245″]
a: No. This statement is not testable or falsifiable. “Bad thoughts and behaviors” are excessively vague and subjective variables that would be impossible to measure or agree upon in a reliable way. The statement might be “falsifiable” if you came up with a counterexample: a “wicked” place that was not punished by a natural disaster. But some would question whether the people in that place were really wicked, and others would continue to predict that a natural disaster was bound to strike that place at some point. There is no reason to suspect that people’s immoral behavior affects the weather unless you bring up the intervention of a supernatural being, making this idea even harder to test.
Testing a Vaccine
Let’s examine the scientific process by discussing an actual scientific experiment conducted by researchers at the University of Washington. These researchers investigated whether a vaccine may reduce the incidence of the human papillomavirus (HPV). The experimental process and results were published in an article titled, “ A controlled trial of a human papillomavirus type 16 vaccine .”
Preliminary observations made by the researchers who conducted the HPV experiment are listed below:
- Human papillomavirus (HPV) is the most common sexually transmitted virus in the United States.
- There are about 40 different types of HPV. A significant number of people that have HPV are unaware of it because many of these viruses cause no symptoms.
- Some types of HPV can cause cervical cancer.
- About 4,000 women a year die of cervical cancer in the United States.
Practice Question
Researchers have developed a potential vaccine against HPV and want to test it. What is the first testable hypothesis that the researchers should study?
- HPV causes cervical cancer.
- People should not have unprotected sex with many partners.
- People who get the vaccine will not get HPV.
- The HPV vaccine will protect people against cancer.
[reveal-answer q=”20917″] Show Answer [/reveal-answer] [hidden-answer a=”20917″]Hypothesis A is not the best choice because this information is already known from previous studies. Hypothesis B is not testable because scientific hypotheses are not value statements; they do not include judgments like “should,” “better than,” etc. Scientific evidence certainly might support this value judgment, but a hypothesis would take a different form: “Having unprotected sex with many partners increases a person’s risk for cervical cancer.” Before the researchers can test if the vaccine protects against cancer (hypothesis D), they want to test if it protects against the virus. This statement will make an excellent hypothesis for the next study. The researchers should first test hypothesis C—whether or not the new vaccine can prevent HPV.[/hidden-answer]
Experimental Design
You’ve successfully identified a hypothesis for the University of Washington’s study on HPV: People who get the HPV vaccine will not get HPV.
The next step is to design an experiment that will test this hypothesis. There are several important factors to consider when designing a scientific experiment. First, scientific experiments must have an experimental group. This is the group that receives the experimental treatment necessary to address the hypothesis.
The experimental group receives the vaccine, but how can we know if the vaccine made a difference? Many things may change HPV infection rates in a group of people over time. To clearly show that the vaccine was effective in helping the experimental group, we need to include in our study an otherwise similar control group that does not get the treatment. We can then compare the two groups and determine if the vaccine made a difference. The control group shows us what happens in the absence of the factor under study.
However, the control group cannot get “nothing.” Instead, the control group often receives a placebo. A placebo is a procedure that has no expected therapeutic effect—such as giving a person a sugar pill or a shot containing only plain saline solution with no drug. Scientific studies have shown that the “placebo effect” can alter experimental results because when individuals are told that they are or are not being treated, this knowledge can alter their actions or their emotions, which can then alter the results of the experiment.
Moreover, if the doctor knows which group a patient is in, this can also influence the results of the experiment. Without saying so directly, the doctor may show—through body language or other subtle cues—his or her views about whether the patient is likely to get well. These errors can then alter the patient’s experience and change the results of the experiment. Therefore, many clinical studies are “double blind.” In these studies, neither the doctor nor the patient knows which group the patient is in until all experimental results have been collected.
Both placebo treatments and double-blind procedures are designed to prevent bias. Bias is any systematic error that makes a particular experimental outcome more or less likely. Errors can happen in any experiment: people make mistakes in measurement, instruments fail, computer glitches can alter data. But most such errors are random and don’t favor one outcome over another. Patients’ belief in a treatment can make it more likely to appear to “work.” Placebos and double-blind procedures are used to level the playing field so that both groups of study subjects are treated equally and share similar beliefs about their treatment.
The scientists who are researching the effectiveness of the HPV vaccine will test their hypothesis by separating 2,392 young women into two groups: the control group and the experimental group. Answer the following questions about these two groups.
- This group is given a placebo.
- This group is deliberately infected with HPV.
- This group is given nothing.
- This group is given the HPV vaccine.
[reveal-answer q=”918962″] Show Answers [/reveal-answer] [hidden-answer a=”918962″]
- a: This group is given a placebo. A placebo will be a shot, just like the HPV vaccine, but it will have no active ingredient. It may change peoples’ thinking or behavior to have such a shot given to them, but it will not stimulate the immune systems of the subjects in the same way as predicted for the vaccine itself.
- d: This group is given the HPV vaccine. The experimental group will receive the HPV vaccine and researchers will then be able to see if it works, when compared to the control group.
Experimental Variables
A variable is a characteristic of a subject (in this case, of a person in the study) that can vary over time or among individuals. Sometimes a variable takes the form of a category, such as male or female; often a variable can be measured precisely, such as body height. Ideally, only one variable is different between the control group and the experimental group in a scientific experiment. Otherwise, the researchers will not be able to determine which variable caused any differences seen in the results. For example, imagine that the people in the control group were, on average, much more sexually active than the people in the experimental group. If, at the end of the experiment, the control group had a higher rate of HPV infection, could you confidently determine why? Maybe the experimental subjects were protected by the vaccine, but maybe they were protected by their low level of sexual contact.
To avoid this situation, experimenters make sure that their subject groups are as similar as possible in all variables except for the variable that is being tested in the experiment. This variable, or factor, will be deliberately changed in the experimental group. The one variable that is different between the two groups is called the independent variable. An independent variable is known or hypothesized to cause some outcome. Imagine an educational researcher investigating the effectiveness of a new teaching strategy in a classroom. The experimental group receives the new teaching strategy, while the control group receives the traditional strategy. It is the teaching strategy that is the independent variable in this scenario. In an experiment, the independent variable is the variable that the scientist deliberately changes or imposes on the subjects.
Dependent variables are known or hypothesized consequences; they are the effects that result from changes or differences in an independent variable. In an experiment, the dependent variables are those that the scientist measures before, during, and particularly at the end of the experiment to see if they have changed as expected. The dependent variable must be stated so that it is clear how it will be observed or measured. Rather than comparing “learning” among students (which is a vague and difficult to measure concept), an educational researcher might choose to compare test scores, which are very specific and easy to measure.
In any real-world example, many, many variables MIGHT affect the outcome of an experiment, yet only one or a few independent variables can be tested. Other variables must be kept as similar as possible between the study groups and are called control variables . For our educational research example, if the control group consisted only of people between the ages of 18 and 20 and the experimental group contained people between the ages of 30 and 35, we would not know if it was the teaching strategy or the students’ ages that played a larger role in the results. To avoid this problem, a good study will be set up so that each group contains students with a similar age profile. In a well-designed educational research study, student age will be a controlled variable, along with other possibly important factors like gender, past educational achievement, and pre-existing knowledge of the subject area.
What is the independent variable in this experiment?
- Sex (all of the subjects will be female)
- Presence or absence of the HPV vaccine
- Presence or absence of HPV (the virus)
[reveal-answer q=”68680″]Show Answer[/reveal-answer] [hidden-answer a=”68680″]Answer b. Presence or absence of the HPV vaccine. This is the variable that is different between the control and the experimental groups. All the subjects in this study are female, so this variable is the same in all groups. In a well-designed study, the two groups will be of similar age. The presence or absence of the virus is what the researchers will measure at the end of the experiment. Ideally the two groups will both be HPV-free at the start of the experiment.
List three control variables other than age.
[practice-area rows=”3″][/practice-area] [reveal-answer q=”903121″]Show Answer[/reveal-answer] [hidden-answer a=”903121″]Some possible control variables would be: general health of the women, sexual activity, lifestyle, diet, socioeconomic status, etc.
What is the dependent variable in this experiment?
- Sex (male or female)
- Rates of HPV infection
- Age (years)
[reveal-answer q=”907103″]Show Answer[/reveal-answer] [hidden-answer a=”907103″]Answer b. Rates of HPV infection. The researchers will measure how many individuals got infected with HPV after a given period of time.[/hidden-answer]
Contributors and Attributions
- Revision and adaptation. Authored by : Shelli Carter and Lumen Learning. Provided by : Lumen Learning. License : CC BY-NC-SA: Attribution-NonCommercial-ShareAlike
- Scientific Inquiry. Provided by : Open Learning Initiative. Located at : https://oli.cmu.edu/jcourse/workbook/activity/page?context=434a5c2680020ca6017c03488572e0f8 . Project : Introduction to Biology (Open + Free). License : CC BY-NC-SA: Attribution-NonCommercial-ShareAlike
1.2 The Scientific Methods
Section learning objectives.
By the end of this section, you will be able to do the following:
- Explain how the methods of science are used to make scientific discoveries
- Define a scientific model and describe examples of physical and mathematical models used in physics
- Compare and contrast hypothesis, theory, and law
Teacher Support
The learning objectives in this section will help your students master the following standards:
- (A) know the definition of science and understand that it has limitations, as specified in subsection (b)(2) of this section;
- (B) know that scientific hypotheses are tentative and testable statements that must be capable of being supported or not supported by observational evidence. Hypotheses of durable explanatory power which have been tested over a wide variety of conditions are incorporated into theories;
- (C) know that scientific theories are based on natural and physical phenomena and are capable of being tested by multiple independent researchers. Unlike hypotheses, scientific theories are well-established and highly-reliable explanations, but may be subject to change as new areas of science and new technologies are developed;
- (D) distinguish between scientific hypotheses and scientific theories.
Section Key Terms
[OL] Pre-assessment for this section could involve students sharing or writing down an anecdote about when they used the methods of science. Then, students could label their thought processes in their anecdote with the appropriate scientific methods. The class could also discuss their definitions of theory and law, both outside and within the context of science.
[OL] It should be noted and possibly mentioned that a scientist , as mentioned in this section, does not necessarily mean a trained scientist. It could be anyone using methods of science.
Scientific Methods
Scientists often plan and carry out investigations to answer questions about the universe around us. These investigations may lead to natural laws. Such laws are intrinsic to the universe, meaning that humans did not create them and cannot change them. We can only discover and understand them. Their discovery is a very human endeavor, with all the elements of mystery, imagination, struggle, triumph, and disappointment inherent in any creative effort. The cornerstone of discovering natural laws is observation. Science must describe the universe as it is, not as we imagine or wish it to be.
We all are curious to some extent. We look around, make generalizations, and try to understand what we see. For example, we look up and wonder whether one type of cloud signals an oncoming storm. As we become serious about exploring nature, we become more organized and formal in collecting and analyzing data. We attempt greater precision, perform controlled experiments (if we can), and write down ideas about how data may be organized. We then formulate models, theories, and laws based on the data we have collected, and communicate those results with others. This, in a nutshell, describes the scientific method that scientists employ to decide scientific issues on the basis of evidence from observation and experiment.
An investigation often begins with a scientist making an observation . The scientist observes a pattern or trend within the natural world. Observation may generate questions that the scientist wishes to answer. Next, the scientist may perform some research about the topic and devise a hypothesis . A hypothesis is a testable statement that describes how something in the natural world works. In essence, a hypothesis is an educated guess that explains something about an observation.
[OL] An educated guess is used throughout this section in describing a hypothesis to combat the tendency to think of a theory as an educated guess.
Scientists may test the hypothesis by performing an experiment . During an experiment, the scientist collects data that will help them learn about the phenomenon they are studying. Then the scientists analyze the results of the experiment (that is, the data), often using statistical, mathematical, and/or graphical methods. From the data analysis, they draw conclusions. They may conclude that their experiment either supports or rejects their hypothesis. If the hypothesis is supported, the scientist usually goes on to test another hypothesis related to the first. If their hypothesis is rejected, they will often then test a new and different hypothesis in their effort to learn more about whatever they are studying.
Scientific processes can be applied to many situations. Let’s say that you try to turn on your car, but it will not start. You have just made an observation! You ask yourself, "Why won’t my car start?" You can now use scientific processes to answer this question. First, you generate a hypothesis such as, "The car won’t start because it has no gasoline in the gas tank." To test this hypothesis, you put gasoline in the car and try to start it again. If the car starts, then your hypothesis is supported by the experiment. If the car does not start, then your hypothesis is rejected. You will then need to think up a new hypothesis to test such as, "My car won’t start because the fuel pump is broken." Hopefully, your investigations lead you to discover why the car won’t start and enable you to fix it.
A model is a representation of something that is often too difficult (or impossible) to study directly. Models can take the form of physical models, equations, computer programs, or simulations—computer graphics/animations. Models are tools that are especially useful in modern physics because they let us visualize phenomena that we normally cannot observe with our senses, such as very small objects or objects that move at high speeds. For example, we can understand the structure of an atom using models, without seeing an atom with our own eyes. Although images of single atoms are now possible, these images are extremely difficult to achieve and are only possible due to the success of our models. The existence of these images is a consequence rather than a source of our understanding of atoms. Models are always approximate, so they are simpler to consider than the real situation; the more complete a model is, the more complicated it must be. Models put the intangible or the extremely complex into human terms that we can visualize, discuss, and hypothesize about.
Scientific models are constructed based on the results of previous experiments. Even still, models often only describe a phenomenon partially or in a few limited situations. Some phenomena are so complex that they may be impossible to model them in their entirety, even using computers. An example is the electron cloud model of the atom in which electrons are moving around the atom’s center in distinct clouds ( Figure 1.12 ), that represent the likelihood of finding an electron in different places. This model helps us to visualize the structure of an atom. However, it does not show us exactly where an electron will be within its cloud at any one particular time.
As mentioned previously, physicists use a variety of models including equations, physical models, computer simulations, etc. For example, three-dimensional models are often commonly used in chemistry and physics to model molecules. Properties other than appearance or location are usually modelled using mathematics, where functions are used to show how these properties relate to one another. Processes such as the formation of a star or the planets, can also be modelled using computer simulations. Once a simulation is correctly programmed based on actual experimental data, the simulation can allow us to view processes that happened in the past or happen too quickly or slowly for us to observe directly. In addition, scientists can also run virtual experiments using computer-based models. In a model of planet formation, for example, the scientist could alter the amount or type of rocks present in space and see how it affects planet formation.
Scientists use models and experimental results to construct explanations of observations or design solutions to problems. For example, one way to make a car more fuel efficient is to reduce the friction or drag caused by air flowing around the moving car. This can be done by designing the body shape of the car to be more aerodynamic, such as by using rounded corners instead of sharp ones. Engineers can then construct physical models of the car body, place them in a wind tunnel, and examine the flow of air around the model. This can also be done mathematically in a computer simulation. The air flow pattern can be analyzed for regions smooth air flow and for eddies that indicate drag. The model of the car body may have to be altered slightly to produce the smoothest pattern of air flow (i.e., the least drag). The pattern with the least drag may be the solution to increasing fuel efficiency of the car. This solution might then be incorporated into the car design.
Using Models and the Scientific Processes
Be sure to secure loose items before opening the window or door.
In this activity, you will learn about scientific models by making a model of how air flows through your classroom or a room in your house.
- One room with at least one window or door that can be opened
- Work with a group of four, as directed by your teacher. Close all of the windows and doors in the room you are working in. Your teacher may assign you a specific window or door to study.
- Before opening any windows or doors, draw a to-scale diagram of your room. First, measure the length and width of your room using the tape measure. Then, transform the measurement using a scale that could fit on your paper, such as 5 centimeters = 1 meter.
- Your teacher will assign you a specific window or door to study air flow. On your diagram, add arrows showing your hypothesis (before opening any windows or doors) of how air will flow through the room when your assigned window or door is opened. Use pencil so that you can easily make changes to your diagram.
- On your diagram, mark four locations where you would like to test air flow in your room. To test for airflow, hold a strip of single ply tissue paper between the thumb and index finger. Note the direction that the paper moves when exposed to the airflow. Then, for each location, predict which way the paper will move if your air flow diagram is correct.
- Now, each member of your group will stand in one of the four selected areas. Each member will test the airflow Agree upon an approximate height at which everyone will hold their papers.
- When you teacher tells you to, open your assigned window and/or door. Each person should note the direction that their paper points immediately after the window or door was opened. Record your results on your diagram.
- Did the airflow test data support or refute the hypothetical model of air flow shown in your diagram? Why or why not? Correct your model based on your experimental evidence.
- With your group, discuss how accurate your model is. What limitations did it have? Write down the limitations that your group agreed upon.
- Yes, you could use your model to predict air flow through a new window. The earlier experiment of air flow would help you model the system more accurately.
- Yes, you could use your model to predict air flow through a new window. The earlier experiment of air flow is not useful for modeling the new system.
- No, you cannot model a system to predict the air flow through a new window. The earlier experiment of air flow would help you model the system more accurately.
- No, you cannot model a system to predict the air flow through a new window. The earlier experiment of air flow is not useful for modeling the new system.
This Snap Lab! has students construct a model of how air flows in their classroom. Each group of four students will create a model of air flow in their classroom using a scale drawing of the room. Then, the groups will test the validity of their model by placing weathervanes that they have constructed around the room and opening a window or door. By observing the weather vanes, students will see how air actually flows through the room from a specific window or door. Students will then correct their model based on their experimental evidence. The following material list is given per group:
- One room with at least one window or door that can be opened (An optimal configuration would be one window or door per group.)
- Several pieces of construction paper (at least four per group)
- Strips of single ply tissue paper
- One tape measure (long enough to measure the dimensions of the room)
- Group size can vary depending on the number of windows/doors available and the number of students in the class.
- The room dimensions could be provided by the teacher. Also, students may need a brief introduction in how to make a drawing to scale.
- This is another opportunity to discuss controlled experiments in terms of why the students should hold the strips of tissue paper at the same height and in the same way. One student could also serve as a control and stand far away from the window/door or in another area that will not receive air flow from the window/door.
- You will probably need to coordinate this when multiple windows or doors are used. Only one window or door should be opened at a time for best results. Between openings, allow a short period (5 minutes) when all windows and doors are closed, if possible.
Answers to the Grasp Check will vary, but the air flow in the new window or door should be based on what the students observed in their experiment.
Scientific Laws and Theories
A scientific law is a description of a pattern in nature that is true in all circumstances that have been studied. That is, physical laws are meant to be universal , meaning that they apply throughout the known universe. Laws are often also concise, whereas theories are more complicated. A law can be expressed in the form of a single sentence or mathematical equation. For example, Newton’s second law of motion , which relates the motion of an object to the force applied ( F ), the mass of the object ( m ), and the object’s acceleration ( a ), is simply stated using the equation
Scientific ideas and explanations that are true in many, but not all situations in the universe are usually called principles . An example is Pascal’s principle , which explains properties of liquids, but not solids or gases. However, the distinction between laws and principles is sometimes not carefully made in science.
A theory is an explanation for patterns in nature that is supported by much scientific evidence and verified multiple times by multiple researchers. While many people confuse theories with educated guesses or hypotheses, theories have withstood more rigorous testing and verification than hypotheses.
[OL] Explain to students that in informal, everyday English the word theory can be used to describe an idea that is possibly true but that has not been proven to be true. This use of the word theory often leads people to think that scientific theories are nothing more than educated guesses. This is not just a misconception among students, but among the general public as well.
As a closing idea about scientific processes, we want to point out that scientific laws and theories, even those that have been supported by experiments for centuries, can still be changed by new discoveries. This is especially true when new technologies emerge that allow us to observe things that were formerly unobservable. Imagine how viewing previously invisible objects with a microscope or viewing Earth for the first time from space may have instantly changed our scientific theories and laws! What discoveries still await us in the future? The constant retesting and perfecting of our scientific laws and theories allows our knowledge of nature to progress. For this reason, many scientists are reluctant to say that their studies prove anything. By saying support instead of prove , it keeps the door open for future discoveries, even if they won’t occur for centuries or even millennia.
[OL] With regard to scientists avoiding using the word prove , the general public knows that science has proven certain things such as that the heart pumps blood and the Earth is round. However, scientists should shy away from using prove because it is impossible to test every single instance and every set of conditions in a system to absolutely prove anything. Using support or similar terminology leaves the door open for further discovery.
Check Your Understanding
- Models are simpler to analyze.
- Models give more accurate results.
- Models provide more reliable predictions.
- Models do not require any computer calculations.
- They are the same.
- A hypothesis has been thoroughly tested and found to be true.
- A hypothesis is a tentative assumption based on what is already known.
- A hypothesis is a broad explanation firmly supported by evidence.
- A scientific model is a representation of something that can be easily studied directly. It is useful for studying things that can be easily analyzed by humans.
- A scientific model is a representation of something that is often too difficult to study directly. It is useful for studying a complex system or systems that humans cannot observe directly.
- A scientific model is a representation of scientific equipment. It is useful for studying working principles of scientific equipment.
- A scientific model is a representation of a laboratory where experiments are performed. It is useful for studying requirements needed inside the laboratory.
- The hypothesis must be validated by scientific experiments.
- The hypothesis must not include any physical quantity.
- The hypothesis must be a short and concise statement.
- The hypothesis must apply to all the situations in the universe.
- A scientific theory is an explanation of natural phenomena that is supported by evidence.
- A scientific theory is an explanation of natural phenomena without the support of evidence.
- A scientific theory is an educated guess about the natural phenomena occurring in nature.
- A scientific theory is an uneducated guess about natural phenomena occurring in nature.
- A hypothesis is an explanation of the natural world with experimental support, while a scientific theory is an educated guess about a natural phenomenon.
- A hypothesis is an educated guess about natural phenomenon, while a scientific theory is an explanation of natural world with experimental support.
- A hypothesis is experimental evidence of a natural phenomenon, while a scientific theory is an explanation of the natural world with experimental support.
- A hypothesis is an explanation of the natural world with experimental support, while a scientific theory is experimental evidence of a natural phenomenon.
Use the Check Your Understanding questions to assess students’ achievement of the section’s learning objectives. If students are struggling with a specific objective, the Check Your Understanding will help identify which objective and direct students to the relevant content.
As an Amazon Associate we earn from qualifying purchases.
This book may not be used in the training of large language models or otherwise be ingested into large language models or generative AI offerings without OpenStax's permission.
Want to cite, share, or modify this book? This book uses the Creative Commons Attribution License and you must attribute Texas Education Agency (TEA). The original material is available at: https://www.texasgateway.org/book/tea-physics . Changes were made to the original material, including updates to art, structure, and other content updates.
Access for free at https://openstax.org/books/physics/pages/1-introduction
- Authors: Paul Peter Urone, Roger Hinrichs
- Publisher/website: OpenStax
- Book title: Physics
- Publication date: Mar 26, 2020
- Location: Houston, Texas
- Book URL: https://openstax.org/books/physics/pages/1-introduction
- Section URL: https://openstax.org/books/physics/pages/1-2-the-scientific-methods
© Jan 19, 2024 Texas Education Agency (TEA). The OpenStax name, OpenStax logo, OpenStax book covers, OpenStax CNX name, and OpenStax CNX logo are not subject to the Creative Commons license and may not be reproduced without the prior and express written consent of Rice University.
Thank you for visiting nature.com. You are using a browser version with limited support for CSS. To obtain the best experience, we recommend you use a more up to date browser (or turn off compatibility mode in Internet Explorer). In the meantime, to ensure continued support, we are displaying the site without styles and JavaScript.
- View all journals
- Explore content
- About the journal
- Publish with us
- Sign up for alerts
- Perspective
- Published: 03 June 2024
A self-reinforcing cycle hypothesis in heart failure pathogenesis
- Carlos Fernandez-Patron ORCID: orcid.org/0000-0002-8033-0645 1 ,
- Gary D. Lopaschuk ORCID: orcid.org/0000-0003-1010-0454 2 &
- Eugenio Hardy ORCID: orcid.org/0000-0002-8351-4255 3
Nature Cardiovascular Research ( 2024 ) Cite this article
Metrics details
- Energy metabolism
- Heart failure
- Metabolic diseases
- Multienzyme complexes
Heart failure is a progressive syndrome with high morbidity and mortality rates. Here, we suggest that chronic exposure of the heart to risk factors for heart failure damages heart mitochondria, thereby impairing energy production to levels that can suppress the heart’s ability to pump blood and repair mitochondria (both energy-consuming processes). As damaged mitochondria accumulate, the heart becomes deprived of energy in a ‘self-reinforcing cycle’, which can persist after the heart is no longer chronically exposed to (or after antagonism of) the risk factors that initiated the cycle. Together with other previously described pathological mechanisms, this proposed cycle can help explain (1) why heart failure progresses, (2) why it can recur after cessation of treatment, and (3) why heart failure is often accompanied by dysfunction of multiple organs. Ideally, therapy of heart failure syndrome would be best attempted before the self-reinforcing cycle is triggered or designed to break the self-reinforcing cycle.
This is a preview of subscription content, access via your institution
Access options
Subscribe to this journal
Receive 12 digital issues and online access to articles
111,21 € per year
only 9,27 € per issue
Buy this article
- Purchase on Springer Link
- Instant access to full article PDF
Prices may be subject to local taxes which are calculated during checkout
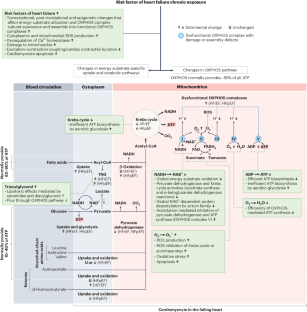
Similar content being viewed by others
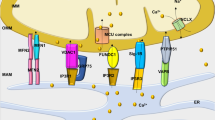
Mitochondrial Ca2+ regulation in the etiology of heart failure: physiological and pathophysiological implications
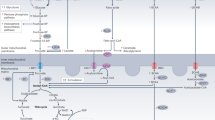
Metabolic mechanisms in physiological and pathological cardiac hypertrophy: new paradigms and challenges
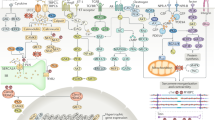
Cellular and molecular pathobiology of heart failure with preserved ejection fraction
Savarese, G. et al. Global burden of heart failure: a comprehensive and updated review of epidemiology. Cardiovasc. Res. 118 , 3272–3287 (2023).
Article PubMed Google Scholar
Neubauer, S. The failing heart—an engine out of fuel. N. Engl. J. Med. 356 , 1140–1151 (2007). Critical appraisal of the cardiac inefficiency model of heart failure with a focus on the interactions between the cardiac mitochondrial OXPHOS pathway and the phosphagen system involving creatine .
Lopaschuk, G. D., Karwi, Q. G., Tian, R., Wende, A. R. & Abel, E. D. Cardiac energy metabolism in heart failure. Circ. Res. 128 , 1487–1513 (2021).
Article CAS PubMed PubMed Central Google Scholar
Packer, M., Lam, C. S. P., Lund, L. H., Maurer, M. S. & Borlaug, B. A. Characterization of the inflammatory–metabolic phenotype of heart failure with a preserved ejection fraction: a hypothesis to explain influence of sex on the evolution and potential treatment of the disease. Eur. J. Heart Fail. 22 , 1551–1567 (2020).
Decherd, G. & Visscher, M. B. Energy metabolism of the failing heart. J. Exp. Med. 59 , 195–199 (1934). A cardiac inefficiency model is first proposed to explain heart failure .
Packer, M. How should physicians view heart failure? The philosophical and physiological evolution of three conceptual models of the disease. Am. J. Cardiol. 71 , 3C–11C (1993). Three distinct conceptual models of heart failure developed over the span of 50 years have provided a rational basis for the treatment of the disease .
Article CAS PubMed Google Scholar
Seta, Y., Shan, K., Bozkurt, B., Oral, H. & Mann, D. L. Basic mechanisms in heart failure: the cytokine hypothesis. J. Card. Fail. 2 , 243–249 (1996). Cytokines, much like the neurohormones, may contribute to the development and progression of heart failure .
Brown, D. A. et al. Expert consensus document: mitochondrial function as a therapeutic target in heart failure. Nat. Rev. Cardiol. 14 , 238–250 (2017).
Packer, M. et al. Blinded withdrawal of long-term randomized treatment with empagliflozin or placebo in patients with heart failure. Circulation 148 , 1011–1022 (2023). Effects of empagliflozin treatment in patients with heart failure dissipate rapidly after withdrawal of the drug .
Mann, D. L., Barger, P. M. & Burkhoff, D. Myocardial recovery and the failing heart: myth, magic, or molecular target? J. Am. Coll. Cardiol. 60 , 2465–2472 (2012).
Article PubMed PubMed Central Google Scholar
Zhou, B. & Tian, R. Mitochondrial dysfunction in pathophysiology of heart failure. J. Clin. Invest. 128 , 3716–3726 (2018). Mitochondria-mediated mechanisms may drive the demise of the heart before the ultimate ATP depletion .
Allard, M. F., Schonekess, B. O., Henning, S. L., English, D. R. & Lopaschuk, G. D. Contribution of oxidative metabolism and glycolysis to ATP production in hypertrophied hearts. Am. J. Physiol. 267 , H742–H750 (1994).
CAS PubMed Google Scholar
Jaswal, J. S., Keung, W., Wang, W., Ussher, J. R. & Lopaschuk, G. D. Targeting fatty acid and carbohydrate oxidation—a novel therapeutic intervention in the ischemic and failing heart. Biochim. Biophys. Acta 1813 , 1333–1350 (2011).
Luptak, I. et al. Long-term effects of increased glucose entry on mouse hearts during normal aging and ischemic stress. Circulation 116 , 901–909 (2007).
Aubert, G., Vega, R. B. & Kelly, D. P. Perturbations in the gene regulatory pathways controlling mitochondrial energy production in the failing heart. Biochim. Biophys. Acta 1833 , 840–847 (2013).
Fillmore, N., Wagg, C. S., Zhang, L., Fukushima, A. & Lopaschuk, G. D. Cardiac branched-chain amino acid oxidation is reduced during insulin resistance in the heart. Am. J. Physiol. Endocrinol. Metab. 315 , E1046–E1052 (2018).
Letts, J. A. & Sazanov, L. A. Clarifying the supercomplex: the higher-order organization of the mitochondrial electron transport chain. Nat. Struct. Mol. Biol. 24 , 800–808 (2017).
Mitchell, P. Coupling of phosphorylation to electron and hydrogen transfer by a chemi-osmotic type of mechanism. Nature 191 , 144–148 (1961).
Ciccarelli, M. et al. Reciprocal organ interactions during heart failure: a position paper from the ESC Working Group on Myocardial Function. Cardiovasc. Res. 117 , 2416–2433 (2021).
Bang, C. et al. Intercellular communication lessons in heart failure. Eur. J. Heart Fail. 17 , 1091–1103 (2015).
Ingwall, J. S. & Weiss, R. G. Is the failing heart energy starved? On using chemical energy to support cardiac function. Circ. Res. 95 , 135–145 (2004).
Ingwall, J. S. Energy metabolism in heart failure and remodelling. Cardiovasc. Res. 81 , 412–419 (2009). Critical appraisal of the cardiac inefficiency model .
Luptak, I. et al. Decreased ATP production and myocardial contractile reserve in metabolic heart disease. J. Mol. Cell. Cardiol. 116 , 106–114 (2018).
Abo Alrob, O. & Lopaschuk, G. D. Role of CoA and acetyl-CoA in regulating cardiac fatty acid and glucose oxidation. Biochem. Soc. Trans. 42 , 1043–1051 (2014).
Bertero, E. & Maack, C. Calcium signaling and reactive oxygen species in mitochondria. Circ. Res. 122 , 1460–1478 (2018). Alterations of intermediate substrate metabolism and oxidative stress, rather than an ATP deficit per se, are proposed to account for maladaptive remodeling and dysfunction during resting conditions .
Lopaschuk, G. D., Ussher, J. R., Folmes, C. D., Jaswal, J. S. & Stanley, W. C. Myocardial fatty acid metabolism in health and disease. Physiol. Rev. 90 , 207–258 (2010).
Bertero, E. & Maack, C. Metabolic remodelling in heart failure. Nat. Rev. Cardiol. 15 , 457–470 (2018).
Miller, B. F. & Hamilton, K. L. A perspective on the determination of mitochondrial biogenesis. Am. J. Physiol. Endocrinol. Metab. 302 , E496–E499 (2012).
Signes, A. & Fernandez-Vizarra, E. Assembly of mammalian oxidative phosphorylation complexes I–V and supercomplexes. Essays Biochem. 62 , 255–270 (2018).
Levine, B., Kalman, J., Mayer, L., Fillit, H. M. & Packer, M. Elevated circulating levels of tumour necrosis factor in severe chronic heart failure. N. Engl. J. Med. 323 , 236–241 (1990).
Packer, M. Heart failure: the most important, preventable, and treatable cardiovascular complication of type 2 diabetes. Diabetes Care 41 , 11–13 (2018).
De Jong, K. A. & Lopaschuk, G. D. Complex energy metabolic changes in heart failure with preserved ejection fraction and heart failure with reduced ejection fraction. Can. J. Cardiol. 33 , 860–871 (2017).
Murashige, D. et al. Extra-cardiac BCAA catabolism lowers blood pressure and protects from heart failure. Cell Metab. 34 , 1749–1764 (2022).
Liu, S. et al. OXPHOS deficiency activates global adaptation pathways to maintain mitochondrial membrane potential. EMBO Rep. 22 , e51606 (2021).
Lopaschuk, G. D., Rebeyka, I. M. & Allard, M. F. Metabolic modulation: a means to mend a broken heart. Circulation 105 , 140–142 (2002).
Kumar, A. A., Kelly, D. P. & Chirinos, J. A. Mitochondrial dysfunction in heart failure with preserved ejection fraction. Circulation 139 , 1435–1450 (2019).
Ridker, P. M. & Rane, M. Interleukin-6 signaling and anti-interleukin-6 therapeutics in cardiovascular disease. Circ. Res. 128 , 1728–1746 (2021).
Packer, M. et al. Empagliflozin in patients with heart failure, reduced ejection fraction, and volume overload: EMPEROR-Reduced Trial. J. Am. Coll. Cardiol. 77 , 1381–1392 (2021).
Hartupee, J. & Mann, D. L. Neurohormonal activation in heart failure with reduced ejection fraction. Nat. Rev. Cardiol. 14 , 30–38 (2017).
Shah, A. M. Parsing the role of NADPH oxidase enzymes and reactive oxygen species in heart failure. Circulation 131 , 602–604 (2015).
Teuber, J. P., Essandoh, K., Hummel, S. L., Madamanchi, N. R. & Brody, M. J. NADPH oxidases in diastolic dysfunction and heart failure with preserved ejection fraction. Antioxidants 11 , 1822 (2022).
Wang, X., Bosonea, A. M. & Fernandez-Patron, C. Metalloproteinases: key and common mediators of multiple GPCRs and candidate therapeutic targets in models of hypertensive cardiac disease. Drug Discov. Today Dis. Models 9 , e103–e108 (2012).
Hao, L., Nishimura, T., Wo, H. & Fernandez-Patron, C. Vascular responses to α1-adrenergic receptors in small rat mesenteric arteries depend on mitochondrial reactive oxygen species. Arterioscler. Thromb. Vasc. Biol. 26 , 819–825 (2006).
Gallo, L. A., Wright, E. M. & Vallon, V. Probing SGLT2 as a therapeutic target for diabetes: basic physiology and consequences. Diab. Vasc. Dis. Res. 12 , 78–89 (2015).
Packer, M. et al. Effect of empagliflozin on worsening heart failure events in patients with heart failure and preserved ejection fraction: EMPEROR-Preserved Trial. Circulation 144 , 1284–1294 (2021).
Lopaschuk, G. D. & Verma, S. Mechanisms of cardiovascular benefits of sodium glucose co-transporter 2 (SGLT2) inhibitors: a state-of-the-art review. JACC Basic Transl. Sci. 5 , 632–644 (2020).
Mazidi, M., Rezaie, P., Gao, H. K. & Kengne, A. P. Effect of sodium–glucose cotransport-2 inhibitors on blood pressure in people with type 2 diabetes mellitus: a systematic review and meta-analysis of 43 randomized control trials with 22 528 patients. J. Am. Heart Assoc. 6 , e004007 (2017).
Packer, M. Evolution of the neurohormonal hypothesis to explain the progression of chronic heart failure. Eur. Heart J. 16 , 4–6 (1995).
Byrne, N. J. et al. Empagliflozin blunts worsening cardiac dysfunction associated with reduced NLRP3 (nucleotide-binding domain-like receptor protein 3) inflammasome activation in heart failure. Circ. Heart. Fail. 13 , e006277 (2020).
Pereira, M. J. & Eriksson, J. W. Emerging role of SGLT-2 inhibitors for the treatment of obesity. Drugs 79 , 219–230 (2019).
Packer, M., Anker, S. D., Butler, J., Filippatos, G. & Zannad, F. Effects of sodium–glucose cotransporter 2 inhibitors for the treatment of patients with heart failure: proposal of a novel mechanism of action. JAMA Cardiol. 2 , 1025–1029 (2017).
Baartscheer, A. et al. Empagliflozin decreases myocardial cytoplasmic Na + through inhibition of the cardiac Na + /H + exchanger in rats and rabbits. Diabetologia 60 , 568–573 (2017).
Mentzer, R. M. Jr. et al. Sodium–hydrogen exchange inhibition by cariporide to reduce the risk of ischemic cardiac events in patients undergoing coronary artery bypass grafting: results of the EXPEDITION study. Ann. Thorac. Surg. 85 , 1261–1270 (2008).
Drakos, S. G. et al. Distinct transcriptomic and proteomic profile specifies patients who have heart failure with potential of myocardial recovery on mechanical unloading and circulatory support. Circulation 147 , 409–424 (2023).
Lopez-Campistrous, A. et al. Mitochondrial dysfunction in the hypertensive rat brain: respiratory complexes exhibit assembly defects in hypertension. Hypertension 51 , 412–419 (2008).
Ghezzi, D. & Zeviani, M. Human diseases associated with defects in assembly of OXPHOS complexes. Essays Biochem. 62 , 271–286 (2018).
Ait-Aissa, K. et al. Mitochondrial oxidative phosphorylation defect in the heart of subjects with coronary artery disease. Sci. Rep. 9 , 7623 (2019).
Gureev, A. P., Shaforostova, E. A. & Popov, V. N. Regulation of mitochondrial biogenesis as a way for active longevity: interaction between the Nrf2 and PGC-1α signaling pathways. Front. Genet. 10 , 435 (2019).
Teintze, M., Slaughter, M., Weiss, H. & Neupert, W. Biogenesis of mitochondrial ubiquinol:cytochrome c reductase (cytochrome bc 1 complex). Precursor proteins and their transfer into mitochondria. J. Biol. Chem. 257 , 10364–10371 (1982).
Tang, J. X., Thompson, K., Taylor, R. W. & Olahova, M. Mitochondrial OXPHOS biogenesis: co-regulation of protein synthesis, import, and assembly pathways. Int. J. Mol. Sci. 21 , 3820 (2020).
Marchingo, J. M. & Cantrell, D. A. Protein synthesis, degradation, and energy metabolism in T cell immunity. Cell. Mol. Immunol. 19 , 303–315 (2022).
Dyck, J. R. & Lopaschuk, G. D. AMPK alterations in cardiac physiology and pathology: enemy or ally? J. Physiol. 574 , 95–112 (2006).
Litvinukova, M. et al. Cells of the adult human heart. Nature 588 , 466–472 (2020).
Mann, D. L. Innate immunity and the failing heart: the cytokine hypothesis revisited. Circ. Res. 116 , 1254–1268 (2015).
Diebold, L. P. et al. Mitochondrial complex III is necessary for endothelial cell proliferation during angiogenesis. Nat. Metab. 1 , 158–171 (2019).
Hargreaves, M. & Spriet, L. L. Skeletal muscle energy metabolism during exercise. Nat. Metab. 2 , 817–828 (2020).
Shulman, R. G. & Rothman, D. L. The ‘glycogen shunt’ in exercising muscle: a role for glycogen in muscle energetics and fatigue. Proc. Natl Acad. Sci. USA 98 , 457–461 (2001).
Pandey, A. et al. Exercise intolerance in older adults with heart failure with preserved ejection fraction: JACC State-of-the-Art Review. J. Am. Coll. Cardiol. 78 , 1166–1187 (2021).
Nilsson, K. R., Duscha, B. D., Hranitzky, P. M. & Kraus, W. E. Chronic heart failure and exercise intolerance: the hemodynamic paradox. Curr. Cardiol. Rev. 4 , 92–100 (2008).
Lawson, C. A. et al. Outcome trends in people with heart failure, type 2 diabetes mellitus and chronic kidney disease in the UK over twenty years. EClinicalMedicine 32 , 100739 (2021).
Hajduk, A. M., Kiefe, C. I., Person, S. D., Gore, J. G. & Saczynski, J. S. Cognitive change in heart failure: a systematic review. Circ. Cardiovasc. Qual. Outcomes 6 , 451–460 (2013).
Packer, M. Does a target dose or a target heart rate matter to outcomes when prescribing β-blockers to patients with chronic heart failure? Circ. Cardiovasc. Qual. Outcomes 11 , e004605 (2018).
Ide, T. et al. Mitochondrial electron transport complex I is a potential source of oxygen free radicals in the failing myocardium. Circ. Res. 85 , 357–363 (1999).
Hansson, A. et al. A switch in metabolism precedes increased mitochondrial biogenesis in respiratory chain-deficient mouse hearts. Proc. Natl Acad. Sci. USA 101 , 3136–3141 (2004).
Bugger, H. et al. Proteomic remodelling of mitochondrial oxidative pathways in pressure overload-induced heart failure. Cardiovasc. Res. 85 , 376–384 (2010).
Wang, X. et al. Cardiac disruption of SDHAF4-mediated mitochondrial complex II assembly promotes dilated cardiomyopathy. Nat. Commun. 13 , 3947 (2022).
Sihag, S., Cresci, S., Li, A. Y., Sucharov, C. C. & Lehman, J. J. PGC-1α and ERRα target gene downregulation is a signature of the failing human heart. J. Mol. Cell. Cardiol. 46 , 201–212 (2009).
Karamanlidis, G. et al. Mitochondrial complex I deficiency increases protein acetylation and accelerates heart failure. Cell Metab. 18 , 239–250 (2013).
Ke, B. X. et al. Tissue-specific splicing of an Ndufs6 gene-trap insertion generates a mitochondrial complex I deficiency-specific cardiomyopathy. Proc. Natl Acad. Sci. USA 109 , 6165–6170 (2012).
Arany, Z. et al. Transcriptional coactivator PGC-1α controls the energy state and contractile function of cardiac muscle. Cell Metab. 1 , 259–271 (2005).
Liu, T. et al. Mitochondrial proteome remodelling in ischemic heart failure. Life Sci. 101 , 27–36 (2014).
Hou, T. et al. NDUFAB1 confers cardio-protection by enhancing mitochondrial bioenergetics through coordination of respiratory complex and supercomplex assembly. Cell Res. 29 , 754–766 (2019).
Sebastiao, M. J. et al. Unveiling human proteome signatures of heart failure with preserved ejection fraction. Biomedicines 10 , 2943 (2022).
Marin-Garcia, J., Goldenthal, M. J., Pierpont, M. E. & Ananthakrishnan, R. Impaired mitochondrial function in idiopathic dilated cardiomyopathy: biochemical and molecular analysis. J. Card. Fail. 1 , 285–291 (1995).
Unverferth, D. V., Lee, S. W. & Wallick, E. T. Human myocardial adenosine triphosphatase activities in health and heart failure. Am. Heart J. 115 , 139–146 (1988).
Ide, T. et al. Mitochondrial DNA damage and dysfunction associated with oxidative stress in failing hearts after myocardial infarction. Circ. Res. 88 , 529–535 (2001).
Jullig, M. et al. Is the failing heart out of fuel or a worn engine running rich? A study of mitochondria in old spontaneously hypertensive rats. Proteomics 8 , 2556–2572 (2008).
Quigley, A. F., Kapsa, R. M., Esmore, D., Hale, G. & Byrne, E. Mitochondrial respiratory chain activity in idiopathic dilated cardiomyopathy. J. Card. Fail. 6 , 47–55 (2000).
Rosca, M. G. et al. Cardiac mitochondria in heart failure: decrease in respirasomes and oxidative phosphorylation. Cardiovasc. Res. 80 , 30–39 (2008).
Gomez, L. A., Monette, J. S., Chavez, J. D., Maier, C. S. & Hagen, T. M. Supercomplexes of the mitochondrial electron transport chain decline in the aging rat heart. Arch. Biochem. Biophys. 490 , 30–35 (2009).
Goldberg, I. J., Trent, C. M. & Schulze, P. C. Lipid metabolism and toxicity in the heart. Cell Metab. 15 , 805–812 (2012).
Mookerjee, S. A., Gerencser, A. A., Nicholls, D. G. & Brand, M. D. Quantifying intracellular rates of glycolytic and oxidative ATP production and consumption using extracellular flux measurements. J. Biol. Chem. 293 , 12649–12652 (2018).
Martinez-Reyes, I. & Chandel, N. S. Mitochondrial TCA cycle metabolites control physiology and disease. Nat. Commun. 11 , 102 (2020).
Shi, Y. et al. Epigenetic regulation in cardiovascular disease: mechanisms and advances in clinical trials. Signal Transduct. Target. Ther. 7 , 200 (2022).
Zhang, X. et al. MicroRNA-195 regulates metabolism in failing myocardium via alterations in sirtuin 3 expression and mitochondrial protein acetylation. Circulation 137 , 2052–2067 (2018).
Vinothkumar, K. R., Zhu, J. & Hirst, J. Architecture of mammalian respiratory complex I. Nature 515 , 80–84 (2014).
Du, Z. et al. Structure of the human respiratory complex II. Proc. Natl Acad. Sci. USA 120 , e2216713120 (2023).
Solmaz, S. R. & Hunte, C. Structure of complex III with bound cytochrome c in reduced state and definition of a minimal core interface for electron transfer. J. Biol. Chem. 283 , 17542–17549 (2008).
Watt, I. N., Montgomery, M. G., Runswick, M. J., Leslie, A. G. & Walker, J. E. Bioenergetic cost of making an adenosine triphosphate molecule in animal mitochondria. Proc. Natl Acad. Sci. USA 107 , 16823–16827 (2010).
He, J. et al. Assembly of the membrane domain of ATP synthase in human mitochondria. Proc. Natl Acad. Sci. USA 115 , 2988–2993 (2018).
Download references
Author information
Authors and affiliations.
Cardiovascular Research Centre, Department of Biochemistry, Faculty of Medicine and Dentistry, College of Health Sciences, University of Alberta, Edmonton, Alberta, Canada
Carlos Fernandez-Patron
Cardiovascular Research Centre, Department of Pediatrics, University of Alberta, Edmonton, Alberta, Canada
Gary D. Lopaschuk
Center of Molecular Immunology, Havana, Cuba
Eugenio Hardy
You can also search for this author in PubMed Google Scholar
Contributions
C.F.-P. conceived the hypothesis. C.F.-P. and E.H. conceived, wrote and edited the manuscript and drafted the figures. G.D.L. made key edits and additions to the intellectual content and figures. All authors contributed to the critical analysis of the literature.
Corresponding authors
Correspondence to Carlos Fernandez-Patron or Eugenio Hardy .
Ethics declarations
Competing interests.
The authors declare no competing interests.
Peer review
Peer review information.
Nature Cardiovascular Research thanks Edoardo Bertero and the other, anonymous, reviewer(s) for their contribution to the peer review of this work.
Additional information
Publisher’s note Springer Nature remains neutral with regard to jurisdictional claims in published maps and institutional affiliations.
Rights and permissions
Springer Nature or its licensor (e.g. a society or other partner) holds exclusive rights to this article under a publishing agreement with the author(s) or other rightsholder(s); author self-archiving of the accepted manuscript version of this article is solely governed by the terms of such publishing agreement and applicable law.
Reprints and permissions
About this article
Cite this article.
Fernandez-Patron, C., Lopaschuk, G.D. & Hardy, E. A self-reinforcing cycle hypothesis in heart failure pathogenesis. Nat Cardiovasc Res (2024). https://doi.org/10.1038/s44161-024-00480-6
Download citation
Received : 04 January 2024
Accepted : 25 April 2024
Published : 03 June 2024
DOI : https://doi.org/10.1038/s44161-024-00480-6
Share this article
Anyone you share the following link with will be able to read this content:
Sorry, a shareable link is not currently available for this article.
Provided by the Springer Nature SharedIt content-sharing initiative
Quick links
- Explore articles by subject
- Guide to authors
- Editorial policies
Sign up for the Nature Briefing newsletter — what matters in science, free to your inbox daily.

The Change Hypothesis: A Simple Template for Leaders
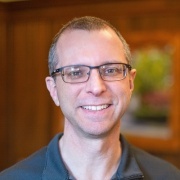
- Website for Mark Wavle
- Contact Mark Wavle
- Twitter for Mark Wavle
- LinkedIn for Mark Wavle
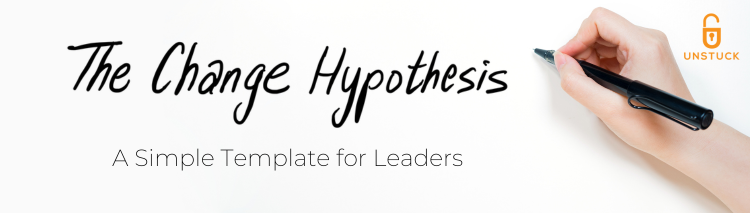
Change. It's a word that can evoke excitement or trepidation. As a leader, navigating change requires a delicate balance. Whether you’re a Scrum Master, Product Owner, Developer, or manager, you need to be passionate about the improvements you seek, but also adaptable to new information and perspectives.
The Change Hypothesis can be your secret weapon!
Creating a testable hypothesis using this simple template helps you analyze the change, articulate it clearly, and guide those you’re leading toward the desired outcome:
We believe that, by [ (3) taking these actions ], we will [(2) achieve this desired future state ] and avoid [(1) the current status quo pain points ]. We'll observe if that's happening by [(4) reviewing these measures ].
Here's a roadmap to guide you through leading successful change:
(1) Current Status Quo Pain Points
Change, by its very nature, disrupts the status quo. We are creatures of habit, and venturing outside our comfort zones can be unsettling. Ignoring this discomfort is a recipe for resistance.
Instead, acknowledge the pain points of the current situation.
Use storytelling to illustrate the negative consequences of inaction. Maybe a particular process is leading to customer frustration, or inefficiencies are hindering productivity. Quantify the impact with data to make the urgency undeniable.
(2) Desired Future State
Once you've established the "why" behind the change, it's time to share the "where." Craft a clear and inspiring vision of the future state. What will things look like after the change is implemented? How will it benefit the organization and those involved?
Storytelling is powerful here too. Vividly describe the improvements people can expect. Don't just tell them what will change, show them. But remember, vision alone isn't enough. Supplement your narrative with data-driven metrics that will track progress and demonstrate the change's success.
(3) Taking Action
People crave a clear path forward, so answer the question, “What’s my role in this?”
Your challenge is to present options that are specific enough for people to understand and act on, while also being open enough that they can have ownership of their part of the change. Try giving some specific example actions, coupled with a few parameters that give boundaries around the broader types of behaviors that will support the change. This fosters a sense of ownership and builds momentum as initial wins are achieved.
(4) Reviewing Measures
Not all metrics are created equal. When selecting metrics to track the progress of your change initiative, resist the urge to focus on activity alone. Did people attend the training session? While important, this doesn't necessarily tell you if they're applying the new skills.
Instead, focus on outcome-based metrics. Look for quantifiable measures that reflect the impact of the change. For example, if your goal is to improve customer satisfaction, track changes in customer satisfaction scores after the change is implemented.
By identifying the outcome-based metrics, everyone gains valuable insights into the effectiveness of the change efforts. This encourages course-correction and discussion that ultimately lead to achieving the desired outcomes.
Embrace the Hypothesis
Think of change as an experiment. Since change involving human behavior is complex, we cannot be certain about the connection between actions and outcomes. The humility and curiosity of a hypothesis help us lead boldly in uncertainty.
The beauty of the Change Hypothesis lies in its adaptability. As you gather feedback and encounter challenges, revisit your hypothesis. Based on what you learn, refine your actions or adjust your vision. This iterative approach keeps the change process moving forward, even when the path isn't always linear.
Leading change isn't about brute force. It's about fostering a shared vision, providing a clear path forward, and being flexible as you navigate the journey together. By following this simple template, you can increase your chances of guiding your team through change and achieving lasting success.
And this is how you can get your change unstuck.
![statement is testable hypothesis [Unstuck Agile Logo]](https://scrumorg-website-prod.s3.amazonaws.com/drupal/inline-images/Unstuck%20Agile%20-%20Logo%20White%20BG%20Orange%20-%20Circle%20250px_1.png)
For more, subscribe to the Unstuck Agile newsletter !
What did you think about this post?
Share with your network.
- Share this page via email
- Share this page on Facebook
- Share this page on Twitter
- Share this page on LinkedIn
View the discussion thread.
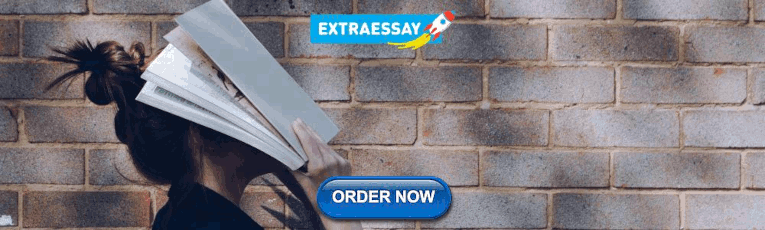
IMAGES
VIDEO
COMMENTS
A hypothesis is a tentative answer to a scientific question. A testable hypothesis is a hypothesis that can be proved or disproved as a result of testing, data collection, or experience. Only testable hypotheses can be used to conceive and perform an experiment using the scientific method .
4. Refine your hypothesis. You need to make sure your hypothesis is specific and testable. There are various ways of phrasing a hypothesis, but all the terms you use should have clear definitions, and the hypothesis should contain: The relevant variables; The specific group being studied; The predicted outcome of the experiment or analysis; 5.
Formulate a clear and testable hypothesis: Based on the research question, use existing knowledge to form a clear and testable hypothesis. The hypothesis should state a predicted relationship between two or more variables that can be measured and manipulated. ... State the null hypothesis: The null hypothesis is a statement that there is no ...
A hypothesis is a tentative statement about the relationship between two or more variables. It is a specific, testable prediction about what you expect to happen in a study. It is a preliminary answer to your question that helps guide the research process. Consider a study designed to examine the relationship between sleep deprivation and test ...
In summary, a hypothesis is a precise, testable statement of what researchers expect to happen in a study and why. Hypotheses connect theory to data and guide the research process towards expanding scientific understanding. An experimental hypothesis predicts what change(s) will occur in the dependent variable when the independent variable is ...
Step 5: Phrase your hypothesis in three ways. To identify the variables, you can write a simple prediction in if … then form. The first part of the sentence states the independent variable and the second part states the dependent variable. If a first-year student starts attending more lectures, then their exam scores will improve.
Table of contents. Step 1: State your null and alternate hypothesis. Step 2: Collect data. Step 3: Perform a statistical test. Step 4: Decide whether to reject or fail to reject your null hypothesis. Step 5: Present your findings. Other interesting articles. Frequently asked questions about hypothesis testing.
An alternative hypothesis is a statement that assumes there is a significant difference or relationship between variables. It is often used as an alternative to the null hypothesis and is tested against the null hypothesis to determine which statement is more accurate. ... Testable: A hypothesis must be able to be tested through observation or ...
hypothesis. science. scientific hypothesis, an idea that proposes a tentative explanation about a phenomenon or a narrow set of phenomena observed in the natural world. The two primary features of a scientific hypothesis are falsifiability and testability, which are reflected in an "If…then" statement summarizing the idea and in the ...
A scientific hypothesis is a tentative, testable explanation for a phenomenon in the natural world. ... However, an untestable statement can be reworded to make it testable. For example, the ...
Formulating Hypotheses for Different Study Designs. Generating a testable working hypothesis is the first step towards conducting original research. Such research may prove or disprove the proposed hypothesis. Case reports, case series, online surveys and other observational studies, clinical trials, and narrative reviews help to generate ...
A testable hypothesis is not a simple statement. It is rather an informed, predictive statement that provides a clear introduction to a study, its goals, and the possible outcomes. There are some important things to consider when building a compelling, testable hypothesis. Clearly state the prediction you are proposing.
A testable hypothesis is not a simple statement. It is rather an intricate statement that needs to offer a clear introduction to a scientific experiment, its intentions, and the possible outcomes. However, there are some important things to consider when building a compelling hypothesis. 1. State the problem that you are trying to solve.
A good hypothesis should be clear, concise, and testable. It typically takes a statement form, predicting a potential outcome or relationship between variables. Make sure that your hypothesis is focused and answers your research question. For example, a hypothesis for the research question stated above might be: "If sunflower plants are ...
Merriam Webster defines a hypothesis as "an assumption or concession made for the sake of argument.". In other words, a hypothesis is an educated guess. Scientists make a reasonable assumption--or a hypothesis--then design an experiment to test whether it's true or not.
H 1. . An hypothesis test is a statistical decision; the conclusion will either be to reject the null hypothesis in favor of the alternative, or to fail to reject the null hypothesis. The decision that we make must, of course, be based on the observed value. x.
A hypothesis proposes a relationship between the independent and dependent variable. A hypothesis is a prediction of the outcome of a test. It forms the basis for designing an experiment in the scientific method.A good hypothesis is testable, meaning it makes a prediction you can check with observation or experimentation.
Make sure your hypothesis clearly posits a relationship between variables. Make sure your hypothesis is testable considering your available time and resources. Before writing a thesis, it is important to create a strong hypothesis statement. This statement is a prediction of what you think will happen in your research study.
Hypothesis B is not testable because scientific hypotheses are not value statements; they do not include judgments like "should," "better than," etc. Scientific evidence certainly might support this value judgment, but a hypothesis would take a different form: "Having unprotected sex with many partners increases a person's risk for ...
Observation may generate questions that the scientist wishes to answer. Next, the scientist may perform some research about the topic and devise a hypothesis. A hypothesis is a testable statement that describes how something in the natural world works. In essence, a hypothesis is an educated guess that explains something about an observation.
A testable hypothesis is one that can be used as the basis for an experiment. It predicts the correlation between two variables and can be tested by varying one of the variables. If the variables cannot be measured, the hypothesis cannot be proved or disproved. If one of the variables cannot be varied, it is impossible to conduct an experiment.
The statement that is a testable hypothesis is "If people brush their teeth twice a day, they will get fewer cavities than if they don't brush their teeth."Option C is a testable hypothesis because it can be tested through an experiment. The hypothesis can be proven true or false by conducting an experiment that involves comparing the rate of ...
The self-reinforcing cycle hypothesis identifies two interconnected pathological events affecting mitochondria in the failing heart: (1) occurrence and accumulation of assembly defects or damage ...
The Change Hypothesis can be your secret weapon! Creating a testable hypothesis using this simple template helps you analyze the change, articulate it clearly, and guide those you're leading toward the desired outcome: and avoid [ (1) the current status quo pain points]. We'll observe if that's happening by [ (4) reviewing these measures].