quality assurance Recently Published Documents
Total documents.
- Latest Documents
- Most Cited Documents
- Contributed Authors
- Related Sources
- Related Keywords
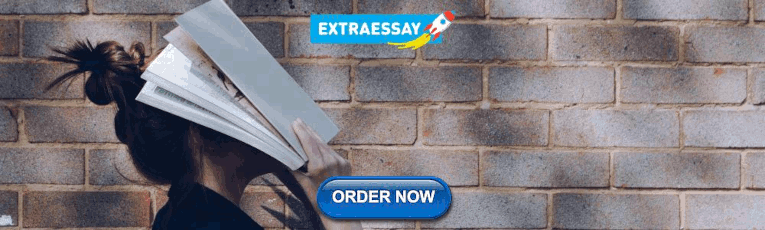
Quality Assurance Information System-The Case of the TEI of Athens
Systematic assessment of data quality and quality assurance/quality control (qa/qc) of current research on microplastics in biosolids and agricultural soils, sigma metrics in quality control- an innovative tool.
The clinical laboratory in today’s world is a rapidly evolving field which faces a constant pressure to produce quick and reliable results. Sigma metric is a new tool which helps to reduce process variability, quantitate the approximate number of analytical errors, and evaluate and guide for better quality control (QC) practices.To analyze sigma metrics of 16 biochemistry analytes using ERBA XL 200 Biochemistry analyzer, interpret parameter performance, compare analyzer performance with other Middle East studies and modify existing QC practices.This study was undertaken at a clinical laboratory for a period of 12 months from January to December 2020 for the following analytes: albumin (ALB), alanine amino transferase (SGPT), aspartate amino transferase (SGOT), alkaline phosphatase (ALKP), bilirubin total (BIL T), bilirubin direct (BIL D), calcium (CAL), cholesterol (CHOL), creatinine (CREAT), gamma glutamyl transferase (GGT), glucose (GLUC), high density lipoprotein (HDL), triglyceride (TG), total protein (PROT), uric acid (UA) and urea. The Coefficient of variance (CV%) and Bias % were calculated from internal quality control (IQC) and external quality assurance scheme (EQAS) records respectively. Total allowable error (TEa) was obtained using guidelines Clinical Laboratories Improvement Act guidelines (CLIA). Sigma metrics was calculated using CV%, Bias% and TEa for the above parameters. It was found that 5 analytes in level 1 and 8 analytes in level 2 had greater than 6 sigma performance indicating world class quality. Cholesterol, glucose (level 1 and 2) and creatinine level 1 showed >4 sigma performance i.e acceptable performance. Urea (both levels) and GGT (level 1) showed <3 sigma and were therefore identified as the problem analytes. Sigma metrics helps to assess analytic methodologies and can serve as an important self assessment tool for quality assurance in the clinical laboratory. Sigma metric evaluation in this study helped to evaluate the quality of several analytes and also categorize them from high performing to problematic analytes, indicating the utility of this tool. In conclusion, parameters showing lesser than 3 sigma need strict monitoring and modification of quality control procedure with change in method if necessary.
SISTEM PENJAMINAN MUTU INTERNAL (SPMI)
Abstract: The purpose of this research is to look at the educational achievements of students through an internal quality assurance system and as a tool to achieve and maintain school progress. Research, with a quantative approach. The data obtained is obtained through interview techniques, observations, and library studies. The results of the study were analyzed by using data reduction, presentation of data and drawing conclusions. The findings of the meaning of the importance of SPMI are implemented in elementary school educational institutions. The study was conducted at one of SMAN 3 Wajo's schools. The results of this study show that: (1) SPMI which is carried out continuously contributes to the acquisition of superior accreditation ratings. (2) The SPMI cycle that is carried out in its entirety has guided the course of various tasks from school stakeholders. (3) Quality culture can be created through the implementation of SPMI.Keywords: Internal Quality Assurance System; Quality of SMAN 3 Wajo School
Quality assurance for on‐table adaptive magnetic resonance guided radiation therapy: A software tool to complement secondary dose calculation and failure modes discovered in clinical routine
Editorial comment: factors impacting us-lirads visualization scores—optimizing future quality assurance and standards, the association of laryngeal position on videolaryngoscopy and time taken to intubate using spatial point pattern analysis of prospectively collected quality assurance data, the impact of policy changes, dedicated funding and implementation support on early intervention programs for psychosis.
Introduction Early intervention services for psychosis (EIS) are associated with improved clinical and economic outcomes. In Quebec, clinicians led the development of EIS from the late 1980s until 2017 when the provincial government announced EIS-specific funding, implementation support and provincial standards. This provides an interesting context to understand the impacts of policy commitments on EIS. Our primary objective was to describe the implementation of EIS three years after this increased political involvement. Methods This cross-sectional descriptive study was conducted in 2020 through a 161-question online survey, modeled after our team's earlier surveys, on the following themes: program characteristics, accessibility, program operations, clinical services, training/supervision, and quality assurance. Descriptive statistics were performed. When relevant, we compared data on programs founded before and after 2017. Results Twenty-eight of 33 existing EIS completed the survey. Between 2016 and 2020, the proportion of Quebec's population having access to EIS rose from 46% to 88%; >1,300 yearly admissions were reported by surveyed EIS, surpassing governments’ epidemiological estimates. Most programs set accessibility targets; adopted inclusive intake criteria and an open referral policy; engaged in education of referral sources. A wide range of biopsychosocial interventions and assertive outreach were offered by interdisciplinary teams. Administrative/organisational components were less widely implemented, such as clinical/administrative data collection, respecting recommended patient-to-case manager ratios and quality assurance. Conclusion Increased governmental implementation support including dedicated funding led to widespread implementation of good-quality, accessible EIS. Though some differences were found between programs founded before and after 2017, there was no overall discernible impact of year of implementation. Persisting challenges to collecting data may impede monitoring, data-informed decision-making, and quality improvement. Maintaining fidelity and meeting provincial standards may prove challenging as programs mature and adapt to their catchment area's specificities and as caseloads increase. Governmental incidence estimates may need recalculation considering recent epidemiological data.
Current Status of Quality Assurance Scheme in Selected Undergraduate Medical Colleges of Bangladesh
This descriptive cross sectional study was carried out to determine the current status of Quality Assurance Scheme in undergraduate medical colleges of Bangladesh. This study was carried out in eight (four Government and four Non- Government) medical colleges in Bangladesh over a period from July 2015 to June 2016. The present study had an interview schedule with open question for college authority and another interview schedule with open question for head of department of medical college. Study revealed that 87.5% of college had Quality Assurance Scheme (QAS) in their college, 75% of college authority had regular meeting of academic coordination committee in their college, 50% of college had active Medical Education Unit in their college, 87.5% of college authority said positively on publication of journal in their college. In the present study researchers interviewed 53 heads of department with open question about distribution, collection of personal review form, submission with recommendation to the academic co-coordinator, and annual review meeting of faculty development. The researchers revealed from the interviews that there is total absence of this practice which is directed in national guidelines and tools for Quality Assurance Scheme (QAS) for medical colleges of Bangladesh. Bangladesh Journal of Medical Education Vol.13(1) January 2022: 33-39
AN APPLICATION OF CADASTRAL FABRIC SYSTEM IN IMPROVING POSITIONAL ACCURACY OF CADASTRAL DATABASES IN MALAYSIA
Abstract. Cadastral fabric is perceived as a feasible solution to improve the speed, efficiency and quality of the cadastral measurement data to implement Positional Accuracy Improvement (PAI) and to support Coordinated Cadastral System (CCS) and Dynamic Coordinated Cadastral System (DCCS) in Malaysia. In light of this, this study aims to propose a system to upgrade the positional accuracy of the existing cadastral system through the utilisation of the cadastral fabric system. A comprehensive investigation on the capability of the proposed system is carried out. A total of four evaluation aspects is incorporated in the study to investigate the feasibility and capability of the software, viz. performance of geodetic least squares adjustment, quality assurance techniques, supporting functions, and user friendliness. This study utilises secondary data obtained from the Department of Surveying and Mapping Malaysia (DSMM). The test area is coded as Block B21701 which is located in Selangor, Malaysia. Results show that least square adjustment for the entire network is completed in a timely manner. Various quality assurance techniques are implementable, namely error ellipses, magnitude of correction vectors and adjustment trajectory, as well as inspection of adjusted online bearings. In addition, the system supports coordinate versioning, coordinates of various datum or projection. Last but not least, user friendliness of the system is identified through the software interface, interaction and automation functions. With that, it is concluded that the proposed system is highly feasible and capable to create a Cadastral Fabric to improve the positional accuracy of existing cadastral system used in Malaysia.
Export Citation Format
Share document.
- Open access
- Published: 19 December 2011
Quality assurance of qualitative research: a review of the discourse
- Joanna Reynolds 1 ,
- James Kizito 2 ,
- Nkoli Ezumah 3 ,
- Peter Mangesho 4 ,
- Elizabeth Allen 5 &
- Clare Chandler 1
Health Research Policy and Systems volume 9 , Article number: 43 ( 2011 ) Cite this article
43k Accesses
55 Citations
5 Altmetric
Metrics details
Increasing demand for qualitative research within global health has emerged alongside increasing demand for demonstration of quality of research, in line with the evidence-based model of medicine. In quantitative health sciences research, in particular clinical trials, there exist clear and widely-recognised guidelines for conducting quality assurance of research. However, no comparable guidelines exist for qualitative research and although there are long-standing debates on what constitutes 'quality' in qualitative research, the concept of 'quality assurance' has not been explored widely. In acknowledgement of this gap, we sought to review discourses around quality assurance of qualitative research, as a first step towards developing guidance.
A range of databases, journals and grey literature sources were searched, and papers were included if they explicitly addressed quality assurance within a qualitative paradigm. A meta-narrative approach was used to review and synthesise the literature.
Among the 37 papers included in the review, two dominant narratives were interpreted from the literature, reflecting contrasting approaches to quality assurance. The first focuses on demonstrating quality within research outputs; the second focuses on principles for quality practice throughout the research process. The second narrative appears to offer an approach to quality assurance that befits the values of qualitative research, emphasising the need to consider quality throughout the research process.
Conclusions
The paper identifies the strengths of the approaches represented in each narrative and recommend these are brought together in the development of a flexible framework to help qualitative researchers to define, apply and demonstrate principles of quality in their research.
Peer Review reports
The global health movement is increasingly calling for qualitative research to accompany its projects and programmes [ 1 ]. This demand, and the funding that goes with it, has led to critical debates among qualitative researchers, particularly over their role as applied or theoretical researchers [ 2 ]. An additional challenge emanating from this demand is to justify research findings and methodological rigour in terms that are meaningful and useful to global public health practitioners. A key area that has grown in quantitative health research has been in quality assurance activities, following the social movement towards evidence-based medicine and global public health [ 3 ]. Through the eyes of this movement, the quality of research affects not only the trajectory of academic disciplines but also local and global health policies. Clinical trials researchers and managers have led much of health research into an era of structured standardised procedures that demarcate and assure quality [ 4 , 5 ].
By contrast, disciplines using qualitative research methods have, to date, engaged far less frequently with quality assurance as a concept or set of procedures, and no standardised guidance for assuring quality exists. The lack of a unified approach to assuring quality can prove unhelpful for the qualitative researcher [ 6 , 7 ], particularly when working in the global health arena, where research needs both to withstand external scrutiny and provide confidence in interpretation of results by internal collaborators Furthermore, past and existing debates on what constitutes 'good' qualitative research have tended to be centred firmly within social science disciplines such as sociology or anthropology, and as such, their language and content may prove difficult to penetrate for the qualitative researcher operating within a multi-disciplinary, and largely positivist, global health environment.
The authors and colleagues within the ACT Consortium [ 8 ] conduct qualitative research that is mostly rooted in anthropology and sociology, to explore the use of antimalarial medicines and intervention trials around antimalarial drug use, within the global health field. Through this work, within the context of clinical trials following Good Clinical Practice (GCP) guidelines [ 4 ], we have identified a number of challenges relating to the demands for evidence of quality and for quality assurance of qualitative research. The quality assurance procedures available for quantitative research, such as GCP training and auditing, are rooted in a positivist epistemology and are not easily translated to the reflexive, subjective nature of qualitative research and the interpretivist-constructionist epistemological position held by many social scientists, including the authors. Experiences of spatial distance between collaborators and those working in remote study field sites have also raised questions around how best to ensure that a qualitative research study is being conducted to high quality standards when the day-to-day research activity is unobservable by collaborators.
In response to the perceived need for the authors' qualitative studies to maintain and demonstrate quality in research processes and outcomes, we sought to identify existing guidance for quality assurance of qualitative research. In the absence of an established unified approach encapsulated in guidance format, we saw the need to review literature addressing the concept and practice of quality assurance of qualitative research, as a precursor to developing suitable guidance.
In this paper, we examine how quality assurance has been conceptualised and defined within qualitative paradigms. The specific objectives of the review were to, firstly, identify literature that expressly addresses the concept of quality assurance of qualitative research, and secondly, to identify common narratives across the existing discourses of quality assurance.
Search strategy
Keywords were identified from a preliminary review of methodological papers and textbooks on qualitative research, reflecting the concepts of 'quality assurance' and 'qualitative research', and all their relevant synonyms. The pool of keywords was augmented and refined iteratively as the search progressed and as the nature of the body of literature became apparent. Five electronic databases-Academic Search Complete, CINAHL Plus, IBSS, Medline and Web of Science-were searched systematically between October and December 2010, using combinations of the following keywords: "quality assurance", "quality assess*", "quality control*", "quality monitor*", "quality manage*, "audit*", "quality", "valid*", "rigo*r", "trustworth*", "legitima*", "authentic*", "strength", "power", "reliabil*", "accura*","thorough*", "credibil*", "fidelity", "authorit*", "integrity", "value", "worth*", "good*", "excellen*", "qualitative AND (research OR inquiry OR approach* OR method* OR paradigm OR epistemolog* OR study). Grey literature was also searched for using Google, and the key phrases "quality assurance" AND "qualitative research".
Several relevant journals- International Journal of Qualitative Methods, International Journal of Social Research Methodology and Social Science and Medicine - were hand searched for applicable papers using the same keywords. Finally, additional literature, in particular books and book chapters, was identified through snowballing techniques, both backwards by following references of eligible papers and forwards through citation chasing. At the point where no new references were identified from the above techniques, the decision was made to curtail the search and begin reviewing, reflecting the practical and time implications of adopting further search strategies.
Inclusion and exclusion criteria
Inclusion criteria were identified prior to the search, to include:
Methodological discussion papers, books or book chapters addressing qualitative research with explicit focus on issues of assuring quality.
Guidance or training documents (in 'grey literature') addressing quality assurance in qualitative research.
Excluded were:
Publications primarily addressing critical appraisal or evaluation of qualitative research for decision-making, reviews or publication. These topics were considered to be distinct from the activity of quality assurance which occurs before writing up and publication.
Publications focusing only on one or more specific qualitative methods or methodological approaches, for example grounded theory or focus groups; focusing on a single stage of the research process only, for example, data collection; or primarily addressing mixed methods of qualitative and quantitative research. It was agreed by the authors that these method-specific papers would not help inform narratives about the discourse of quality assurance, but may become useful at a later date when developing detailed guidance.
Publications not in the English language.
Review methodology
A meta-narrative approach was chosen for the reviewing and synthesis of the literature. This is a systematic method developed by Greenhalgh et al [ 9 ] to make sense of complex, conflicting and diverse sources of literature, interpreting the over-arching narratives across different research traditions and paradigms [ 9 , 10 ]. Within the meta-narrative approach, literature is mapped in terms of its paradigmatic and philosophical underpinnings, critically appraised and then synthesised by constructing narrative accounts of the contributions made by each perspective to the different dimensions of the topic [ 9 ]. Due to the discursive nature of the literature sought, representing different debates and philosophical traditions, the meta-narrative approach was deemed most appropriate for review and synthesis. A process of evaluating papers according to predefined quality criteria and using methods to minimise bias, as in traditional, Cochrane-style systematic reviewing, was not considered suitable or feasible to achieve the objectives.
Each paper was read twice by JR, summarised and analysed to determine the paper's academic tradition, the debates around quality assurance in qualitative research identified and discussed, the definition(s) used for 'quality' and the values underpinning this, and recommended methods or strategies for assuring quality in qualitative research. At the outset of the review, the authors attempted to identify the epistemological position of each paper and to use as a category by which to interpret conceptualisations of quality assurance. However, it emerged that fewer than half of the publications explicitly presented their epistemology; consequently, epistemological position was not used in the analytical approach to this review, but rather as contextual information for a paper, where present.
Following the appraisal of each paper individually, the literature was then grouped by academic disciplines, by epistemological position (where evident) and by recommendations. This grouping enabled the authors to identify narratives across the literature, and to interpret these in association with the research question. The narratives were developed thematically, following the same process used when conducting thematic analysis of qualitative data. First, the authors identified key idea units in each of the papers, then considered and grouped these ideas into broader cross-cutting themes and constructs. These themes, together with consideration of the epistemologies of the papers, were then used to develop overarching narratives emerging from the reviewed literature.
Search results
The above search strategy yielded 93 papers, of which 37 fulfilled the inclusion and exclusion criteria on reading the abstracts or introductory passages. Of the 56 papers rejected, 26 were papers specifically focused on the critical evaluation or appraisal of qualitative research for decision-making, reviews or publication. The majority of the others were rejected for focusing solely on guidance for a specific qualitative method or single stage of the research process, such as data analysis. Dates of publication ranged from 1994 to 2010. This relatively short and recent timeframe can perhaps be attributed in part to the recent history of publishing qualitative research within the health sciences. It was not until the mid-1990s that leading medical publications such as the British Medical Journal began including qualitative studies [ 11 , 12 ], reflecting an increasing acknowledgement of the value of qualitative research within the predominant evidence-based medicine model [ 13 , 14 ]. Within evidence-based medicine, the emphasis on assessment of quality of research is strong, and as such, may account for the timeframe in which consideration of assuring quality of qualitative research emerged.
Among the 37 papers accepted for inclusion in the review, a majority, 19, were from the fields of health, medical or nursing research [ 6 , 15 – 32 ]. 11 papers represented social science in broad terms, but most commonly from a largely sociological perspective [ 33 – 43 ]. Three papers came from education [ 44 – 46 ], two from communication studies [ 47 , 48 ] and one each from family planning [ 49 ] and social policy [ 50 ]. In terms of the types of literature sourced, there were 27 methodological discussion papers, 3 papers containing methodological discussion with one case study, two editorials, two methodology books, two guidance documents and one paper reporting primary research.
Appraisal of literature
Epistemological positions.
In only 10 publications were the authors' epistemological positions clearly identifiable, either explicitly stated or implied in their argument. Of these publications, five represented a postpositivist-realist position [ 16 , 24 , 39 , 44 , 47 ], and five represented an interpretive-constructionist position [ 17 , 21 , 25 , 34 , 38 ]; see Table 1 for further explanation of the authors' use of these terms. Many of the remaining publications appeared to reflect a postpositivist position due to the way in which authors distinguished qualitative research from positivist, quantitative research, and due to the frequent use of terminology derived from Lincoln and Guba's influential postpositivist criteria for quality [ 51 ].
Two strong narratives across the body of literature were interpreted through the review process, plus one other minor narrative.
Narrative 1: quality as assessment of output
A majority of the publications reviewed (n = 22) demonstrated, explicitly or implicitly, an evaluative perspective of quality assurance, linked to assessment of quality by the presence of certain indicators in the research output [ 15 , 16 , 18 – 22 , 24 , 26 , 27 , 30 , 32 , 36 , 39 , 40 , 42 , 44 , 45 , 47 – 50 ]. These publications were characterized by a 'post-hoc' approach whereby quality assurance was framed in terms of demonstrating that particular standards or criteria have been met in the research process. The publications in this narrative typically offered or referred to sets of criteria for research quality, listing specific methods or techniques deemed to be indicators of quality, and the documenting of which in the research output would be assurance of quality [ 15 , 18 – 20 , 24 , 26 , 32 , 39 , 42 , 47 , 48 , 50 ].
Theoretical perspectives of quality
Many of the authors addressing quality of qualitative research from the output perspective drew upon recent debates that juxtapose qualitative and quantitative research in efforts to increase its credibility as an epistemology. Several of the earlier publications from the 1990s discussed the context of an apparent lack of confidence in quality of qualitative research, particularly against the rising prominence of the evidence-based model within health and medical disciplines [ 16 , 19 , 27 ]. This contextual background links into the debate raised in a number of the publications around whether qualitative research should be judged by the same constructs and criteria of quality as quantitative research.
Many publications engaged directly with the discourse of the post-positivist movement of the mid-1980s and early 1990s to develop criteria of quality unique to qualitative research, recognizing that criteria rooted in the positivist tradition were inappropriate for qualitative work [ 18 , 20 , 24 , 26 , 39 , 44 , 47 , 49 , 50 ]. The post-positivist criteria developed by Lincoln and Guba [ 51 ], based around the construct of 'trustworthiness', were referenced frequently and appeared to be the basis upon which a number of authors made their recommendations for improving quality of qualitative research [ 18 , 26 , 39 , 47 , 50 ]. A number of publications explicitly drew on a post-positivist epistemology in their approach to quality of qualitative research, emphasising the need to ensure research presents a 'valid' and 'credible' account of the social reality [ 16 , 18 , 24 , 39 , 44 , 47 ]. In addition, a multitude of other, often rather abstract, constructs denoting quality were identified across the literature contributing to this narrative, including: 'rigour', 'validity', 'credibility', 'reliability', 'accuracy', 'relevance', 'transferability' 'representativeness', 'dependability' and more.
Methods of quality assurance
Checklists of quality criteria, or markers of 'best practice', were common within this output-focused narrative [ 15 , 16 , 19 , 20 , 24 , 32 , 39 , 42 , 47 , 48 ], with arguments for their value centring on a perceived need for standardised methods by which to determine quality in qualitative research [ 20 , 42 , 50 ]. Typically, these checklists comprised specific techniques and methods, the presence of which in qualitative research, was deemed to be an indicator of quality. Among the publications that did not proffer checklists by which to determine quality, methodological techniques signalling quality were also prominent among the authors' recommendations [ 26 , 40 , 44 , 49 ].
A wide range of techniques were referenced across the literature in this narrative as indicators of quality, but common to most publications were recommendations for the use of triangulation, member (or participant) validation of findings, peer review of findings, deviant or negative case analysis and multiple coders of data. Often these techniques were presented in the publications with little explanation of their theoretical underpinnings or in what circumstances they would be appropriate. Furthermore, there was little discussion within the narrative of the quality of these techniques themselves, and how to ensure they are conducted well.
Recognition of limitations
Two of the more recent papers in this review highlight debates of a more fundamental challenge around defining quality, linked to the challenges in defining the qualitative approach itself [ 26 , 32 ]. These papers, and others, reflect upon the plethora of different terminology and methods used in discourse around quality in qualitative research, as well as the numerous different checklists and criteria available to evaluate quality [ 20 , 32 , 40 , 42 ]. Some critique is offered of the inflexibility of fixed lists of criteria by which to determine quality, with authors emphasizing that standards, and the corresponding techniques by which to achieve them, should be selected in accordance with the epistemological position underpinning each research study [ 18 , 20 , 22 , 30 , 32 , 45 ]. However, in much of the literature there is little guidance around how to determine which constructs of quality are most applicable, and how to select the appropriate techniques for its demonstration.
Narrative 2: assuring quality of process
The second narrative identified was less prominent than the first, with fewer publications addressing the assurance of quality in terms of the research process (n = 13). Among these, several explicitly stated the need to consider how to assure quality through the research process, rather than merely evaluating it at output stage [ 6 , 17 , 31 , 33 , 34 , 37 , 38 , 43 ]. The other papers addressed aspects of good qualitative research or researcher that could be considered process rather than output-oriented, without explicitly defining them as quality assurance methods [ 23 , 25 , 35 , 41 , 46 ]. These included process-based methods such as recommending the use of field diaries for on-going self-reflection [ 25 ], and researcher-centred attributes such as an 'underlying methodological awareness' [ 46 ].
Conceptualisations of quality within the literature contributing to this narrative appeared most commonly to reflect a fundamental, internal set of values or principles indicative of the qualitative approach, rather than theoretical constructs such as 'validity' more traditionally linked to the positivist paradigm. These were often presented as principles to be understood and upheld by the research teams throughout the research process, from designing a study, through data collection to analysis and interpretation [ 17 , 31 , 34 , 37 , 38 ]. Six common principles were identified across the narrative: reflexivity of the researcher's position, assumptions and practice; transparency of decisions made and assumptions held; comprehensiveness of approach to the research question; responsibility towards decision-making acknowledged by the researcher; upholding good ethical practice throughout the research; and a systematic approach to designing, conducting and analyzing a study.
Of the four papers in this narrative which explicitly presented an epistemological position, all represented an interpretive/constructionist approach to qualitative research. These principles reflected the prevailing argument in this narrative that unthinking application of techniques or rules of method does not guarantee quality, but rather an understanding of and engagement with the values unique to qualitative paradigms are crucial for conducting quality research [ 6 , 25 , 31 ].
Critique of output-based approach
Within this process-focused narrative emerged a strong theme of critique of the approach to evaluating quality of qualitative research by the research output [ 6 , 17 , 25 , 31 , 33 , 35 , 37 , 38 , 43 , 46 ]. The principle argument underpinning this theme was that judging quality of research by its output does not help assure or manage quality in the process that leads up to it, but rather, the discussion of what constitutes quality should be maintained throughout the research [ 43 , 46 ]. Furthermore, several papers explicitly criticised the use of set criteria or standards against which to determine the quality of qualitative research [ 6 , 34 , 37 , 46 ], arguing that checklists are inappropriate as they may fail to accommodate the subjectivity and creativity of qualitative inquiry. As such, many studies may appear lacking or of poor quality against such criteria [ 46 ].
A number of authors within this narrative argued that checklists can promote the 'uncritical' use of techniques considered indicative of quality research, such as triangulation. Meeting specific criteria may not be a true indication of the quality of the activities or decisions made in the research process [ 37 , 43 ] and methodological techniques become relied upon as "technical fixes" [ 6 ] which do not automatically lead to good research practice or findings. Authors argued that the promotion of such checklists of may result in diminished researcher responsibility for their role in assuring quality throughout the research process [ 6 , 25 , 35 , 38 ], leading to a lack of methodological awareness, responsiveness and accountability [ 38 ].
Assuring quality of the research process
A number of activities were identified across this narrative to be used along the course of qualitative research to improve or assure its quality. They included the researcher conducting an audit or decision trail to document all decisions and interpretations made at each stage of the research [ 25 , 33 , 37 ]; on-going dynamic discussion of quality issues among the research team [ 46 ]; and developing reflexive field diaries in which researchers can explore and capture their own assumptions and biases [ 17 ]. Beyond these specific suggestions, however, were only broader, more conceptual recommendations without detailed guidance on exactly how they could be enacted. These included encouraging researchers to embrace their responsibility for decision making [ 38 ], understanding and applying a broad understanding of the rationale and assumptions behind qualitative research [ 6 ], and ensuring that the 'attitude' with which research is conducted, as well as the methods, are appropriate [ 37 ].
Although specific recommendations to assure quality were not present in all papers contributing to this narrative, there were some commonalities across each publication in the form of the principles or values that the authors identified as underpinning good quality qualitative research. Some of the publications made explicit reference to principles of good practice that should be appreciated and followed to help assure good quality qualitative research, including transparency, comprehensiveness, reflexivity, ethical practice and being systematic [ 6 , 25 , 35 , 37 ]. Across the other publications in this narrative, these principles emerged from definitions or constructs of quality [ 34 ], from recommendations of strategies to improve the research process [ 17 , 31 , 38 , 43 ], or through critiques of the output-focused approach to evaluating quality [ 33 ].
Minor narrative
Two papers did not contribute coherently to either of the two major narratives, but were similar in their approach towards addressing quality of qualitative research [ 28 , 29 ]. Both were methodological discussion papers which engaged with recent and ongoing debates around quality of qualitative research. The authors drew upon the plurality of views of quality within qualitative research, and linked it to the qualitative struggle to demonstrate credibility alongside quantitative research [ 29 ], and the contested nature of qualitative research itself [ 28 ].
The publications also shared critique of existing discourse around quality of qualitative research, but without presentation of alternative ways to assure it. Both papers critiqued the output-focused approach, conceptualising quality in terms of the demonstration of particular technical methods. However, neither paper offers a clear interpretation of the process of quality assurance; when and how it should be conducted, and what it should seek to achieve. One paper synthesised other literature and described abstract principles of qualitative research that indicate quality, but it was not clear whether these were principles were intended as guidance for the research process or standards against which to evaluate the output. Similarly, the second paper argues that quality cannot be assured by predetermined techniques, but does not offer more constructive guidance. Perhaps it can be said that these two papers encapsulate the difficulties that have been faced within the qualitative research field with defining quality and articulating appropriate ways to assure that it reflects the principles of the qualitative approach, which itself is contested.
Synthesis of the two major narratives
The key features of the two major narratives emerging from the review, assuring quality by output and assuring quality by process, have been captured in Table 2 . This table details the perspectives held by each approach, the context in which the narratives are situated, how quality is conceptualised, and examples from the literature of recommended ways in which to assure quality.
The literature reviewed showed a lack of consensus between qualitative research approaches about how to assure quality of research. This reflects past and on-going debates among qualitative researchers about how to define quality, and even the nature of qualitative research itself. The two main narratives that emerged from the reviewed literature reflected differing approaches to quality assurance and, underpinning these differing conceptualisations of quality in qualitative research.
Among the literature that directly discusses quality assurance in qualitative research, the most dominant narrative detected was that of an output-oriented approach. Within this narrative, quality is conceptualised in relation to theoretical constructs such as validity or rigour, derived from the positivist paradigm, and is demonstrated by the inclusion of certain recommended methodological techniques. By contrast, the second, process-oriented narrative presented conceptualisations of quality that were linked to principles or values considered inherent to the qualitative approach, to be understood and enacted throughout the research process. A third, minor narrative offered critique of current and recent discourses on assuring quality of qualitative research but did not appear to offer alternative ways by which to conceptualise or conduct quality assurance.
Strengths of the output-oriented approach for assuring quality of qualitative studies include the acceptability and credibility of this approach within the dominant positivist environment where decision-making is based on 'objective' criteria of quality [ 11 ]. Checklists equip those unfamiliar with qualitative research with the means to assess its quality [ 6 ]. In this way, qualitative research can become more widely accessible, accepted and integrated into decision-making. This has been demonstrated in the increasing presence of qualitative studies in leading medical research journals [ 11 , 12 ]. However, as argued by those contributing to the second narrative in this review, the following of check-lists does not equate with understanding of and commitment to the theoretical underpinnings of qualitative paradigms or what constitutes quality within the approach. The privileging of guidelines as a mechanism to demonstrate quality can mislead inexperienced qualitative researchers as to what constitutes good qualitative research. This runs the risk of reducing qualitative research to a limited set of methods, requiring little theoretical expertise [ 52 ] and diverting attention away from the analytic content of research unique to the qualitative approach [ 14 ]. Ultimately, one can argue that a solely output-oriented approach risks the values of qualitative research becoming skewed towards the demands of the positivist paradigm without retaining quality in the substance of the research process.
By contrast, strengths of the process-oriented approach include the ability of the researcher to address the quality of their research in relation to the core principles or values of qualitative research (see Table 2 ). For example, previous assumptions that incorporating participant-observation methods over an extended period of time in 'the field' constituted 'good' anthropology and an indicator of quality have been challenged on the basis that fieldwork as a method should not be conducted uncritically [ 53 ], without acknowledgement of other important steps, including exploring variability and contradiction [ 54 ], and being explicit about methodological choices made and the theoretical reasons behind them [ 55 ]. The core principles identified in this narrative also represent continuous, researcher-led activities, rather than externally-determined indicators such as validity, or end-points. Reflexivity, for example, is an active, iterative process [ 56 ], described as ' an attitude of attending systematically to the context of knowledge construction... at every step of the research process' [p484, 23]. As such, this approach emphasises the need to consider quality throughout the whole course of research, and locates the responsibility for enacting good qualitative research practice firmly in the lap of the researcher(s).
The question remains, however, as to how researchers can demonstrate to others that core principles have guided their research process. The paucity of guidelines among those advocating a process-oriented approach suggests these are either not possible or not desirable to disseminate. Guidelines, by their largely fixed nature, could be considered incompatible with flexible, pluralistic, qualitative research. Awareness and understanding of the fundamental principles of qualitative research (such as those six identified in this review) could be considered sufficient to ensure that researchers conduct the whole research process to a high standard. Indeed, it could be argued that this type of approach has been promoted within qualitative research fields beyond the health sciences for several decades, since debates around how to do 'good' qualitative research emerged publically [ 41 , 43 , 51 ]. However, the premises of this approach are challenged by increasing scrutiny over the accuracy and ethics of the generation of information through scientific activity [ 57 , 58 ]. Previous critiques of a post-hoc evaluation approach to quality, in favour of procedural mechanisms to ensure good research [ 43 ], have not responded to the demand in some research contexts, particularly in global health, for externally demonstrable quality assurance procedures.
The authors propose, therefore, that some form of guidelines may be possible and desirable, although in a less structured format than those representing a more positivistic paradigm and based on researcher-led principles of good practice rather than externally-determined constructs of quality such as validity. However, first it is important to acknowledge some of the limitations of our search and interpretations.
Limitations
The number of papers included in the review was relatively low. The search was limited to publications explicitly focused on 'quality assurance', and the inclusion criteria may have excluded relevant literature that uses different terminologies, particularly as this concept has not commonly been used within qualitative methods literature. As has been demonstrated in the narratives identified, approaches to quality assurance are linked closely to conceptualisations of quality, about which there is a much larger body of literature than was reviewed for this paper. The possibility of these publications being missed, along with other hard-to-find and grey literature, has implications for the robustness of the narratives identified.
This limitation is perhaps most evident in the lack of literature in this review identified from the field of anthropology. Debates around concepts such as validity and what constitutes 'knowledge' from research have long been of interest to anthropologists [ 55 ], but the absence of these in the publications which met the inclusion criteria raises questions about the search strategy used. Although the search strategy was revised iteratively during the search process to capture variations of quality assurance, anthropological references did not emerge. The choice was made not to pursue the search further for practical and time-related reasons, but also as we felt that limiting the review to quality assurance as originally described would be useful for understanding the literature that a researcher would likely encounter when exploring quality assurance of qualitative research. The lack of clear anthropological voice in this literature reflects the paucity of engagement with the theoretical basis of this discipline in the health sciences, unlike other social sciences such as sociology [ 52 ]. As such, anthropology's contributions to debates on qualitative research methods within health and medical research have been somewhat overlooked [ 59 ].
Hence, this review presents only a part of the discourse of assuring quality of qualitative research, but it does reflect the part that has dominated the fields of health and medical research. Although this review leaves some unanswered questions about defining and assuring quality across different qualitative disciplines, we believe it gives a valuable insight into the types of narratives a typical researcher would begin to engage with if coming from a global health research perspective.
Recommendations
The narratives emerging from this literature review indicate the challenges related to approaching quality assurance from a perspective shaped by the positivist fields of evidence-based medicine, but also the lack of clear, structured guidance based on the intrinsic principles of qualitative research. We recommend that the strengths of both the output-oriented and process-oriented narratives be brought together to create guidance that reflects core principles of qualitative research but also responds to expectations of the global health field for explicitly assured quality in research. The fundamental principles characterising qualitative research, such as the six presented in Table 2 , offer the basis of an approach to assuring quality that is reflexive of and appropriate to the specific values of qualitative research.
The next step in developing guidance should focus on identifying practical and specific advice to researchers as to how to engage with these principles and demonstrate enactment of the principles at each stage of the research process while being wary of promoting unthinking use of 'technical fixes' [ 6 ]. We recommend the development of a framework that helps researchers to identify their core principles, appropriate for their epistemological and methodological approach, and ways to demonstrate that these have been upheld throughout the research process. Current generic quality assurance activities, such as the use of standard operating procedures (SOPs) and monitoring visits could be attuned to the principles of the qualitative research being undertaken through an approach that demonstrates quality without constraining the research or compromising core principles. The development of such a framework should be undertaken in a collaborative way between researchers and field teams undertaking qualitative research in practice. We propose that this framework be flexible enough to accommodate different qualitative methodologies without dictating essential activities for promoting quality. Unlike previous guidance, we propose the framework should also respond to different demands from multi-disciplinary research teams and from external, positivist, audiences for evidence of quality assurance procedures, as may be faced, for example, in the field of global health research. This review has also highlighted the challenges of accessing a broad range of literature from across different social science disciplines (in particular anthropology) when conducting searches using standard approaches adopted in the health sciences. Further consideration should be taken as to how best to encourage wider search parameters, familiarisation with different sources of literature and greater acceptance of non-traditional disciplinary perspectives within health and medical literature reviews.
Within the context of global health research, there is an increasing demand for the qualitative research field to move forwards in developing and establishing coherent mechanisms for quality assurance of qualitative research. The findings of this review have helped to clarify ways in which quality assurance has been conceptualised, and indicates a promising direction in which to take the next steps in this process. Yet, it also raises broader questions around how quality is conceptualised in relation to qualitative research, and how different qualitative disciplines and paradigms are represented in debates around the use of qualitative methods in health and medical research. We recommend the development of a flexible framework to help qualitative researchers to define, apply and demonstrate principles of quality in their research.
Gilson L, Hanson K, Sheikh K, Agyepong IA, Ssengooba F, Bennett S: Building the field of health policy and systems research: social science matters. PLoS Med. 2011, 8: e1001079
Article PubMed PubMed Central Google Scholar
Janes CR, Corbett KK: Anthropology and Global Health. Annual Review of Anthropology. 2009, 38: 167-183.
Article Google Scholar
Pope C: Resisting Evidence: The Study of Evidence-Based Medicine as a Contemporary Social Movement. Health:. 2003, 7: 267-282.
Google Scholar
ICH: ICH Topic E 6 (R1) Guideline for Good Clinical Practice. Book ICH Topic E 6 (R1) Guideline for Good Clinical Practice. 1996, City: European Medicines Agency, Editor ed.^eds.
Good Clinical Practice: Frequently asked questions. [ http://www.mhra.gov.uk/Howweregulate/Medicines/Inspectionandstandards/GoodClinicalPractice/Frequentlyaskedquestions/index.htm#1 ]
Barbour RS: Checklists for improving rigour in qualitative research: a case of the tail wagging the dog?. British Medical Journal. 2001, 322: 1115-1117.
Article CAS PubMed PubMed Central Google Scholar
Dixon-Woods M, Shaw RL, Agarwal S, Smith JA: The problem of appraising qualitative research. Quality and Safety in Health Care. 2004, 13: 223-225.
ACT Consortium. [ http://www.actconsortium.org ]
Greenhalgh T, Robert G, Macfarlane F, Bate P, Kyriakidou O, Peacock R: Storylines of research in diffusion of innovation: a meta-narrative approach to systematic review. Social Science & Medicine. 2005, 61: 417-430.
Greenhalgh T, Potts H, Wong G, al e: Tensions and Paradoxes in Electronic Patient Record Research: A Systematic Literature Review Using the Meta-narrative Method. The Milbank Quarterly. 2009, 87: 729-788.
Stige B, Malterud K, Midtgarden T: Toward an Agenda for Evaluation of Qualitative Research. Qualitative Health Research. 2009, 19: 1504-1516.
Article PubMed Google Scholar
Pope C, Mays N: Critical reflections on the rise of qualitative research. BMJ. 2009, 339: b3425
Dixon-Woods M, Fitzpatrick R, Roberts K: Including qualitative research in systematic reviews: opportunities and problems. Journal of Evaluation in Clinical Practice. 2001, 7: 125-133.
Article CAS PubMed Google Scholar
Eakin JM, Mykhalovskiy E: Reframing the evaluation of qualitative health research: reflections on a review of appraisal guidelines in the health sciences. Journal of Evaluation in Clinical Practice. 2003, 9: 187-194.
Plochg T, van Zwieten M: Guidelines for quality assurance in health and health care research: Qualitative Research. Book Guidelines for quality assurance in health and health care research: Qualitative Research. 2002, City: Amsterdam Centre for Health and Health Care Research, Editor ed.^eds.
Boulton M, Fitzpatrick R: 'Quality' in qualitative research. Critical Public Health. 1994, 5: 19-26.
Bradbury-Jones C: Enhancing rigour in qualitative health research: exploring subjectivity through Peshkin's I's. Journal of Advanced Nursing. 2007, 59: 290-298.
Devers K: How will we know "good" qualitative research when we see it? Beginning the dialogue in health services research. Health Services Research. 1999, 34: 1153-1188.
CAS PubMed PubMed Central Google Scholar
Green J, Britten N: Qualitative research and evidence based medicine. British Medical Journal. 1998, 316: 1230-1232.
Kitto SC, Chesters J, Grbich C: Quality in qualitative research. Medical Journal of Australia. 2008, 188: 243-246.
PubMed Google Scholar
Koch T: Establishing rigour in qualitative research: the decision trail. Journal of Advanced Nursing. 1994, 19: 976-986.
Macdonald ME: Growing Quality in Qualitative Health Research. International Journal of Qualitative Methods. 2009, 8: 97-101.
Malterud K: Qualitative research: standards, challenges, and guidelines. The Lancet. 2001, 358: 483-488.
Article CAS Google Scholar
Mays N, Pope C: Assessing quality in qualitative research. British Medical Journal. 2000, 320: 50-52.
McBrien B: Evidence-based care: enhancing the rigour of a qualitative study. British Journal of Nursing. 2008, 17: 1286-1289.
Nelson AM: Addressing the threat of evidence-based practice to qualitative inquiry through increasing attention to quality: A discussion paper. International Journal of Nursing Studies. 2008, 45: 316-322.
Peck E, Secker J: Quality criteria for qualitative research: does context make a difference?. Qualitative Health Research. 1999, 9: 552-558.
Rolfe G: Validity, trustworthiness and rigour: quality and the idea of qualitative research. Journal of Advanced Nursing. 2006, 53: 304-310.
Ryan-Nicholls KD, Will CI: Rigour in qualitative research: mechanisms for control. Nurse Researcher. 2009, 16: 70-85.
Secker J, Wimbush E, Watson J, Milburn K: Qualitative methods in health promotion research: some criteria for quality. Health Education Journal. 1995, 54: 74-87.
Tobin GA, Begley CM: Methodological rigour within a qualitative framework. Journal of Advanced Nursing. 2004, 48: 388-396.
Whittemore R, Chase SK, Mandle CL: Validity in Qualitative Research. Qualitative Health Research. 2001, 11: 522-537.
Akkerman S, Admiraal W, Brekelmans M, al e: Auditing quality of research in social sciences. Quality and quantity. 2008, 42 (2): 257-274.
Bergman MM, Coxon APM: The Quality in Qualitative Methods. Forum Qualitative Sozialforschung/Forum: Qualitative Social Research. 2005, 6:
Brown A: Qualitative method and compromise in applied social research. Qualitative Research. 2010, 10 (2): 229-248.
Dale A: Editorial: Quality in Social Research. International Journal of Social Research Methodology. 2006, 9: 79-82.
Flick U: Managing quality in qualitative research. 2007, London: Sage Publications
Book Google Scholar
Koro-Ljungberg M: Validity, responsibility, and aporia. Qualitative inquiry. 2010, 16 (8): 603-610.
Lewis J: Redefining Qualitative Methods: Believability in the Fifth Moment. International Journal of Qualitative Methods. 2009, 8: 1-14.
Research Information Network: Quality assurance and assessment of quality research. Book Quality assurance and assessment of quality research. 2010, City: Research Information Network, Editor ed.^eds.
Seale C: The Quality of Qualitative Research. 1999, London: SAGE Publications
Tracy SJ: Qualitative Quality: Eight "Big-Tent" Criteria for Excellent Qualitative Research. Qualitative inquiry. 2010, 16: 837-851.
Morse JM, Barrett M, Mayan M, Olson K, Spiers J: Verification Strategies for Establishing Reliability and Validity in Qualitative Research. International Journal of Qualitative Methods. 2002, 1: 1-19.
Johnson RB: Examining the validity structure of qualitative research. Education. 1997, 118: 282
Creswell JW, Miller DL: Determining Validity in Qualitative Inquiry. Theory Into Practice. 2000, 39: 124
Torrance H: Building confidence in qualitative research: engaging the demands of policy. Qualitative inquiry. 2008, 14 (4): 507-527.
Shenton AK: Strategies for ensuring trustworthiness in qualitative research projects. Education for Information. 2004, 22: 63-75.
Barker M: Assessing the 'Quality' in Qualitative Research. European Journal of Communication. 2003, 18: 315-335.
Forrest Keenan K, van Teijlingen E: The quality of qualitative research in family planning and reproductive health care. Journal of Family Planning and Reproductive Health Care. 2004, 30: 257-259.
Becker S, Bryman A, Sempik J: Defining 'Quality' in Social Policy Research: Views, Perceptions and a Framework for Discussion. Book Defining 'Quality' in Social Policy Research: Views, Perceptions and a Framework for Discussion. 2006, City: Social Policy Association, Editor ed.^eds.
Lincoln YS, Guba EG: Naturalistic inquiry. 1985, Beverly Hills, CA: SAGE Publications
Lambert H, McKevitt C: Anthropology in health research: from qualitative methods to multidisciplinarity. British Medical Journal. 2002, 325: 210-213.
Gupta A, Ferguson J: Introduction-discipline and practice: "the field" as site, method, and location in anthropology". Anthropological locations: boundaries and grounds of a field science. Edited by: Gupta A, Ferguson J. 1997, Berkeley: University of California Press, 1-46.
Manderson L, Aaby P: An epidemic in the field? Rapid assessment procedures and health research. Social Science & Medicine. 1992, 35: 839-850.
Sanjek R: On ethnographic validity. Fieldnotes: the makings of anthropology. Edited by: Sanjek R. 1990, Ithaca, NY: Cornell University Press, 385-418.
Barry C, Britten N, Barber N, al e: Using reflexivity to optimize teamwork in qualitative research. Qualitative Health research. 1999, 9: 26-44.
Murphy E, Dingwall R: Informed consent, anticipatory regulation and ethnographic practice. Social Science & Medicine. 2007, 65: 2223-2234.
Glickman SW, McHutchison JG, Peterson ED, Cairns CB, Harrington RA, Califf RM, Schulman KA: Ethical and Scientific Implications of the Globalization of Clinical Research. New England Journal of Medicine. 2009, 360: 816-823.
Savage J: Ethnography and health care. BMJ. 2000, 321: 1400-1402.
Denzin N, Lincoln YS: Introduction: the discipline and practice of qualitative research. The SAGE Handbook of Qualitative Research. Edited by: Denzin N, Lincoln YS. 2005, Thousand Oaks, CA: SAGE, 3
Download references
Acknowledgements and funding
The authors would like to acknowledge with gratitude the input and insights of Denise Allen in developing the discussion and recommendations of this paper, and in particular, offering an important anthropological voice. JR, JK, PM and CC have full salary support and NE and EA have partial salary support from the ACT Consortium, which is funded through a grant from the Bill & Melinda Gates Foundation to the London School of Hygiene and Tropical Medicine.
Author information
Authors and affiliations.
Department of Global Health & Development, London School of Hygiene & Tropical Medicine, London, UK
Joanna Reynolds & Clare Chandler
Infectious Diseases Research Collaboration, Mulago Hospital Complex, Kampala, Uganda
James Kizito
Department of Sociology/Anthropology, University of Nigeria, Nsukka, Nigeria
Nkoli Ezumah
National Institute for Medical Research, Amani Centre, Muheza, Tanzania
Peter Mangesho
Division of Clinical Pharmacology, Department of Medicine, University of Cape Town, Cape Town, South Africa
Elizabeth Allen
You can also search for this author in PubMed Google Scholar
Corresponding author
Correspondence to Joanna Reynolds .
Additional information
Competing interests.
The authors declare that they have no competing interests.
Authors' contributions
JR helped with the design of the review, searched for and reviewed the literature and wrote the first draft of the manuscript. JK, NE, PM and EA contributed to the interpretation of the results and the writing the manuscript. CC conceived of the review and helped with its design, interpretation of results and writing the manuscript. All authors read and approved the final manuscript.
Rights and permissions
This article is published under license to BioMed Central Ltd. This is an Open Access article distributed under the terms of the Creative Commons Attribution License ( http://creativecommons.org/licenses/by/2.0 ), which permits unrestricted use, distribution, and reproduction in any medium, provided the original work is properly cited.
Reprints and permissions
About this article
Cite this article.
Reynolds, J., Kizito, J., Ezumah, N. et al. Quality assurance of qualitative research: a review of the discourse. Health Res Policy Sys 9 , 43 (2011). https://doi.org/10.1186/1478-4505-9-43
Download citation
Received : 15 July 2011
Accepted : 19 December 2011
Published : 19 December 2011
DOI : https://doi.org/10.1186/1478-4505-9-43
Share this article
Anyone you share the following link with will be able to read this content:
Sorry, a shareable link is not currently available for this article.
Provided by the Springer Nature SharedIt content-sharing initiative
- Qualitative
- global health
- quality assurance
- meta-narrative
- literature review
Health Research Policy and Systems
ISSN: 1478-4505
- Submission enquiries: Access here and click Contact Us
- General enquiries: [email protected]

An official website of the United States government
The .gov means it’s official. Federal government websites often end in .gov or .mil. Before sharing sensitive information, make sure you’re on a federal government site.
The site is secure. The https:// ensures that you are connecting to the official website and that any information you provide is encrypted and transmitted securely.
- Publications
- Account settings
Preview improvements coming to the PMC website in October 2024. Learn More or Try it out now .
- Advanced Search
- Journal List
- Biomed Res Int

Magnitude of the Quality Assurance, Quality Control, and Testing in the Shiraz Cohort Heart Study
Nader parsa.
1 Cardiovascular Research Center, Shiraz University of Medical Sciences, Shiraz, IR, Iran
Mohammad Javad Zibaeenezhad
Maurizio trevisan.
2 City College of New York Provost & Senior Vice President for Academic Affairs, Dean of Medical School, New York, USA
3 College of Health Sciences Vin University Hanoi, Vietnam
Ali Karimi Akhormeh
Mehrab sayadi, associated data.
At this point, “not applicable” in current manuscript.
To determine the conclusive integrity in the Shiraz Cohort Heart Study (SCHS) project, management began quality assurance (QA) and quality control (QC) of the collected data throughout the study end-points. The QA is a focused process that prevents and detects data collection errors and verification of intended requirements in the SCHS. The QC is a subset of QA intended to capture errors in processing data through testing and preventive processes to identify problems, defects, or intended requirements. SCHS involved 10,000 males and females aged 40-70 over a 10-year follow-up period with cardiovascular diseases (CVDs) in the city of Shiraz, Iran. The study measured events and access to preventive care in Shiraz city. The SCHS identified unique barriers to select national study models in developing standardized measures related to variations in ethnicity, religion, cross-cultural considerations, and others. A suggested response to this problem was to develop a mechanism to standardize elements of the questionnaire, study design, and method of administration. This action was based on the geographically normal distribution of the Family Physician Health and Medical Services in Shiraz. Important QA and QC decisions were developed and adopted in the construction of the SCHS and follow-up to ensure conclusive integrity.
1. Introduction
Since the development and refinement of QA, QC, and testing tools in clinical research, the planning and conduction of large studies improved greatly. A substantial literature on QA and QC has appeared in the framework of cohort studies and clinical trials. There has been an emphasis on data quality improvement through standardization of research protocols and education of personnel involved in study and data managing systems [ 1 – 3 ]. Moreover, QA and QC tools were developed for the planning, execution, and effective analysis of epidemiological studies [ 4 ]. Description of QA and QC procedures are largely in the context of a study Greenberg et al. [ 5 ], the Hypertension Primary Prevention Trial [ 6 ], or the Optic Neuritis Treatment Trial [ 7 , 8 ], with some guidelines given within several articles [ 9 , 10 ]. There are not many found in the literature in the framework of nonclinical trial studies, even though some study detailed approaches [ 4 , 11 , 12 ] and general QA and QC guidelines have been addressed [ 13 – 15 ]. There were fewer longitudinal research studies found that exclusively describe QA and QC in their projects to achieve international quality standardization [ 5 , 10 , 16 , 17 ].
The present evaluation aimed to describe and apply QA-QC operations related to the SCHS in the sequence within which they were developed.
1.1. Rationale for QA, QC, and Testing of the SCHS
QA was applied as a preventive process prior to data collection, while QC is a corrective function completed at the beginning and through the end of the data collection process in order to identify and correct errors or discrepancies in the data that are encountered during the entire SCHS.
Key QA and QC decisions were adopted in the construction of this cohort, its follow-up, and integrity of the conclusions drawn of the collected data. Testing was accomplished as a subset of QC as preventive operations that ensured the identification of problems, errors, and defects in software or predetermined requirements. Verification of study ensured that the software system met all types of functionality and subsequently ensured that the functionalities met the intended behavior of validation. As a result, verification, validation, investigation, inspection, and audit were strictly applied for minimizing and eradicating all potential sources of type-I, type-II errors, discrepancies of transcription at data entry and data manipulation for analysis to prevent systemic errors which could lead to an incorrect report of data relations, and poor quality data which could decrease the power in the study.
2. Method and Material
The study aimed to comprehensively follow-up 10,000 males and females in an exclusive-center, based on interviews and tests of varied complexities and diverse ethnicities over a 10-year period with greatly developed QA, QC, and testing tools among Family Physician Health and Medical Services, private clinics, or organizations with geographically normal distributions of the Shiraz metropolitan city.
The magnitude of the SCHS is as a unique metropolitan city cohort study accomplished by careful selection of research instruments. The training, accreditation with individual staff certifications, pretesting, pilot study, and preparation of operation manuals for the methods were all done within the SCHS study facilities.
Evaluation of the baseline data collection followed the approved questionnaires. Follow-up to the questionnaires were communication with the many subgroup populations to standardize the wording of the questions. This gains better understanding of the characteristics within the subgroups and increased understanding by the interviewers. In addition, the questionnaires should be complete and clear as well as the person giving the interview, and the mechanical instruments and technical measurements need to be accurate.
Routinely, QA, QC, and testing were repeated during the beginning, throughout, and after the gathering phase of data with the intent to improve the design and final performance on completion of the operation.
Following the study design and methods, administration of the SCHS geographically normal distributions model combines multiple Family Physician Health and Medical Services base locations into an exclusive single-center cohort study and laboratory test results for data collection that automatically prevent any further QA and QC implementation. A preferred single-center model compared to multiple-center model is more beneficial and exclusively subject to maximum and precisely directed supervision for QA, QC, and testing related to long-term; data collection, clinical laboratory test results, storage biobank samples, and other issues in terms of changes over time; in addition, relationships between QC supervisor, site personnel and to the management of performance at individual sites. In this study, QA, QC, and testing intermittent operations were carried out comprising checkup including the site technicians, test-retest studies, and the monitoring of data through a system of cross visits and supervisions. The dependability of the information was estimated from data that testified the achievement of quality goals. The SCHS QA, QC, and testing systems were according to international experiences, while necessary adjustments were performed by the Steering Committee and its Advisory Committees, on the principles determined by the QA and QC Committee.
In summary, methodological assessment and magnitude of reliability, validity, and accuracy related to raw data quality are indicated in Figure 1 .

Assessment and Magnitude of Reliability, Validity and Accuracy related to Raw Data Quality
3. Results of QA and QC Components Implementation
3.1. result of qa implementation.
As a result, the major implementation component of the QA process was related to protocol development and the creation of operational documents for the SCHS. The operations manual, with clear and detailed descriptions, constituted the study's navigation guidance of all of the activities and was a task vital in the planning for the research team and investigators.
Therefore, objectives and research design, detailed attributes of the population to be studied, sample size, and selection of the instruments to obtain relevant information, including logistic, functional, and financial standpoint by the SCHS Steering and Advisory Committees were developed. The study design of the data collection instruments contained content, format, and step-by-step instructions for finalizing the instrument. The limitations of multiple types of instruments were studied during the development phase so that problems were identified and lessened to the greatest extent possible.
The Operations Committee addressed questions that may arise about protocol implementation to safeguard that the protocols are followed and to address difficulties that may arise. The QA and QC committees monitor the actual data quality and address minor problems for corrections by periodic site cross visits.
We tested volunteers that were like the planned cohort with self-administered questionnaires to identify any problems in the questions prior to the initiation of the actual study. We were looking for any obstacles in response to interviewers that were different to the respondents due to differences in age, gender, or ethnicity. Interviewers were also directed in how to respond to questions by the participants to further clarify the response.
All perspectives of the SCHS protocol were documented in a manual of operations [ 17 ]. Therefore, when the operations manual was created, there was a process to review and correct any section that seemed ambiguous or subject to misinterpretation. Furthermore, SCHS methods were designed to make sure the data produced were accurate, reliable, and valid according to periodic recalibration of equipment and replacement of defective equipment, and do not reflect bias that may arise in subgroups of Family Physician clinics or organization and over time being studied. Also, various equipment used to collect and measure data was standardized and identical throughout the cohort that minimized variabilities.
Consequently, SCHS committee has developed methods for reviewing and updating the protocol and to communicate changes to all study personnel as needed. We conclude the skills and professional dedication individuals had direct bearing on the final quality of data in the study. Therefore, this committee developed procedures to obtain and maintain performance certification of study procedures and processes for monitoring those requirements throughout the course of the study. Practically pivotal testing was conducted for all study personnel to ascertain reliability of self-reported data of self-administered questionnaires to identify any problematic questions before data collection and data entry. For more ascertainment in some cases, we double verified to capture and correct errors of data entry from the original entry.
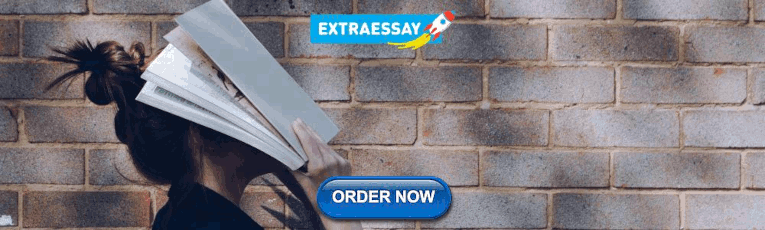
3.2. Result of QC Implementation
As a resultant, the leading accomplishment constituent property of the QC was to identify and correct the causes of either bias or unwarranted disorder in the data sets in any stage of study. The complexity related to QC of SCHS activities was divided into initial, during, and after stages of those issues relevant to the field and reading centers or laboratory. The following processes were necessary for QC procedures of this study before discussing the different stages of management throughout the collection of data.
Moreover, staff incentives for appropriate QC assessments and data collection in this study were encouraged to obtain top quality work. This was achieved in reminding staff that they were an integral part of the larger study to encourage interest in the quality of their work. Periodic QC staff engagement surveys and reviews of work done by staff were sent to the SCHS center to identify how well the site was doing in meeting recruitment goals as well as the total recruited toward the target goals that included gender and ethnicity to make sure participants met the study eligibility criteria.
Experience identified that the QC director should not be the person observing and monitoring the interview as this may negatively impact the technique of the interviewer and the participant's responses. Therefore, the SCHS monitoring key personnel were forbidden to approach these activities.
Literally, in data cleanup of discrepancy issues, the coprincipal investigator routinely analyzes related problems and detecting errors such as extreme or inconsistent values for accuracy purposes. In this study, multiple measures for some variable such as blood pressures due to variations were taken at one point in time to identify possible data errors and to calculate finer measures of data accuracy.
In order to address potential problems that could adversely affect the quality of the study, such as staff turnover and technician drift, staff were required to be certified at the SCHS center along with requirements for a minimum number of procedures to be performed (weekly or monthly) to maintain their certification and thus minimize drift. Furthermore, field center QC activities included efficient training updates by Shiraz Cardiovascular Research Center scientists and recertification throughout the course of the study that ensured minimal QC problems and a major impact on data quality and refreshing protocol.
It was important that equipment be maintained and calibrated regularly in the QC study research center, for all aspects of field study (such as scales calibration and freezers temperature) to minimize any measurement bias or error related to the equipment.
The QC protocols included directives for the cleanliness of the work environment that included sanitizing of equipment (cuffs for measuring blood pressure) and quantitative control of the materials to perform tests and measurements (electrodes, ECG paper, gauze, gloves, alcohol 70%, conductive gel, and etc.). In addition, the room's temperature for blood sample and measurement of blood pressure was monitored throughout the day maintained between 20°C and 24°C.
The final step of the initial QC in this study was transferring blood samples to laboratory for processing (serum, DNA extraction, Buffy Coat) and biobank storage at the -86°C freezer in the central laboratory of the Research Tower for subsequent analytical purposes.
During the collection of the data and before entering the data into the tracking and management systems, we believed there would be some inconsistent participant response over time. Thus, data are collected by well-trained interviewers, as some data had limitations or possible suspension (such as a few proportions of participants reporting one time that they are current smokers and another time they were never smokers). The possible QC solutions were to again contact the participants who gave inconsistent responses and seek clarification. This process helped to verify the data by filling in the missing and inconsistent values. Therefore, in this regard, the quality control of appropriate answers as a repeated measure procedure was conducted for more clarification and consistency of collected data.
4. Discussions of QA and QC Components Implementation
4.1. discussion of qa implementation.
In general, for QA accomplishments to achieve more relevant practical provisions to each situation, pretest and testing of all instruments and procedures were implemented. These performances were pretested and tested in the research context by way of a pilot study in which the entire protocol is completed on volunteers who are demographically like the anticipated SCHS cohort. The pretesting and then testing of the SCHS instruments and procedures, before we included them in the operational manual for the project, were essential to begin the training of the research team.
The unique authenticity of the SCHS study required validation of the instrument as it relates to various ethnicities, religion, cross-cultural considerations, and other design features before it was adopted by the real study.
When the SCHS protocol was developed and documented, training and issuing certification for study personnel, according to the specificities of each procedure, were implemented. Central and identical training was pivotal as it had a direct impact on the interviewers or technicians to perceive the value and consistency of the data. The rationales of training and certification activities resulted in standardization which crucially reduced costs over time. At the end of the training process or when later research team licenses were issued.
Practical procedural lab training included interviewing, blood sampling, and processing (serum, extracted DNA, Buffy Coat) as well as freezing and transporting to the long-term biobank storage (-86°C).
Eventually, while training of the study team was completed, the pilot study was serially conducted with increasing complexity. All validity and reliability performances related to the QA process of study were applied. The SCHS pilot study involved all features of the protocol including interviews, computed variables, entry and transmission of the data to a coordination center, and dispatching samples to the reading center or laboratories.
4.2. Discussion of QC Implementation
Since this study is interested in measuring real change in outcome variables over time, QC procedures assessed and minimized irrelevant variability in these exposure measures that were vital. It should be noted that to separate random biologic variability, measurement error, and true change was difficult. However, it was essential to obtain good estimates of biologic variability and measurement error so that true change could be measured. For this purpose, we approached the available procedure. These proceedings were calibration set at some point early in the study at the reading center or laboratory in a blinded manner at regular intervals with the results tabulated at the SCHS coordination center. This means identified comprehensive drift over a period of time or the introduction of bias into the data.
Therefore, data were carefully reread by the assigned readers in a blinded fashion designed substudy carried out to assess and give estimates of the interreader and intrareader variability.
When estimations of interreader and intrareader variability were presented, the study allowed the estimation of additional characteristics of variability such as field center technician and biologic variability combined. The combination of a reader and technician effect affords an overall measurement error. Therefore, this measurement error could result in any type-I or type-II errors.
In the SCHS, when a continuous data outcome variable was measured with error, we presumed a type-I error may have occurred. Thus, to best explain and clarify the concern, we applied a regression analysis to look at the association between the outcome variable and a set of exposure variables and adjust for the baseline value of the outcome variable. This result could identify a relationship between observed changes in the outcome and exposure variables even when there was no relationship no association existed between the variables and the real change in the outcome variable that was supported by previous research [ 18 ].
In this study, elevated levels of random measurement were thought prone to type-II error, and this may conceal a true association between outcome variable and a set of exposure variables.
In the SCHS study, QC analyses were pivotal to data processed at a reading center or laboratory over a frame of time. Quality problems that led to a type-II error could be encountered and may involve falsification by staff at the QC reading center and laboratory to falsely show improved efficiency in these centers. When falsification was suspected, the techniques of set calibration or longitudinal plots were applied. When falsification was identified, the reader was retrained until performing at an acceptable level before data processed by the technician would be analyzed to see if a statistical correction were achievable or dismissed.
Also, to achieve minimum data loss due to mishandling, mislabeling, or other obstacles for laboratory data, the SCHS well organized the reading center to monitor processing and in cooperation with the coordinating center made sure that enough systems were available to track data between the SCHS field, reading and coordination centers.
Ethical criteria defined by the study implied the adoption of certain processes to pledge the confidentiality of information, such as registering only the recruitment number on the forms and questionnaires [ 19 ]. The list with names and recruitment numbers was the responsibility of the director, and it was disclosed only in some situations, like to relay test results. The research team signed a data confidentiality agreement.
Practically, initial data collection started with the pilot group with gradual increase planned according to the experience of the research team linked to the protocol, flowchart of tests, and interviews. This enabled a more thorough QC that led to the creation of field diaries, which were revised daily and discussed in meetings weekly with the QC team. These tasks were carried out and shared with the QC director of the study center directly or by electronic mail, discussion group, by telephone, or over the internet. At this stage doubts, uncertainty and falsification of records were identified specific problems reviewed, annotated, and commentated to the responsible people for final correction.
After the initial difficulties were overcome, the detailed checklists that were adopted at the initial stage of data collection by the SCHS steering committee were revised and simplified to be used for the final study. Periodically, the interviews of a certain week were recorded, and randomly, some were evaluated by a coprincipal. At this step, emphasis was given to the interview's fluency, the correct completion of the answers, and suitability in the participant communication.
When data collection and entry occurred in the system, the correct marking of the answers of the interview (missing, unknown, blank answers, skip errors, or other inconsistencies) was made by the system. Random repetition of measurements by the same person or by another was used for some tests and questionnaires. At this point, consideration was taken so that the measurements by the same interviewer or technician could not remember the previous results, and that, the test and retest results were independent. This procedure also used for the reliability of laboratory and echocardiography and was not more than 15-20 minutes. For the degree of agreement related to test-retest of accuracy, reliability, and validity, Cohen's Kappa statistical coefficients were implemented [ 20 ].
A final aspect of field center data quality must be considered in the SCHS related to inconsistent participant response over time. Thus, data were correctly collected by interviewer staff, though the limitations of reported data in advanced are well-known. As an example, a few participants reported initially that they were current smokers and then later that they were never smokers. Thus, for this purpose and clarification of suspicious inconsistent data, a double-blind retest was conducted at each visit for some data. Findings of any artificial or actual triggers such as unknown, missing, incomplete, and lack of homogeneity of data for each follow-up event, we applied quality control measures to find problems by the random double-blind test and retest, for any inconsistencies in data collection. We performed daily and weekly quality assurance, quality control, and testing before data entry, to remove any risk of influencing environmental, societal, or emotional factors. However, if the coprinciple of the study assumes there were problems, that person directly reevaluates and retests with another reviewer. To enhance quality assurance and quality control and certainty, double-blind checking by Kappa statistical coefficients agreement data is applied to locate any inconsistent data. The quality control protocol should recognize that such inconsistencies will occur and have a method in place for handling them and clean up data. Possible solutions are to recontact participants with inconsistent responses and ask for clarification, to set inconsistent responses to missing values, or, if possible, to use an independent source (such as laboratory results and other related collected data) to verify the data and report to the Advisory Committees. If data was still incomplete and not able to correct after contacting participants and an in-depth revision and assessment of collected data before data entry, the coprincipal of the SCHS and QA and QC teams were approached for the solutions.
Consequently, in this study, after in-depth assessment and revision of collected data and data entry by the coprincipal of the SCHS QA, QC teams approximately 2% of the files had minor inconsistency according to a previously defined script. This finding was produced to meet the study's requirement goals, and data incorporated into the system were also generated for exploitation. Moreover, the magnitude of the QA and QC processes in this study required a considerable review of study resources and should not be underestimated.
5. In Conclusion
We achieved a well-designed SCHS study with in-depth QA and QC proceedings by the coprincipal of the study and dedication by the research team to the process, accuracy, reliability, validity, and integrity of conclusions can be established, and the acquired experience would be useful to other cohort studies.
Acknowledgments
We are thankful to all researchers in this paper references regarding the valuable scientific suggestions and guidance for improvement of this work. In addition, we appreciate Vice Chancellery of Research Affairs of Shiraz University of Medical Sciences regarding funding the study. This work was supported by the Vice Chancellery of Research Affairs of Shiraz University of Medical Sciences (grant number 93-7102). The funding source has no role in the study design and neither in the execution, interpretation, writing, and results submission.
Data Availability
Ethical approval.
This study is in accordance with the Helsinki Declaration and has been approved by the Research Ethics Committee of Shiraz University of Medical Sciences (No: 2017–358) and signing a written informed consent in the preliminary step. Consequently, participants are free to withdraw on their request at any time. 24 Collected data are kept encrypted in a software with authorities' access only. Findings of the study will be published at a national or international scale through peer-reviewed journals.
A signed written consent form is/will be received from each participant.
Conflicts of Interest
There is no conflict of interests to declare.
Authors' Contributions
N.P. conceived the conceptualization. N.P., A.KA., and M.S. gathered the data. N.P., M.S., and A.KA conducted the formal analysis. N.P. and MJ.Z performed the funding acquisition. N.P. and M.S. worked the methodology. N.P. and MJ.Z. did the supervision. N.P. and A.KA. wrote the original draft. N.P. and M.T. reviewed and edited the manuscript. The manuscript was read and approved by all authors.

Data Science Journal

- Download PDF (English) XML (English)
- Alt. Display
Research Papers
Data quality assurance at research data repositories.
- Maxi Kindling
- Dorothea Strecker
This paper presents findings from a survey on the status quo of data quality assurance practices at research data repositories.
The personalised online survey was conducted among repositories indexed in re3data in 2021. It covered the scope of the repository, types of data quality assessment, quality criteria, responsibilities, details of the review process, and data quality information and yielded 332 complete responses.
The results demonstrate that most repositories perform data quality assurance measures, and overall, research data repositories significantly contribute to data quality. Quality assurance at research data repositories is multifaceted and nonlinear, and although there are some common patterns, individual approaches to ensuring data quality are diverse. The survey showed that data quality assurance sets high expectations for repositories and requires a lot of resources. Several challenges were discovered: for example, the adequate recognition of the contribution of data reviewers and repositories, the path dependence of data review on review processes for text publications, and the lack of data quality information. The study could not confirm that the certification status of a repository is a clear indicator of whether a repository conducts in-depth quality assurance.
- research data
- research data quality
- data quality assurance
- research data repositories
1 Introduction
Upon collection, research data are rarely fit for analysis or publication in a repository; instead, additional processing and other measures are necessary to ensure that data conform to quality expectations. In the context of research data, quality is an ubiquitous yet elusive concept. It is stated as the motivation behind data curation ( Johnston et al. 2018 ) and the FAIR Principles ( Wilkinson et al. 2016 ), often without describing or defining the concept in more detail.
So far, data quality assurance practices at research data repositories have not been researched systematically. Ensuring data quality is sometimes conceptualised as a part of data curation, which makes it difficult to get specific insights into data quality assurance processes. In addition, the role of research data repositories in the quality assurance process remains unclear. Given the expertise and resources repositories provide, it must be assumed that they contribute to data quality assurance. However, little is known about how they define data quality or what quality assurance measures they take; as a result, their contributions remain largely invisible.
Certificates evaluate certain aspects of research data repositories, including quality assurance measures. So far, it is unknown whether there is a relationship between the certification status of a repository and the data quality assurance measures it performs.
To address these gaps, this study aims at analysing the status quo of data quality assurance at research data repositories. It investigates the measures of data quality assurance that repositories take as well as the influence of repository certification on the prevalence of these measures.
2 Literature
2.1 data quality.
The term quality can refer to inherent or essential characteristics of an object, but it can also be used in the context of evaluating, rating, or comparing objects ( Merriam-Webster 2022 ). In this paper, we focus on quality in the second sense. Definitions of quality sometimes refer to intrinsic characteristics of an object that are universal ( Wang & Strong 1996 ), but more often, they are context-dependent and situational. For example, widely used context-dependent definitions describe the quality of a thing based on its conformance to a set of requirements ( ISO 2015 ) or in relation to the needs of a stakeholder intending to use it for a specific purpose; this idea is commonly referred to as fitness for use ( Juran 1951 ). In the context of data, quality is often conceptualised as fitness for use, highlighting the need to take the perspective of data users into account ( Wang & Strong 1996 ).
A stated objective in many definitions of research data quality is the reusability of data ( Peer, Stephenson & Green 2014 ). For example, the FAIR Principles conceptualise data quality as a ‘function of its ability to be accurately and appropriately found, re-used, and cited over time, by all stakeholders, both human and mechanical’ ( Wilkinson et al. 2016: 3 ).
Definitions of data quality are often supplemented by dimensions that outline general aspects of data quality and criteria that specify what characteristics make data fit for use in a certain context. Wang and Strong ( 1996 ) published the most widely cited framework for data quality to date, and it remains a milestone in describing quality criteria from the perspective of data users. It includes 20 quality dimensions grouped into four categories. Although the framework is applied in the context of research data, its original scope was business data, and it remains unclear whether all criteria are applicable to research data ( RfII 2020 ; Koltay 2020 ). Theoretical reflections on data quality also started evolving around this time ( Lee et al. 2002 ; Madnick et al. 2009 ). In the current discourse around data quality, the FAIR Principles have become central ( Peng et al. 2022 ) as well as aspects of openness ( Koltay 2020 ).
It is important to note that quality dimensions and criteria mentioned in the literature are not always congruent nor do they always coincide ( Lee et al. 2002 ), highlighting the context dependence of research data quality. In addition, definitions of concepts and the use of terminology in sources also varies. Therefore, in a pragmatic approach, this study focuses on quality criteria as an expression of characteristics that make data fit for use.
The literature mentions a variety of data quality criteria: for example, accuracy, appropriate use of methods, consistency, coverage, or reuse potential. Table 1 lists quality criteria that are mentioned in the literature. The list is not exhaustive, but it gives examples of criteria used for the evaluation of data quality.
Examples of data quality criteria mentioned in the literature.
Metadata and data documentation are important factors of data quality ( Austin et al. 2016 ; Lafia et al. 2021 ) because datasets require context to be useful. Therefore, Assante et al. ( 2016 ) argue that if data quality is conceptualised as fitness for use, repositories should prioritise providing sufficient metadata and documentation to enable data users to evaluate the fitness of a dataset for their use case ( Assante et al. 2016 ). In that sense, metadata quality and data quality are strongly connected. Lawrence et al. ( 2011 ) even state that ‘quality data is not possible without quality metadata’ ( Lawrence et al. 2011: 15 ).
2.2 Data quality assurance
Data quality assurance is a concept that is associated with processes and techniques for assessing, measuring, and improving quality. In the context of data publications, quality assurance is seen as the process of assessing data and taking necessary actions to make sure that they meet the requirements of the purpose for which they are used ( Peer, Stephenson & Green 2014 ). This process spans the entire research data life cycle ( RfII 2020 ). Following a contextual approach to data quality as fitness for use, assessing data quality needs to take into account both the dataset and the context in which it would be used ( Canali 2020 ).
It is important to note that there is some overlap between the concepts data quality assurance, data stewardship , and data curation . Peng et al. ( 2015 ) describe data quality assurance as a component of data stewardship (the responsible safeguarding of data) that contributes to the usefulness of data over time. Definitions of data quality assurance and data curation also partially intersect; for example, data curation is also often tied to the idea of producing data that are fit for a specific purpose ( CASRAI 2022a ). Aspects of quality assurance are sometimes subsumed under data curation activities ( Lafia et al. 2021 ). However, conceptualising data quality assurance as simply an aspect of data stewardship or data curation makes it difficult to analyse and understand specific characteristics of data quality assurance. Overall, more research on the intersection of these concepts is required.
Data quality assurance includes multiple activities, of which the assessment of data quality is one. Often, the literature divides data quality assessment into two processes: evaluating formal or technical aspects of data and evaluating aspects related to the content or scientific value of datasets ( Austin et al. 2017 ). This idea is grounded in the multifaceted nature of the quality assurance process that may require several reviewers with different skill sets, for example, domain experts and data curators, and in the observation that repositories provide varying degrees of review, for example, by only considering technical aspects of quality ( Mayernik et al. 2015 ). Practices and norms are sometimes adopted from the peer review of text publications, with the assumption that this will produce scientific output—data publications—of similar value and trustworthiness ( Parsons & Fox 2013 ).
2.3 Data quality assurance and repositories
Repositories are important actors in ensuring data quality, but they follow different approaches ( Peer, Stephenson & Green 2014 ). Some adopt a self-deposit model, where most responsibilities for quality assurance lie with the data depositor ( Austin et al. 2016 ), whereas others take on a more active role. The level of data curation performed at repositories and, as a result, the quality of metadata varies ( Koshoffer et al. 2018 ).
Repository features and functionalities support certain dimensions of data quality: for example, increasing the usability of data by providing comprehensive data documentation ( Trisovic et al. 2021 ). Nevertheless, implementing data quality assurance is a complex task for research data repositories because of the continuous nature of the process, shared responsibilities involving multiple stakeholders, and the many facets of data quality ( Assante et al. 2016 ). Quality assurance incurs costs for research data repositories but contributes to the efficiency of data management and the long-term usability and reuse value of data ( Parr et al. 2019 ). In focus groups, researchers have stated that they consider quality assurance among the most important curation activities at research data repositories ( Johnston et al. 2018 ).
Repositories adopt different strategies for meeting staffing needs of quality assurance. For example, discipline-specific repositories may rely on data curators with a background in the respective discipline, whereas data curators at institutional repositories without a clear disciplinary focus may collaborate with subject specialists ( Lee & Stvilia 2017 ).
Some repositories seek formal certification to increase users’ trust in their services. Certificates can take quality assurance measures into account; for example, CoreTrustSeal asks applicants to describe their approach to ensuring (meta)data quality ( CTS 2019 ). However, repository certification cannot and does not intend to guarantee that all individual datasets published with a service are of high quality ( Assante et al. 2016 ). So far, the relationship between repository certification and the degree of quality assurance has not yet been investigated by systematic studies.
2.4 Data quality information
To ensure transparency and assist repository users in making informed decisions about data reuse, documentation of data quality and quality assurance measures performed at the level of individual datasets is necessary ( Downs et al. 2021 ; Peng et al. 2022 ). Currently, the availability of data quality information is limited, whereas, ideally, it should be published in a machine-readable format, taking both researchers’ and service providers’ perspectives into account ( Assante et al. 2016 ). This could soon change, as the development of tools checking the compliance of data publications with the FAIR Principles facilitates certain aspects of data quality assessment, therefore making quality estimations more widely available ( Mangione, Candela & Castelli 2022 ). Some disciplines, like the earth sciences, are already taking steps towards making information on the quality of individual datasets visible ( Peng et al. 2022 ). A potential reason for the current lack of quality information overall might be the notion of repositories achieving pristine datasets through cleaning data. Plantin ( 2019 ) argues that to maintain this perception, repositories may choose to make interventions performed as part of the quality assurance process invisible to the outside. Repositories should also provide information on the quality assurance processes they generally apply ( Peer, Stephenson & Green 2014 ). Registries such as re3data record aspects of quality assurance measures that research data repositories perform ( Kindling et al. 2017 ). As mentioned above, certification might be an indicator that a repository conducts quality assurance, but this relationship has not yet been examined in detail.
Overall, there is a lack of systematic research into whether or how repositories share quality information, both on the repository and the dataset levels.
3 Methodology
This study aims at analysing aspects of data quality assurance at research data repositories indexed in re3data. Following an exploratory approach, it covers the scope of the repository, types of data quality assessment, quality criteria, responsibilities, details of the review process, and data quality information.
3.1 Survey design
The study was conducted as a personalised online survey with a combination of closed- and open-ended questions. Each participant received a personalised invitation link to the survey. The questionnaire’s design was based on the findings from qualitative analyses of (1) quality assurance measures described by repositories in CoreTrustSeal self-assessment documents and (2) guidelines of data journals ( Kindling & Strecker 2021 ). These preliminary studies identified a set of quality criteria and quality assurance measures for data publications applied by repositories and data journals.
Following a pretest among 11 repository operators and experts in the field, the questionnaire was restructured, questions were worded more clearly, and ambiguous terms were defined in explanatory texts. The final questionnaire comprised 24 questions; 21 questions were mandatory, and 3 were optional. Eleven questions were only displayed if the participant had selected certain answers in previous questions. To cover the diverse approaches to quality assurance more comprehensively, participants were frequently offered to choose the option ‘not applicable’ and invited to describe aspects not foreseen in the survey design in free-text fields. In total, 13 questions included free-text fields, and 4 were free-text only. Supplementary File 1: Appendix: Overview of Survey Questions provides an overview of the question and response types.
In the survey tool, each personalised invitation link was paired with a variable with the re3data ID of the repository, making it possible to combine survey results with re3data metadata in the analysis.
3.2 Survey administration
On October 13, 2020, contact information for all repositories indexed in re3data at the time (2674) was extracted from the elements repositoryContact and institutionContact . If the information was available for a repository, values from repositoryContact were used preferentially; otherwise, values from institutionContact were added. Additional contact information could be obtained from contact pages of some remaining repositories with English- or German-language websites. The list of contact information was updated after a newsletter was sent to the repositories. Where possible, alternative contact information for invalid email addresses was added. After this process, contact information was available for 1893 repositories as of January 29, 2021. Four additional repositories asked to be included after becoming aware of the survey. In total, 1897 repositories were invited. Invitations for the survey were sent out on May 18, 2021, followed by reminders on June 1 and June 7. The survey was closed on June 15, 2021.
3.3 Response
Of the 1897 repositories that were invited, 332 completed the questionnaire. For a population of 1897 at a confidence interval of 95%, the minimal sample size is 320. The sampling error with 332 responses is 4.89%. Therefore, the results of the survey can be considered robust.
As Figure 1 demonstrates, compared to all repositories indexed in re3data at the time (2674), disciplinary repositories are slightly under-represented and institutional repositories slightly over-represented in the sample. However, because all repository types are present in all combinations in the sample, the issue is not considered severe.
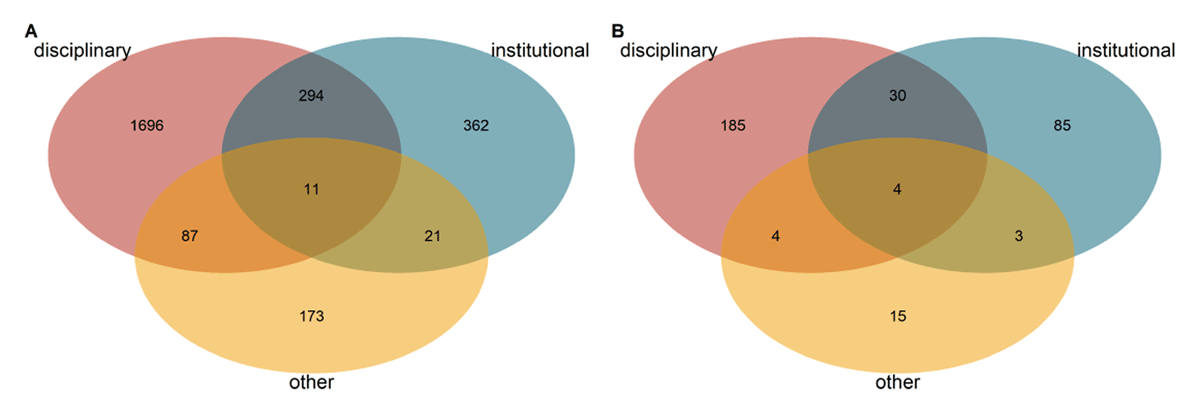
Types of all repositories indexed in re3data ( A ; NA: 30) and repositories included in the analysis ( B ; NA: 6).
3.4 Data processing
Prior to data analysis, incomplete responses were removed; 332 complete responses were included in the analysis.
Participants selected the variable Other 328 times for 14 questions. The analysis of corresponding free-text fields revealed that in 49 cases, the content of free-text fields specifying the selected variable Other matched one of the predefined variables. These variables were reassigned and, where applicable, replaced Other .
Survey data was supplemented by information on repository certification from a re3data database dump that was generated on April 22, 2021. The variable certification status is derived from the element certificate in the re3data metadata schema ( Strecker et al. 2021 ) and describes whether a repository has obtained any type of formal certification (53 in the sample), for example, from CoreTrustSeal. Differences across certification status were evaluated using chi-square ( χ 2 ) tests, and effect sizes are reported using Cramér’s V (V). After anonymisation, the data, codebook, and survey instrument were made openly available ( Kindling, Strecker & Wang 2022 ).
The following section outlines the findings of the analysis.
4.1 Scope of the repository
The repositories participating in the survey vary in scope, both in terms of the extent of the services they offer (Q01, N = 332, n = 568) and in terms of the types of data they hold (Q02, N = 332, n = 1471). Services are extended to the hosting institution (n = 193, 58.1%), other institutions or projects (n = 158, 47.6%), or to any source (n = 110, 33.1%). Some repositories aggregate metadata from other data providers (n = 86, 25.9%) On average, the repositories selected 5.4 types of data that fall within their scope. Among these data types, the most widespread are measured values (n = 146, 44%), images (n = 110, 33.1%), data from analysed sample material (n = 107, 32.2%), and databases (n = 107, 32.2%). Some participants state that the repository does not focus on a specific data type (n = 77, 23.2%).
Repositories apply different criteria to ensure a homogenous collection (Q03, N = 332, n = 805), for example, based on collection profiles or policies. Most repositories check whether data fit the scope of the repository in general (n = 237, 71.4%). Other criteria include that data passed formal assessment before deposit (n = 106, 31.9%), that data are described in a publicly accessible document (n = 93, 28%), and that data correspond to a peer-reviewed publication (n = 91, 27.4%). Some participants state that a collection policy is not applicable to the repository (n = 26, 7.8%). In the free-text field, additional criteria were listed, including technical suitability or data availability.
Repositories report offering a wide range of support services (Q04, N = 332, n = 1461). On average, repositories selected 4.8 distinct services. Most frequently, repositories offer direct, individualised support to data depositors (n = 244, 73.5%). Other common types of support services include data deposit guidelines (n = 208, 62.7%) and data format recommendations (n = 204, 61.4%). Some repositories state that support for data depositors is not applicable to the repository (n = 23, 6.9%). In the free-text field, guidelines for specific aspects of data curation (data protection, anonymisation, data management plans) were mentioned as additional types of support services.
4.2 Types of data quality assessment
The survey distinguished between formal assessment of data (Q05, N = 332, n = 332) and data review (Q10, N = 332, n = 332). Formal assessment refers to technical, administrative, and access-related aspects of data, whereas data review refers to the process by which experts, either from the hosting institution or from other institutions, evaluate the scientific quality of datasets.
As Figure 2 A shows, the majority of participants report that formal aspects of data are assessed at the repository (n = 207, 62.3%). Others do not conduct formal assessment (n = 65, 19.6%) or formal assessment is not applicable (n = 36, 10.8%). The analysis revealed no statistically significant relationship between the formal assessment of data and the certification status of a repository. About half (n = 171, 51.5%) of the responding repositories perform data review either for all (n = 105, 31.6%) or some (n = 66, 19.9%) datasets (see Figure 2 B ). About a quarter do not conduct data review (n = 90, 27.1%), and for others, data review is not applicable (n = 52, 15.7%). The association between performing data review and certification status of the repository is statistically significant ( χ 2 (3, N = 332) = 9.8, p = 0.02) but weak (V = 0.18).
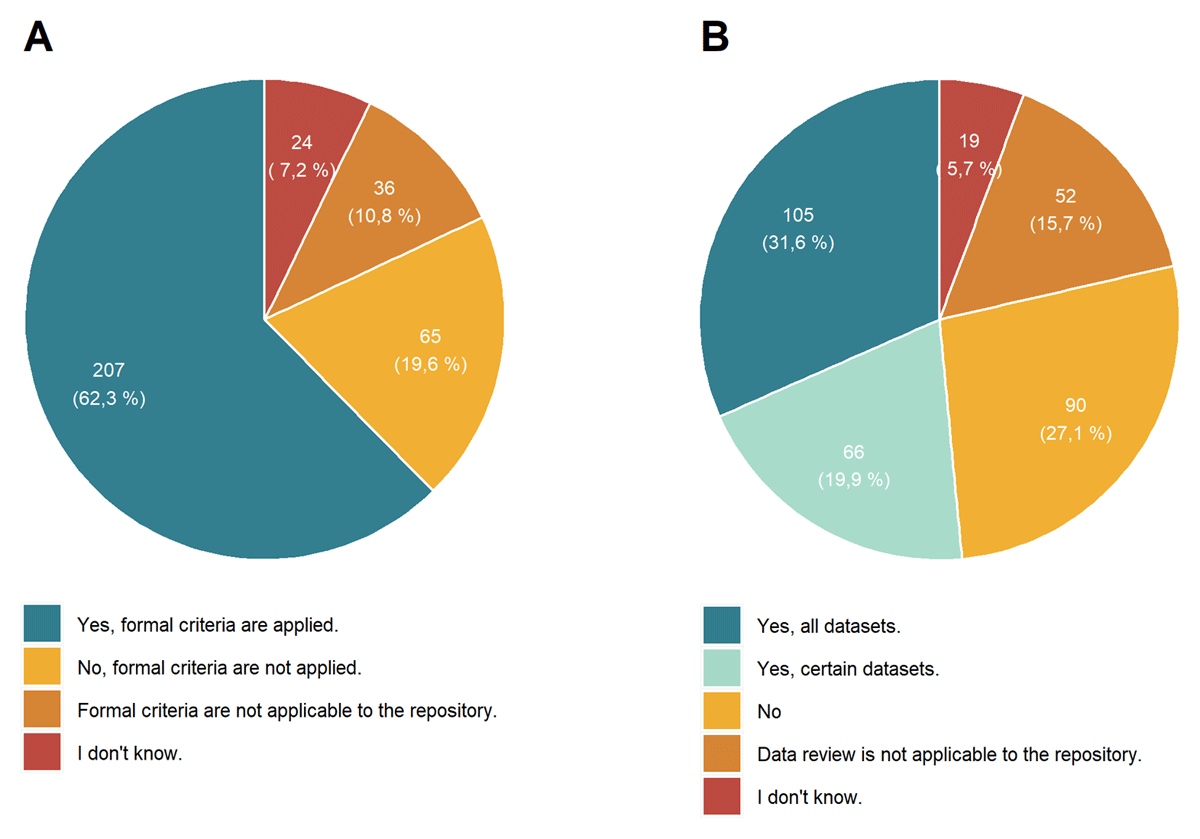
Question 05: Are formal criteria applied to data before publication? (A) ; Question 10: Are data reviewed beyond the application of formal criteria? (B) .
Overall, 22.9% (n = 76) of the responding repositories perform neither formal assessment nor review of data, whereas 77.1% (n = 256) conduct at least one type of data quality assessment. Of these, 134 perform either formal assessment (n = 85) or review of data (n = 49), and 122 perform both, as Figure 3 shows.
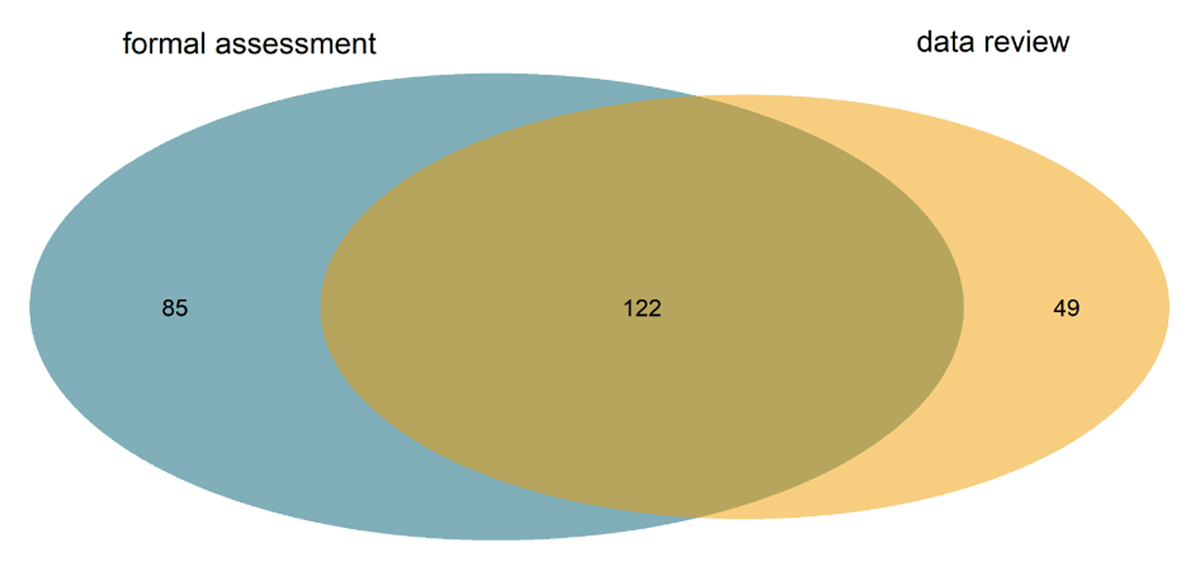
Types of data quality assessment performed at responding repositories.
4.3 Criteria for the formal assessment of data
Repositories that perform formal assessment of data were asked what criteria guide their process. Figure 4 shows the criteria repositories apply when assessing formal aspects of data (Q06, N = 207, n = 3519). Almost all respondents (n = 201, 97.1%) state that either the repository, the data provider, or both check for a basic description of data. Other widely applied criteria include the clarification of copyright and usage rights (n = 186, 89.9%), compliance with a metadata schema (n = 185, 89.4%), provision of provenance information (n = 184, 88.9%), and compliance with the FAIR Principles (n = 181, 87.5%). The criteria applied least frequently are that data pass statistical tests (n = 64, 30.9%) and the declaration of conflicts of interests (n = 72, 34.8%).
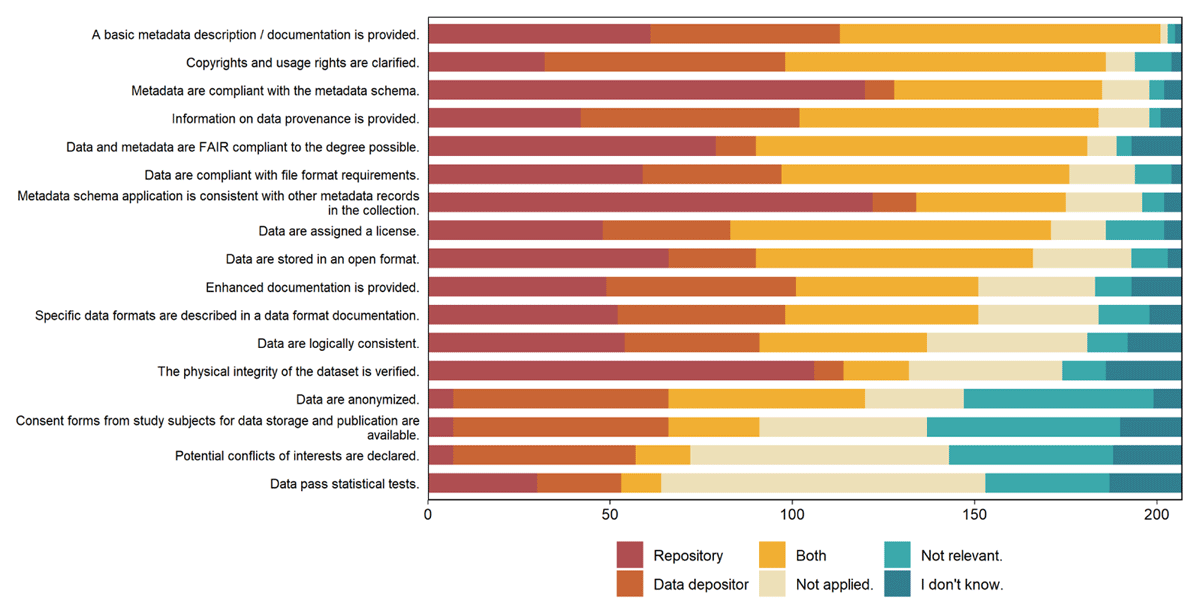
Question 06: Who is responsible for the assessment and curation according to the following formal criteria? (multiple choice).
Respondents added a variety of additional formal criteria in the subsequent free-text field (Q07, N = 63, n = 63). For example, responses mentioned the adherence to community standards, automatic quality checks, and fingerprinting.
4.4 Criteria for the review of data
The repositories performing data review were asked what criteria the process was based on. Figure 5 shows relevancy ratings of criteria for the review of data (Q11, N = 171, n = 3591). Most respondents (n = 163, 95.3%) state that the overall data and documentation quality is very relevant or relevant for data review at their repository. Other criteria that were commonly rated very relevant or relevant include appropriate data documentation (n = 155, 90.6%), suitability to the scope of the repository (n = 143, 83.6%), and accuracy (n = 135, 79%). The criteria rated very relevant or relevant least frequently are the novelty (n = 41, 23.9%) and timeliness (n = 58, 33.9%) of data.
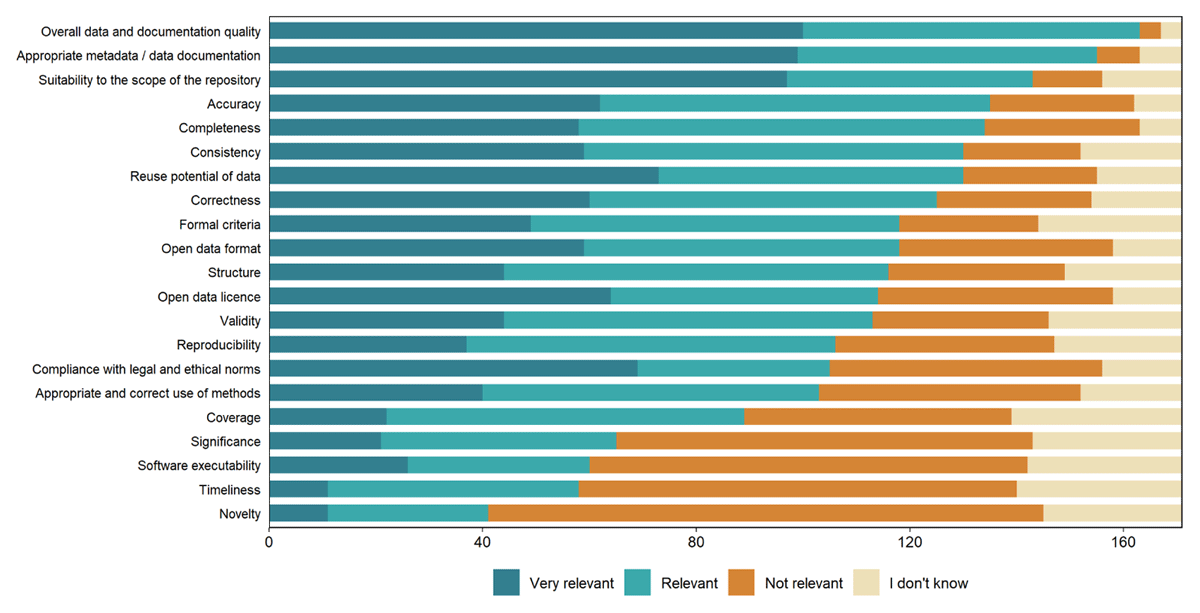
Question 11: How relevant are the following quality criteria for data review at your repository? (multiple choice).
Respondents were encouraged to list any additional criteria for review of data in a free-text field (Q12, N = 28, n = 28). Responses mentioned data anonymity and included laboratory protocols or references to corresponding publications.
4.5 Responsibility
A number of questions focused on identifying responsibilities for quality assurance activities. At the repositories that perform a formal assessment of data (Q08, N = 207, n = 518), the responsibility for the process mainly falls to the staff at the institution hosting the repository. Most respondents report that data curators (n = 137, 66.2%) or data managers (n = 109, 52.7%) at the hosting institution conduct the formal assessment, followed by technical administrators (n = 76, 36.7%) and subject experts (n = 75, 36.2%). Data providers are also regularly involved in this step of data quality assurance (n = 75, 36.2%). Subject experts from other institutions contribute to the formal review less frequently (n = 31, 15%). The process is rarely outsourced to external partners (n = 3, 1.4%). Overall, multiple different roles seem to be involved in assessing formal criteria: respondents selected up to 6 different roles, with an average of 2.5 roles per repository.
On a more detailed level, responsibilities for the formal assessment of data vary across criteria (Q06, N = 207, n = 3519). Besides the general application of criteria for the formal assessment of data, Figure 4 also shows who is responsible for applying these criteria. These results show that the application of some criteria is seen more within the responsibility of either the data depositor or the repository. For example, providing enhanced documentation, anonymising data, making consent forms available, and the declaration of potential conflicts of interest are mainly the responsibility of the data provider. On the other hand, repositories are mainly responsible for ensuring that metadata are compliant with a metadata schema and that the metadata schema application is consistent with other metadata records in the collection and for verifying the physical integrity of datasets. The application of other criteria appears to be a shared responsibility of data depositor and repository, including ensuring a basic description of data, clarifying copyrights and usage rights, providing information on data provenance, seeking compliance with the FAIR Principles or file format requirements, assigning licences, and storing data in open formats.
Similar to the formal assessment of data, the institution hosting the repository mainly assumes responsibilities for data review (Q13, N = 171, n = 435). Most respondents report that data curators (n = 116, 67.8%) or data managers (n = 101, 59.1%) at the hosting institution review data, followed by subject experts (n = 69, 40.4%) and technical administrators (n = 52, 30.4%). Data providers are also regularly (n = 52, 30.4%) involved in reviewing data. Subject experts from other institutions contribute to the process less frequently (n = 33, 19.3%), and it is rarely outsourced to external partners (n = 2, 1.1%). Several roles within a repository tend to contribute to data review: respondents selected up to six different roles, with an average of 2.5 roles per repository. Responses in the free-text field listed additional responsibility mechanisms, including the responsibility of data depositors, the peer review process of journals, and community review of data.
4.6 Data review process
The survey covered a number of aspects of the data review process, including the openness of the process, the acknowledgement of reviewers, and the consequences of data not meeting quality expectations.
Open processes for reviewing data are not common (Q14, N = 171, n = 171). Only a few repositories offer an open process for data review (n = 18, 10.5%). The majority of repositories do not conduct open review of datasets (n = 147, 86%). Some respondents specified details of the open review process in the free-text field, for example, descriptions of community review or references to the review process at the journals of corresponding text publications.
Overall, the recognition of reviewers is rare (Q15, N = 171, n = 175). Most repositories have not implemented measures to acknowledge reviewers (n = 99, 57.9%). Some repositories publish reviewers’ names (n = 19, 11.1%), and a few compensate reviewers by paying them a fee per review (n = 3, 1.8%) or a fixed fee rate (n = 2, 1.2%). Some responses in the free-text field indicated that data review is considered a standard task of repository staff. Other respondents mentioned co-authorship or appreciative emails.
Final decisions on publishing data after the data review process is concluded (Q17, N = 171, n = 245), given that the data depositor agrees, are frequently made by repository staff (n = 132, 77.2%). In other cases, the decision is made by the data depositor (n = 57, 33.3%) or collection editor (n = 33, 19.3%). Responses in the free-text field reflect the diversity of approaches to data review. They name […] being responsible.
Most repositories would consider taking additional steps if submitted datasets do not meet quality expectations (Q18, N = 332, n = 483). Most repositories state that data and metadata are revised until they fulfil required criteria (n = 216, 65.1%); others would consider rejecting data deposit (n = 110, 33.1%). Quality reports are published alongside datasets at some repositories (n = 37, 11.1%), and others recommend alternative repositories (n = 33, 9.9%). Some respondents (n = 58, 17.5%) report that the scenario is not applicable to the repository. In the free-text field, some respondents stressed the responsibility of the data depositor for ensuring data quality. In this self-deposit model, datasets that do not meet quality criteria might still be published.
Of the repositories that would consider rejecting data deposit, some provided an estimation of the rate of rejected datasets in the last two years (Q19, N = 117, n = 117). On average, the respondents reported a rejection rate of 8.2% (see Figure 6 ). The median rejection rate is 3%. Six repositories reached or surpassed a rejection rate of 50%, and one respondent stated that 70% of datasets offered to the repository were rejected. Thirty-one respondents reported rejection rates of 0%.
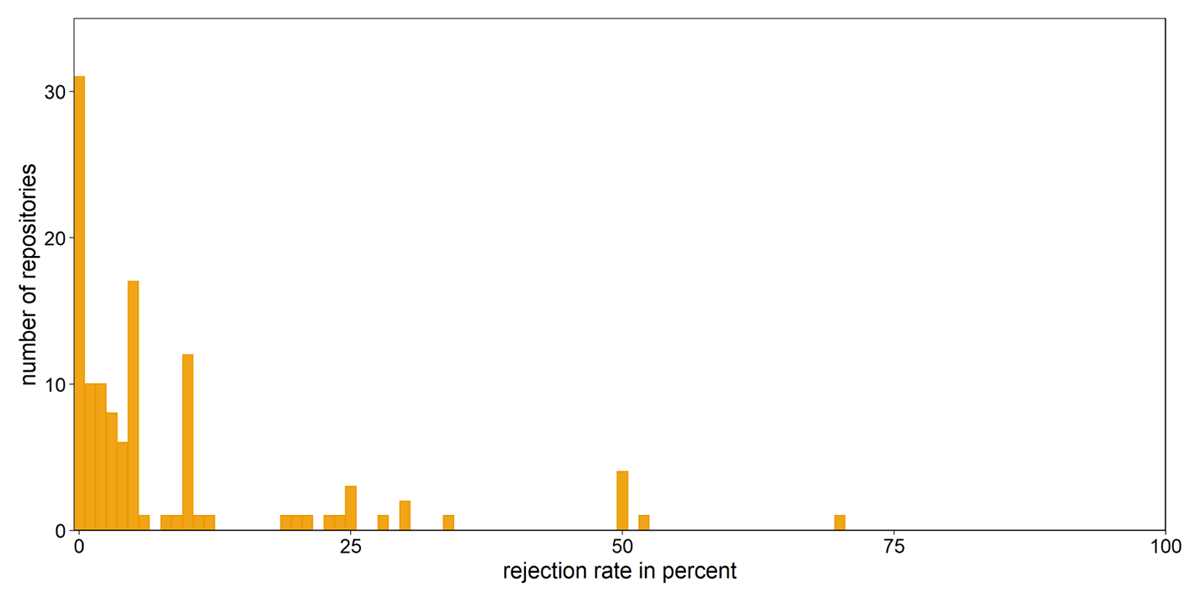
Question 19: What (estimated) ratio of datasets were rejected by your repository in the last two years?
Repositories were offered the opportunity to share any additional thoughts on quality assurance at research data repositories (Q23, N = 84, n = 84). Some respondents emphasised the effort that quality assurance entails and the need for adequate recognition. Other comments described the dependence of quality assurance on various aspects, such as the scope of the repository or data types. Several respondents indicated that there are plans for developing or expanding quality assurance measures and workflows at the repository.
Overall, there is no significant association between the certification status of a repository and the aspects of the review process reported in this section.
4.7 Documenting and publishing results
A series of questions addressed aspects of data quality information. One question focused on the documentation of the formal assessment of data (Q09, N = 207, n = 207). Only a few repositories make results of this process public (n = 27, 13%). Most respondents state that their repository does not publish the results of formal assessment (n = 154, 74.4%), even though it is documented at almost half of the responding repositories (n = 96, 46.4%). In the free-text field, some respondents stated that they consider the documentation of the review obsolete once data is published. The association between documenting the process of formal assessment of data and certification status of the repository is statistically significant ( χ 2 (2, N = 207) = 6.4, p = 0.041) but weak (V = 0.19).
A similar pattern emerged for sharing the results of reviewing data (Q16, N = 171, n = 171). Results are frequently shared with the data depositor (n = 108, 63.2%), but only a few repositories publish a review report alongside the data (n = 9, 5.3%). In the free-text field, some respondents described that review reports are mainly shared internally to facilitate the review process. Others stated that review reports become obsolete with the elimination of quality issues, and subsequent data publication is not shared for this reason. The analysis found no significant relationship between sharing results of data review and the certification status of a repository.
Overall, only 9.3% (n = 31) of the responding repositories publish results of any data quality assurance process. Of these, most (n = 22) publish results of formal assessment only, as Figure 7 shows. Few repositories share results of only data review (n = 4) or of both types of quality assessment (n = 5).
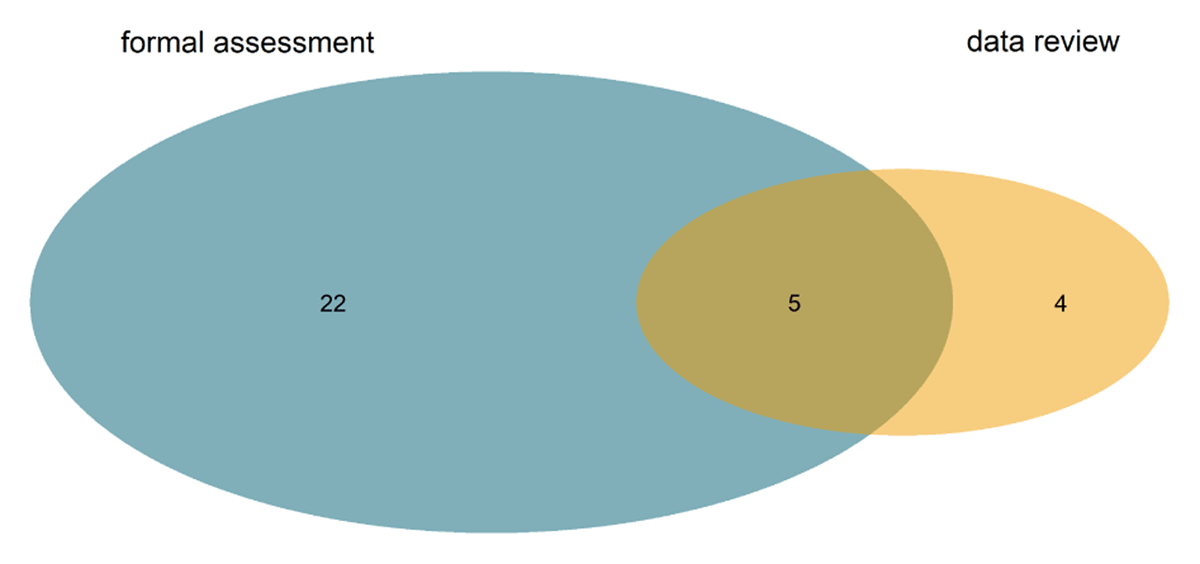
Publication of results of data quality assurance processes at responding repositories.
Repository users are rarely involved in the evaluation of data (Q20, N = 322, n = 387); about a third of participating repositories do not include them (n = 115, 34.6%). Several repositories receive textual feedback. Most do not make this information publicly available (n = 111, 33.4%), but some do (n = 22, 6.6%), for example, in the form of comments. Others conduct user surveys (n = 35, 10.5%) or offer data rating (n = 5, 1.5%). The involvement of repository users in data evaluation is not applicable to some repositories (n = 77, 23.2%). Responses in the free-text field detail a variety of approaches to involving repository users: for example, by organising workshops, enabling the reporting of errors in the data, or documenting conversations with colleagues about datasets at conferences.
Repositories adopt different strategies for communicating indicators of data quality to repository users (Q21, N = 322, n = 803). Most repositories use one or more indicators to communicate aspects of data quality. Most commonly, references to corresponding publications are added (n = 232, 69.9%). Other repositories display data versions (n = 169, 50.9%) or usage statistics (n = 119, 35.8%). Some repositories include data quality information in metadata (n = 88, 26.5%), display data-related citations (n = 72, 21.7%) or quality badges (n = 56, 16.9%). Less common is the publication of user survey results (n = 7, 2.1%) or data ratings (n = 5, 1.5%). Thirty-three (9.9%) respondents stated that public indicators of data quality are not applicable to the repository. Some responses in the free-text field described data quality reports, descriptions of characteristics and limitations that complement published datasets at these repositories.
5 Discussion
5.1 variety of data quality assurance measures.
The survey showed that approaches to and the maturity of quality assurance measures for data repositories are diverse. While most responding repositories perform a form of data quality assurance, not all assume quality assurance responsibilities. Some repositories state that they follow a self-deposit model, where data depositors are responsible for quality assurance.
Some repositories have already put in place a variety of data quality assurance measures. At these repositories, there is some indication of clear workflows, for example, support for data depositors in the form of guidelines or checklists or revisions or rejections if (meta)data of insufficient quality are submitted. Some data quality assurance practices seem to be very common; for example, some criteria for formal assessment and review of data are widely used.
The processes of data quality assurance conducted at repositories are not uniform. The formal assessment and review of data appear to be largely independent processes—repositories do not necessarily perform both. The survey demonstrated that not all measures for ensuring data quality are relevant for all research data repositories. Throughout the survey, some respondents indicated that certain quality assurance measures are not applicable to their repository or mentioned additional quality assurance measures in free-text fields. This suggests that there is no universal approach to quality assurance but that repositories implement measures depending on scope and context.
The survey confirmed that data quality assurance at research data repositories is multifaceted and comprises a variety of activities. Based on the survey results, a framework of data quality assurance at repositories is being developed, which is intended to serve as a theoretical foundation for approaches to quality assurance of data publications at research data repositories ( Kindling et al. 2022 ).
5.2 Responsibilities and the role of repositories in supporting DATA quality
The survey revealed that repositories significantly contribute to data quality, which is demonstrated, for example, by the surprisingly high rejection rates. Many repositories have implemented quality assurance measures, with repository staff assuming essential responsibilities. Responsibilities for data quality assurance are often organised based on a division of labour, as the number of roles involved in the formal assessment and review of data per repository shows. At some repositories, staff with very different backgrounds and skills are involved in quality assurance. These examples challenge the idea that quality assurance at repositories is based on data curators conducting formal assessment and subject specialists being responsible for data review. This raises questions about a clear separation between formal assessment and review of data, which is discussed in more detail in Section 5.4.
Several respondents emphasised the effort data quality assurance entails, yet adequate recognition is still lacking. Initiatives to properly acknowledge the contributions to quality assurance could remedy this imbalance, thereby making research data quality assurance in general and the impact of research data repositories in particular more visible.
The survey demonstrated how multifaceted data quality is. Repositories cannot be realistically expected to apply the full spectrum of quality assurance measures. Instead, repositories need to have a clear understanding of data quality assurance measures they can offer, informed by their mission, scope, and user base.
5.3 Path dependence
The survey confirms a path dependence of data review on the review process of text publications. Overall, the review of data appears to follow established processes for reviewing text publications. So far, few repositories have implemented an open review process, and forms of post-publication data review by inviting public feedback on datasets from repository users are still rare. The evaluation of data is often connected to corresponding text publications; for example, data corresponding to a peer-reviewed publication is a common factor for including datasets in the collection of a repository. The most widely used indicator for data quality is a link to the corresponding publication. Data quality assurance at research data repositories also faces similar challenges to the review processes for text publications; for example, despite their valuable contributions, reviewers are often not acknowledged.
The survey also sheds light on friction in implementing data review processes modelled after peer review for text publications. Most importantly, responsibilities for both formal assessment of data and data review currently lie almost exclusively with the institution hosting the repository. Outsourcing of data quality assurance is very rare. The strong reliance on repository staff for data review might raise questions about the independence of the peer review process, as outlined by Lawrence et al. ( 2011 ), for data archives.
Responses to free-text fields indicate that some repositories consider data review a standard task of repository staff. These expectations demand a lot of resources at the hosting institution and might not match the reality. In addition, a data review process that mainly relies on repository staff can be time-consuming and slow, potentially delaying the data publication process.
Repositories and other stakeholders should reconsider whether it is useful to emulate aspects of the review process of text publications, and if other mechanisms, such as post-publication user feedback, can be implemented.
5.4 No clear distinction between formal assessment and data review
The survey indicated that some assumptions described in the literature do not apply to all repositories. For example, it challenges a clear distinction between the formal assessment and review of data. Contrary to descriptions sometimes found in the literature, the survey demonstrates that data curators and managers do not have a clear focus on formal assessment and subject experts are not mainly responsible for data review. Instead, both roles tend to share responsibility for both tasks.
A clear chronological sequence of quality assurance measures, from an initial assessment before the ingest phase to the assessment of formal criteria to data review, was already questioned in the survey pretest. Some repositories reported that they perform data review before ingest, for example, in the context of research projects where repositories assisted in the management of data. Quality assurance should not be conceptualised as a linear process with distinct phases but should be adapted to the respective context and needs.
5.5 No clear distinction between data quality and metadata quality
The survey confirms that data and metadata quality are enmeshed; there is no clear distinction between the concepts. The most widely applied criteria for both formal assessment and review of data are related to metadata or data documentation. This observation matches the fact that repositories traditionally have a strong focus on metadata, as metadata underpin essential repository functions, for example, dataset search and retrieval. The results suggest that repositories might be entering into data quality assurance, which might be a relatively new task for some services, by addressing metadata quality first, an area in which they have a lot of experience. Criteria related to metadata quality are also likely more common because they are easier to measure.
Overall, implementing quality criteria related more clearly to data (as opposed to metadata) might require a more mature service because these tasks go beyond traditional repository responsibilities. Further research could explore how repositories assess data-related quality criteria and whether these approaches can be generalised to fit other repositories.
5.6 Lack of data quality information
Only about a quarter of participating repositories publish results of the formal assessment or review of data alongside the dataset. This is surprising because a lot of repositories (1) conduct data quality assessment and (2) internally document aspects of these processes.
The survey revealed that repositories seem to be more willing to share quality information if issues with data remain after publication: the number of respondents stating that their repository would consider publishing a quality report alongside a dataset if it did not fully meet quality standards is higher than the number of repositories publishing results of formal assessment and/or review of data. Responses in the free-text fields indicate that some repositories question the necessity of providing data quality information once quality issues are resolved. The discussion about if and when data quality information should be shared has far-reaching implications: for example, in the context of making repositories’ successful contributions to data quality visible, tracking data provenance, and other Open Science principles. These questions should therefore be explored further.
The survey revealed that, at the moment, most repositories do not make feedback from data (re-)users public, for example, in the form of comments or ratings. Although public feedback could support decision making by potential data (re-)users, repositories would also need to take steps to prevent potential misuse.
Overall, it is not clear what format is most suitable for reporting data quality information. For example, consistent practices have not yet been established, and not all metadata schemas support reporting data quality information in structured metadata. More research in this area is required to make data quality information more widely available.
5.7 Weak association between formal certification and data quality assurance
Combining survey data with re3data metadata made it possible to analyse the association between formal certification and data quality assurance. Overall, the data revealed only two statistically significant associations with formal certification—performing data review and documenting the formal assessment of data—and these associations were weak. These results suggest that data quality assurance and formal repository certification are largely independent. The survey could not confirm that the certification status of a repository is a clear indicator of whether a repository conducts in-depth quality assurance measures.
The main reason for this is likely that most available repository certificates do not focus on data quality assurance specifically. For example, the goal of CoreTrustSeal certification is to evaluate repositories based on a core set of requirements intended to reflect sustainability and trustworthiness of the service, making certification more widely available. Although it is not the primary focus in CoreTrustSeal, data quality is addressed in several of these requirements, most notably in Requirement 11: ‘The repository has appropriate expertise to address technical data and metadata quality and ensures that sufficient information is available for end users to make quality-related evaluations’ ( CTS 2019: 15 ).
A successful application for CoreTrustSeal certification therefore requires repositories to conduct some level of quality assessment and documentation of data processing and curation, but CoreTrustSeal does not stipulate exact data quality assurance measures that must be performed. That would not be reasonable for a certificate that evaluates manifold aspects of repository operations as well as a broad spectrum of repository types; as outlined above, approaches to quality assurance are not uniform and depend on the scope and context of the individual repository. Survey results reflect this: all but two of the participating CoreTrustSeal-certified repositories (n = 33, 94.3%) conduct some form of data quality assessment—either formal assessment, review of data, or both. However, statistical analysis revealed no strong associations between specific measures of data quality assurance and CoreTrustSeal certification.
These observations could start further reflections on ways to effectively communicate information on the quality assurance measures a repository performs: who might be interested in this information and what entities besides certification organisations might deliver it. Certificates like CoreTrustSeal could try to cover data quality assurance more thoroughly, but that might be difficult given the current lack of consensus on the topic in the repository community.
At the level of individual datasets, there are more suitable indicators for signalling data quality to repository users—for example, badges or ratings—but they are not yet widely adopted. Initiatives for measuring the FAIR compliance of datasets might change this by making indicators more widely available. More research is necessary to study the prevalence of these quality indicators and their usefulness for repository users.
6 Conclusion
The survey demonstrated that quality assurance at research data repositories is multifaceted and nonlinear. Although there are some common patterns, individual approaches to ensuring data quality are diverse. In the context of research data, data quality and metadata quality are enmeshed and cannot be clearly separated.
Research data repositories significantly contribute to data quality. However, data quality assurance sets high expectations for repositories and requires a lot of resources. These challenges need to be addressed, for example, by critically assessing the path dependence of data review on review processes for text publications. Other approaches might be more suitable to ensuring the quality of data and should be explored further, for example, involvement of repository users in the form of post-publication data review.
Information on results of the formal assessment and review of individual datasets is not yet widely available. Approaches to publishing data quality information should be explored—for the benefit of repository users, for making the labour of data review visible, and for fostering the recognition of data publications as scientific records. How this information can be captured and exposed in a meaningful way needs to be discussed.
Similarly, information on data quality assurance measures repositories perform is currently lacking. The analysis has demonstrated that the certification status of a repository is not a clear indicator of whether it conducts in-depth data quality assurance measures. The project re3data COREF is currently evaluating how information on data quality assurance measures can be described in registries at the repository level.
Overall, a deeper understanding of data quality assurance at research data repositories can lead to a better recognition of efforts and allocation of resources.
Although this paper constitutes the first systematic and comprehensive survey of quality assurance practices at research data repositories, more research is needed to capture individual approaches to data quality assurance.
6.1 Limitations
The qualitative studies conducted before the survey identified a variety of quality criteria and quality assurance measures applied by repositories and data journals. In contrast, a questionnaire limits the number of possible responses. To obtain structured statements about a large number of repositories and at the same time capture the diversity of individual approaches, the questionnaire was supplemented by free-text fields.
The scope of the questionnaire was limited to capturing the status quo of quality assurance measures at research data repositories. Therefore, this study neither evaluates the success of these measures nor takes into account future plans.
Although the survey was distributed to a large number of repositories as possible, the results still represent a convenience sample of repositories listed in re3data. As a result, there might be a self-selection bias towards repositories already performing data quality assurance measures. Data quality assurance might also be considered a sensitive subject; therefore, some repositories may have been hesitant to participate. This issue was addressed by assuring participants of full anonymity in the survey invitation and in the privacy statement. However, it is possible that repositories where quality assurance is not applicable or not feasible might be under-represented in this paper.
Additional File
The additional file for this article can be found as follows:
Overview of Survey Questions. DOI: https://doi.org/10.5334/dsj-2022-018.s1
Acknowledgements
We would like to thank the repository community for participating in the survey and the valuable contributions to the pretest. The survey was part of a PhD project on quality assurance of research data publications at the Berlin School of Library and Information Science, Humboldt-Universität zu Berlin, and the project re3data COREF. We would like to thank all project members for their feedback and Yi Wang in particular for her valuable support in survey administration and data analysis.
Funding Information
re3data COREF is a joint project by DataCite, Helmholtz Open Science Office, Humboldt-Universität zu Berlin, and KIT Library. The project is funded by the German Research Foundation (DFG) under the award number 422587133.
Competing Interests
The authors have no competing interests to declare.
Assante, M, et al. 2016. Are scientific data repositories coping with research data publishing? Data Science Journal , 15(6). DOI: https://doi.org/10.5334/dsj-2016-006
Austin, C, et al. 2016. Research data repositories: Review of current features, gap analysis, and recommendations for minimum requirements. IASSIST Quarterly , 39(4): 24. DOI: https://doi.org/10.29173/iq904
Austin, C, et al. 2017. Key components of data publishing: Using current best practices to develop a reference model for data publishing. International Journal on Digital Libraries , 18(2): 77–92. DOI: https://doi.org/10.1007/s00799-016-0178-2
Batini, C and Scannapieco, M. 2016. Data quality dimensions. In: Batini, C and Scannapieco, M (eds.), Data and information quality: Dimensions, principles and techniques . Berlin: Springer. pp. 21–51. DOI: https://doi.org/10.1007/978-3-319-24106-7_2
Cai, L and Zhu, Y. 2015. The challenges of data quality and data quality assessment in the big data era. Data Science Journal , 14(2). DOI: https://doi.org/10.5334/dsj-2015-002
Canali, S. 2020. Towards a contextual approach to data quality. Data , 5(4): 90. DOI: https://doi.org/10.3390/data5040090
CASRAI (Consortia Advancing Standards in Research Administration Information). 2022a. Curation. Available at https://casrai.org/term/curation/ [Last accessed 30 August 2022].
CASRAI (Consortia Advancing Standards in Research Administration Information). 2022b. Data quality. Available at https://casrai.org/term/data-quality/ [Last accessed 30 August 2022].
CTS (CoreTrustSeal Standards and Certification Board). 2019. CoreTrustSeal trustworthy data repositories requirements 2020–2022. DOI: https://doi.org/10.5281/zenodo.3638211
Downs, R, et al. 2021. Perspectives on citizen science data quality. Frontiers in Climate , 3. DOI: https://doi.org/10.3389/fclim.2021.615032
ISO (International Organization for Standardization). 2015. Quality management systems—Fundamentals and vocabulary (ISO 9000:2015). Available at https://www.iso.org/standard/45481.html [Last accessed 30 August 2022].
Johnston, L, et al. 2018. How important are data curation activities to researchers? Gaps and opportunities for academic libraries. Journal of Librarianship and Scholarly Communication , 6(1). DOI: https://doi.org/10.7710/2162-3309.2198
Juran, JM. 1951. Quality-control handbook . New York: McGraw-Hill.
Kindling, M and Strecker, D. 2021. How to ensure ‘good’ data? A presentation at Open Repositories 2021. Available at https://coref.project.re3data.org/blog/how-to-ensure-good-data-a-presentation-at-open-repositories-2021 [Last accessed 30 August 2022].
Kindling, M, Strecker, D and Wang, Y. 2022. Data quality assurance at research data repositories: Survey data (1.0) [data set]. Zenodo . DOI: https://doi.org/10.5281/ZENODO.6457849
Kindling, M, et al. 2017. The landscape of research data repositories in 2015: A re3data analysis. D-Lib Magazine , 23(3/4). DOI: https://doi.org/10.1045/march2017-kindling
Kindling, M, et al. 2022. Data quality assurance at research data repositories—Results from a survey. In: International Digital Curation Conference on 13–16 June 2022. DOI: https://doi.org/10.5281/ZENODO.6638409
Koltay, T. 2020. Quality of open research data: Values, convergences and governance. Information , 11(4): 175. DOI: https://doi.org/10.3390/info11040175
Koshoffer, A, et al. 2018. Giving datasets context: A comparison study of institutional repositories that apply varying degrees of curation. International Journal of Digital Curation , 13(1): 15–34. DOI: https://doi.org/10.2218/ijdc.v13i1.632
Lafia, S, et al. 2021. Leveraging machine learning to detect data curation activities. In: IEEE 17th International Conference on eScience, Innsbruck, Austria, 20–23 September 2021. DOI: https://doi.org/10.1109/eScience51609.2021.00025
Lawrence, B, et al. 2011. Citation and peer review of data: Moving towards formal data publication. International Journal of Digital Curation , 6(2). DOI: https://doi.org/10.2218/ijdc.v6i2.205
Lee, DJ and Stvilia, B. 2017. Practices of research data curation in institutional repositories: A qualitative view from repository staff. PLoS ONE : e0173987. DOI: https://doi.org/10.1371/journal.pone.0173987
Lee, Y, et al. 2002. AIMQ: A methodology for information quality assessment. Information & Management , 40(2): 133–146. DOI: https://doi.org/10.1016/S0378-7206(02)00043-5
Madnick, S, et al. 2009. Overview and framework for data and information quality research. Journal of Data and Information Quality , 1(1): 1–22. DOI: https://doi.org/10.1145/1515693.1516680
Mangione, D, Candela, L and Castelli, D. 2022. A taxonomy of tools and approaches for FAIRification. In: CEUR Workshop Proceedings, Padova, Italy, 24–25 February 2022. Available at http://ircdl2022.dei.unipd.it/downloads/papers/IRCDL_2022_paper_18.pdf [Last accessed 30 August 2022].
Mayernik, M, et al. 2015. Peer review of datasets: When, why, and how. Bulletin of the American Meteorological Society , 96(2): 191–201. DOI: https://doi.org/10.1175/BAMS-D-13-00083.1
Merriam-Webster. 2022. Quality. Available at https://www.merriam-webster.com/dictionary/quality [Last accessed 30 August 2022].
OKF (Open Knowledge Foundation). n.d. Open Definition: Version 2.1. Available at http://opendefinition.org/ .
Palmer, C, Weber, N and Cragin, M. 2011. The analytic potential of scientific data: Understanding re-use value. Proceedings of the American Society for Information Science and Technology , 48(1): 1–10. DOI: https://doi.org/10.1002/meet.2011.14504801174
Parr, C, et al. 2019. A discussion of value metrics for data repositories in earth and environmental sciences. Data Science Journal , 18: 58. DOI: https://doi.org/10.5334/dsj-2019-058
Parsons, M and Fox, P. 2013. Is data publication the right metaphor? Data Science Journal , 12: WDS32–WDS46. DOI: https://doi.org/10.2481/dsj.WDS-042
Peer, L, Stephenson, E and Green, A. 2014. Committing to data quality review. International Journal of Digital Curation , 9(1): 263–291. DOI: https://doi.org/10.2218/ijdc.v9i1.317
Peng, G, et al. 2015. A unified framework for measuring stewardship practices applied to digital environmental datasets. Data Science Journal , 13: 231–253. DOI: https://doi.org/10.2481/dsj.14-049
Peng, G, et al. 2022. Global community guidelines for documenting, sharing, and reusing quality information of individual digital datasets. Data Science Journal , 21: 8. DOI: https://doi.org/10.5334/dsj-2022-008
Plantin, J -C. 2019. Data cleaners for pristine datasets: Visibility and invisibility of data processors in social science. Science, Technology, & Human Values , 44(1): 52–73. DOI: https://doi.org/10.1177/0162243918781268
RfII (German Council for Scientific Information Infrastructures). 2020. The data quality challenge: Recommendations for sustainable research in the digital turn. Göttingen. Available at https://nbn-resolving.org/urn:nbn:de:101:1-2020041412321918717265
Strecker, D, et al. 2021. Metadata schema for the description of research data repositories: Version 3.1. DOI: https://doi.org/10.48440/re3.010
Trisovic, A, et al. 2021. Repository approaches to improving the quality of shared data and code. Data , 6(2): 15. DOI: https://doi.org/10.3390/data6020015
Wang, R and Strong, D. 1996. Beyond accuracy: What data quality means to data consumers. Journal of Management Information Systems , 12(4): 5–33. DOI: https://doi.org/10.1080/07421222.1996.11518099
Wilkinson, M, et al. 2016. The FAIR Guiding Principles for scientific data management and stewardship. Scientific Data , 3(1): 160018. DOI: https://doi.org/10.1038/sdata.2016.18
Quality Assurance in Research
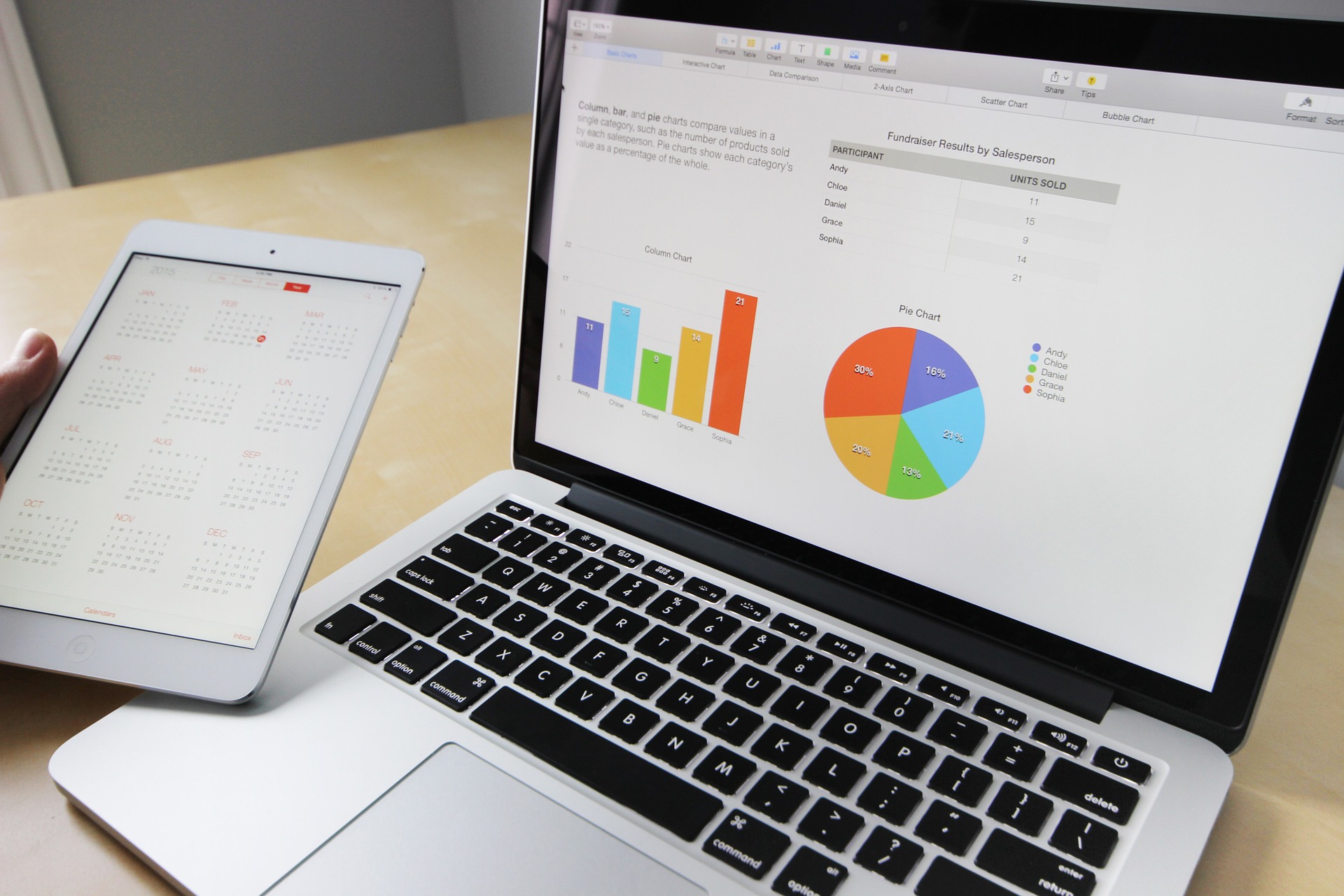
In research contexts, quality assurance (QA) refers to strategies and policies for ensuring that data integrity, quality, and reliability are maintained at every stage of the project. This includes strategies for preventing errors from entering the datasets, taking precautions before data is collected, and establishing procedures while data is used in a study.
Quality assurance is important for many reasons. The most obvious is that the whole point of research projects is to produce reliable data that yield rigorous and reproducible research results. There are other important factors as well. Internal Review Boards (IRBs), funding agencies, and other organizations that oversee research activity often require quality assurance procedures be implemented into project workflows to ensure that all policies are followed and that disbursed funds are going to well-organized and executed projects. There are also compliance issues in which research projects must be able to establish that data collection and analysis followed all protocols for human and animal subjects, privacy rules and regulations such as HIPAA and FERPA, and other safeguards that guarantee research projects are conducted in a responsible manner. In some instances, administrative audits are conducted to evaluate your project’s quality assurance and policy compliance.
Having quality assurance practices in place helps your project compliance and also helps evaluating your own research and data management practices to produce the best results possible.
Here are some steps you can take to promote quality assurance in your research:
Establishing clear data normalization protocols: Normalizing the data you record can have substantial impacts in all aspects of your research project. Normalizing means standardizing all the features and categories of data so that everyone working on the project has a clear sense for how to record it as it’s collected. Planning ahead and having clearly defined protocols for data collection before beginning the collection process means that all data that is part of the project adheres to the same standards.
Using consistent data formats and measurement standards: Using consistent format and measurement standards is part of the normalization process, and often you can find controlled vocabularies or ontologies that will provide established structural and definitional guidelines for your data based on your discipline. This will result in consistency in your data, not only within your own project, but also for others who may want to use it later on for further analysis or evaluation.
Rigorous data handling and analysis procedures: This is one of the most crucial components of quality assurance because data collection introduces significant opportunities for human error to undermine the integrity of data. At every stage of data collection in which a researcher records, transforms, or analyzes data, there is the potential for simple mistakes. Identifying those stages in data collection where errors are more likely to occur, and putting preventative measures in place can minimize those errors. Simple things such as establishing data and measurement formats can help, but also the tools you select for data collection can have significant impacts.
Select data collection and storage tools that promote data consistency: Spreadsheets for instance, are notorious for making it easy for errors to occur in data collection because they offer few controls on how it’s entered. Other tools such as databases or fillable forms provide features that allow you to control how data is entered. If you have a large team of researchers collecting data from the field or in varying contexts it’s easy for inconsistencies to arise. If the tools the researchers are using require consistency, you can be more successful at maintaining data integrity at every stage of handling data.
Metadata documenting how data was collected, recorded, and processed: Documenting how your data was handled throughout your project is good practice for a host of reasons, and it’s particularly helpful for maintaining data integrity. Making your data handling procedures explicit and formalized in the way metadata demands requires, first, that you consider these issues carefully. It also clarifies any ambiguities in your workflow so that a researcher during the project or making use of your research outputs at a later date could identify when the data is correct and where errors may have occurred.
Research staff training: Perhaps the most important thing you can do to produce consistent and reliable data is to make sure everyone working on the research project, from seasoned researchers to graduate and undergraduate project team members, have proper training in all the data collection and analysis procedures. Having everyone on the same page means that you can be confident that each person working on the project knows how their data handling tasks contribute to the overall project’s data quality goals.
Want to learn more about this topic? Check out the following resources:
The UK Data Service provides detailed information on establishing quality assurance strategies in your research.
DataOne provides guidance on how to craft a quality assurance plan that will allow you to “think systematically” as you put these protocols in place.
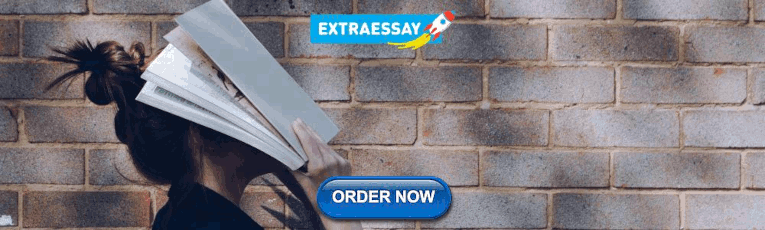
COMMENTS
The paper is also an attempt to initiate research for the emerging 2030 agenda for QM, here referred to as 'Quality 2030'. This article is based on extensive data gathered during a workshop process conducted in two main steps: (1) a collaborative brainstorming workshop with 22 researchers and practitioners (spring 2019) and (2) an ...
The paper examines the potential of agile methodologies in improving quality assurance in the process industry. The paper presents and explains quality management and assurance processes, key ...
The researchers revealed from the interviews that there is total absence of this practice which is directed in national guidelines and tools for Quality Assurance Scheme (QAS) for medical colleges of Bangladesh. Bangladesh Journal of Medical Education Vol.13 (1) January 2022: 33-39. Download Full-text.
Since publication in 2003 of a review 'Internal quality control: planning and implementation strategies,' 1 quality control (QC) has evolved as part of a comprehensive quality management system (QMS). The language of quality today is defined by International Standard Organization (ISO) in an effort to standardize terminology and quality management practices for world-wide applications.
Even during wars and Conflicts, quality assurance is essential [9]. The significance of quality assurance in higher education is acknowledged by policymakers as well. They depend on accreditation and quality assurance procedures to guarantee that the public funds spent on higher education provide positive outcomes.
Each paper was read twice by JR, summarised and analysed to determine the paper's academic tradition, the debates around quality assurance in qualitative research identified and discussed, the definition(s) used for 'quality' and the values underpinning this, and recommended methods or strategies for assuring quality in qualitative research.
Research Article. free access. Principles and Practices of Analytical Method Validation: Validation of Analytical Methods is Time‐consuming but Essential ... Abstracts of the Society of Quality Assurance 27th Annual Meeting, San Antonio, Texas, USA, 27 March - 1 April 2011. Pages: S1-S56. April 2011. Volume 14, Issue 1-2. Pages: 1-37 ...
quality control (QC) and quality assurance (QA). In addition it serves as a starting point for implementing a quality system approach within an organization. The paper offers practical guidance to the implementation of quality and the importance of QC in its relationship to QA. It can be used in conjunction with the various quality
Explore the latest full-text research PDFs, articles, conference papers, preprints and more on QUALITY ASSURANCE. Find methods information, sources, references or conduct a literature review on ...
Explore the latest full-text research PDFs, articles, conference papers, preprints and more on SOFTWARE QUALITY ASSURANCE. Find methods information, sources, references or conduct a literature ...
The paper also explores international perspectives on quality assurance in higher education, emphasizing regional differences and global initiatives. It looks at how standards-setting organizations, professional groups, and quality control organizations influence policies and encourage institutional cooperation.
Among the 37 papers included in the review, two dominant narratives were interpreted from the literature, reflecting contrasting approaches to quality assurance. The first focuses on demonstrating quality within research outputs; the second focuses on principles for quality practice throughout the research process.
In this editorial, we attempt to answer what characterizes research quality before we first examine how to achieve and subsequently evidence research of high quality. 2. What research quality is. 2.1. What research quality historically is. Research assessment plays an essential role in academic appointments, in annual performance reviews, in ...
We are thankful to all researchers in this paper references regarding the valuable scientific suggestions and guidance for improvement of this work. ... Sampson R., Tyson C., Mamay-Gentilin S. Quality assurance of research protocols conducted in the community: the National Institute on Drug Abuse Clinical Trials Network experience. Clinical ...
This paper provides an overview of the current research status and an analysis of the present state of knowledge in the area of software quality assurance. An extensive literature survey was conducted for this purpose. The articles identified were systematically classified into suitable cate- gories. We first present the categorization scheme ...
Quality Assurance in Health Care , Vol. 1, No. 2/3, pp. 147-159, 1989 Printed in Great Britain. 1040-6166/89 $3.00 + 0.00 Pergamon Press pic RESEARCH NEEDS IN QUALITY ASSURANCE Hannu Vuori* WHO Regional Office for Europe Copenhagen Denmark Originally written as a background paper for the discussion on the research needs in
Quality Assurance (QA) is a management method that is defined as "all those planne d. and systematic actions needed to provide adequate confidence that a product, service or. result will satisfy ...
Quality Assurance in Education publishes original empirical or theoretical articles on Quality Assurance issues, including dimensions and indicators of Quality and Quality Improvement, as applicable to education at all levels, including pre-primary, primary, secondary, higher and professional education. Periodically, QAE also publishes systematic reviews, research syntheses and assessment ...
This paper presents findings from a survey on the status quo of data quality assurance practices at research data repositories. The personalised online survey was conducted among repositories indexed in re3data in 2021. It covered the scope of the repository, types of data quality assessment, quality criteria, responsibilities, details of the ...
Quality Assurance in Research. In research contexts, quality assurance (QA) refers to strategies and policies for ensuring that data integrity, quality, and reliability are maintained at every stage of the project. This includes strategies for preventing errors from entering the datasets, taking precautions before data is collected, and ...
Explore the latest full-text research PDFs, articles, conference papers, preprints and more on PHARMACEUTICAL QUALITY ASSURANCE. Find methods information, sources, references or conduct a ...
A quality assurance program provides many benefits to the University and its research program. It is imperative that research programs can assure adherence to federal research compliance mandates in order to protect the operation and reputation of the University, its human research program and researchers.
Access the portal of NASS, the official source of agricultural data and statistics in the US, and explore various reports and products.
quality control of the process aims to monitor the uncertainty (repeatability, reproducibility) and trueness of the measurement results. 5.1.2.1 Uncertainty of the measurement results. The ...