Visual Perception Theory In Psychology
Saul Mcleod, PhD
Editor-in-Chief for Simply Psychology
BSc (Hons) Psychology, MRes, PhD, University of Manchester
Saul Mcleod, PhD., is a qualified psychology teacher with over 18 years of experience in further and higher education. He has been published in peer-reviewed journals, including the Journal of Clinical Psychology.
Learn about our Editorial Process
Olivia Guy-Evans, MSc
Associate Editor for Simply Psychology
BSc (Hons) Psychology, MSc Psychology of Education
Olivia Guy-Evans is a writer and associate editor for Simply Psychology. She has previously worked in healthcare and educational sectors.
On This Page:
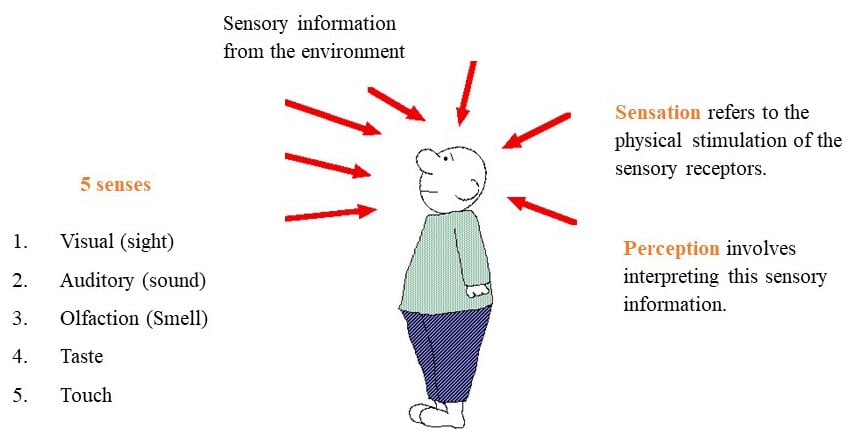
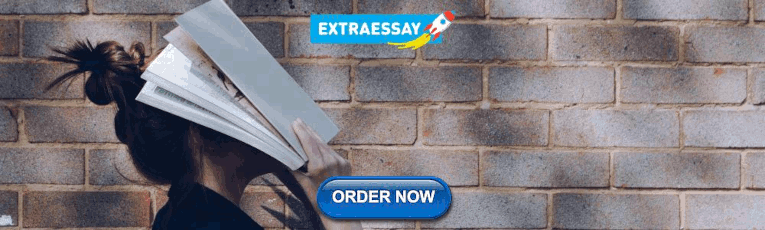
What is Visual Perception?
To receive information from the environment, we are equipped with sense organs, e.g., the eye, ear, and nose. Each sense organ is part of a sensory system that receives sensory inputs and transmits sensory information to the brain.
A particular problem for psychologists is explaining how the physical energy received by sense organs forms the basis of perceptual experience. Sensory inputs are somehow converted into perceptions of desks and computers, flowers and buildings, cars and planes, into sights, sounds, smells, tastes, and touch experiences.
A major theoretical issue on which psychologists are divided is the extent to which perception relies directly on the information present in the environment. Some argue that perceptual processes are not direct but depend on the perceiver’s expectations and previous knowledge as well as the information available in the stimulus itself.
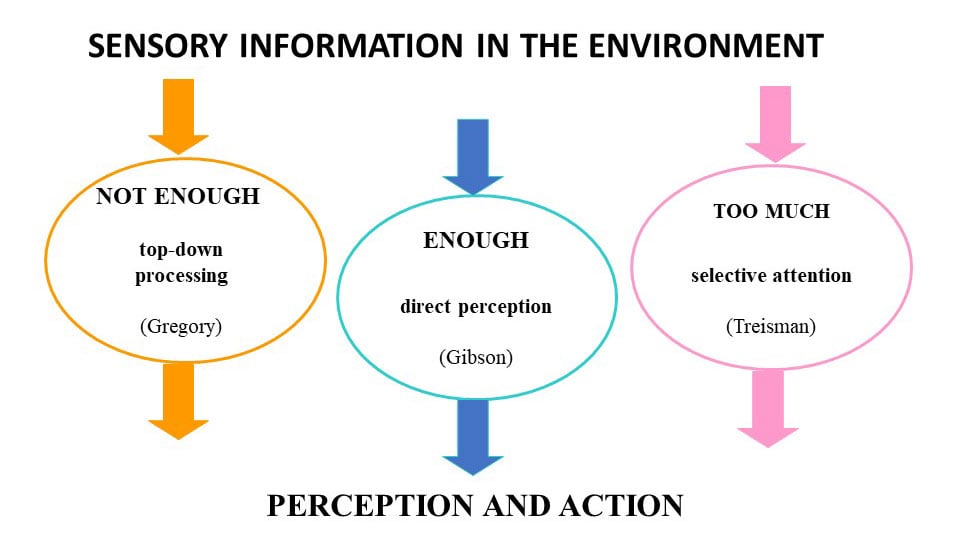
This controversy is discussed with respect to Gibson (1966), who has proposed a direct theory of perception which is a “bottom-up” theory, and Gregory (1970), who has proposed a constructivist (indirect) theory of perception which is a “top-down” theory.
Psychologists distinguish between two types of processes in perception: bottom-up processing and top-down processing .
Bottom-up processing is also known as data-driven processing because perception begins with the stimulus itself. Processing is carried out in one direction from the retina to the visual cortex, with each successive stage in the visual pathway carrying out an ever more complex analysis of the input.
Top-down processing refers to the use of contextual information in pattern recognition. For example, understanding difficult handwriting is easier when reading complete sentences than reading single and isolated words. This is because the meaning of the surrounding words provides a context to aid understanding.
Gregory (1970) and Top-Down Processing Theory
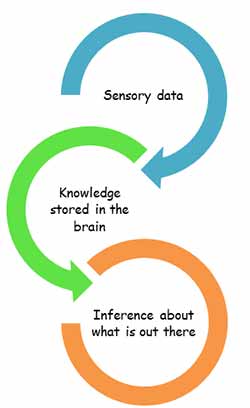
Psychologist Richard Gregory (1970) argued that perception is a constructive process that relies on top-down processing.
Stimulus information from our environment is frequently ambiguous, so to interpret it, we require higher cognitive information either from past experiences or stored knowledge in order to make inferences about what we perceive. Helmholtz called it the ‘likelihood principle’.
For Gregory, perception is a hypothesis which is based on prior knowledge. In this way, we are actively constructing our perception of reality based on our environment and stored information.
- A lot of information reaches the eye, but much is lost by the time it reaches the brain (Gregory estimates about 90% is lost).
- Therefore, the brain has to guess what a person sees based on past experiences. We actively construct our perception of reality.
- Richard Gregory proposed that perception involves a lot of hypothesis testing to make sense of the information presented to the sense organs.
- Our perceptions of the world are hypotheses based on past experiences and stored information.
- Sensory receptors receive information from the environment, which is then combined with previously stored information about the world which we have built up as a result of experience.
- The formation of incorrect hypotheses will lead to errors of perception (e.g., visual illusions like the Necker cube).
Supporting Evidence
There seems to be an overwhelming need to reconstruct the face, similar to Helmholtz’s description of “unconscious inference.” An assumption based on past experience.
Perceptions can be ambiguous
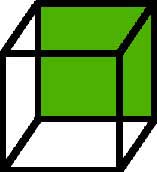
The Necker cube is a good example of this. When you stare at the crosses on the cube, the orientation can suddenly change or “flip.”
It becomes unstable, and a single physical pattern can produce two perceptions.
Gregory argued that this object appears to flip between orientations because the brain develops two equally plausible hypotheses and is unable to decide between them.
When the perception changes though there is no change in the sensory input, the change of appearance cannot be due to bottom-up processing. It must be set downwards by the prevailing perceptual hypothesis of what is near and what is far.
Perception allows behavior to be generally appropriate to non-sensed object characteristics.
Critical Evaluation of Gregory’s Theory
1. the nature of perceptual hypotheses.
If perceptions make use of hypothesis testing, the question can be asked, “what kind of hypotheses are they?” Scientists modify a hypothesis according to the support they find for it, so are we, as perceivers, also able to modify our hypotheses? In some cases, it would seem the answer is yes. For example, look at the figure below:
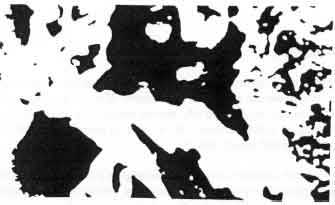
This probably looks like a random arrangement of black shapes. In fact, there is a hidden face in there; can you see it? The face is looking straight ahead and is in the top half of the picture in the center. Now can you see it? The figure is strongly lit from the side and has long hair and a beard.
Once the face is discovered, very rapid perceptual learning takes place and the ambiguous picture now obviously contains a face each time we look at it. We have learned to perceive the stimulus in a different way.
Although in some cases, as in the ambiguous face picture, there is a direct relationship between modifying hypotheses and perception, in other cases, this is not so evident. For example, illusions persist even when we have full knowledge of them (e.g., the inverted face, Gregory 1974).
One would expect that the knowledge we have learned (from, say, touching the face and confirming that it is not “normal”) would modify our hypotheses in an adaptive manner. The current hypothesis testing theories cannot explain this lack of a relationship between learning and perception.
2. Perceptual Development
A perplexing question for the constructivists who propose perception is essentially top-down in nature is “how can the neonate ever perceive?” If we all have to construct our own worlds based on past experiences, why are our perceptions so similar, even across cultures? Relying on individual constructs for making sense of the world makes perception a very individual and chancy process.
The constructivist approach stresses the role of knowledge in perception and therefore is against the nativist approach to perceptual development.
However, a substantial body of evidence has been accrued favoring the nativist approach. For example, Newborn infants show shape constancy (Slater & Morison, 1985); they prefer their mother’s voice to other voices (De Casper & Fifer, 1980); and it has been established that they prefer normal features to scrambled features as early as 5 minutes after birth.
3. Sensory Evidence
Perhaps the major criticism of the constructivists is that they have underestimated the richness of sensory evidence available to perceivers in the real world (as opposed to the laboratory, where much of the constructivists” evidence has come from).
Constructivists like Gregory frequently use the example of size constancy to support their explanations. That is, we correctly perceive the size of an object even though the retinal image of an object shrinks as the object recedes. They propose that sensory evidence from other sources must be available for us to be able to do this.
However, in the real world, retinal images are rarely seen in isolation (as is possible in the laboratory). There is a rich array of sensory information, including other objects, background, the distant horizon, and movement. This rich source of sensory information is important to the second approach to explaining perception that we will examine, namely the direct approach to perception as proposed by Gibson.
Gibson argues strongly against the idea that perception involves top-down processing and criticizes Gregory’s discussion of visual illusions on the grounds that they are artificial examples and not images found in our normal visual environments.
This is crucial because Gregory accepts that misperceptions are the exception rather than the norm. Illusions may be interesting phenomena, but they might not be that information about the debate.
Gibson (1966) and Bottom-Up Processing
Gibson’s bottom-up theory suggests that perception involves innate mechanisms forged by evolution and that no learning is required. This suggests that perception is necessary for survival – without perception, we would live in a very dangerous environment.
Our ancestors would have needed perception to escape from harmful predators, suggesting perception is evolutionary.
James Gibson (1966) argues that perception is direct and not subject to hypothesis testing, as Gregory proposed. There is enough information in our environment to make sense of the world in a direct way.
His theory is sometimes known as the ‘Ecological Theory’ because of the claim that perception can be explained solely in terms of the environment.
For Gibson: the sensation is perception: what you see is what you get. There is no need for processing (interpretation) as the information we receive about size, shape, distance, etc., is sufficiently detailed for us to interact directly with the environment.
Gibson (1972) argued that perception is a bottom-up process, which means that sensory information is analyzed in one direction: from simple analysis of raw sensory data to the ever-increasing complexity of analysis through the visual system.
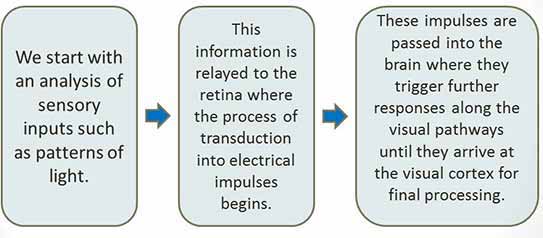
Features of Gibson’s Theory
The optic array.
Perception involves ‘picking up’ the rich information provided by the optic array in a direct way with little/no processing involved.
Because of movement and different intensities of light shining in different directions, it is an ever-changing source of sensory information. Therefore, if you move, the structure of the optic array changes.
According to Gibson, we have the mechanisms to interpret this unstable sensory input, meaning we experience a stable and meaningful view of the world.
Changes in the flow of the optic array contain important information about what type of movement is taking place. The flow of the optic array will either move from or towards a particular point.
If the flow appears to be coming from the point, it means you are moving towards it. If the optic array is moving towards the point, you are moving away from it.
Invariant Features
the optic array contains invariant information that remains constant as the observer moves. Invariants are aspects of the environment that don’t change. They supply us with crucial information.
Two good examples of invariants are texture and linear perspective.
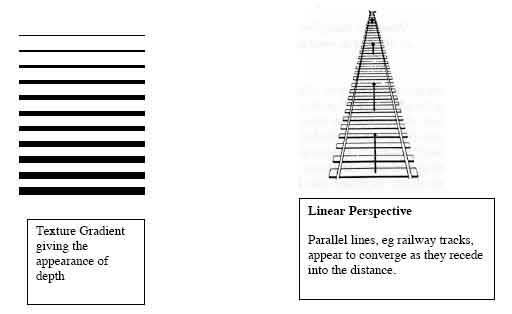
Another invariant is the horizon-ratio relation. The ratio above and below the horizon is constant for objects of the same size standing on the same ground.
OPTICAL ARRAY : The patterns of light that reach the eye from the environment.
RELATIVE BRIGHTNESS : Objects with brighter, clearer images are perceived as closer
TEXTURE GRADIENT : The grain of texture gets smaller as the object recedes. Gives the impression of surfaces receding into the distance.
RELATIVE SIZE : When an object moves further away from the eye, the image gets smaller. Objects with smaller images are seen as more distant.
SUPERIMPOSITION : If the image of one object blocks the image of another, the first object is seen as closer.
HEIGHT IN THE VISUAL FIELD : Objects further away are generally higher in the visual field
Evaluation of Gibson’s (1966) Direct Theory of Perception
Gibson’s theory is a highly ecologically valid theory as it puts perception back into the real world.
A large number of applications can be applied in terms of his theory, e.g., training pilots, runway markings, and road markings.
It’s an excellent explanation for perception when viewing conditions are clear. Gibson’s theory also highlights the richness of information in an optic array and provides an account of perception in animals, babies, and humans.
His theory is reductionist as it seeks to explain perception solely in terms of the environment. There is strong evidence to show that the brain and long-term memory can influence perception. In this case, it could be said that Gregory’s theory is far more plausible.
Gibson’s theory also only supports one side of the nature-nurture debate, that being the nature side. Again, Gregory’s theory is far more plausible as it suggests that what we see with our eyes is not enough, and we use knowledge already stored in our brains, supporting both sides of the debate.
Visual Illusions
Gibson’s emphasis on DIRECT perception provides an explanation for the (generally) fast and accurate perception of the environment. However, his theory cannot explain why perceptions are sometimes inaccurate, e.g., in illusions.
He claimed the illusions used in experimental work constituted extremely artificial perceptual situations unlikely to be encountered in the real world, however, this dismissal cannot realistically be applied to all illusions.
For example, Gibson’s theory cannot account for perceptual errors like the general tendency for people to overestimate vertical extents relative to horizontal ones.
Neither can Gibson’s theory explain naturally occurring illusions. For example, if you stare for some time at a waterfall and then transfer your gaze to a stationary object, the object appears to move in the opposite direction.
Bottom-up or Top-down Processing?
Neither direct nor constructivist theories of perception seem capable of explaining all perceptions all of the time.
Gibson’s theory appears to be based on perceivers operating under ideal viewing conditions, where stimulus information is plentiful and is available for a suitable length of time. Constructivist theories, like Gregory”s, have typically involved viewing under less-than-ideal conditions.
Research by Tulving et al. manipulated both the clarity of the stimulus input and the impact of the perceptual context in a word identification task. As the clarity of the stimulus (through exposure duration) and the amount of context increased, so did the likelihood of correct identification.
However, as the exposure duration increased, so the impact of context was reduced, suggesting that if stimulus information is high, then the need to use other sources of information is reduced.
One theory that explains how top-down and bottom-up processes may be seen as interacting with each other to produce the best interpretation of the stimulus was proposed by Neisser (1976) – known as the “Perceptual Cycle.”
DeCasper, A. J., & Fifer, W. P. (1980). Of human bonding: Newborns prefer their mothers” voices . Science , 208(4448), 1174-1176.
Gibson, J. J. (1966). The Senses Considered as Perceptual Systems. Boston: Houghton Mifflin.
Gibson, J. J. (1972). A Theory of Direct Visual Perception. In J. Royce, W. Rozenboom (Eds.). The Psychology of Knowing . New York: Gordon & Breach.
Gregory, R. (1970). The Intelligent Eye . London: Weidenfeld and Nicolson.
Gregory, R. (1974). Concepts and Mechanisms of Perception . London: Duckworth.
Necker, L. (1832). LXI. Observations on some remarkable optical phenomena seen in Switzerland; and on an optical phenomenon which occurs on viewing a figure of a crystal or geometrical solid . The London and Edinburgh Philosophical Magazine and Journal of Science, 1 (5), 329-337.
Slater, A., Morison, V., Somers, M., Mattock, A., Brown, E., & Taylor, D. (1990). Newborn and older infants” perception of partly occluded objects. Infant behavior and Development , 13(1), 33-49.
Further Information
Trichromatic Theory of Color Vision
Held and Hein (1963) Movement-Produced Stimulation in the Development of Visually Guided Behavior
What do visual illusions teach us?

Related Articles
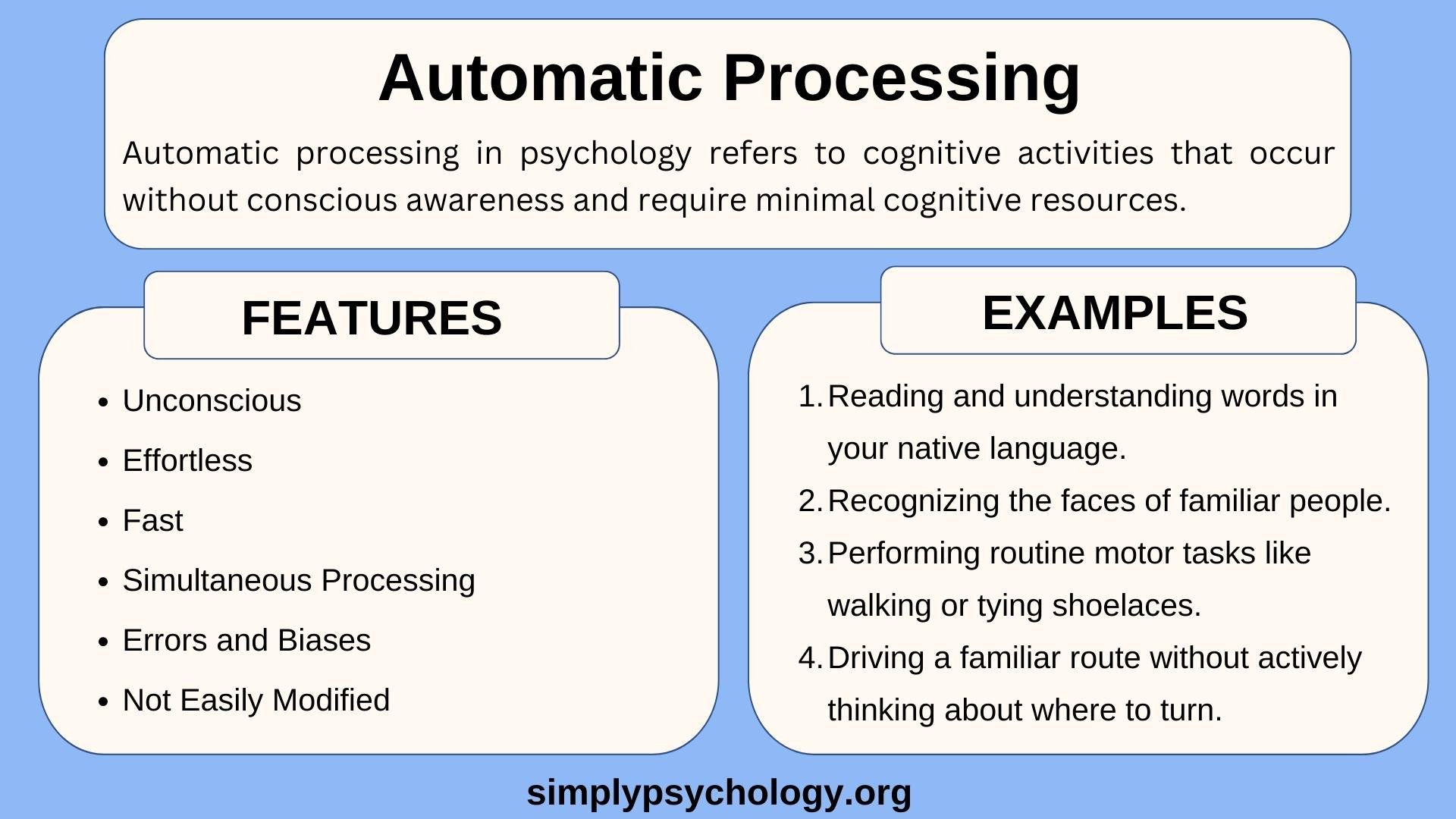
Cognitive Psychology
Automatic Processing in Psychology: Definition & Examples
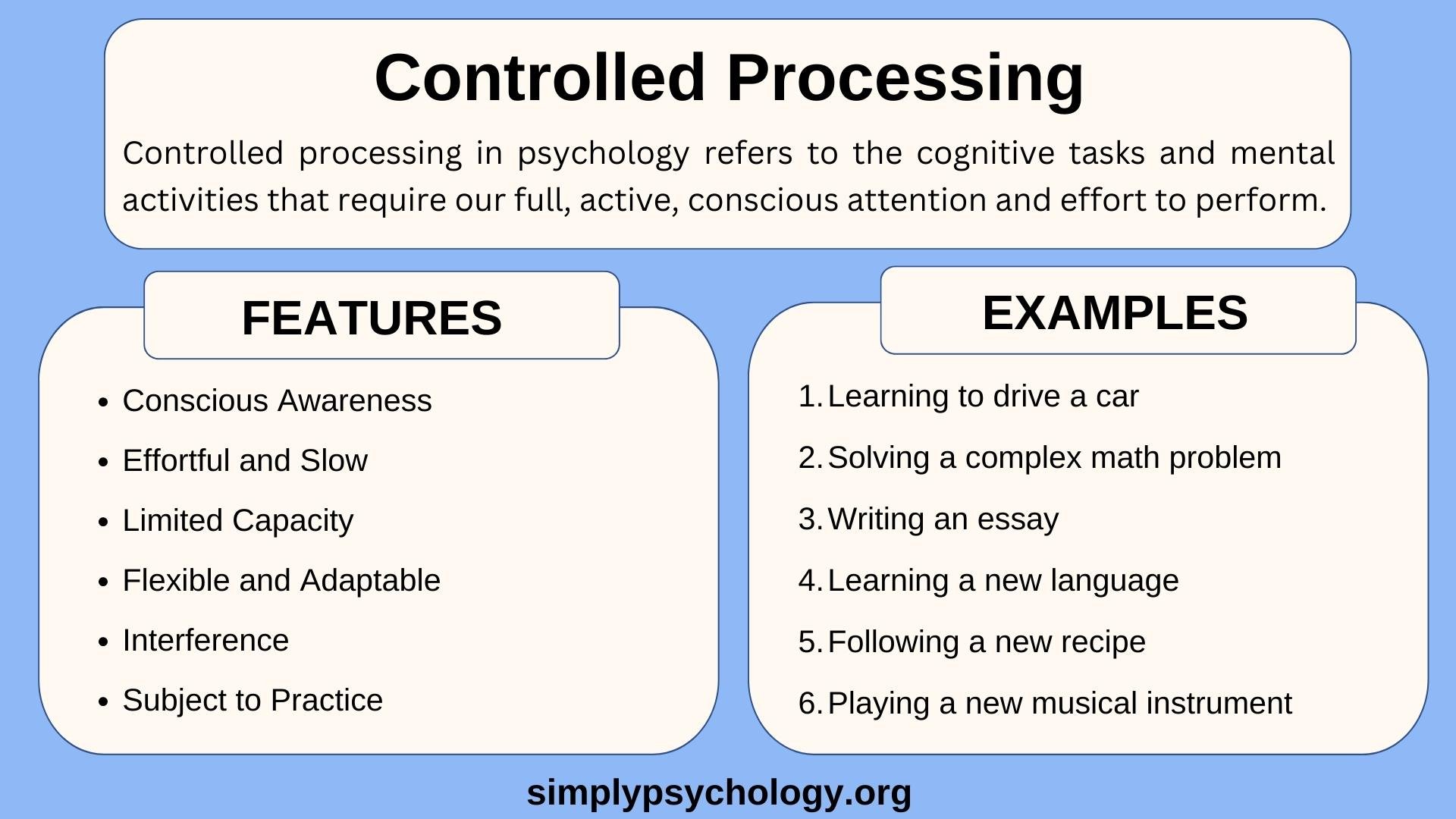
Controlled Processing in Psychology: Definition & Examples
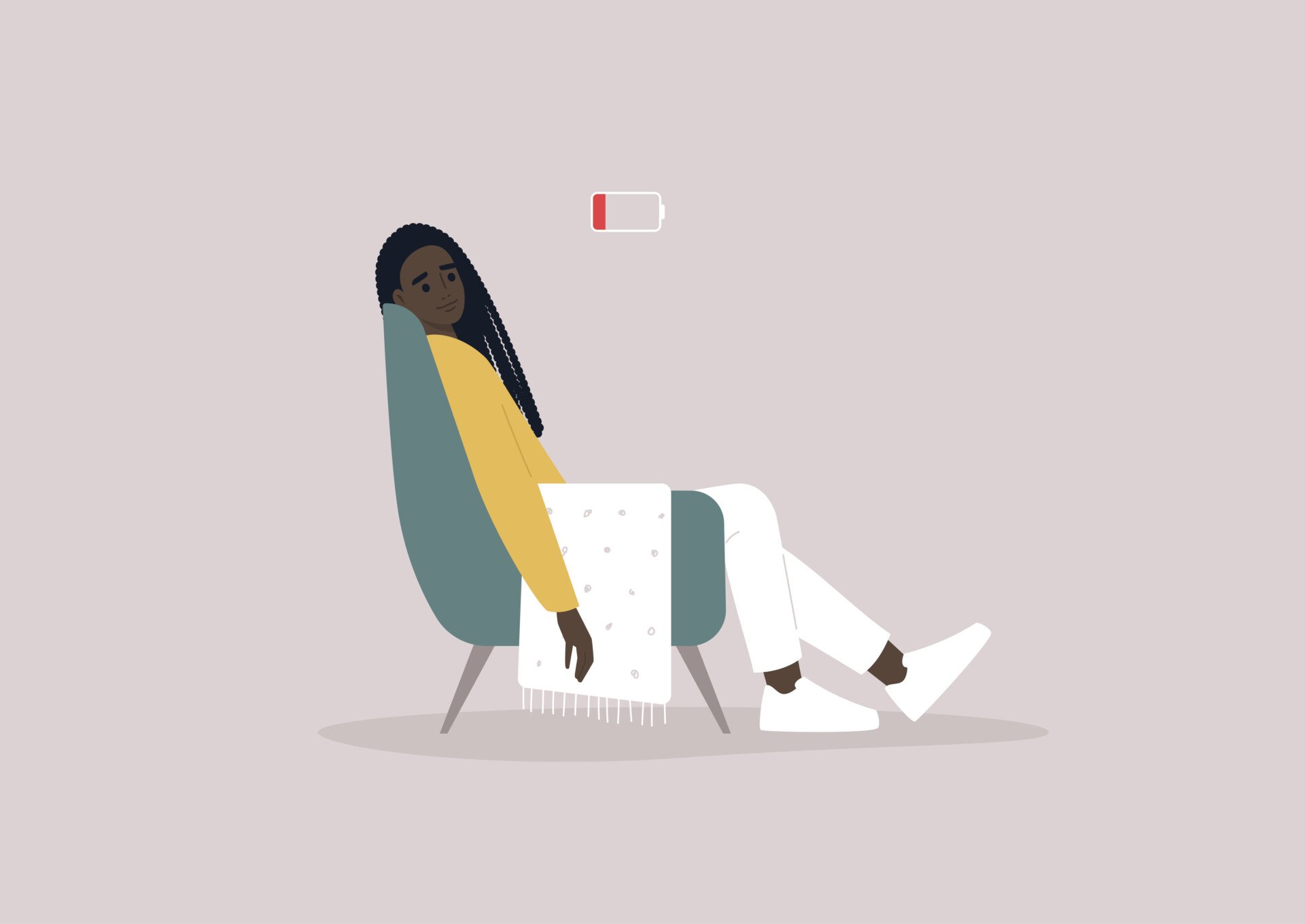
How Ego Depletion Can Drain Your Willpower
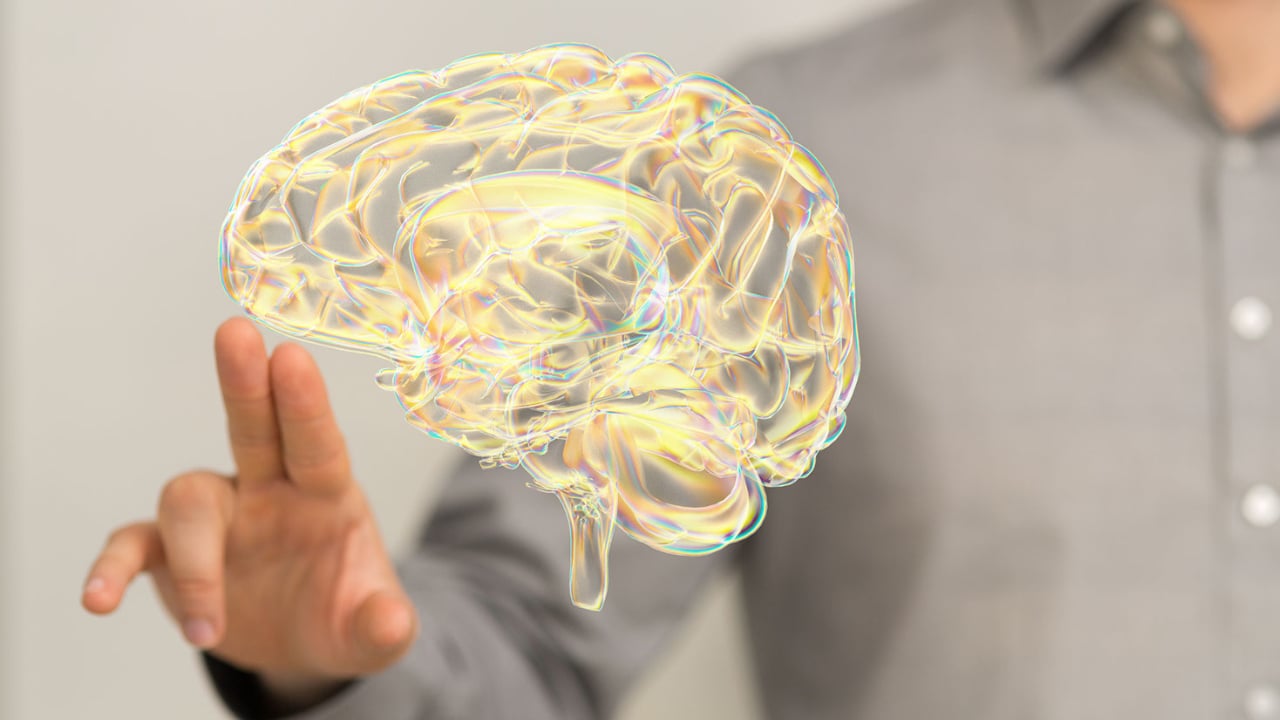
What is the Default Mode Network?
Theories of Selective Attention in Psychology
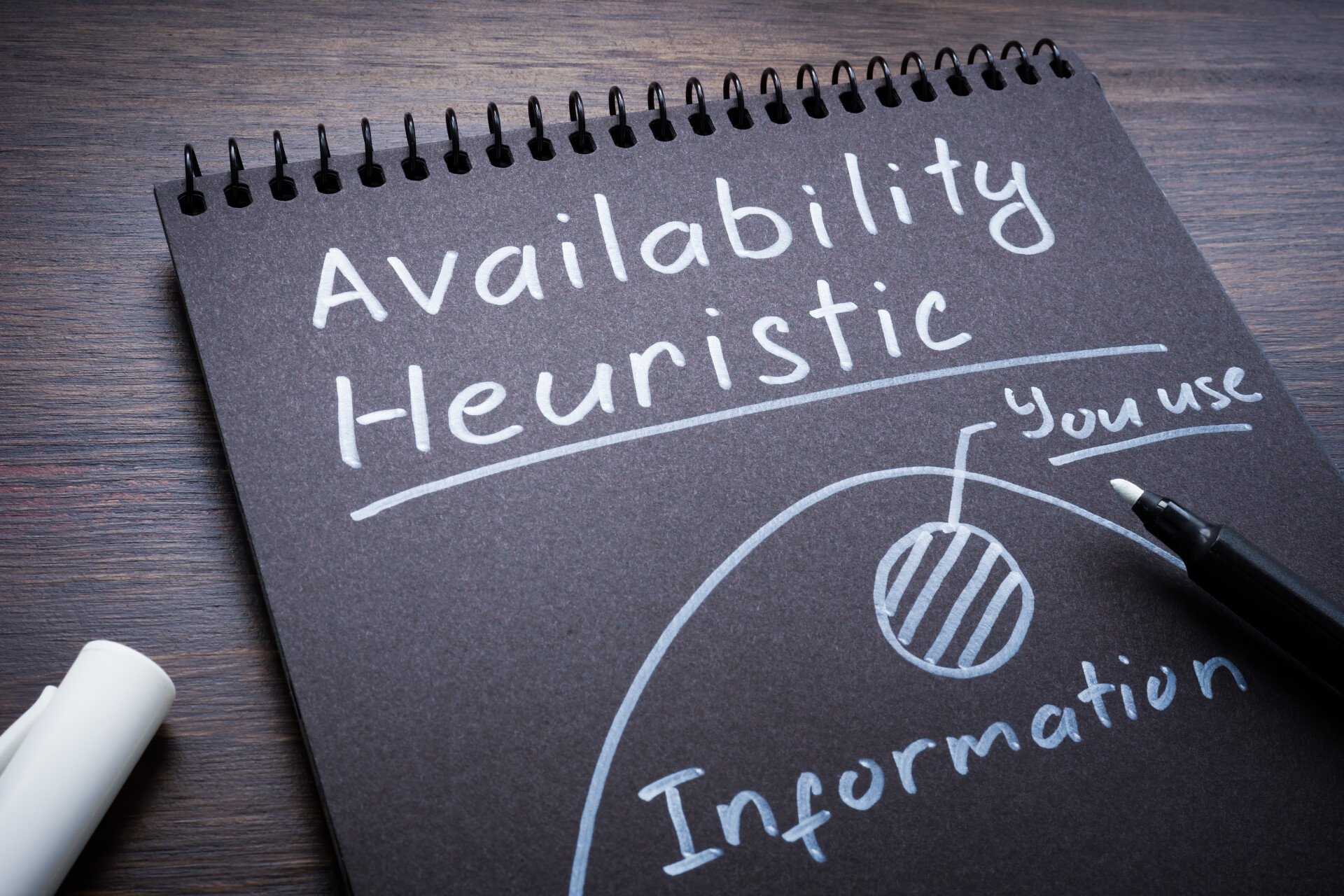
Availability Heuristic and Decision Making
5.6 The Gestalt Principles of Perception
Learning objectives.
By the end of this section, you will be able to:
- Explain the figure-ground relationship
- Define Gestalt principles of grouping
- Describe how perceptual set is influenced by an individual’s characteristics and mental state
In the early part of the 20th century, Max Wertheimer published a paper demonstrating that individuals perceived motion in rapidly flickering static images—an insight that came to him as he used a child’s toy tachistoscope. Wertheimer, and his assistants Wolfgang Köhler and Kurt Koffka, who later became his partners, believed that perception involved more than simply combining sensory stimuli. This belief led to a new movement within the field of psychology known as Gestalt psychology. The word gestalt literally means form or pattern, but its use reflects the idea that the whole is different from the sum of its parts. In other words, the brain creates a perception that is more than simply the sum of available sensory inputs, and it does so in predictable ways. Gestalt psychologists translated these predictable ways into principles by which we organize sensory information. As a result, Gestalt psychology has been extremely influential in the area of sensation and perception (Rock & Palmer, 1990).
Gestalt perspectives in psychology represent investigations into ambiguous stimuli to determine where and how these ambiguities are being resolved by the brain. They are also aimed at understanding sensory and perception as processing information as groups or wholes instead of constructed wholes from many small parts. This perspective has been supported by modern cognitive science through fMRI research demonstrating that some parts of the brain, specifically the lateral occipital lobe, and the fusiform gyrus, are involved in the processing of whole objects, as opposed to the primary occipital areas that process individual elements of stimuli (Kubilius, Wagemans & Op de Beeck, 2011).
One Gestalt principle is the figure-ground relationship. According to this principle, we tend to segment our visual world into figure and ground. Figure is the object or person that is the focus of the visual field, while the ground is the background. As the figure below shows, our perception can vary tremendously, depending on what is perceived as figure and what is perceived as ground. Presumably, our ability to interpret sensory information depends on what we label as figure and what we label as ground in any particular case, although this assumption has been called into question (Peterson & Gibson, 1994; Vecera & O’Reilly, 1998).

The concept of figure-ground relationship explains why this image can be perceived either as a vase or as a pair of faces.
Another Gestalt principle for organizing sensory stimuli into meaningful perception is proximity . This principle asserts that things that are close to one another tend to be grouped together, as the figure below illustrates.
The Gestalt principle of proximity suggests that you see (a) one block of dots on the left side and (b) three columns on the right side.
How we read something provides another illustration of the proximity concept. For example, we read this sentence like this, notl iket hiso rt hat. We group the letters of a given word together because there are no spaces between the letters, and we perceive words because there are spaces between each word. Here are some more examples: Cany oum akes enseo ft hiss entence? What doth es e wor dsmea n?
We might also use the principle of similarity to group things in our visual fields. According to this principle, things that are alike tend to be grouped together (figure below). For example, when watching a football game, we tend to group individuals based on the colors of their uniforms. When watching an offensive drive, we can get a sense of the two teams simply by grouping along this dimension.
When looking at this array of dots, we likely perceive alternating rows of colors. We are grouping these dots according to the principle of similarity.
Two additional Gestalt principles are the law of continuity (or good continuation) and closure. The law of continuity suggests that we are more likely to perceive continuous, smooth flowing lines rather than jagged, broken lines (figure below). The principle of closure states that we organize our perceptions into complete objects rather than as a series of parts (figure below).
Good continuation would suggest that we are more likely to perceive this as two overlapping lines, rather than four lines meeting in the center.
Closure suggests that we will perceive a complete circle and rectangle rather than a series of segments..
According to Gestalt theorists, pattern perception, or our ability to discriminate among different figures and shapes, occurs by following the principles described above. You probably feel fairly certain that your perception accurately matches the real world, but this is not always the case. Our perceptions are based on perceptual hypotheses: educated guesses that we make while interpreting sensory information. These hypotheses are informed by a number of factors, including our personalities, experiences, and expectations. We use these hypotheses to generate our perceptual set. For instance, research has demonstrated that those who are given verbal priming produce a biased interpretation of complex ambiguous figures (Goolkasian & Woodbury, 2010).
Template Approach
Ulrich Neisser (1967), author of one of the first cognitive psychology textbook suggested pattern recognition would be simplified, although abilities would still exist, if all the patterns we experienced were identical. According to this theory, it would be easier for us to recognize something if it matched exactly with what we had perceived before. Obviously the real environment is infinitely dynamic producing countless combinations of orientation, size. So how is it that we can still read a letter g whether it is capitalized, non-capitalized or in someone else hand writing? Neisser suggested that categorization of information is performed by way of the brain creating mental templates , stored models of all possible categorizable patterns (Radvansky & Ashcraft, 2014). When a computer reads your debt card information it is comparing the information you enter to a template of what the number should look like (has a specific amount of numbers, no letters or symbols…). The template view perception is able to easily explain how we recognize pieces of our environment, but it is not able to explain why we are still able to recognize things when it is not viewed from the same angle, distance, or in the same context.
In order to address the shortfalls of the template model of perception, the feature detection approach to visual perception suggests we recognize specific features of what we are looking at, for example the straight lines in an H versus the curved line of a letter C. Rather than matching an entire template-like pattern for the capital letter H, we identify the elemental features that are present in the H. Several people have suggested theories of feature-based pattern recognition, one of which was described by Selfridge (1959) and is known as the pandemonium model suggesting that information being perceived is processed through various stages by what Selfridge described as mental demons, who shout out loud as they attempt to identify patterns in the stimuli. These pattern demons are at the lowest level of perception so after they are able to identify patterns, computational demons further analyze features to match to templates such as straight or curved lines. Finally at the highest level of discrimination, cognitive demons which allow stimuli to be categorized in terms of context and other higher order classifications, and the decisions demon decides among all the demons shouting about what the stimuli is which while be selected for interpretation.
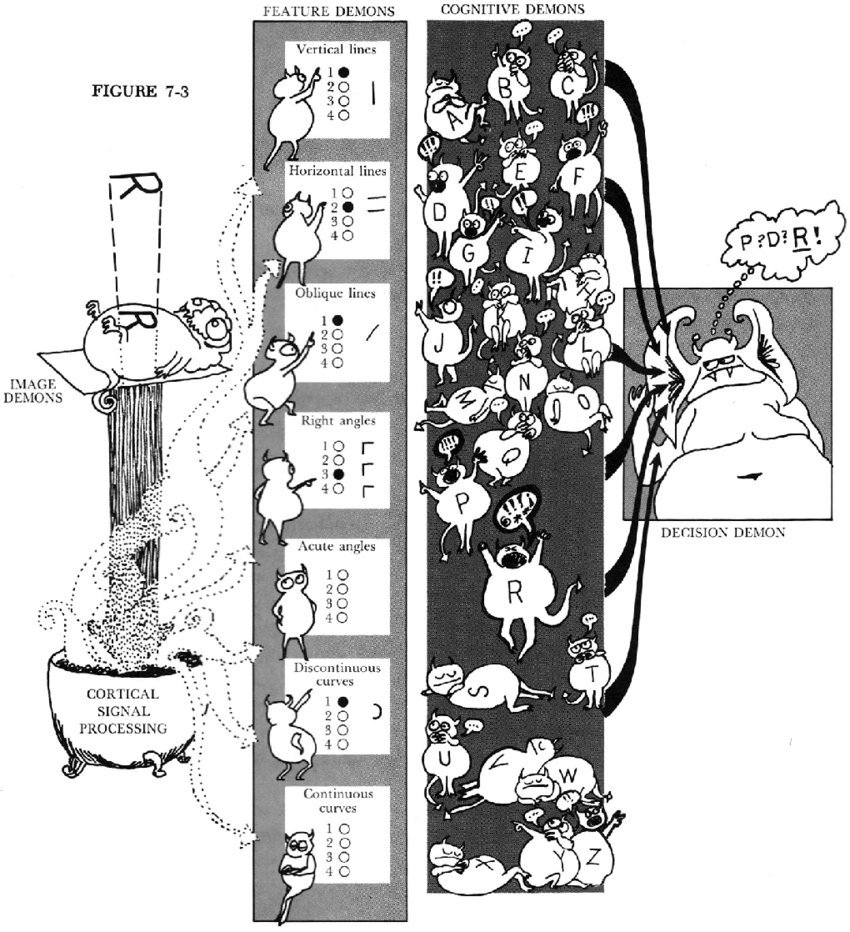
Selfridge’s pandemonium model showing the various levels of demons which make estimations and pass the information on to the next level before the decision demon makes the best estimation to what the stimuli is. Adapted from Lindsay and Norman (1972).
Although Selfridges ideas regarding layers of shouting demons that make up our ability to discriminate features of our environment, the model actually incorporates several ideas that are important for pattern recognition. First, at its foundation, this model is a feature detection model that incorporates higher levels of processing as the information is processed in time. Second, the Selfridge model of many different shouting demons incorporates ideas of parallel processing suggesting many different forms of stimuli can be analyzed and processed to some extent at the same time. Third and finally, the model suggests that perception in a very real sense is a series of problem solving procedures where we are able to take bits of information and piece it all together to create something we are able to recognize and classify as something meaningful.
In addition to sounding initially improbable by being based on a series of shouting fictional demons, one of the main critiques of Selfridge’s demon model of feature detection is that it is primarily a bottom-up , or data-driven processing system. This means the feature detection and processing for discrimination all comes from what we get out of the environment. Modern progress in cognitive science has argued against strictly bottom-up processing models suggesting that context plays an extremely important role in determining what you are perceiving and discriminating between stimuli. To build off previous models, cognitive scientist suggested an additional top-down , or conceptually-driven account in which context and higher level knowledge such as context something tends to occur in or a persons expectations influence lower-level processes.
Finally the most modern theories that attempt to describe how information is processed for our perception and discrimination are known as connectionist models. Connectionist models incorporate an enormous amount of mathematical computations which work in parallel and across series of interrelated web like structures using top-down and bottom-up processes to narrow down what the most probably solution for the discrimination would be. Each unit in a connectionist layer is massively connected in a giant web with many or al the units in the next layer of discrimination. Within these models, even if there is not many features present in the stimulus, the number of computations in a single run for discrimination become incredibly large because of all the connections that exist between each unit and layer.
The Depths of Perception: Bias, Prejudice, and Cultural Factors
In this chapter, you have learned that perception is a complex process. Built from sensations, but influenced by our own experiences, biases, prejudices, and cultures , perceptions can be very different from person to person. Research suggests that implicit racial prejudice and stereotypes affect perception. For instance, several studies have demonstrated that non-Black participants identify weapons faster and are more likely to identify non-weapons as weapons when the image of the weapon is paired with the image of a Black person (Payne, 2001; Payne, Shimizu, & Jacoby, 2005). Furthermore, White individuals’ decisions to shoot an armed target in a video game is made more quickly when the target is Black (Correll, Park, Judd, & Wittenbrink, 2002; Correll, Urland, & Ito, 2006). This research is important, considering the number of very high-profile cases in the last few decades in which young Blacks were killed by people who claimed to believe that the unarmed individuals were armed and/or represented some threat to their personal safety.
Gestalt theorists have been incredibly influential in the areas of sensation and perception. Gestalt principles such as figure-ground relationship, grouping by proximity or similarity, the law of good continuation, and closure are all used to help explain how we organize sensory information. Our perceptions are not infallible, and they can be influenced by bias, prejudice, and other factors.
References:
Openstax Psychology text by Kathryn Dumper, William Jenkins, Arlene Lacombe, Marilyn Lovett and Marion Perlmutter licensed under CC BY v4.0. https://openstax.org/details/books/psychology
Review Questions:
1. According to the principle of ________, objects that occur close to one another tend to be grouped together.
a. similarity
b. good continuation
c. proximity
2. Our tendency to perceive things as complete objects rather than as a series of parts is known as the principle of ________.
d. similarity
3. According to the law of ________, we are more likely to perceive smoothly flowing lines rather than choppy or jagged lines.
4. The main point of focus in a visual display is known as the ________.
b. perceptual set
Critical Thinking Question:
1. The central tenet of Gestalt psychology is that the whole is different from the sum of its parts. What does this mean in the context of perception?
2. Take a look at the following figure. How might you influence whether people see a duck or a rabbit?

Personal Application Question:
1. Have you ever listened to a song on the radio and sung along only to find out later that you have been singing the wrong lyrics? Once you found the correct lyrics, did your perception of the song change?
figure-ground relationship
Gestalt psychology
- good continuation
pattern perception
perceptual hypothesis
principle of closure
Key Takeaways
1. This means that perception cannot be understood completely simply by combining the parts. Rather, the relationship that exists among those parts (which would be established according to the principles described in this chapter) is important in organizing and interpreting sensory information into a perceptual set.
2. Playing on their expectations could be used to influence what they were most likely to see. For instance, telling a story about Peter Rabbit and then presenting this image would bias perception along rabbit lines.
closure: organizing our perceptions into complete objects rather than as a series of parts
figure-ground relationship: segmenting our visual world into figure and ground
Gestalt psychology: field of psychology based on the idea that the whole is different from the sum of its parts
good continuation: (also, continuity) we are more likely to perceive continuous, smooth flowing lines rather than jagged, broken lines
pattern perception: ability to discriminate among different figures and shapes
perceptual hypothesis: educated guess used to interpret sensory information
principle of closure: organize perceptions into complete objects rather than as a series of parts
proximity: things that are close to one another tend to be grouped together
similarity: things that are alike tend to be grouped together
Review Questions
According to the principle of ________, objects that occur close to one another tend to be grouped together.
Our tendency to perceive things as complete objects rather than as a series of parts is known as the principle of ________.
According to the law of ________, we are more likely to perceive smoothly flowing lines rather than choppy or jagged lines.
The main point of focus in a visual display is known as the ________.
- perceptual set
Critical Thinking Question
The central tenet of Gestalt psychology is that the whole is different from the sum of its parts. What does this mean in the context of perception?
Take a look at the following figure. How might you influence whether people see a duck or a rabbit?
Answer: Playing on their expectations could be used to influence what they were most likely to see. For instance, telling a story about Peter Rabbit and then presenting this image would bias perception along rabbit lines.
Personal Application Question
Have you ever listened to a song on the radio and sung along only to find out later that you have been singing the wrong lyrics? Once you found the correct lyrics, did your perception of the song change?

Share This Book
- Increase Font Size
Key Theories On The Psychology Of Perception
Perception is defined as “ the process or result of becoming aware of objects, relationships, and events by means of the senses, which includes such activities as recognizing, observing, and discriminating.” This process allows us to notice and then interpret stimuli and sensory input around us so we can understand and respond accordingly. While perception may seem simple, it’s actually a complex and highly individualized process with many psychological components and implications. Below, we’ll cover the basics of perception psychology along with a few of the leading theories on this topic.
How we perceive the world around us, from auditory to visual perception
Let’s start with a brief overview of the basic mechanisms of direct perception, or the ways in which we’re able to perceive the world around us (i.e., through sensory receptors). Scientists now recognize seven senses that humans can use to gather information about our surroundings. These sense organs and systems allow us to recognize faces, notice smells, and respond to other stimuli:
Visual perception: sight and visual information perceived through the eyes
Auditory perception: sounds or auditory signals perceived through the ears
Gustatory perception: awareness of flavor and taste on the tongue
Olfactory perception: smelling via the nose
Tactile perception: awareness of sensation on the skin
Vestibular sense: perception of balance and motion or physical energy
Proprioception: perceptual context of the body’s position in space
The psychology of what the perception process involves
Perception psychology is a division of cognitive psychology that studies how humans receive and understand the information delivered through the senses—also known as the perceptual experience. As mentioned above, perception processes include a network of bodily systems and sense organs that receive information and then process it. As we interact with the physical world, our brains interpret this information to make sense of what we experience.
Our brains also automatically attempt to group perceptions to help us understand and interpret our world. There are six main principles of the sensory systems the human mind uses to organize what it perceives:
Similarity, or grouping things that look like each other. Items with the same shape, size, and/or color make up parts of perceived patterns that appear to belong together.
Proximity, or grouping things according to how physically close they are to each other. The closer together they are, the more likely the brain will identify them as a group—even if they don’t have any connection to each other.
Continuity, or the tendency to perceive individual elements and other objects as a whole rather than a series of parts
Inclusiveness, or perceiving all elements of an image before recognizing the parts of it. For example, you may sense one object—a car—before recognizing the color, make, or who is inside.
Closure, or seeing a partial image or ambiguous picture and filling in the gaps of what you believe should be there. This ability allows one to overlook a partial understanding and perceive the situation in its entirety, despite missing information.
Prägnanz, or the tendency to simplify complex stimuli into a simple pattern. An example is using visual systems to look at a complex building and being aware of where the front door is while not registering the structure’s many other features.
Main theories on the psychology of the perception process
Psychologists and researchers continue to explore the nuances of this complex field. As of today, here’s a brief overview of some of the key perception psychology theories out there. Note that none of these completely explains the process in every instance; this field of study is ongoing.
Perception psychology according to Bruner
Jerome S. Bruner was an American psychologist who theorized that people go through various processes before they form opinions about what they have observed. According to Bruner, people use different informational cues to ultimately define their perceptions. This information-seeking continues until the individual comes across a familiar part and the mind categorizes it. If signals are distorted or do not fit a person’s initial perceptions, the images are forgotten or ignored while a picture forms on the most familiar perceptions.
Perception psychology according to Gibson
James J. Gibson is another American psychologist who studied perception psychology. Gibson is known for his philosophy of the direct theory of visual perception in particular, also called the “bottom-up” processing theory. He believed we can explain visual perception solely in terms of the environment, beginning with a sensory stimulus. In each stage of the perceptual processes, the eyes send signals to the brain to continue analyzing—from the bottom up—until it can conclude what the person is seeing.
Gibson theorized that the starting point of the bottom-up process of visual perception begins with the pattern of light that reaches our eyes. These signals then form the basis of our understanding of perceptions because they convey unambiguous information about the spatial layout we perceive. He further defined perception according to what he called affordances. He identified six affordances of perception, including:
Optical array: the patterns of light that travel from the environment to the eyes
Relative brightness: the perception that brighter, more evident objects are closer than darker, out-of-focus objects
Texture gradient: The grain of texture becomes less defined as an object recedes, indicating that the object may be further in the distance.
Relative size: Objects that are farther away will appear smaller.
Superimposition: When one image partially blocks another, the viewer sees the first image as being closer to them. Superimposition is similar to inattentional blindness , in which the eyes cannot see an object because another object fully engages them.
Height in the visual field: Objects that are further away from the viewer typically appear higher in the visual field.
Perception psychology, according to Gregory
Richard Langton Gregory was a British psychologist and Emeritus Professor of Neuropsychology at the University of Bristol. Gregory was also the author of the constructivist theory of perception, or the "top-down" processing theory—which takes the opposite approach of Gibson’s “bottom-up” theory. It assumes that our cognitive processes—including memory and perception—result from our continuously generating hypotheses about the world from the top down. In other words, we recognize patterns by understanding the context in which we perceive them.
Consider handwriting as an example. The handwriting of many individuals can be difficult for others to read; however, if we can pick out a few words here or there, it helps us understand the text’s context, and that helps us figure out the words we could not read. In other words, Gregory's theory assumes we have previous knowledge of what we are perceiving in addition to the stimulus itself. Because stimuli can often be ambiguous, correctly perceiving them requires a higher level of cognition because we must draw from stored knowledge or past experiences to help us understand our perceptions. He believed perception is based on our accumulated knowledge and that we actively construct perceptions whether they’re correct or not—though an incorrect hypothesis can lead to errors in perception.
Exploring how thoughts affect perception with a therapist
The way we perceive objects, individuals, events, and our environment can have a significant impact on our mood, emotions, and behaviors. In some cases, our perceptions can be distorted, which can lead to distressing feelings or even symptoms of a mental health condition like depression or anxiety. Talk therapy— cognitive behavioral therapy (CBT) in particular—is one way to learn how to recognize any cognitive distortions you may be experiencing and shift your thoughts in a more realistic, balanced, and healthy direction.
Regularly attending in-person therapy sessions is not possible for everyone. Some may not have adequate provider options in their area, while others may have trouble commuting to and from in-office sessions. In cases like these, online therapy can represent a viable alternative. A platform like BetterHelp can match you with a licensed therapist who you can meet with via video, phone, and/or in-app messaging, all from the comfort of home. Research suggests that virtual therapy is “no less efficacious” than the in-person variety in many cases, so you can generally feel confident in selecting whichever format may work best for you.
What are examples of perception in psychology?
Some examples of types of perception include taste perception, such as being able to identify various flavors in what you’re eating, or visual perception, such as being able to identify and distinguish between a rock, a tree, and a flower.
What is the simple definition of perception?
The simple, specific meaning of perception is how we use our five senses—plus our senses of balance and our perception of our own body position—to experience the world around us. Perception involves actions like seeing, touching, tasting, and smelling in order to take in our surroundings and then using automatic neural processing to make sense of them.
What are the 4 stages of perception?
The perception process involves four basic stages. First, the individual is exposed to a stimulus through their environment and becomes aware of it through one or more of their perception skills, or senses. Second, their brain registers the stimulus based on the information gathered through the sense(s). Next, the information is organized based on a person’s existing knowledge and beliefs. Finally, the person interprets the stimulus based on their own knowledge and beliefs, such as a good or bad smell, a dangerous or non-dangerous animal, a pleasant or grating sound, etc.
What is perceptual psychology simple?
Perceptual psychology is made up of various theories from studies over the years about why and how we take in information from the environments around us and perceive things in a certain way. There are many elements that go into why a person may perceive something the way they do, such as existing knowledge, beliefs, culture, and even mental health. Perceptual psychologists study these unconscious processes that contribute to a person’s perception.
What are 4 examples of perception?
Perception refers to how we see and make sense of the world around us. Four examples include seeing a sunset, smelling a fragrant flower, hearing music playing, and touching a soft blanket.
What is an example of perception in human behavior?
Perception is how our sensory organs detect or perceive stimuli in our surroundings. An example of perception as it relates to human behavior is two people seeing a dog and reacting differently based on their past experiences, knowledge, and beliefs. One might react in fear because they were chased by a dog as a child or in disgust because they think all dogs smell bad. The other might react in excitement and go to interact with the dog because they have a beloved dog of their own at home.
What is an example of perception effect?
There are several different perception effects the human mind uses to categorize, organize, and make sense of the world, and of which we are typically not consciously aware. For example, we’re likely to unconsciously group things that resemble each other—such as objects of the same shape, size, or color—because our brain tells us that they belong together.
What are the 3 factors that influence perception?
There are many different factors that can affect perception, so much so that the field of perception psychology, a type of social psychology, is devoted to examining and understanding them. A few examples of factors that could influence the way an individual perceives something include past experiences, prior knowledge, and cultural values.
What is an example of perception and personality?
The way we perceive words and sounds, sights, smells, tastes, and other forms of stimuli is influenced by our personality. For example, the words one person perceives through auditory signals and then interprets to find offensive may be welcomed by another due to a natural tendency toward humor, optimism/pessimism, etc.
What is perception and why is it important?
Overall, perception is our ability to identify stimuli in the world around us and interpret it according to our own values, personality, culture, and other factors. It’s important because it’s the means through which we sense and then interpret the world around us.
- Exploring Extinction Psychology Medically reviewed by Arianna Williams , LPC, CCTP
- Is Transpersonal Psychology Right For Me? Medically reviewed by April Justice , LICSW
- Psychologists
- Relationships and Relations
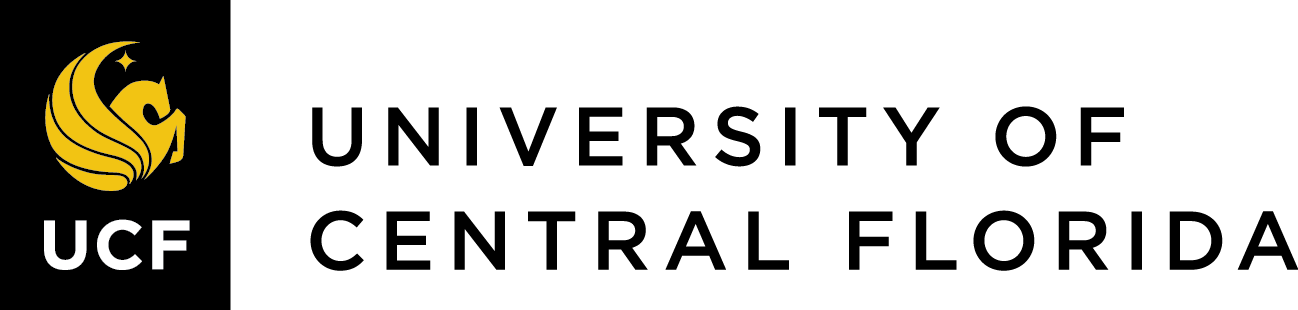
Sensation and Perception
What is Perception?
Learning objectives.
- Discuss the roles attention, motivation, and sensory adaptation play in perception
While our sensory receptors are constantly collecting information from the environment, it is ultimately how we interpret that information that affects how we interact with the world. Perception refers to the way sensory information is organized, interpreted, and consciously experienced. Perception involves both bottom-up and top-down processing. Bottom-up processing refers to the fact that perceptions are built from sensory input. On the other hand, how we interpret those sensations is influenced by our available knowledge, our experiences, and our thoughts. This is called top-down processing .
Look at the shape in Figure 1 below. Seen alone, your brain engages in bottom-up processing. There are two thick vertical lines and three thin horizontal lines. There is no context to give it a specific meaning, so there is no top-down processing involved.
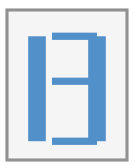
Now, look at the same shape in two different contexts. Surrounded by sequential letters, your brain expects the shape to be a letter and to complete the sequence. In that context, you perceive the lines to form the shape of the letter “B.”
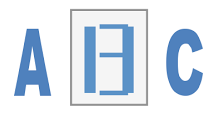
Surrounded by numbers, the same shape now looks like the number “13.”
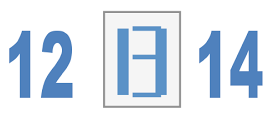
When given a context, your perception is driven by your cognitive expectations. Now you are processing the shape in a top-down fashion.
One way to think of this concept is that sensation is a physical process, whereas perception is psychological. For example, upon walking into a kitchen and smelling the scent of baking cinnamon rolls, the sensation is the scent receptors detecting the odor of cinnamon, but the perception may be “Mmm, this smells like the bread Grandma used to bake when the family gathered for holidays.”
Although our perceptions are built from sensations, not all sensations result in perception. In fact, we often don’t perceive stimuli that remain relatively constant over prolonged periods of time. This is known as sensory adaptation . Imagine entering a classroom with an old analog clock. Upon first entering the room, you can hear the ticking of the clock; as you begin to engage in conversation with classmates or listen to your professor greet the class, you are no longer aware of the ticking. The clock is still ticking, and that information is still affecting sensory receptors of the auditory system. The fact that you no longer perceive the sound demonstrates sensory adaptation and shows that while closely associated, sensation and perception are different.
Attention and Perception
There is another factor that affects sensation and perception: attention. Attention plays a significant role in determining what is sensed versus what is perceived. Imagine you are at a party full of music, chatter, and laughter. You get involved in an interesting conversation with a friend, and you tune out all the background noise. If someone interrupted you to ask what song had just finished playing, you would probably be unable to answer that question.
See for yourself how inattentional blindness works by watching this selective attention test from Simons and Chabris (1999):
You can view the transcript for “selective attention test” here (opens in new window) .
One of the most interesting demonstrations of how important attention is in determining our perception of the environment occurred in a famous study conducted by Daniel Simons and Christopher Chabris (1999). In this study, participants watched a video of people dressed in black and white passing basketballs. Participants were asked to count the number of times the team in white passed the ball. During the video, a person dressed in a black gorilla costume walks among the two teams. You would think that someone would notice the gorilla, right? Nearly half of the people who watched the video didn’t notice the gorilla at all, despite the fact that he was clearly visible for nine seconds. Because participants were so focused on the number of times the white team was passing the ball, they completely tuned out other visual information. Failure to notice something that is completely visible because of a lack of attention is called inattentional blindness .
In a similar experiment, researchers tested inattentional blindness by asking participants to observe images moving across a computer screen. They were instructed to focus on either white or black objects, disregarding the other color. When a red cross passed across the screen, about one third of subjects did not notice it (Figure 4) (Most, Simons, Scholl, & Chabris, 2000).
Link to Learning
Read more on inattentional blindness at the Noba Project website .
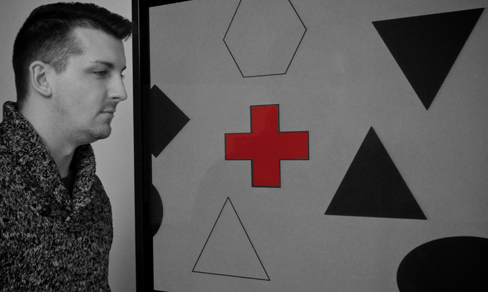
Motivations, Expectations, and Perception
Motivation can also affect perception. Have you ever been expecting a really important phone call and, while taking a shower, you think you hear the phone ringing, only to discover that it is not? If so, then you have experienced how motivation to detect a meaningful stimulus can shift our ability to discriminate between a true sensory stimulus and background noise. The ability to identify a stimulus when it is embedded in a distracting background is called signal detection theory . This might also explain why a mother is awakened by a quiet murmur from her baby but not by other sounds that occur while she is asleep. Signal detection theory has practical applications, such as increasing air traffic controller accuracy. Controllers need to be able to detect planes among many signals (blips) that appear on the radar screen and follow those planes as they move through the sky. In fact, the original work of the researcher who developed signal detection theory was focused on improving the sensitivity of air traffic controllers to plane blips (Swets, 1964).
Our perceptions can also be affected by our beliefs, values, prejudices, expectations, and life experiences. As you will see later in this module, individuals who are deprived of the experience of binocular vision during critical periods of development have trouble perceiving depth (Fawcett, Wang, & Birch, 2005). The shared experiences of people within a given cultural context can have pronounced effects on perception. For example, Marshall Segall, Donald Campbell, and Melville Herskovits (1963) published the results of a multinational study in which they demonstrated that individuals from Western cultures were more prone to experience certain types of visual illusions than individuals from non-Western cultures, and vice versa. One such illusion that Westerners were more likely to experience was the Müller-Lyer illusion (Figure 5): The lines appear to be different lengths, but they are actually the same length.
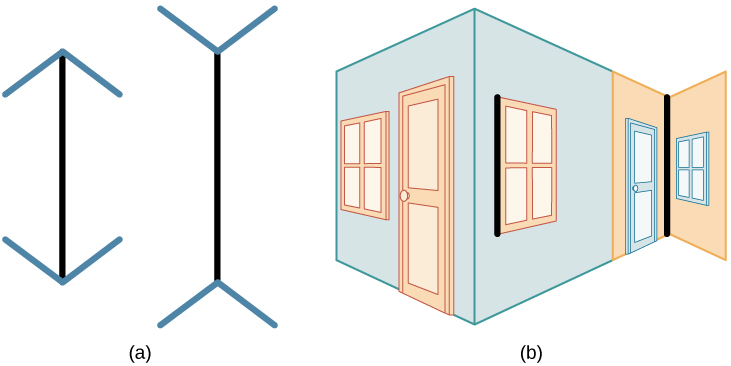
These perceptual differences were consistent with differences in the types of environmental features experienced on a regular basis by people in a given cultural context. People in Western cultures, for example, have a perceptual context of buildings with straight lines, what Segall’s study called a carpentered world (Segall et al., 1966). In contrast, people from certain non-Western cultures with an uncarpentered view, such as the Zulu of South Africa, whose villages are made up of round huts arranged in circles, are less susceptible to this illusion (Segall et al., 1999). It is not just vision that is affected by cultural factors. Indeed, research has demonstrated that the ability to identify an odor, and rate its pleasantness and its intensity, varies cross-culturally (Ayabe-Kanamura, Saito, Distel, Martínez-Gómez, & Hudson, 1998).
Children described as thrill seekers are more likely to show taste preferences for intense sour flavors (Liem, Westerbeek, Wolterink, Kok, & de Graaf, 2004), which suggests that basic aspects of personality might affect perception. Furthermore, individuals who hold positive attitudes toward reduced-fat foods are more likely to rate foods labeled as reduced fat as tasting better than people who have less positive attitudes about these products (Aaron, Mela, & Evans, 1994).
Review the differences between sensation and perception in this CrashCourse Psychology video:
You can view the transcript for “Sensation and Perception: Crash Course Psychology #5” here (opens in new window) .
Think It Over
Think about a time when you failed to notice something around you because your attention was focused elsewhere. If someone pointed it out, were you surprised that you hadn’t noticed it right away?
CC licensed content, Original
- Modification, adaptation, and original content. Provided by : Lumen Learning. License : CC BY: Attribution
CC licensed content, Shared previously
- Sensation and Perception. Authored by : OpenStax College. Located at : https://openstax.org/books/psychology-2e/pages/5-1-sensation-versus-perception . License : CC BY: Attribution . License Terms : Download for free at https://openstax.org/books/psychology-2e/pages/1-introduction
- Section on bottom-up versus top-down processing. Authored by : Dr. Scott Roberts, Dr. Ryan Curtis, Samantha Levy, and Dr. Dylan Selterman. Provided by : OpenPsyc. Located at : http://openpsyc.blogspot.com/2014/06/bottom-up-vs-top-down-processing.html . License : CC BY-NC-SA: Attribution-NonCommercial-ShareAlike
All rights reserved content
- Sensation & Perception – Crash Course Psychology #5. Provided by : CrashCourse. Located at : https://www.youtube.com/watch?v=unWnZvXJH2o . License : Other . License Terms : Standard YouTube License
way that sensory information is interpreted and consciously experienced
system in which perceptions are built from sensory input
interpretation of sensations is influenced by available knowledge, experiences, and thoughts
the reduction in sensitivity after prolonged exposure to a stimulus
failure to notice something that is completely visible because of a lack of attention
General Psychology Copyright © by OpenStax and Lumen Learning is licensed under a Creative Commons Attribution 4.0 International License , except where otherwise noted.
Share This Book

An official website of the United States government
The .gov means it’s official. Federal government websites often end in .gov or .mil. Before sharing sensitive information, make sure you’re on a federal government site.
The site is secure. The https:// ensures that you are connecting to the official website and that any information you provide is encrypted and transmitted securely.
- Publications
- Account settings
Preview improvements coming to the PMC website in October 2024. Learn More or Try it out now .
- Advanced Search
- Journal List
- HHS Author Manuscripts

The simplicity principle in perception and cognition
Jacob feldman.
Department of Psychology, Center for Cognitive Science, Rutgers University, New Brunswick, NJ
The simplicity principle, traditionally referred to as Occam’s razor, is the idea that simpler explanations of observations should be preferred to more complex ones. In recent decades the principle has been clarified via the incorporation of modern notions of computation and probability, allowing a more precise understanding of how exactly complexity minimization facilitates inference. The simplicity principle has found many applications in modern cognitive science, in contexts as diverse as perception, categorization, reasoning, and neuroscience. In all these areas, the common idea is that the mind seeks the simplest available interpretation of observations— or, more precisely, that it balances a bias towards simplicity with a somewhat opposed constraint to choose models consistent with perceptual or cognitive observations. This brief tutorial surveys some of the uses of the simplicity principle across cognitive science, emphasizing how complexity minimization in a number of forms has been incorporated into probabilistic models of inference.
Occam’s razor
The principle of simplicity or parsimony —broadly, the idea that simpler explanations of observations should be preferred to more complex ones—is conventionally attributed to William of Occam, after whom it is traditionally referred to as Occam’s razor . 1 Since then philosophers of science have adopted a bias towards simpler explanations as a foundational principle of inference, guiding the selection of hypotheses whenever multiple hypothesis are consistent with data—as is nearly always the case.
But why should simpler theories be preferred? Practicing scientists have generally assumed it is because they are actually more likely to be correct. But it has never been clear exactly why this should be so. Hume’s principle of “Uniformity of Nature” suggests that simpler theories are preferable because they make a good match for a highly regular, lawful world. Conversely, some philosophers have assumed that the bias towards simplicity is an essentially aesthetic preference, akin to elegance or beauty that mathematicians prize in theorems, conveying no particular claim to correctness (see Sober, 1975 ). Simpler theories were seen as more manageable, more comprehensible, and more testable ( Popper, 1934/1959 ), but not necessarily more truthful. In the twentieth century some authors (e.g. Quine, 1965 ; Jeffreys, 1939/1961 ) began to argue that simpler theories were, in fact, more likely to be true, but until recently the precise connection between simplicity and truth remained, at best, extremely unclear. To understand the connection, we need at the very least a more precise definition of simplicity . Such a definition only arrived in the last few decades.
Mathematical definitions of simplicity and complexity
Historically it has generally been assumed that simplicity and complexity were inherently subjective notions, impervious to clear, rigorous, or universal definitions. A theory that is simple in one method of expression may seem complex in another, implying that simplicity lies “in the eye of the beholder.” A notorious example was the competition between Julian Schwinger’s elaborate formalization of quantum thermodynamics, and Richard Feynman’s apparently simpler account (based on “funny little diagrams”)—which, notwithstanding their apparent difference in complexity, were eventually shown by Freeman Dyson to be equivalent ( Krauss, 2011 ). Cases like this seemed to imply that complexity depends on the chosen method of expression, and thus that no quantification of complexity could be universal.
Kolmogorov complexity
This all changed in the 1960s with the introduction of a principled and convincing mathematical definition of complexity now known as Kolmogorov complexity or algorithmic complexity . Introduced in slightly different forms by Solomonoff (1964) , Kolmogorov (1965) , and Chaitin (1966) , the main idea is that the complexity of a string of characters reflects the degree of incompressibility , as measured by the length of a computer program required to faithfully express the string (see M. Li & Vitányi, 1997 ). Simple strings are those that can be expressed by brief computer programs, and complex datasets are those that cannot. The idea is usually formalized by considering a string S (a sequence of symbols), and then considering the length (in symbols) of the shortest computer program capable of generating it S . For example, the loop (in pseudocode) “ for i=1 to 1000: print '1' ” prints a string of 1000 characters, but the program itself contains only 26; this string is highly compressible. By contrast a “typical” random string of 1000 characters (e.g. “ 39383827262226 …”) can’t be compressed in this way, although it can be expressed by a program that is itself about 1000 characters long (e.g. “ Print '9486390348473969683…' ”, which has 1008 characters). More generally, a string that contains regularities or patterns—of any form that can be expressed in the computer language—can be faithfully reproduced by a short program that takes advantages of these patterns, while a relatively complex or “random” string cannot be similarly compressed. This measure of complexity is inherently capped at approximately the length of the original string, because any string, no matter how irregular, can be reproduced exactly simply by quoting it verbatim as in the example above. In this view, simplicity is essentially compressibility .
Critically, Kolmogorov complexity is universal in the sense that it does not depend “very much” on the computer language in which the program is written. Turing (1937) had demonstrated the existence of computers that can, in a well-defined sense, carry out any concretely specifiable algorithm, now referred to as universal Turing machines. In modern terminology, we can think of them as computer languages that are general enough to express any computable function—including, critically, to “simulate” other computer languages. Assume a string S that can be expressed by computer language L 1 in K 1 ( S ) steps, meaning that its Kolmogorov complexity is at least as low as K 1 ( S ). Assume that some other language L 2 can be expressed in language L 1 in a finite number | L 2 | of steps—in modern terms, | L 2 | is the length of a compiler for language L 2 written in language L 1 . It follows fairly immediately that string S can be expressed in language L 2 in | L 2 | + K 1 ( S ) steps, meaning that the complexity of S in language L 2 (i.e. K 2 ( S )) is at least as low as | L 2 |+ K 1 ( S )—because that’s how many steps it takes to translate L 2 into L 1 and then express S in L 2 . The “translation component” | L 2 | may be very large, if the computer languages are very different, but it is finite—and, critically, it does not depend on the length of the string S . This means that as strings get longer and longer, the translation component of their complexity matters less and less, and in this sense their complexity is asymptotically independent of the programming language. For this reason the Kolmogorov complexity K ( S ) of a string S is usually thought of as a universal measure of its inherent complexity or randomness.
Note that the actual value of the K ( S ) is uncomputable. 2 For long strings it can be approximated by effective string-compression algorithms such as Lempel-Ziv ( M. Li & Vitányi, 1997 ) implemented in the common utility gzip ( Ziv & Lempel, 1977 ), meaning that the Kolmogorov complexity of a long string S is approximately the length, in characters, of the gzipped version of S . For shorter strings such approximations are in principle less reliable, though recent work by Gauvrit, Singmann, Soler-Toscano, and Zenil (2016) has for the first time provided practical techniques for estimating the Kolmogorov complexity of short strings, opening an intriguing research avenue for evaluating the role of complexity in psychological models.
Information-theoretic Description Length
Another important approach to the quantification of complexity was initiated by Shannon (1948) . Shannon showed that in a set of messages m 1 , m 2 , … which occur with probability p 1 , p 2 …, each message conveys information given by −log p i , which quantifies the degree of “surprise” or unexpectedness entailed by the message. Consequently, if one seeks to convey a set of messages in the fewest symbols possible, one should adopt a coding language in which each message m i is assigned a code of length approximately −log p i symbols. Such a procedure will minimize the expected total code length, that is, achieve the most compressed expression possible. As a result, the quantity −log p i is sometimes referred to as the Description Length (DL). The DL of a message is in effect a measure of complexity, because it quantifies how many symbols are required to express m i in a maximally compressed code. That is, just like Kolmogorov complexity, the DL quantifies how many symbols are required to express a particular message after maximal compression . In this way Shannon showed that complexity is intimately related to probability, a profound insight that pervades the modern understanding of both concepts.
Rissanen (1989) took the next step by elevating this insight into a fundamental principle of inference, which he called the Minimum Description Length (MDL) principle (see Grünwald, 2005 , and compare the closely related approach referred to as Minimum Message Length ; Wallace, 2004 ). In its classic formulation, the MDL principle begins by imagining that we are trying to explain some data X via some set of alternative models Y i . For any given model Y , the joint probability p ( X ∧ Y ) that both model and data are true can be written as p ( X | Y ) p ( Y ), the product of the probability of the model and the probability of the data conditioned on the model. The DL of this conjunction, that is, its negative log probability, is simply
That is, the complexity (DL) of the model and data is the sum of the complexity of the model, plus the complexity of the data given the model—bearing in mind that, via Shannon’s definition, the “complexity” of the data is really its surprisingness given the model. This neat additive formulation captures something very basic about scientific theorizing: that we are trying to simultaneously minimize the complexity of our theories and the unexpectedness (surprise) of the data given our theories—that is, that we seek elegant models that also explain the data reasonably well. This perfectly encapsulates Einstein’s (perhaps aprocryphal) quip that our theories should be “as simple as possible, but no simpler.”
Bayesian inference
The intimate relationship between Occam’s razor and rational probabilistic inference was probably first pointed out by Jeffreys (1939/1961) , one of the principal developers of the modern conception of Bayesian inference. Jeffreys argued in some detail that the simplest interpretation was indeed the most likely one, and in particular advocated adopting priors that penalize complexity, that is, placing higher priors on simpler models and lower priors on more complex ones. More recently, Edwards (1972) has also argued that probability theory inherently favors simpler inductions, though on the basis of the likelihood rather than the prior.
The close connection between Occam’s razor and Bayes’ rule can be appreciated most directly simply by observing that the hypothesis with the highest posterior is, ipso facto, also the hypothesis iwth the minimum DL in Shannon’s sense. In a Bayesian framework, the posterior belief in hypothesis H after considering data D , notated p ( H | D ), is proportional to the product of its prior p ( H ) and its likelihood p ( D | H ),
(see Yuille & Bülthoff, 1996 or Feldman, 2014 for tutorial introductions). The hypothesis that maximizes this quantity, sometimes called the maximum a posteriori or MAP, is the hypothesis that is the most probable, in that it maximizes the tradeoff between prior plausibility and fit to the observed data. Hence in this simple sense, if one assumes an optimal description language in the sense defined by Shannon, the winning hypothesis is both the most probable and the simplest.
Even under broader set of assumptions, Bayesian inference inherently favors simpler hypotheses because of the way it assigns probability ( MacKay, 2003 ), a tendency often referred to as the “Bayes occam” factor. In practice, a Bayesian hypothesis space often consists of one more more parameterized families of hypotheses. The more parameters a family has, the smaller the probability volume devoted to each individual hypothesis (that is, each setting of the parameters), since the total probability assigned to all hypotheses must sum to one. Hence if one thinks of the number of parameters as a measure of the complexity of the model family, the prior necessarily decreases with complexity. A similar argument applies to the likelihood as well ( Tenenbaum & Griffiths, 2001 ), suggesting that Bayesian inference automatically favors more restrictive (i.e., simpler) hypotheses even without an overt prior bias.
In the modern literature on machine learning and statistical learning, the intimate connection between simplicity and probability is part of what is called the bias / variance trade-off , a terminology introduced by Geman, Bienenstock, and Doursat (1992) . Very broadly speaking, more complex theories are inherently—that is, by virtue of their complexity and thus flexibility—capable of more precise fits to training data, but simpler theories tend to generalize better. This leads to a trade-off in which optimal inference requires a balance between adhering to data and preferring simpler theories (see Duda, Hart, & Stork, 2001 or Hastie, Tibshirani, & Friedman, 2001 for discussion). This tradeoff is widely regarded as a central aspect of all probabilistic inference, and is yet another reason why probability and simplicity are intertwined; almost regardless of the nature of the inference problem, a bias towards simpler theories (in this context sometimes called regularization) is required in order to prevent overfitting the training data and thus generalizing poorly.
Finally, notice that just as one can create a complexity from a probability by taking a negative logarithm, one can create a probability from a complexity by exponentiation. Given a set of strings S each having Kolmogorov complexity K ( S ), one can construct a set of probabilities
(see M. Li & Vitányi, 1997 ). Such a distribution assigns higher probability to simpler strings (here thought of as models of data), and lower probability to more complex ones. This construction may seem contrived, but, as Solomonoff (1964) observed, it yields a set of probabilities—for example, a prior in the Bayesian sense—that is universal in precisely the same sense that Kolmogorov complexity itself is universal: namely, that is approximately correct regardless of the details of the coding language. Such a “universal prior” closes the loop connecting probability to complexity.
This connection between simplicity and probability has many nuances not mentioned here, and is not without controversy (see MacKay, 2003 for a more substantial discussion), and is viewed somewhat differently by those from Bayesian and information-theoretic traditions (see Burnham & Anderson, 2002 ). However, notwithstanding the many subtleties, it is important to understand that in the modern technical literature and in cognitive science, Bayesian inference and complexity minimization are usually treated as deeply intertwined, if not practically the same thing.
The simplicity principle in psychology
In psychology and cognitive science, the simplicity principle posits that the mind draws interpretations of the world—mental models or mental representations—that are as simple as possible, or, at least, that are biased towards simplicity ( Chater, 1997 ; Chater & Vitányi, 2003 ). The idea takes different forms in different areas of cognition, depending on the nature of the many perceptual and cognitive problems the mind encounters: perceptual interpretations of sense data, memory encodings of experience, causal interpretations of observations, and so forth. In MDL and Bayesian formulations, the principle can be extended to allow a tradeoff, inherent in these frameworks, between simplicity and consistency with sense data and experience—meaning that the interpretation drawn by the mind in light of simplicity may not actually be consistent with observation. Nevertheless in many areas of cognition, briefly surveyed in the next few sections, researchers have found that human thought incorporates a bias towards simplicity.
Note that complexity often arises in psychological experiments as a nuisance variable, simply because it can have such a salient effect on performance. Many experiments include simple and complex conditions (often labeled in other ways such as “high-load” and “low-load”, etc.), even when complexity per se is not the main topic of inquiry. Typically, “complex” conditions simply involve a larger number of items or features, although it should be noted that the sheer number of elements in a construct is not generally a good proxy for complexity, since (as will be seen below) patterns with an equal number of elements can vary widely in regularity or compressibility. This review will not generally include such studies, but will instead focus on studies in which the bias towards simplicity is the main topic of interest.
The principle of simplicity first arose in perceptual psychology via the Gestalt notion of Prägnanz , a broad term meant to encompass “such properties as regularity, symmetry, simplicity, and others” ( Koffka, 1935 ). The idea is that the mind prefers coherent and plausible interpretations of sensory data, for example interpreting contours as the boundaries of objects, completing shapes plausibly behind occluders, and so forth. Notwithstanding its somewhat vague definition, Prägnanz is often thought of as a kind of simplicity principle, sometimes under the rubric minimum principle (see Boselie & Wouterlood, 1989 ; Kanizsa, 1979 ). The idea is that more coherent or “Prägnant” interpretations are in some sense simpler than alternatives ( Hatfield & Epstein, 1985 ).
In the 1950s, following the introduction of computers and the dissemination of Shannon’s ideas about information, some psychologists began to take up information-theoretic quantifications of complexity and Prägnanz. Attneave (1954) ’s influential paper expressed the idea of simplicity in terms of “economy of perceptual description,” and for the first time compared Shannon’s formal information measure to human performance. Around the same time, Hochberg and McAlister (1953) quantified the complexity of a stimulus in a Kolmogorov-like way (a decade before Kolmogorov, Chaitin and Solomonoff), adding up the number of steps in the simplest generative procedure required to replicate a stimulus (e.g., the number of segments, turns, corners and bends required to recreate a given line drawing; see also Hochberg, 1964 ). They demonstrated that subjects shown an ambiguous figure preferred interpretations in inverse proportion to their complexity quantified in this manner.
The attempt to create a general complexity measure for perceptual interpretations reached a greater level of sophistication in the work of Leeuwenberg (1971) . Leeuwenberg, along with his followers in the tradition later known as Structural Information Theory, articulated a coding language based on pattern repetitions, symmetries, and, later, other kinds of regularities ( van der Helm, 2014 , 2015 ). Predictions derived from the theory have been used to account for various phenomena of visual completion (e.g. van Lier, Leeuwenberg, & van der Helm, 1995 ; van Lier, van der Helm, & Leeuwenberg, 1994 ) as well as motion interpretation ( Restle, 1979 ). While these results are impressive, it should be noted that complexity in a fixed coding language such as SIT’s cannot necessarily be assumed to be universal in the sense of Kolmogorov complexity unless the language has been shown to be able to express all visual patterns (including shading, color, texture, etc., which the SIT coding language does not usually include) which to the author’s knowledge never been demonstrated.
Regardless of the details of the complexity measure, the simplicity principle in visual perception has often been placed in opposition to the Likelihood principle (see Hatfield & Epstein, 1985 ; Pomerantz & Kubovy, 1986 ), which is the tendency of the visual system to see the most probable interpretation (see e.g. Boselie & Leeuwenberg, 1986 ; Leeuwenberg & Boselie, 1988 ; Moravec & Beck, 1986 ), which is in turn descended from notions of ecological probability in perception originated by Egon Brunswik ( Brunswik & Kamiya, 1953 ; Brunswik, 1956 ). However as discussed above, complexity minimiziation and probabilistic inference are now recognized to be closely aligned, and indeed not always clearly distinguishable from each other. In the perception literature this connection was first recognized in an influential paper by Chater (1996) , who argued that in many contexts the simplest visual interpretation is also the most likely to be veridical. The more specific connection between Bayesian inference and complexity minimization has been explored in a number of places since (e.g. Vitanyi & Li, 2000 ; Feldman, 2009 ).
The idea that the visual system chooses the simplest model consistent with visual input was expressed in a particularly memorable way in an influential paper by Adelson and Pentland (1996) . They imagined the process of scene model construction via a metaphor in which the scene must be created by a combination of a metalworker, a painter, and a lighting designer, each of whom charges fees for constructive operations such creating a surface, bending a surface, painting a surface, or adding a light source. The brain’s task is to construct a scene consistent with the image data for the least cost—in other words, to construct a scene model with minimum complexity in this particular “coding language.” Because the coding language is in principle capable of generating any observable scene (albeit possibly with an enormous number of surfaces and colors, etc.), this dollar cost is a close analog of the Kolmogorov complexity. Simple images are those that can be rendered via inexpensive models, and the simplest (cheapest) model of the image is the most likely hypothesis about what arrangement of surfaces in the real world actually generated it.
The role of simplicity in vision has been particularly prominent in relation to perceptual organization and vision, where it originated. The work of Leeuwenberg and his followers on simplicity in perceptual organization has already been mentioned. In the computational literature, Darrell, Sclaroff, and Pentland (1990) showed how the visual image can be parsed into coherent objects by choosing the decomposition with minimum DL. Feldman (1997) similarly showed how the most plausible grouping interpretation can be chosen via a suitably chosen complexity minimization. Similarly, configurations of dots are clustered in part based on simplicity criteria ( Gershman & Niv, 2013 ). Regardless of the specifics of the complexity measures, all these results suggest that the human visual system divides the image into coherent units in part based on the simplicity principle.
Similar principles govern how individual objects are represented. In the Bayesian shape representation framework of Feldman and Singh (2006) , individual shapes are parsed into individual parts by choosing the simplest (MDL) skeletal representation consistent with the bounding contour. A closely related complexity measure has been shown to influence the detectability of both open contours in noise ( Wilder, Feldman, & Singh, 2015a ) as well as closed contours, that is, whole shapes ( Wilder, Feldman, & Singh, 2015b ). Finally, a simplicity bias influences how the visual system interpets 3D structure in line drawings; the system apparently chooses the simplest 3D shape consistent with the configuration of line elements ( Y. Li & Pizlo, 2011 ).
Categorization and concept learning
The role of simplicity biases was recognized early in the machine learning literature (e.g. Medin, Wattenmaker, & Michalski, 1987 ; Iba, Wogulis, & Langley, 1988 ). Algorithmic approaches to learning have grown enoromously since then, diverging into a number of frameworks with their own complexity measures. PAC(“probably approximately correct”) learning, introduced by Valiant (1984) , often uses a complexity measure called VC (Vapnik-Chervonenkis) dimension (see Abu-Mostafa, 1989 ), which as with nearly all complexity measures relates to how model complexity needs to be constrained in order to ensure learnability. As mentioned above, statistical learning theory, including the theory of neural networks (e.g. Poggio, Rifkin, Mukherjee, & Niyogi, 2004 ) more generally assumes a tradeoff between model complexity and fit to training data in order to promote effective generalization.
In the psychological literature on concept learning, the role of simplicity was noticed early ( Neisser & Weene, 1962 ; Haygood, 1963 ; Looney & Haygood, 1968 ). Rosch (1978) articulated a “principle of cognitive economy” as a motivation for why the mind reflexively organizes the world into coherent categories. However this idea actually played relatively little role in the models that dominated the categorization literature for the next several decades, exemplar models (e.g. Nosofsky, 1986 ; Kruschke, 1992 ). Exemplar models assume that categorization is a by-product of the storage of specific examples, which are then used as standards against which to judge the category membership of future examples. Exemplar models do not have an overt simplicity bias, because they do not involve any abstraction process per se, although later analysis made it clear that they implicitly regularize to a degree modulated by certain parameter settings ( Jäkel, Schölkopf, & Wichmann, 2008 ; Briscoe & Feldman, 2006 ). Later “hybrid” (prototype plus exemplar) models, such as that of Nosofsky, Palmeri, and McKinley (1994) and others that followed, posited that learning proceeds by discovering collections of items that are well-described by a simple rule, which can be stored separately from “exceptional” (irregular) items; such a strategy obviously requires an overt simplicity bias. Pothos and Chater (2001) showed that unsupervised categorization too can be understood as a process of complexity minimization, using an MDL criterion that maximizes similarity within clusters and minimizes it between them. Similarly, Hahn, Chater, and Richardson (2003) showed how similarity , an essential construct in almost all categorization models, can be understood in terms of the Kolmogorov complexity of the transformation between objects.
The role of complexity in category learning has been studied the most directly in the context of Boolean categories, that is, categories built out of combinations of binary features ( Feldman, 2003 ). Because Boolean categories involve finite combinations of discrete features, it is possible to test them comprehensively, including every distinct logical type ( Shepard, Hovland, & Jenkins, 1961 ). In early work, several studies had suggested that the subjective difficulty of Boolean concepts could be tied to their logical complexity ( Haygood, 1963 ; Neisser & Weene, 1962 ). More recently, Feldman (2000) undertook a more comprehensive study incorporating a much larger set of concept types. The results show that subjects’ ability to learn Boolean concepts declines with their inherent logical complexity, suggesting (yet again) a bias towards simplicity in learning.
In its traditional definition (see Wegener, 1987 ), Boolean complexity is defined as the length (in variable names, or literals) of the shortest propositional formula equivalent to a given set of examples. For example, the propositional formula ( A ∧ B ) ∨ ( A ∧ B ′) describes two training examples, one with features A and B , the other with features A and not B . (In this notation, A and B are features, A ′ is the negation of feature A , ∧ means “and,” and ∨ means “or.”) This expression can be reduced to A ∧ ( B ∨ B ′) which in turn reduces to A , meaning that the original examples can be fully expressed simply by their common feature A ; its Boolean complexity is 1. By contrast, the examples ( A ∧ B ) ∨ ( A ′ ∧ B ′) cannot be reduced at all (it is “incompressible”) so its Boolean complexity is 4. This definition parallels that of Kolmogorov complexity, in that it quantifies the length of the shortest faithful representation of the original formula, and enjoys an analogous kind of universality: Boolean complexity is universal across logical bases (i.e. choice of connectives) up to a multiplicative factor.
The simplicity bias in Boolean concept learning has been corroborated in a number of studies, though there are still a number of distinct views about how to properly formulate the complexity measure ( Lafond, Lacouture, & Mineau, 2007 ; Vigo, 2009 ; Fass & Feldman, 2002 ; Mathy, 2010 ; Aitkin & Feldman, 2006 ; Feldman, 2006 ). Mathy and collaborators have measured complexity in terms of the length of a decision tree ( Mathy & Bradmetz, 2004 ), and even shown that response times can be tied to the process of decompression from a simplified representation ( Bradmetz & Mathy, 2008 ). Finally, Goodman, Tenenbaum, Feldman, and Griffiths (2008) were able to explain a large swath of concept learning data with a Bayesian model that assigns probability to logical formulae (i.e., models) in proportion to the length of their derivation in a context-free grammar, thus in effect favoring simple models over more complex ones.
Compressibility has a particularly direct connection with memory, because it is obviously advantageous for any memory system to compress material before storing it. The more information can be effectively compressed—that is, the lower its Kolmogorov complexity—the more can be stored. Mathy and Feldman (2012) demonstrated this connection fairly directly by manipulating the compressibility of digit sequences to be held by subjects in verbal short term memory (STM), for example including “runs” (chains of rising or falling digits, like 3-4-5-6-7 or 8-7-6) of various lengths. The more regular (and thus compressible) the sequence, the more digits ordinary subjects could retain correctly. The implication is that verbal STM incorporates an active compression or pattern-finding mechanism which allows it to minimize memory resources. Children, too, show an effect of compressibility, meaning that their verbal STM capacity also varies in direct proportion to the compressiblity of the material to be remembered ( Mathy, Fartoukh, Gauvrit, & Guida, 2016 ). Intriguingly, while their digit span rises with age, the effect of compressibility is apparently constant, meaning that as children develop they retain a fixed capacity to compress information.
A closely related debate involves the capacity of visual STM, the buffer in which visual information is briefly held. Like verbal STM, visual STM had traditionally been assumed to contain a fixed number (about 3 or 4) of slots without regard to information load. But this “fixed slots” view has been challenged in favor of a “continuous-resource” view in which memory resources are flexibly allocated depending on intrinsic information load. For example, several studies have found that the number of items stored depends on the complexity of each item ( Luria, Sessa, Gotler, Jolicoeur, & Dell’Acqua, 2010 ) or the precision with which each is represented ( Alvarez & Cavanagh, 2004 ), implying that capacity is bound by the total information content rather than by a fixed slot limit. Ma, Husain, and Bays (2014) provide a good recent summary, concluding that visual STM capacity depends on a continuous information limit rather than a set of discrete slots. All these findings relate directly to the simplicity bias, because they imply an underlying compression system in which information is reflexively represented in the most parsimonious way possible. Although the nature of the underlying neural code is not yet well understood, recent models assume a maximally compressed code that is efficient in the Shannon sense ( Sims, Jacobs, & Knill, 2012 ).
Causal reasoning
Another natural setting for a simplicity bias is in the inference of causal explanations from observations. When a doctor is confronted with a set of symptoms (say, fatigue, fever, and a runny nose) it is simpler and thus more reasonable to diagnose a single cause (the flu) rather than a set of distinct causes (anemia, sepsis, and seasonal allergies). Here again the simplicity bias can be described in Bayesian terms, as the assignment of a higher prior to a single cause than to a set of three distinct causes (which, being independent, would have a prior approximately proportional the third power of that of a single cause, a much lower number). The confidence inspired by a simple explanation of a complex set of facts ( Eureka! ) derives in part from the fact that it is unlikely for a simple theory to fit “by accident” ( Feldman, 2004 ). Accordingly, Little and Shiffrin (2009) found that subjects favor simple explanations of data (e.g., lower-degree polynomial models) over more complex (higher-degree) explanations. Similarly, studies of children’s explanations of causal relations have found that they too favor explanations that minimize the number of distinct causes ( Lombrozo, 2007 ; Bonawitz & Lombrozo, 2012 ).
Neuroscience
Finally, we briefly mention the role that complexity, and in particular minimization of coding length, plays in theoretical neuroscience. Barlow (1961) famously advocated efficient coding as a fundamental principle of sensory representation, arguing that the brain encodes sensory signals so as to minimize the redundancy of the raw stimulus array. This idea is essentially equivalent to the notion of compression later connected with Kolmogorov complexity, as it entails an Occam-like compression of the raw sensory signal in order to extract the regularities latent in the raw sensory signal.
Barlow’s idea has exerted a profound and far-reaching influence on neursocience in the decades since. The idea that neural networks extract regularity from sensory data, sometimes referred to as dimensionality reduction , is a central principle of theoretical neuroscience ( Hinton & Salakhutdinov, 2006 ). Similarly, neural receptive fields are now thought to be designed so as to optimally (that is, with maximal informational efficiency) encode visual stimuli ( Field, 1987 ). Another important development along the same lines is in the quantification of information along neural spike trains, which is based on the idea that the sequence of action potential constitutes an optimally efficient encoding of the information conveyed by sensory receptors ( Rieke, Warland, de Ruyter van Steveninck, & Bialek, 1996 ). All these developments have in common an Occam-like reduction of the complexity of the raw stimulus array in order to further the behavioral goals of the organism.
Finally note that the brain’s neural circuitry itself, viewed from the perspective of graph theory, seems to minimize circuit complexity and other computational costs ( Bullmore & Sporns, 2012 ). While this is admittedly speculative, it is possible that (in some not-yet-understood sense) the mental bias towards relatively simple interpretations of the world might be related to a neural bias towards simplicity in the underlying neural architecture.
As the many examples above illustrate, a bias towards simplicity pervades mental function. Examples can be found in perception, learning, categorization, reasoning, and neuroscience. Some of these findings involve Kolmogorov complexity directly; others involve information-theoretic concepts like DL (negative log probability) and information load; and others involve simplicity biases that arise in the context of Bayesian inference—all of which are closely related from a mathematical point of view. Broadly speaking, it may be that, as Hume first suggested, the mind cannot apprehend the world without assuming some form of underlying regularity, an idea sometimes called the “Principle of Natural Modes” ( Richards & Bobick, 1988 ).
However, notwithstanding the ubiquity of simplicity biases, it actually remains unclear whether Occam’s razor is, in fact, a primary driving principle of human inference. As discussed above, simplicity biases are deeply intertwined with—indeed scarcely separable from—information theory and Bayesian probability theory. From the point of view of modern theory, almost any form of rational inference will entail some kind of simplicity bias. Hence rather than being a foundational principle, the human simplicity bias may simply be an epiphenomenon of a more basic goal of mental function, such as veridicality ( Pizlo, Sawada, Li, Kropatsch, & Steinman, 2010 ), optimal estimation ( Knill, Kersten, & Yuille, 1996 ), or, perhaps most fundamentally, adaptive functionality ( Hoffman, Singh, & Prakash, 2015 ).
Acknowledgments
This research was supported by NIH (NEI) Grant EY021494. I am grateful to Manish Singh and the member of the Visual Cognition Lab for many stimulating discussions.
1 Occam himself was arguing against the existence of “universals” (i.e., generalizations), maintaining that one should not posit the existence of entities beyond those that can be directly observed; see Hannam, 2009 .
2 Kolmogorov complexity is uncomputable for essentially the same reason there is no “smallest uninteresting number”—if there were, that would indeed be very interesting. (See Chaitin, 1974 on what Bertrand Russell called the Berry paradox.) Similarly, if there were a computable procedure for computing K ( S ), then some strings of complexity at least K ( S ) could be reproduced by a program of the form “Print the shortest string with complexity K ( S ).” Such a program would take fewer than K ( S ) characters to encode—a contradiction. See Schöning and Pruim (1998) for a more careful discussion.
- Abu-Mostafa YS. The Vapnik-Chervonenkis dimension: Information versus complexity in learning. Neural Computation. 1989; 1 :312–317. [ Google Scholar ]
- Adelson EH, Pentland AP. The perception of shading and reflectance. In: Knill David C, Richards W, editors. Perception as Bayesian inference. Cambridge: Cambridge University Press; 1996. pp. 409–423. [ Google Scholar ]
- Aitkin CD, Feldman J. Subjective complexity of categories defined over three-valued features; Proceedings of the 28th conference of the cognitive science society; 2006. pp. 961¢–966¢. [ Google Scholar ]
- Alvarez GA, Cavanagh P. The capacity of visual short-term memory is set both by visual information load and by number of objects. Psychol Sci. 2004; 15 (2):106–111. [ PubMed ] [ Google Scholar ]
- Attneave F. Some informational aspects of visual perception. Psychological Review. 1954; 61 :183–193. [ PubMed ] [ Google Scholar ]
- Barlow HB. In: Possible principles underlying the transformation of sensory messages. Rosenblith WA, editor. Cambridge: M.I.T. Press; 1961. pp. 217–234. [ Google Scholar ]
- Bonawitz EB, Lombrozo T. Occam’s rattle: children’s use of simplicity and probability to constrain inference. Developmental Psychology. 2012; 48 (4):1156–1164. [ PubMed ] [ Google Scholar ]
- Boselie F, Leeuwenberg E. A test of the minimum principle requires a perceptual coding system. Perception. 1986; 15 :331–354. [ PubMed ] [ Google Scholar ]
- Boselie F, Wouterlood D. The minimum principle and visual pattern completion. Psychological Research. 1989; 51 :93–101. [ PubMed ] [ Google Scholar ]
- Bradmetz J, Mathy F. Response times seen as decompression times in Boolean concept use. Psychological Research. 2008; 72 :211–234. [ PubMed ] [ Google Scholar ]
- Briscoe E, Feldman J. Conceptual complexity and the bias-variance tradeoff; Proceedings of the conference of the cognitive science society; 2006. pp. 1038–1043. [ Google Scholar ]
- Brunswik E. Perception and the representative design of psychological experiments. Berkeley: University of California Press; 1956. [ Google Scholar ]
- Brunswik E, Kamiya J. Ecological cue-validity of ‘proximity’ and of other Gestalt factors. American Journal of Psychology. 1953; 66 :20–32. [ PubMed ] [ Google Scholar ]
- Bullmore E, Sporns O. The economy of brain network organization. Nature Reviews Neuroscience. 2012; 13 (5):336–349. [ PubMed ] [ Google Scholar ]
- Burnham KP, Anderson DR. Model selection and multi-model inference: A practical information-theoretic approach. New York: Springer; 2002. [ Google Scholar ]
- Chaitin GJ. On the length of programs for computing finite binary sequences. Journal of the Association for Computing Machinery. 1966; 13 :547–569. [ Google Scholar ]
- Chaitin GJ. Information-theoretic computational complexity. IEEE Transactions on Information Theory. 1974; IT-20 (1):10–15. [ Google Scholar ]
- Chater N. Reconciling simplicity and likelihood principles in perceptual organization. Psychological Review. 1996; 103 (3):566–581. [ PubMed ] [ Google Scholar ]
- Chater N. Simplicity and the mind. Psychologist. 1997; 10 (11):495–498. [ Google Scholar ]
- Chater N, Vitányi P. Simplicity: a unifying principle in cognitive science. Trends in Cognitive Sciences. 2003; 7 (1):19–22. [ PubMed ] [ Google Scholar ]
- Darrell T, Sclaroff S, Pentland A. Proceedings third international conference on computer vision. Los Alamitos, CA: IEEE Computer Society Press; 1990. Segmentation by minimal description. [ Google Scholar ]
- Duda RO, Hart PE, Stork DG. Pattern classification. New York: John Wiley and Sons, Inc; 2001. [ Google Scholar ]
- Edwards AWF. Likelihood. Cambridge: Cambridge University Press; 1972. [ Google Scholar ]
- Fass D, Feldman J. Categorization under complexity: a unified MDL account of human learning of regular and irregular categories. In: Becker S, Thrun S, Obermayer K, editors. Advances in neural information processing 15. Cambridge: MIT Press; 2002. [ Google Scholar ]
- Feldman J. Regularity-based perceptual grouping. Computational Intelligence. 1997; 13 (4):582–623. [ Google Scholar ]
- Feldman J. Minimization of Boolean complexity in human concept learning. Nature. 2000; 407 :630–633. [ PubMed ] [ Google Scholar ]
- Feldman J. The simplicity principle in human concept learning. Current Directions in Psychological Science. 2003; 12 (6):227–232. [ Google Scholar ]
- Feldman J. How surprising is a simple pattern? Quantifying “Eureka!” Cognition. 2004; 93 :199–224. [ PubMed ] [ Google Scholar ]
- Feldman J. An algebra of human concept learning. Journal of Mathematical Psychology. 2006; 50 :339–368. [ Google Scholar ]
- Feldman J. Bayes and the simplicity principle in perception. Psychological Review. 2009; 116 (4):875–887. [ PubMed ] [ Google Scholar ]
- Feldman J. Bayesian models of perceptual organization. In: Wagemans J, editor. Handbook of perceptual organization. Oxford: Oxford University Press; 2014. [ Google Scholar ]
- Feldman J, Singh M. Bayesian estimation of the shape skeleton. Proceedings of the National Academy of Sciences. 2006; 103 (47):18014–18019. [ PMC free article ] [ PubMed ] [ Google Scholar ]
- Field DJ. Relations between the statistics of natural images and the response properties of cortical cells. J. Optical Society of America A. 1987; 4 (12):2379–2394. [ PubMed ] [ Google Scholar ]
- Gauvrit N, Singmann H, Soler-Toscano F, Zenil H. Algorithmic complexity for psychology: a user-friendly implementation of the coding theorem method. Behavioral Research Methods. 2016; 48 (1):314–329. [ PubMed ] [ Google Scholar ]
- Geman S, Bienenstock E, Doursat R. Neural networks and the bias/variance dilemma. Neural computation. 1992; 4 :1–58. [ Google Scholar ]
- Gershman SJ, Niv Y. Perceptual estimation obeys Occam’s razor. Frontiers in Psychology. 2013; 4 :623. [ PMC free article ] [ PubMed ] [ Google Scholar ]
- Goodman ND, Tenenbaum JB, Feldman J, Griffiths TL. A rational analysis of rule-based concept learning. Cognitive Science. 2008; 32 (1):108–154. [ PubMed ] [ Google Scholar ]
- Grünwald PD. A tutorial introduction to the minimum description length principle. In: Grünwald PD, Myung IJ, Pitt M, editors. Advances in minimum description length: Theory and applications. Cambridge, MA: MIT press; 2005. [ Google Scholar ]
- Hahn U, Chater N, Richardson LB. Similarity as transformation. Cognition. 2003; 87 :1–32. [ PubMed ] [ Google Scholar ]
- Hannam J. God’s philosophers: How the medieval world laid the foundations of modern science. Icon Books; 2009. [ Google Scholar ]
- Hastie T, Tibshirani R, Friedman J. The elements of statistical learning: Data mining, inference, and prediction. New York: Springer; 2001. [ Google Scholar ]
- Hatfield G, Epstein W. The status of the minimum principle in the theoretical analysis of visual perception. Psychological Bulletin. 1985; 97 (2):155–186. [ PubMed ] [ Google Scholar ]
- Haygood RC. Unpublished doctoral dissertation. University of Utah; 1963. Rule and attribute learning as aspects of conceptual behavior. [ Google Scholar ]
- Hinton GE, Salakhutdinov RR. Reducing the dimensionality of data with neural networks. Science. 2006; 313 (5786):504–507. [ PubMed ] [ Google Scholar ]
- Hochberg J. Perception. New Jersey: Pretince-Hall; 1964. [ Google Scholar ]
- Hochberg J, McAlister E. A quantitative approach to figural “goodness” Journal of Experimental Psychology. 1953; 46 :361–364. [ PubMed ] [ Google Scholar ]
- Hoffman DD, Singh M, Prakash C. The interface theory of perception. Psychonomic Bulletin and Review. 2015; 22 (6):1480–1506. [ PubMed ] [ Google Scholar ]
- Iba W, Wogulis J, Langley P. Proceedings of the fifth international conference on machine learning. Ann Arbor, MI; 1988. Trading off simplicity and coverage in incremental concept learning; p. 7379. [ Google Scholar ]
- Jäkel F, Schölkopf B, Wichmann F. Generalization and similarity in exemplar models of categorization: Insights from machine learning. Psychonomic Bulletin and Review. 2008; 15 (2):256–271. [ PubMed ] [ Google Scholar ]
- Jeffreys H. Theory of probability. third. Oxford: Clarendon Press; 1939/1961. [ Google Scholar ]
- Kanizsa G. Organization in vision: essays on Gestalt perception. New York: Praeger Publishers; 1979. [ Google Scholar ]
- Knill DC, Kersten D, Yuille A. Introduction: a Bayesian formulation of visual perception. In: Knill DC, Richards W, editors. Perception as Bayesian inference. Cambridge: Cambridge University Press; 1996. pp. 123–162. [ Google Scholar ]
- Koffka K. Principles of Gestalt psychology. New York: Harcourt; 1935. [ Google Scholar ]
- Kolmogorov AN. Three approaches to the quantitative definition of information. Problems of Information Transmission. 1965; 1 (1):1–7. [ Google Scholar ]
- Krauss LM. Quantum man: Richard feynman’s life in science. New York: Atlas & Co; 2011. [ Google Scholar ]
- Kruschke J. ALCOVE: An exemplar-based connectionist model of category learning. Psychological Review. 1992; 99 (1):22–44. [ PubMed ] [ Google Scholar ]
- Lafond D, Lacouture Y, Mineau G. Complexity minimization in rule-based category learning: Revising the catalog of Boolean concepts and evidence for non-minimal rules. Journal of Mathematical Psychology. 2007; 51 :57–74. [ Google Scholar ]
- Leeuwenberg ELJ. A perceptual coding language for visual and auditory patterns. American Journal of Psychology. 1971; 84 (3):307–349. [ PubMed ] [ Google Scholar ]
- Leeuwenberg ELJ, Boselie F. Against the likelihood principle in visual form perception. Psychological Review. 1988; 95 :485–491. [ PubMed ] [ Google Scholar ]
- Li M, Vitányi P. An introduction to Kolmogorov complexity and its applications. New York: Springer; 1997. [ Google Scholar ]
- Li Y, Pizlo Z. Depth cues versus the simplicity principle in 3D shape perception. Topics in Cognitive Science. 2011; 3 (4):667–685. [ PubMed ] [ Google Scholar ]
- Little DR, Shiffrin RM. Simplicity bias in the estimation of causal functions; Proceedings of the 31st annual conference of the cognitive science society; 2009. pp. 1157–1162. [ Google Scholar ]
- Lombrozo T. Simplicity and probability in causal explanation. Cognitive Psychology. 2007; 55 (3):232–257. [ PubMed ] [ Google Scholar ]
- Looney NJ, Haygood RC. Effects of number of relevant dimensions in disjunctive concept learning. Journal of Experimental Psychology. 1968; 78 (1):169–171. [ PubMed ] [ Google Scholar ]
- Luria R, Sessa P, Gotler A, Jolicoeur P, Dell’Acqua R. Visual short-term memory capacity for simple and complex objects. J Cogn Neurosci. 2010; 22 (3):496–512. [ PubMed ] [ Google Scholar ]
- Ma WJ, Husain M, Bays PM. Changing concepts of working memory. Nat. Neurosci. 2014; 17 (3):347–356. [ PMC free article ] [ PubMed ] [ Google Scholar ]
- MacKay DJC. Information theory, inference, and learning algorithms. Cambridge: Cambridge University Press; 2003. [ Google Scholar ]
- Mathy F. Assessing conceptual complexity and compressibility using information gain and mutual information. Tutorials in Quantitative Methods for Psychology. 2010; 6 (1):16–30. [ Google Scholar ]
- Mathy F, Bradmetz J. A theory of the graceful complexification of concepts and their learnability. Current Psychology of Cognition. 2004; 22 :41–82. [ Google Scholar ]
- Mathy F, Fartoukh M, Gauvrit N, Guida A. Developmental abilities to form chunks in immediate memory and its non-relationship to span development. Frontiers in Psychology. 2016; 7 :201. [ PMC free article ] [ PubMed ] [ Google Scholar ]
- Mathy F, Feldman J. What’s magic about magic numbers? Chunking and data compression in short-term memory. Cognition. 2012; 122 (3):346–362. [ PubMed ] [ Google Scholar ]
- Medin DL, Wattenmaker WD, Michalski RS. Constraints and preferences in inductive learning: an experimental study of human and machine performance. Cognitive Science. 1987; 11 :299–339. [ Google Scholar ]
- Moravec L, Beck J. A modal completion: simplicity is not the explanation. Bulletin of the Psychonomic Society. 1986; 24 (4):269–272. [ Google Scholar ]
- Neisser U, Weene P. Hierarchies in concept attainment. Journal of Experimental Psychology. 1962; 64 (6):640–645. [ PubMed ] [ Google Scholar ]
- Nosofsky RM. Attention, similarity, and the identification-categorization relationship. Journal of Experimental Psychology: General. 1986; 115 (1):39–61. [ PubMed ] [ Google Scholar ]
- Nosofsky RM, Palmeri TJ, McKinley SC. Rule-plus-exception model of classification learning. Psychological Review. 1994; 101 (1):53–79. [ PubMed ] [ Google Scholar ]
- Pizlo Z, Sawada T, Li Y, Kropatsch WG, Steinman RM. New approach to the perception of 3D shape based on veridicality, complexity, symmetry and volume. Vision Res. 2010; 50 (1):1–11. [ PubMed ] [ Google Scholar ]
- Poggio T, Rifkin R, Mukherjee S, Niyogi P. General conditions for predictivity in learning theory. Nature. 2004; 428 :419–422. [ PubMed ] [ Google Scholar ]
- Pomerantz JR, Kubovy M. Theoretical approaches to perceptual organization. In: Boff KR, Kaufman L, Thomas JP, editors. Handbook of perception and human performance, vol. 2: Cognitive processes and performance. New York: John Wiley & Sons; 1986. pp. 36-1–36-46. [ Google Scholar ]
- Popper KR. The logic of scientific discovery. New York: Basic Books; 1934/1959. [ Google Scholar ]
- Pothos EM, Chater N. Categorization by simplicity: a minimum description length approach to unsupervised clustering. In: Hahn U, Ramscar M, editors. Similarity and categorization. Oxford: Oxford; 2001. pp. 51–72. [ Google Scholar ]
- Quine WVO. On simple theories of a complex world. In: Foster MH, Martin ML, editors. Probability, confirmation, and simplicity: Readings in the philosophy of inductive logic. New York: Odyssey Press; 1965. pp. 250–252. [ Google Scholar ]
- Restle F. Coding theory of the perception of motion configurations. Psychological Review. 1979; 86 (1):1–24. [ PubMed ] [ Google Scholar ]
- Richards WA, Bobick A. Playing twenty questions with nature. In: Pylyshyn Z, editor. Computational processes in human vision: An interdisciplinary perspective. Norwood, NJ: Ablex Publishing Corporation; 1988. pp. 3–26. [ Google Scholar ]
- Rieke F, Warland D, de Ruyter van Steveninck R, Bialek W. Spikes: exploring the neural code. Cambridge: M.I.T. Press; 1996. [ Google Scholar ]
- Rissanen J. Stochastic complexity in statistical inquiry. Singapore: World Scientific; 1989. [ Google Scholar ]
- Rosch E. Principles of categorization. In: Rosch E, Lloyd B, editors. Cognition and categorization. Lawrence Erlbaum; 1978. [ Google Scholar ]
- Schöning U, Pruim R. Gems of theoretical computer science. Berlin: Springer; 1998. [ Google Scholar ]
- Shannon C. A mathematical theory of communication. The Bell System Technical Journal. 1948; 27 :379–423. [ Google Scholar ]
- Shepard RN, Hovland CL, Jenkins HM. Learning and memorization of classifications. Psychological Monographs: General and Applied. 1961; 75 (13):1–42. [ Google Scholar ]
- Sims CR, Jacobs RA, Knill DC. An ideal observer analysis of visual working memory. Psychol Rev. 2012; 119 (4):807–830. [ PMC free article ] [ PubMed ] [ Google Scholar ]
- Sober E. Simplicity. London: Oxford University Press; 1975. [ Google Scholar ]
- Solomonoff R. A formal theory of inductive inference: part II. Information and Control. 1964; 7 :224–254. [ Google Scholar ]
- Tenenbaum JB, Griffiths TL. Generalization, similarity, and Bayesian inference. Behavioral and brain sciences. 2001; 24 (4):629–640. [ PubMed ] [ Google Scholar ]
- Turing AM. On computable numbers, with an application to the entscheidungs problem. Proceedings of the London Mathematical Society. 1937; 2 (42):230–265. [ Google Scholar ]
- Valiant L. A theory of the learnable. Communications of the ACM. 1984; 27 (11):1134–1142. [ Google Scholar ]
- van der Helm PA. Simplicity in perceptual organization. In: Wagemans J, editor. Handbook of perceptual organization. Oxford: Oxford University Press; 2014. [ Google Scholar ]
- van der Helm PA. Simplicity in vision. Cambridge: Cambridge University Press; 2015. [ Google Scholar ]
- van Lier RJ, Leeuwenberg ELJ, van der Helm PA. Multiple completions primed by occlusion patterns. Perception. 1995; 24 :727–740. [ PubMed ] [ Google Scholar ]
- van Lier RJ, van der Helm P, Leeuwenberg ELJ. Integrating global and local aspects of visual occlusion. Perception. 1994; 23 (8):883–903. [ PubMed ] [ Google Scholar ]
- Vigo R. Categorical invariance and structural complexity in human concept learning. Journal of Mathematical Psychology. 2009; 53 :203–221. [ Google Scholar ]
- Vitanyi PMB, Li M. Minimum description length induction, Bayesianism, and Kolmogorov complexity. IEEE Transactions on information theory. 2000; 46 (2):446–464. [ Google Scholar ]
- Wallace CS. Statistical and inductive inference by minimum message length. Springer; 2004. [ Google Scholar ]
- Wegener I. The complexity of Boolean functions. Chichester: John Wiley & Sons; 1987. [ Google Scholar ]
- Wilder J, Feldman J, Singh M. Contour complexity and contour detection. Journal of Vision. 2015a; 15 (6) [ PMC free article ] [ PubMed ] [ Google Scholar ]
- Wilder J, Feldman J, Singh M. The role of shape complexity in the detection of closed contours. Vision Research. 2015b [ PubMed ] [ Google Scholar ]
- Yuille AL, Bülthoff HH. Bayesian decision theory and psychophysics. In: Knill DC, Richards W, editors. Perception as Bayesian inference. Cambridge: Cambridge University Press; 1996. pp. 123–162. [ Google Scholar ]
- Ziv J, Lempel A. Auniversal algorithm for sequential data compression. IEEE Transactions on Information Theory. 1977; 23 (8):337–343. [ Google Scholar ]
- Bipolar Disorder
- Therapy Center
- When To See a Therapist
- Types of Therapy
- Best Online Therapy
- Best Couples Therapy
- Best Family Therapy
- Managing Stress
- Sleep and Dreaming
- Understanding Emotions
- Self-Improvement
- Healthy Relationships
- Student Resources
- Personality Types
- Guided Meditations
- Verywell Mind Insights
- 2024 Verywell Mind 25
- Mental Health in the Classroom
- Editorial Process
- Meet Our Review Board
- Crisis Support
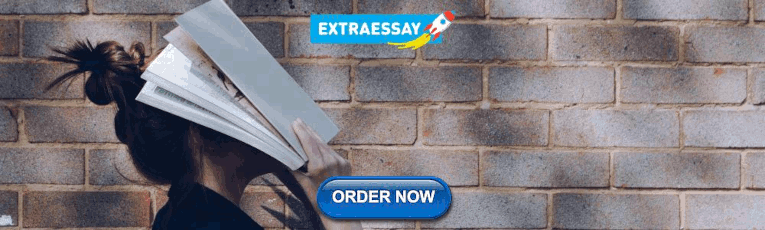
Perceptual Sets in Psychology
Kendra Cherry, MS, is a psychosocial rehabilitation specialist, psychology educator, and author of the "Everything Psychology Book."
:max_bytes(150000):strip_icc():format(webp)/IMG_9791-89504ab694d54b66bbd72cb84ffb860e.jpg)
Sean is a fact-checker and researcher with experience in sociology, field research, and data analytics.
:max_bytes(150000):strip_icc():format(webp)/Sean-Blackburn-1000-a8b2229366944421bc4b2f2ba26a1003.jpg)
Naufal MQ / Getty Images
How It Works
Top-down processing.
- Forces of Influence
- Real Life Impact
A perceptual set refers to a predisposition to perceive things in a certain way. In other words, we often tend to notice only certain aspects of an object or situation while ignoring other details.
What Is a Perceptual Set?
When it comes to our perceptions of the world around us, you might assume that what you see is what you get. However, in truth, research shows that the way you perceive the world through all of your senses is heavily influenced (and biased) by your own past experiences, expectations, motivations , beliefs, emotions , and even your culture.
For example, think about the last time you started a new class. Did you have any expectations at the outset that might have influenced your experience in the class? If you expect a class to be boring, are you more likely to be uninterested in class?
In psychology , this is what is known as a perceptual set.
A perceptual set is basically a tendency to view things only in a certain way.
What exactly is a perceptual set, why does it happen, and how does it influence how we perceive the world around us?
How do psychologists define perceptual sets?
"Perception can also be influenced by an individual's expectations, motives, and interests. The term perceptual set refers to the tendency to perceive objects or situations from a particular frame of reference," explains authors Susan Nolan and Sandra Hockenbury of the textbook Discovering Psychology .
Sometimes, perceptual sets can be helpful. They often lead us to make fairly accurate conclusions about what exists in the world around us. In cases where we find ourselves wrong, we often develop new perceptual sets that are more accurate.
Sometimes, our perceptual sets can lead us astray.
If you have a strong interest in military aircraft, for example, an odd cloud formation in the distance might be interpreted as a fleet of fighter jets; whereas, someone else may see it as a group of migrating ducks in flight.
In one experiment that illustrates this tendency, participants were presented with different non-words, such as sael . Those who were told that they would be reading boating-related words read the word as "sail," while those who were told to expect animal-related words read it as "seal."
A perceptual set is a good example of what is known as top-down processing . In top-down processing, perceptions begin with the most general and move toward the more specific. Such perceptions are heavily influenced by context, expectations, and prior knowledge.
If we expect something to appear in a certain way, we are more likely to perceive it according to our expectations.
Existing schemas , mental frameworks, and concepts often guide perceptual sets. For example, people have a strong schema for faces, making it easier to recognize familiar human faces in the world around us. It also means that when we look at an ambiguous image, we are more likely to see it as a face than some other type of object.
Researchers have also found that when multiple items appear in a single visual scene, perceptual sets will often lead people to miss additional items after locating the first one. For example, airport security officers might be likely to spot a water bottle in a bag but then miss that the bag also contains a firearm.
Forces of Influence
Below are examples of various forces of influence:
- Motivation can play an important role in perceptual sets and how we interpret the world around us. If we are rooting for our favorite sports team, we might be motivated to view members of the opposing team as overly aggressive, weak, or incompetent. In one classic experiment, researchers deprived participants of food for several hours. When they were later shown a set of ambiguous images, those who had been food-deprived were far more likely to interpret the images as food-related objects. Because they were hungry, they were more motivated to see the images in a certain way.
- Expectations also play an important role. If we expect people to behave in certain ways in certain situations, these expectations can influence how we perceive these people and their roles. One of the classic experiments on the impact of expectation on perceptual sets involved showing participants either a series of numbers or letters. Then, the participants were shown an ambiguous image that could either be interpreted as the number 13 or the letter B. Those who had viewed the numbers were more likely to see it as a 13, while those who had viewed the letters were more likely to see it as the letter B.
- Culture also influences how we perceive people, objects, and situations. Surprisingly, researchers have found that people from different cultures even tend to perceive perspective and depth cues differently.
- Emotions can have a dramatic impact on how we perceive the world around us. For example, if we are angry, we might be more likely to perceive hostility in others. One experiment demonstrated that when people came to associate a nonsense syllable with mild electrical shocks, they experienced physiological reactions to the syllable even when it was presented subliminally.
- Attitudes can also have a powerful influence on perception. In one experiment, Gordon Allport demonstrated that prejudice could have an influence on how quickly people categorize people of various races.
Real-Life Examples
Researchers have shown that perceptual sets can have a dramatic impact on day-to-day life.
In one experiment, young children were found to enjoy french fries more when they were served in a McDonald's bag rather than just a plain white bag. In another example, people who were told that an image was of the famed "Loch Ness monster" were more likely to see the mythical creature in the picture, while others who did not have the expectation of seeing a sea creature, saw only a curved tree trunk.
"Once we have formed a wrong idea about reality, we have more difficulty seeing the truth.
As previously mentioned, our perceptual set for faces is so strong that it actually causes us to see faces where there are none. Consider how people often describe seeing a face on the moon or in many of the inanimate objects that we encounter in our everyday lives.
As you can see, perception is not simply a matter of seeing what is in the world around us. A variety of factors can influence how we take in information and how we interpret it, as stimuli are filtered through our personal knowledge, expectations, emotions, and context.
Biggs A, Adamo S, Dowd E, Mitroff S. Examining perceptual and conceptual set biases in multiple-target visual search . Attention, Perception, & Psychophysics . 2015;77(3):844-855. doi:10.3758/s13414-014-0822-0
Nolan SA, Hockenbury SE. Discovering Psychology . Worth Publishers, 2021.
Hardy M, Heyes S. Beginning Psychology . Oxford University Press; 1999.
Gaspelin N, Luck SJ. " Top-down" does not mean "voluntary ". J Cogn . 2018;1(1):25. doi:10.5334/joc.28
Sanford R. The effects of abstinence from food upon imaginal processes: A preliminary experiment . J Psychol . 1936;2(1):129-136. doi:10.1080/00223980.1936.9917447
Bruner J, Minturn A. Perceptual identification and perceptual organization . J Gen Psychol . 1955;53(1):21-28. doi:10.1080/00221309.1955.9710133
de Bruïne G, Vredeveldt A, van Koppen PJ. Cross-cultural differences in object recognition: Comparing asylum seekers from Sub-Saharan Africa and a matched Western European control group . Appl Cogn Psychol . 2018;32(4):463‐473. doi:10.1002/acp.3419
Lazarus RS, McCleary RA. Autonomic discrimination without awareness: A study of subception . Psychological Review. 1951;58(2):113–122. doi:10.1037/h0054104
Barlow FK, Hornsey MJ, Thai M, Sengupta NK, Sibley CG. The wallpaper effect: The contact hypothesis fails for minority group members who live in areas with a high proportion of majority group members . PLoS One . 2013;8(12):e82228. doi:10.1371/journal.pone.0082228
Solomon MR, Russell-Bennett R, Previte J. Consumer Behaviour: Buying, Having, Being . Frenchs Forest, NSW: 2013.
Campbell S. The Loch Ness Monster: The Evidence . Prometheus Books; 1997.
Myers DG. Psychology . 7th ed. Worth Publishers; 2004.
By Kendra Cherry, MSEd Kendra Cherry, MS, is a psychosocial rehabilitation specialist, psychology educator, and author of the "Everything Psychology Book."
Module 6: Sensation and Perception
What you’ll learn to do: define perception and give examples of gestalt principles and multimodal perception.
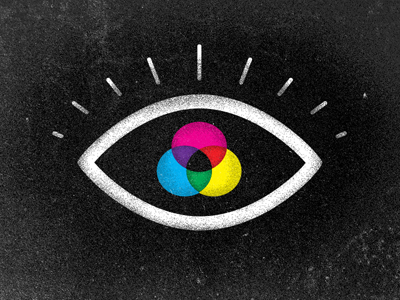
Seeing something is not the same thing as making sense of what you see. Why is it that our senses are so easily fooled? In this section, you will come to see how our perceptions are not infallible, and they can be influenced by bias, prejudice, and other factors. Psychologists are interested in how these false perceptions influence our thoughts and behavior.
Learning Objectives
- Give examples of gestalt principles, including the figure-ground relationship, proximity, similarity, continuity, and closure
- Define the basic terminology and basic principles of multimodal perception
- Give examples of multimodal and crossmodal behavioral effects
Gestalt Principles of Perception
In the early part of the 20th century, Max Wertheimer published a paper demonstrating that individuals perceived motion in rapidly flickering static images—an insight that came to him as he used a child’s toy tachistoscope. Wertheimer, and his assistants Wolfgang Köhler and Kurt Koffka, who later became his partners, believed that perception involved more than simply combining sensory stimuli. This belief led to a new movement within the field of psychology known as Gestalt psychology . The word gestalt literally means form or pattern, but its use reflects the idea that the whole is different from the sum of its parts. In other words, the brain creates a perception that is more than simply the sum of available sensory inputs, and it does so in predictable ways. Gestalt psychologists translated these predictable ways into principles by which we organize sensory information. As a result, Gestalt psychology has been extremely influential in the area of sensation and perception (Rock & Palmer, 1990).
One Gestalt principle is the figure-ground relationship . According to this principle, we tend to segment our visual world into figure and ground. Figure is the object or person that is the focus of the visual field, while the ground is the background. As Figure 1 shows, our perception can vary tremendously, depending on what is perceived as figure and what is perceived as ground. Presumably, our ability to interpret sensory information depends on what we label as figure and what we label as ground in any particular case, although this assumption has been called into question (Peterson & Gibson, 1994; Vecera & O’Reilly, 1998).
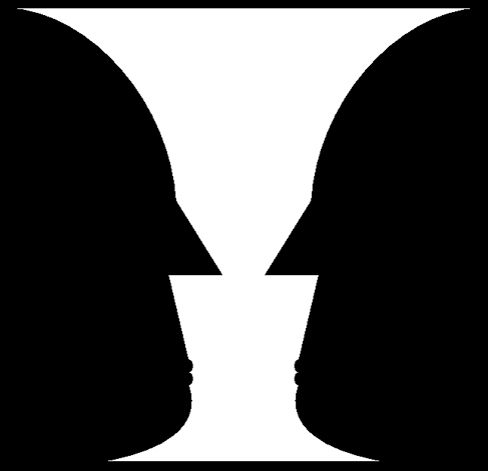
Figure 1. The concept of figure-ground relationship explains why this image can be perceived either as a vase or as a pair of faces.
Another Gestalt principle for organizing sensory stimuli into meaningful perception is proximity . This principle asserts that things that are close to one another tend to be grouped together, as Figure 2 illustrates.
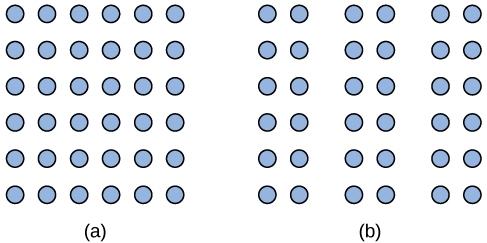
Figure 2. The Gestalt principle of proximity suggests that you see (a) one block of dots on the left side and (b) three columns on the right side.
How we read something provides another illustration of the proximity concept. For example, we read this sentence like this, notl iket hiso rt hat. We group the letters of a given word together because there are no spaces between the letters, and we perceive words because there are spaces between each word. Here are some more examples: Cany oum akes enseo ft hiss entence? What doth es e wor dsmea n?
We might also use the principle of similarity to group things in our visual fields. According to this principle, things that are alike tend to be grouped together (Figure 3). For example, when watching a football game, we tend to group individuals based on the colors of their uniforms. When watching an offensive drive, we can get a sense of the two teams simply by grouping along this dimension.
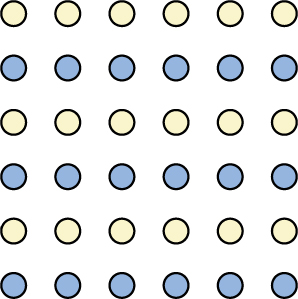
Figure 3. When looking at this array of dots, we likely perceive alternating rows of colors. We are grouping these dots according to the principle of similarity.
Two additional Gestalt principles are the law of continuity (or good continuation) and closure . The law of continuity suggests that we are more likely to perceive continuous, smooth flowing lines rather than jagged, broken lines (Figure 4). The principle of closure states that we organize our perceptions into complete objects rather than as a series of parts (Figure 5).
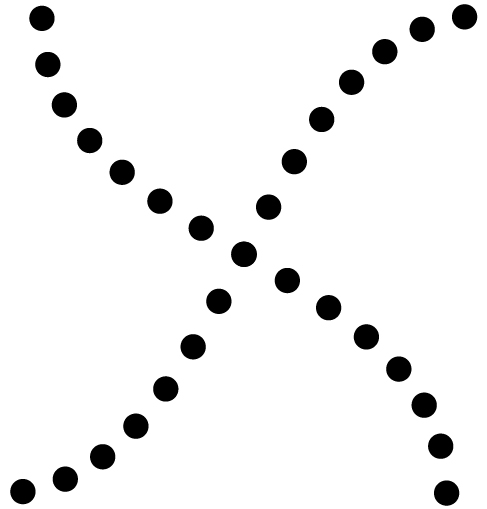
Figure 4. Good continuation would suggest that we are more likely to perceive this as two overlapping lines, rather than four lines meeting in the center.
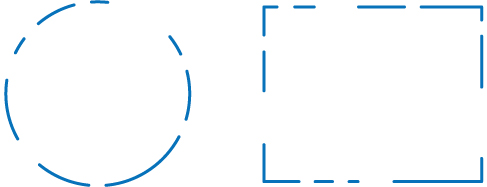
Figure 5. Closure suggests that we will perceive a complete circle and rectangle rather than a series of segments.
Link to Learning
Watch this podcast showing real world illustrations of Gestalt principles.
According to Gestalt theorists, pattern perception , or our ability to discriminate among different figures and shapes, occurs by following the principles described above. You probably feel fairly certain that your perception accurately matches the real world, but this is not always the case. Our perceptions are based on perceptual hypotheses : educated guesses that we make while interpreting sensory information. These hypotheses are informed by a number of factors, including our personalities, experiences, and expectations. We use these hypotheses to generate our perceptual set. For instance, research has demonstrated that those who are given verbal priming produce a biased interpretation of complex ambiguous figures (Goolkasian & Woodbury, 2010).
Dig Deeper: The Depths of Perception: Bias, Prejudice, and Cultural Factors
In this module, you have learned that perception is a complex process. Built from sensations, but influenced by our own experiences, biases, prejudices, and cultures, perceptions can be very different from person to person. Research suggests that implicit racial prejudice and stereotypes affect perception. For instance, several studies have demonstrated that non-Black participants identify weapons faster and are more likely to identify non-weapons as weapons when the image of the weapon is paired with the image of a Black person (Payne, 2001; Payne, Shimizu, & Jacoby, 2005). Furthermore, White individuals’ decisions to shoot an armed target in a video game is made more quickly when the target is Black (Correll, Park, Judd, & Wittenbrink, 2002; Correll, Urland, & Ito, 2006). This research is important, considering the number of very high-profile cases in the last few decades in which young Blacks were killed by people who claimed to believe that the unarmed individuals were armed and/or represented some threat to their personal safety.
Think It Over
Have you ever listened to a song on the radio and sung along only to find out later that you have been singing the wrong lyrics? Once you found the correct lyrics, did your perception of the song change?
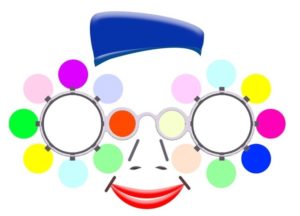
Figure 6. The way we receive the information from the world is called sensation while our interpretation of that information is called perception. [Image: Laurens van Lieshou]
Multi-Modal Perception
Although it has been traditional to study the various senses independently, most of the time, perception operates in the context of information supplied by multiple sensory modalities at the same time. For example, imagine if you witnessed a car collision. You could describe the stimulus generated by this event by considering each of the senses independently; that is, as a set of unimodal stimuli. Your eyes would be stimulated with patterns of light energy bouncing off the cars involved. Your ears would be stimulated with patterns of acoustic energy emanating from the collision. Your nose might even be stimulated by the smell of burning rubber or gasoline. However, all of this information would be relevant to the same thing: your perception of the car collision. Indeed, unless someone was to explicitly ask you to describe your perception in unimodal terms, you would most likely experience the event as a unified bundle of sensations from multiple senses. In other words, your perception would be multimodal . The question is whether the various sources of information involved in this multimodal stimulus are processed separately by the perceptual system or not.
For the last few decades, perceptual research has pointed to the importance of multimodal perception : the effects on the perception of events and objects in the world that are observed when there is information from more than one sensory modality. Most of this research indicates that, at some point in perceptual processing, information from the various sensory modalities is integrated . In other words, the information is combined and treated as a unitary representation of the world.
Behavioral Effects of Multimodal Perception
Although neuroscientists tend to study very simple interactions between neurons, the fact that they’ve found so many crossmodal areas of the cortex seems to hint that the way we experience the world is fundamentally multimodal. Our intuitions about perception are consistent with this; it does not seem as though our perception of events is constrained to the perception of each sensory modality independently. Rather, we perceive a unified world, regardless of the sensory modality through which we perceive it.
It will probably require many more years of research before neuroscientists uncover all the details of the neural machinery involved in this unified experience. In the meantime, experimental psychologists have contributed to our understanding of multimodal perception through investigations of the behavioral effects associated with it. These effects fall into two broad classes. The first class— multimodal phenomena —concerns the binding of inputs from multiple sensory modalities and the effects of this binding on perception. The second class— crossmodal phenomena —concerns the influence of one sensory modality on the perception of another (Spence, Senkowski, & Roder, 2009).
Multimodal Phenomena
Audiovisual speech.
Multimodal phenomena concern stimuli that generate simultaneous (or nearly simultaneous) information in more than one sensory modality. As discussed above, speech is a classic example of this kind of stimulus. When an individual speaks, she generates sound waves that carry meaningful information. If the perceiver is also looking at the speaker, then that perceiver also has access to visual patterns that carry meaningful information. Of course, as anyone who has ever tried to lipread knows, there are limits on how informative visual speech information is. Even so, the visual speech pattern alone is sufficient for very robust speech perception. Most people assume that deaf individuals are much better at lipreading than individuals with normal hearing. It may come as a surprise to learn, however, that some individuals with normal hearing are also remarkably good at lipreading (sometimes called “speechreading”). In fact, there is a wide range of speechreading ability in both normal hearing and deaf populations (Andersson, Lyxell, Rönnberg, & Spens, 2001). However, the reasons for this wide range of performance are not well understood (Auer & Bernstein, 2007; Bernstein, 2006; Bernstein, Auer, & Tucker, 2001; Mohammed et al., 2005).
How does visual information about speech interact with auditory information about speech? One of the earliest investigations of this question examined the accuracy of recognizing spoken words presented in a noisy context, much like in the example above about talking at a crowded party. To study this phenomenon experimentally, some irrelevant noise (“white noise”—which sounds like a radio tuned between stations) was presented to participants. Embedded in the white noise were spoken words, and the participants’ task was to identify the words. There were two conditions: one in which only the auditory component of the words was presented (the “auditory-alone” condition), and one in both the auditory and visual components were presented (the “audiovisual” condition). The noise levels were also varied, so that on some trials, the noise was very loud relative to the loudness of the words, and on other trials, the noise was very soft relative to the words. Sumby and Pollack (1954) found that the accuracy of identifying the spoken words was much higher for the audiovisual condition than it was in the auditory-alone condition. In addition, the pattern of results was consistent with the Principle of Inverse Effectiveness: The advantage gained by audiovisual presentation was highest when the auditory-alone condition performance was lowest (i.e., when the noise was loudest). At these noise levels, the audiovisual advantage was considerable: It was estimated that allowing the participant to see the speaker was equivalent to turning the volume of the noise down by over half. Clearly, the audiovisual advantage can have dramatic effects on behavior.
Another phenomenon using audiovisual speech is a very famous illusion called the “McGurk effect” (named after one of its discoverers). In the classic formulation of the illusion, a movie is recorded of a speaker saying the syllables “gaga.” Another movie is made of the same speaker saying the syllables “baba.” Then, the auditory portion of the “baba” movie is dubbed onto the visual portion of the “gaga” movie. This combined stimulus is presented to participants, who are asked to report what the speaker in the movie said. McGurk and MacDonald (1976) reported that 98 percent of their participants reported hearing the syllable “dada”—which was in neither the visual nor the auditory components of the stimulus. These results indicate that when visual and auditory information about speech is integrated, it can have profound effects on perception.
Tactile/Visual Interactions in Body Ownership
Not all multisensory integration phenomena concern speech, however. One particularly compelling multisensory illusion involves the integration of tactile and visual information in the perception of body ownership. In the “rubber hand illusion” (Botvinick & Cohen, 1998), an observer is situated so that one of his hands is not visible. A fake rubber hand is placed near the obscured hand, but in a visible location. The experimenter then uses a light paintbrush to simultaneously stroke the obscured hand and the rubber hand in the same locations. For example, if the middle finger of the obscured hand is being brushed, then the middle finger of the rubber hand will also be brushed. This sets up a correspondence between the tactile sensations (coming from the obscured hand) and the visual sensations (of the rubber hand). After a short time (around 10 minutes), participants report feeling as though the rubber hand “belongs” to them; that is, that the rubber hand is a part of their body. This feeling can be so strong that surprising the participant by hitting the rubber hand with a hammer often leads to a reflexive withdrawing of the obscured hand—even though it is in no danger at all. It appears, then, that our awareness of our own bodies may be the result of multisensory integration.
Crossmodal Phenomena
Crossmodal phenomena are distinguished from multimodal phenomena in that they concern the influence one sensory modality has on the perception of another.
Visual Influence on Auditory Localization
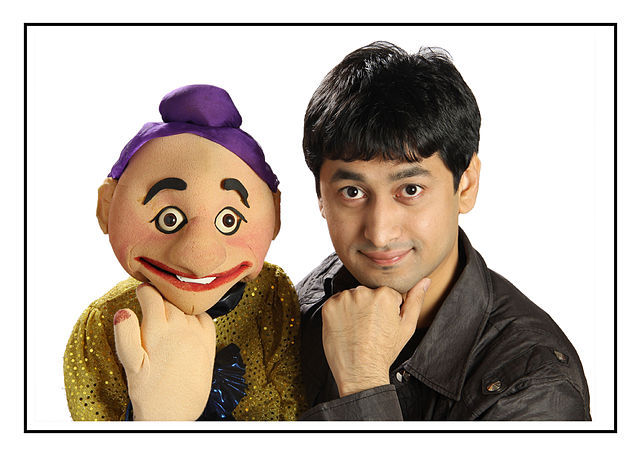
Figure 7. Ventriloquists are able to trick us into believing that what we see and what we hear are the same where, in truth, they are not. [Image: Indiapuppet]
A famous (and commonly experienced) crossmodal illusion is referred to as “the ventriloquism effect.” When a ventriloquist appears to make a puppet speak, she fools the listener into thinking that the location of the origin of the speech sounds is at the puppet’s mouth. In other words, instead of localizing the auditory signal (coming from the mouth of a ventriloquist) to the correct place, our perceptual system localizes it incorrectly (to the mouth of the puppet).
Why might this happen? Consider the information available to the observer about the location of the two components of the stimulus: the sounds from the ventriloquist’s mouth and the visual movement of the puppet’s mouth. Whereas it is very obvious where the visual stimulus is coming from (because you can see it), it is much more difficult to pinpoint the location of the sounds. In other words, the very precise visual location of mouth movement apparently overrides the less well-specified location of the auditory information. More generally, it has been found that the location of a wide variety of auditory stimuli can be affected by the simultaneous presentation of a visual stimulus (Vroomen & De Gelder, 2004). In addition, the ventriloquism effect has been demonstrated for objects in motion: The motion of a visual object can influence the perceived direction of motion of a moving sound source (Soto-Faraco, Kingstone, & Spence, 2003).
Auditory Influence on Visual Perception
A related illusion demonstrates the opposite effect: where sounds have an effect on visual perception. In the double-flash illusion, a participant is asked to stare at a central point on a computer monitor. On the extreme edge of the participant’s vision, a white circle is briefly flashed one time. There is also a simultaneous auditory event: either one beep or two beeps in rapid succession. Remarkably, participants report seeing two visual flashes when the flash is accompanied by two beeps; the same stimulus is seen as a single flash in the context of a single beep or no beep (Shams, Kamitani, & Shimojo, 2000). In other words, the number of heard beeps influences the number of seen flashes!
Participate in the double-flash experiment here .
Take a look at the bouncing balls illusion here .
Another illusion involves the perception of collisions between two circles (called “balls”) moving toward each other and continuing through each other. Such stimuli can be perceived as either two balls moving through each other or as a collision between the two balls that then bounce off each other in opposite directions. Sekuler, Sekuler, and Lau (1997) showed that the presentation of an auditory stimulus at the time of contact between the two balls strongly influenced the perception of a collision event. In this case, the perceived sound influences the interpretation of the ambiguous visual stimulus.
Crossmodal Speech
Several crossmodal phenomena have also been discovered for speech stimuli. These crossmodal speech effects usually show altered perceptual processing of unimodal stimuli (e.g., acoustic patterns) by virtue of prior experience with the alternate unimodal stimulus (e.g., optical patterns). For example, Rosenblum, Miller, and Sanchez (2007) conducted an experiment examining the ability to become familiar with a person’s voice. Their first interesting finding was unimodal: Much like what happens when someone repeatedly hears a person speak, perceivers can become familiar with the “visual voice” of a speaker. That is, they can become familiar with the person’s speaking style simply by seeing that person speak. Even more astounding was their crossmodal finding: Familiarity with this visual information also led to increased recognition of the speaker’s auditory speech, to which participants had never had exposure.
Similarly, it has been shown that when perceivers see a speaking face, they can identify the (auditory-alone) voice of that speaker, and vice versa (Kamachi, Hill, Lander, & Vatikiotis-Bateson, 2003; Lachs & Pisoni, 2004a, 2004b, 2004c; Rosenblum, Smith, Nichols, Lee, & Hale, 2006). In other words, the visual form of a speaker engaged in the act of speaking appears to contain information about what that speaker should sound like. Perhaps more surprisingly, the auditory form of speech seems to contain information about what the speaker should look like.
In the late 17th century, a scientist named William Molyneux asked the famous philosopher John Locke a question relevant to modern studies of multisensory processing. The question was this: Imagine a person who has been blind since birth, and who is able, by virtue of the sense of touch, to identify three dimensional shapes such as spheres or pyramids. Now imagine that this person suddenly receives the ability to see. Would the person, without using the sense of touch, be able to identify those same shapes visually? Can modern research in multimodal perception help answer this question? Why or why not? How do the studies about crossmodal phenomena inform us about the answer to this question?
closure: organizing our perceptions into complete objects rather than as a series of parts crossmodal phenomena : effects that concern the influence of the perception of one sensory modality on the perception of another double flash illusion : the false perception of two visual flashes when a single flash is accompanied by two auditory beeps figure-ground relationship: segmenting our visual world into figure and ground Gestalt psychology: field of psychology based on the idea that the whole is different from the sum of its parts< good continuation: (also, continuity) we are more likely to perceive continuous, smooth flowing lines rather than jagged, broken lines integrated : the process by which the perceptual system combines information arising from more than one modality McGurk effect : an effect in which conflicting visual and auditory components of a speech stimulus result in an illusory percept multimodal : of or pertaining to multiple sensory modalities multimodal perception : the effects that concurrent stimulation in more than one sensory modality has on the perception of events and objects in the world multimodal phenomena : effects that concern the binding of inputs from multiple sensory modalities pattern perception: ability to discriminate among different figures and shapes perceptual hypothesis: educated guess used to interpret sensory information principle of closure: organize perceptions into complete objects rather than as a series of parts proximity: things that are close to one another tend to be grouped together rubber hand illusion : the false perception of a fake hand as belonging to a perceiver, due to multimodal sensory information sensory modalities : a type of sense; for example, vision or audition similarity: things that are alike tend to be grouped together unimodal : of or pertaining to a single sensory modality
- Modification, adaptation, and original content. Provided by : Lumen Learning. License : CC BY: Attribution
- Modification of content on multi-modal perception. Provided by : Lumen Learning. License : CC BY-NC-SA: Attribution-NonCommercial-ShareAlike
- Perception Poster Show Concept. Authored by : Jon Ashcroft. Located at : https://www.flickr.com/photos/theilluminated/5704563713 . License : CC BY: Attribution
- Gestalt Principles of Psychology. Authored by : OpenStax College. Located at : http://cnx.org/contents/[email protected]:cOcxAR_r@5/Gestalt-Principles-of-Percepti#Figure_05_06_Continuity . License : CC BY: Attribution . License Terms : Download for free at http://cnx.org/content/col11629/latest/.
- Multi-Modal Perception. Authored by : Lorin Lachs. Provided by : California State University, Fresno. Located at : http://nobaproject.com/modules/multi-modal-perception . Project : The Noba Project. License : CC BY-NC-SA: Attribution-NonCommercial-ShareAlike
- Perceiving is Believing - Crash Course Psychology #7. Provided by : CrashCourse. Located at : https://www.youtube.com/watch?v=n46umYA_4dM . License : Other . License Terms : Standard YouTube License
- The McGurk Effect. Provided by : BBC. Located at : https://youtu.be/G-lN8vWm3m0?t=32s . License : Other . License Terms : Standard YouTube License
- The Rubber Hand Illusion. Provided by : BBC. Located at : https://youtu.be/sxwn1w7MJvk . License : Other . License Terms : Standard YouTube License
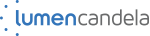
Privacy Policy

- Table of Contents
- Random Entry
- Chronological
- Editorial Information
- About the SEP
- Editorial Board
- How to Cite the SEP
- Special Characters
- Advanced Tools
- Support the SEP
- PDFs for SEP Friends
- Make a Donation
- SEPIA for Libraries
- Entry Contents
Bibliography
Academic tools.
- Friends PDF Preview
- Author and Citation Info
- Back to Top
Perceptual Learning
“Perceptual Learning” refers, roughly, to long-lasting changes in perception that result from practice or experience (see E.J. Gibson 1963). William James, for instance, writes about how a person can become able to differentiate by taste between the upper and lower half of a bottle for a particular kind of wine (1890: 509). Assuming that the change in the person’s perception lasts, is genuinely perceptual (rather than, say, a learned inference), and is based on prior experience, James’ case is a case of perceptual learning.
This entry has three parts. The first part lays out the definition of perceptual learning as long-term changes in perception that result from practice or experience, and then distinguishes perceptual learning from several contrast classes. The second part specifies different varieties of perceptual learning. The third part details cases of perceptual learning in the philosophical literature and says why they are philosophically significant.
1.1 Perceptual Learning as Long-Term Perceptual Changes
1.2 perceptual learning as perceptual changes, 1.3 perceptual learning as resulting from practice or experience, 1.4 potential further criteria for defining perceptual learning, 1.5.1 perceptual development, 1.5.2 perception-based skills, 1.5.3 cognitive penetration, 1.5.4 machine learning, 2.1 differentiation, 2.2 unitization, 2.3 attentional weighting, 2.4 stimulus imprinting, 3.1 the contents of perception, 3.2 cognitive penetration, 3.3 the theory-ladenness of observation, 3.4 modularity, other internet resources, related entries, 1. defining perceptual learning.
In 1963, the psychologist Eleanor Gibson wrote a landmark survey article on perceptual learning in which she purported to define the term. According to Gibson, perceptual learning is “[a]ny relatively permanent and consistent change in the perception of a stimulus array, following practice or experience with this array…” (1963: 29). [ 1 ] Gibson’s definition has three basic parts. First, perceptual learning is long-lasting. Second, it is perceptual. Third, it is the result of practice or experience. This entry expands on each of these features of the definition.
Perceptual learning involves long-term changes in perception. This criterion rules out short term perceptual changes due to sensory adaptation (for more on sensory adaptation see Webster 2012). In the waterfall illusion, for instance, a person who looks at a waterfall for a minute, and then looks away at some rocks, sees the rocks as moving even though they are not. This is a short-term change in perception, lasting perhaps for fifteen to thirty seconds. Since it is not a long-term change in perception, however, it does not count as perceptual learning. In another short term adaptive change, a person who goes indoors after walking through a blizzard may have trouble as her eyes adjust to the new lighting. There is a change in her perception as a result of her experience in the blizzard. But it is not a long-term change, and so it does not count as perceptual learning.
While there are clear cases of long-term experience-induced perceptual changes and clear cases of short-term experience-induced perceptual changes, there may be intermediary cases where it is difficult to tell whether they count as long-term or not. In such cases, in order to determine whether the case is a genuine case of perceptual learning, it may be necessary to look at the mechanisms involved (see section 2 below on the mechanisms of perceptual learning). If the mechanisms involved are characteristic of other cases of perceptual learning, then that is a reason to count the case as an instance of perceptual learning. If the mechanisms involved are uncharacteristic of perceptual learning, then that is a reason not to count the case as an instance of perceptual learning.
Perceptual learning involves changes in perception . [ 2 ] This rules out mere changes in aesthetic taste, among other things. For instance, imagine a contrarian who likes things only insofar as other people do not like those things. Suppose he finds out that everyone else has come to like his favorite microbrew. This might cause him to change how he judges that beer aesthetically. However, the beer may well taste the same to him. So, it is not a case of perceptual learning, but a mere change in the person’s aesthetic judgment. The fact that perceptual learning involves changes in perception also rules out mere changes in belief. Suppose someone acquires the belief that the symphony movement they are hearing is a scherzo. If nothing changes in that person’s perception, this is not a case of perceptual learning. It is a change in the person’s belief, not a change in the person’s perception.
It is important here to distinguish perceptual learning from learning that is simply based on perception (see Dretske 2015: fn. 6). [ 3 ] Perceptual learning involves changes in perception, while learning that is based on perception need not. Looking at my table, I might learn that the cup is on the table. However, this does not involve any long-term changes in perception. It is learning that is based on perception, but it is not perceptual learning. Furthermore, I might learn to put the cup on the table into the dishwasher every time it is empty. Again, this is learning that is based on perception (I need to perceive the cup in order to move it). However, it is not perceptual learning.
One of the main reasons for holding that improvements in perceptual discrimination can be genuinely perceptual is due to somewhat recent evidence from neuroscience. As Manfred Fahle puts it, during the 1970s and 1980s, it tended to be the case that improvements in perceptual discrimination were thought to be cognitive rather than perceptual (2002: xii). However, during the 1990s, pressure was put on the cognitive interpretation due to new neuroscientific evidence in perceptual learning studies. In particular, studies found that learning-induced plasticity occurs in the adult primary sensory cortices much more than researchers had previously thought (Fahle 2002: xii). Neurological evidence of plasticity in adult primary sensory cortices due to learning provides some evidence that changes in perceptual discrimination can be due to perceptual learning. (See also Garraghty & Kass 1992: 522; Gilbert 1996: 269; Goldstone 2003: 238; Gilbert & Li 2012: 250; and Sagi 2011: 1552–53).
Perceptual learning involves perceptual changes of a particular kind, namely, those that result from practice or experience. For this reason, laser eye surgery or cataracts removal do not count as instances of perceptual learning. They are not really cases of learning because they do not result from practice or experience. So, while such cases involve long-term changes in perception, they do not count as cases of perceptual learning.
To be authentic cases of learning, perceptual changes have to be the result of a learning process. As a contrast case, suppose someone undergoes a long-term change in their perception due to a brain lesion. Such a change in perception does not result from a learning process, since the change in perception comes from the lesion, rather from practice or experience. Because of this, the case does not count as an instance of perceptual learning, even though it involves a long-term change in perception.
The conversation above roughly follows Eleanor Gibson’s definition of perceptual learning. However, there are also other accounts in the psychology literature. Robert Goldstone’s account of perceptual learning, for instance, agrees with Gibson’s account in many respects, but it additionally offers a story of why perceptual changes occur. On his account,
Perceptual learning involves relatively long-lasting changes to an organism’s perceptual system that improve its ability to respond to its environment and are caused by this environment . (1998: 587, italics added for emphasis)
This definition offers an answer to the question as to why perceptual learning occurs at all. On Goldstone’s account, perceptual learning occurs to improve an organism’s ability to respond to the environment.
Goldstone’s account admits of two different interpretations. On one interpretation, the account places a condition on perceptual learning: that to count as an instance of perceptual learning, a long-term perceptual change has to improve an organism’s ability to respond to the environment. Such an account gains plausibility if one thinks of “learning” as a success-term. The idea then is that each genuine instance of perceptual learning leads to success for the organism, namely, it improves the organism’s ability to respond to the environment. On a second interpretation of Goldstone’s account, however, it is not that each instance of perceptual learning has to improve an organism’s ability to respond to the environment. Rather, it is that perceptual learning is a general capacity for improving an organism’s ability to respond to the environment, even if perceptual learning fails to do so in some instances. Why might organisms have such a capacity? One possibility is that the capacity is a trait that improves fitness and is the product of natural selection. However, the biological origin of perceptual learning is an area of research that still needs to be carefully explored.
1.5 Contrast Classes
How much of the perceptual development we undergo as infants and young children is the result of learning? There are many difficulties distinguishing development from learning, conceptually (for some discussion, see Carey 2009, especially pp. 11–14). The issue of how to distinguish development from learning bears on the traditional philosophical debate between nativists and empiricists (see Markie 2015, for a summary of that debate). In the perceptual learning literature, for instance, Kellman and Garrigan reject the view that all perceptual development is the result of learning, a view that they consider to be empiricist (2009: 57). Specifically, they think that data on infant perception collected in and around the 1980s provide evidence that at least some perceptual development is innate:
What this research has shown is that the traditional empiricist picture of perceptual development is incorrect. Although perception becomes more precise with age and experience, basic capacities of all sorts – such as the abilities to perceive objects, faces, motion, three-dimensional space, the directions of sounds, coordinate the senses in perceiving events, and other abilities – arise primarily from innate or early-maturing mechanisms (Bushnell, Sai, & Mullin 1989; Gibson et al., 1979; Held 1985; Kellman & Spelke 1983; Meltzoff & Moore 1977; and Slater, Mattock, & Brown 1990). (Kellman & Garrigan 2009: 57)
In short, according to Kellman and Garrigan, evidence on infant perception—including evidence about object perception, the perception of faces, and the perception of three-dimensional space—tells against the view that all perceptual development is learned.
If not all perceptual development is learned, while all perceptual learning is learned, then there is a distinction between perceptual development and perceptual learning. One way to draw the distinction more fully is the following. Perceptual development involves perceptual learning. However, it does not just involve perceptual learning. It also involves what is called maturation . For instance, the abilities that Kellman and Garrigan describe above (object perception, the perception of faces, the perception of three-dimensional space, etc.) fall under the category of maturation.
There are many ways to try to draw the further distinction between perceptual maturation and perceptual learning. Some such ways are found in the debate between nativism and empiricism (see Samet 2008 and Markie 2015) and specifically in the difference between innate and acquired characteristics (see Griffiths 2009 and Cowie 2016). One potential criterion here is that cases of perceptual maturation involve perceptual abilities that are typical of the species, while cases of perceptual learning involve perceptual abilities that are not typical of the species. This criterion seems to get it right for some instances of perceptual learning, say, for those involved in birdwatching. After all, the perceptual abilities acquired in birdwatching are unique to birdwatchers, not typical of the entire human species. However, the criterion seems to get it wrong for other, more universal, instances of perceptual learning. For instance, since human faces are both ubiquitous and important to humans, the perceptual learning involved in face perception is in fact typical of the species.
In the literature on perceptual learning, by contrast, the distinction between perceptual learning and perceptual maturation is often drawn in terms of the role of the environment. On Goldstone’s account of perceptual learning, to count as perceptual learning, perceptual changes must be caused by the environment. It is important to understand why exactly Goldstone thinks that caused by the environment is a crucial feature of the definition. He thinks it is crucial since this criterion distinguishes between perceptual changes that are simply the result of maturation, and perceptual changes that are the result of learning. As Goldstone puts it, “If the changes are not due to environmental inputs, then maturation rather than learning is implicated” (1998: 586). Manfred Fahle puts it similarly by saying that the term maturation “ascribe[s] the main thrust of the changes in a behavior to genetics, not the environment” (2002: xi). For Fahle, this is what distinguishes it from perceptual learning.
A further point of contrast with perceptual learning is perception-based skills, such as dart-throwing or racecar driving. To understand the relationship between perceptual learning and perception-based skills, start by considering the following case. Williams and Davids (1998) reported that when expert soccer players defend opponents, they focus longer on their opponent’s hips than non-experts do. This tuned attention is a long-term change in perception that results from practice or experience. That is, it is an instance of perceptual learning (see section 2.3 below). Such changes certainly serve to enable perception-based skills. For instance, attending to the hips is part of what enables the soccer players to defend well. Since the hips provide a cue for what the offensive player will do next, when the defender attends there, it helps them to do all sorts of things: to keep the offensive player from dribbling by them; to keep the offensive player from completing a pass; and to keep them from shooting and scoring. Without the attentional tuning, the expert soccer players would not be able to perform as high above baseline as they do.
Perceptual learning can enable perception-based skills, yet it is important to distinguish these skills from perceptual learning. In fact, arguably, as Stanley and Krakauer (2013) claim, perceptual learning does not in itself give you a skill, properly speaking. One reason why, drawing on Stanley and Krakauer, is that skills quite plausibly require instruction (at least initially), or observation of someone else (2013: 3). Perceptual learning, by contrast, can at times be unsupervised learning (see Goldstone 2003: 241 and Goldstone & Byrge 2015: section 3). Long-term, learning-induced changes in perception sometimes happen through mere exposure to stimuli, and without any instruction whatsoever. Furthermore, arguably, as Stanley and Krakauer put it, “our skilled actions are always under our rational control…” (2013: 3; see also Stanley & Williamson forthcoming: 6). Yet, there is an important sense in which one cannot control a tuned attentional pattern like that of the expert soccer players mentioned above. Goldstone, for instance, cites a study on attentional tuning by Shiffrin and Schneider (1977). In that study, letters were used first as targets in the experiment, but later letters were used as distractors to be ignored (Goldstone 1998: 589). Due to their prior training with the letters, the subjects’ attention became automatic with respect to the letters in the scene, even though they were trying to deliberately ignore them. More generally, after training, it is difficult to rationally control a tuned attentional pattern because the attention is automatic toward particular properties.
Perceptual learning involves changes in perception that are long-term . This long-term criterion rules out some cases of cognitive penetration, that is, cases where one’s beliefs, thoughts, or desires influence one’s perception (see Macpherson 2012: 24). For instance, to borrow a case from Susanna Siegel (2012), if Jill sees Jack as angry because she just now believes Jack is angry, this need not be a case of perceptual learning, since it need not be a long-term change. After all, if Jill changes her belief that Jack is angry shortly after, she will no longer see his neutral face as angry. It would be a short-term change in her perception, not a long-term one. And so it would not be a case of perceptual learning.
Simply because some cases of cognitive penetration are not cases of perceptual learning, however, it does not follow that no cases of cognitive penetration are cases of perceptual learning. Jerry Fodor distinguishes between synchronic penetration and diachronic penetration, where only the latter involves “experience and training” (1984: 39). The case of Jack and Jill is a case of synchronic penetration, one where the penetration does not involve experience and training. However, at least some cases of perceptual learning might more plausibly fit into the category of diachronic penetration. (For more on the relationship between perceptual learning and cognitive penetration, see section 3.2 )
Machine perception seeks “to enable man-made machines to perceive their environments by sensory means as human and animals do” (Nevatia 1982: 1). Standard cases of machine perception involve computers that are able to recognize speech, faces, or types of objects. Some types of machine perception are simply programmed into the device. For instance, some speech recognition devices (especially older ones) are simply programmed to recognize speech, and do not learn beyond what they have been programmed to do. Other types of machine perception involve “machine learning” where the device learns based on the inputs that it receives, often involving some kind of feedback.
Like cases of perceptual learning, machine learning can be either supervised or unsupervised, although these distinctions mean something very specific in the machine case. In supervised learning, builders test the machine’s initial performance on, say, the recognition of whether a given image contains a face. They then measure the performance error and adjust the parameters of the machine to improve performance (LeCun, Bengio, & Hinton 2015: 436). Importantly, in cases of supervised learning, engineers program into the machine which features it should look for when, say, identifying a face. In cases of unsupervised learning, by contrast, the machine does not have information about its target features. The machine merely aims to find similarities in the given images, and if it is successful, the machine comes to group all the faces together according to their similarities (Dy & Brodley 2004: 845).
In machine learning, one major difficulty is that machines can develop racist and sexist patterns (for several examples, see Crawford 2016). The problem is often that engineers input a biased set of images (such as a set of images that include too many white people) into the machine, from which the machine builds its model (Crawford 2016). This suggests a potential corresponding source of bias in human perceptual learning, based on the inputs that humans receive through media.
2. Varieties of Perceptual Learning
The psychology literature provides ample evidence of perceptual learning. Goldstone (1998) helpfully distinguishes between four different types of perceptual learning in the literature: differentiation, unitization, attentional weighting, and stimulus imprinting. This section surveys these four types of perceptual learning (for further review, see Goldstone 2003; Goldstone, Braithwaite, & Byrge 2012; and Goldstone & Byrge 2015).
When most people reflect on perceptual learning, the cases that tend to come to mind are cases of differentiation . In differentiation, a person comes to perceive the difference between two properties, where they could not perceive this difference before. It is helpful to think of William James’ case of a person learning to distinguish between the upper and lower half of a particular kind of wine. Prior to learning, one cannot perceive the difference between the upper and lower half. However, through practice one becomes able to distinguish between the upper and lower half. This is a paradigm case of differentiation.
Psychologists have studied differentiation in lab environments. In one such study, experimenters took six native Japanese speakers who had lived in the United States from between six months and three years (Logan, Lively, & Pisoni 1991). The subjects were not native English speakers. The experimenters found that they were able to train these subjects to better distinguish between the phonemes /r/ and /l/. This is a case of improved differentiation, where the subjects became better at perceiving the difference between two properties, which they had more trouble telling apart before.
Unitization is the counterpart to differentiation. In unitization, a person comes to perceive as a single property, what they previously perceived as two or more distinct properties. One example of unitization is the perception of written words. When we perceive a written word in English, we do not simply perceive two or more distinct letters. Rather, we perceive those letters as a single word. Put another way, we perceive written words as a single unit (see Smith & Haviland 1972). This is not the case with non-words. When we perceive short strings of letters that are not words, we do not perceive them as a single unit. Goldstone and Byrge provide a list of items for which there is empirical evidence of such unitization:
birds, words, grids of lines, random wire structures, fingerprints, artificial blobs, and three-dimensional creatures made from simple geometric components. (2015: 823)
While unitization and differentiation are converses, the one unifying and the other distinguishing, Goldstone and Byrge also conceive of them as “flip sides of the same coin” (2015: 823). This is because, as they put it, both unitization and differentiation “involve creating perceptual units…” (2015: 823). Regardless of whether the unit arises from the fusion or the differentiation of two other units, both instances of perceptual learning involve the creation of new perceptual units.
In attentional weighting, through practice or experience people come to systematically attend toward certain objects and properties and away from other objects and properties. Paradigm cases of attentional weighting have been shown in sports studies, where it has been found, for instance, that expert fencers attend more to their opponents’ upper trunk area, while non-experts attend more to their opponents’ upper leg area (Hagemann et al., 2010). Practice or experience modulates attention as fencers learn, shifting it towards certain areas and away from other areas.
In the case of the expert fencer, a shift in the weight of attention to the opponents’ upper trunk area facilitates the expert’s fencing skills. However, shifts in attentional weighting can also fail to facilitate skills or even stifle them. For example, a new golfer with inadequate coaching might develop the bad habit of attending to their putter while putting, rather than learning to keep their “eye on the ball.” This unhelpful shift in attentional weighting may well stifle the new golfer’s ability to become a skillful putter.
One way to understand weighted attention is as attention that has become automatic with respect to particular properties. In other words, when the expert fencer attends to the upper trunk area, this attention is no longer governed by her intention (see Wu 2014: 33, for more on this account of automaticity). Rather, as the result of practice, the expert fencer’s attention is now automatic with respect to the trunk area . This italicized part is important. On Wayne Wu’s account of attention, for instance, one might ask whether attention is automatic with respect to different features of the process of attention: “where attention is directed and in what sequence, how long it is sustained, to what specific features in the scene, and so on” (p. 34). In the case of the expert fencer, plausibly her attention is automatic with respect to the trunk area, even if it is not automatic in other respects. This automaticity is the product of her learning process.
Recall that in unitization, what previously looked like two or more objects, properties, or events later looks like a single object, property, or event. Cases of “stimulus imprinting” are like cases of unitization in the end state (you detect a whole pattern), but there is no need for the prior state—no need for that pattern to have previously looked like two or more objects, properties, or events. This is because in stimulus imprinting, the perceptual system builds specialized detectors for whole stimuli or parts of stimuli to which a subject has been repeatedly exposed (Goldstone 1998: 591). Cells in the inferior temporal cortex, for instance, can have a heightened response to particular familiar faces (Perrett et al., 1984, cited in Goldstone 1998: 594). One area where these specialized detectors are helpful is with unclear or quickly presented stimuli (Goldstone 1998: 592). Stimulus imprinting happens entirely without guidance or supervision (Goldstone 2003: 241).
3. The Philosophical Significance of Perceptual Learning
Perceptual learning is philosophically significant both in itself, and for the role that it has played in prior philosophical discussions. Sections 3.1–3.4 will focus on the latter. However, there are good reasons to see perceptual learning as philosophically significant in itself, independently from the role that it has played in prior philosophical discussions.
Why is perceptual learning philosophically significant? One reason is that it says something about the very nature of perception—that perception is more complex than it might seem from the first-person point of view. Specifically, the fact that perceptual learning occurs means that the causes of perceptual states are not just the objects in our immediate environment, as it seems at first glance. Rather, given the reality of perceptual learning, there is a long causal history to our perceptions that involves prior perception. When the expert wine-taster tastes the Cabernet Sauvignon, for example, that glass of wine alone is not the sole cause of her perceptual state. Rather, the cause of her perceptual state includes prior wines and prior perceptions of those wines. One way to put this is to say that perception is more than the immediate inputs into our senses. It is tied to our prior experiences.
Another way in which perceptual learning is philosophically significant is because it shows how perception is a product of both the brain and the world. In this respect, there are some similarities between the role of constancy mechanisms and the role of perceptual learning, in that both involve the brain playing a role in structuring perception in a way that goes beyond the perceptual input. Constancy mechanisms, such as those involved in shape, size, and color constancy, are brain mechanisms that allow us to perceive shapes, sizes, and colors more stably across variations in distance or illumination. In cases of constancy, the brain manipulates the input from the world, and this allows the perceiver to track the shape, size, or color more easily. Similarly, in cases of perceptual learning, the brain manipulates the input from the world. In many cases, this may actually make the perception more helpful, as when through learning the perceptual system weights attention in a particular way, say, towards the features relevant for identifying a Cabernet Sauvignon. Perceptual learning might upgrade the epistemic status of perception, putting the perceiver in a better position with respect to knowledge (see Siegel 2017). At the same time, people can learn incorrectly, leading to perceptions that are unhelpful, as when a new golfer with inadequate coaching develops the bad habit of attending to their putter while putting, rather than attending to the golf ball.
Perceptual learning is philosophically significant in itself. In addition, the rest of section 3 goes on to explore the role that perceptual learning has played in prior philosophical discussions.
In the philosophy literature, cases of perceptual learning have often been used to show that through learning we come to represent new properties in perception, which we did not represent prior to learning. Siegel (2006, 2010), for instance, asks us to suppose that we have been tasked to cut down all and only the pine trees in a particular grove of trees. After several months pass, she says, pine trees might begin to look different to us. This is a case of perceptual learning, a long-term change in our perception following practice or experience with pine trees. Siegel uses the case to argue that perception comes to represent kind properties, like the property of being a pine tree . The idea is that the best way to explain the change in perception is that perception represents the property of being a pine tree after, but not before, learning takes place. That property becomes part of the content of perception: it comes to be presented in perceptual experience (for more background on the contents of perception, see Siegel 2016).
Thomas Reid’s notion of acquired perception has recently been interpreted in a way similar to Siegel’s pine tree case. According to Reid, some of our perceptions, namely acquired perceptions, are the result of prior experience. For instance, Reid writes about how through experience we might come to “perceive that this is the taste of cyder,” or “that this is the smell of an apple,” or that “this [is] the sound of a coach passing” ([1764] 1997: 171). Rebecca Copenhaver (2010, 2016) has interpreted Reid as claiming that through experience properties like being a cider , being an apple , and being a coach can come to be part of the content of our perception.
Cases of perceptual learning might also be used to show that through learning we come to represent new properties in perception, even if those properties are simply low-level properties like colors, shapes, textures, and bare sounds, rather than high-level kind properties like being a pine tree or being a cider . For instance, in discussing the perceptual expertise of jewelers, the 14 th -century Hindu philosopher Vedānta Deśika writes,
[T]he difference among colours [of a precious stone], which was first concealed by their similarity, is eventually made apparent as something sensual…. (Freschi [trans.] manuscript, Other Internet Resources, pp. 12–13)
In this case, the jeweler comes to perceive new colors in the gemstone, which others cannot perceive. This is a case where through learning someone comes to perceive a new low-level property.
The cases from both Reid and Vedānta Deśika both speak to the internal complexity of perception mentioned in the previous section. If Vedānta Deśika’s description of the jeweler case is accurate, then perception is more than the inputs into our senses, since both an expert jeweler and a non-expert can have the same visual inputs, but have different perceptions. Similarly, to take a new example from Reid, suppose that a farmer acquires the ability to literally see the rough amount corn in a heap ([1764] 1997: 172). Since both a farmer and a non-farmer can have the same visual inputs, but have different perceptions, the causes of their perceptions are not just restricted to the immediate objects out in their environment. Perception is more complex than that.
One of the most detailed contemporary discussions of cases of perceptual learning is found in Siewert (1998: section 7.9). Siewert discusses in detail the role that learning plays in altering perceptual phenomenology, although he stops short of saying that this affects the high-level contents of perception. He writes, for instance, that there is a difference in perceptual phenomenology between just seeing “something shaped, situated, and colored in a certain way,” and recognizing that thing as a sunflower (or another type) (1998: 255). Siewert also writes that a person might look different to you after you know them for a long time than they did the first time you met them, and that your neighborhood might look different to you after you have lived there for a long time than the first time you moved in (pp. 256, 258). Furthermore, he writes about how a chessboard in midgame might look differently to a chess player than to a novice, and how a car engine might look differently to a mechanic than to someone unfamiliar with cars (1998: 258). These are all examples where learning affects one’s sensory phenomenology.
Several cases of perceptual learning in the philosophical literature involve language learning, both in the case of written and spoken language. As an example of the former, Christopher Peacocke writes that there is a difference
between the experience of a perceiver completely unfamiliar with Cyrillic script seeing a sentence in that script and the experience of one who understands a language written in that script. (1992: 89)
With regard to spoken language, as Casey O’Callaghan (2011) points out, several philosophers have made the claim that after a person learns a spoken language, sounds in that language comes to sound different to them (O’Callaghan cites Block 1995: 234; Strawson 2010: 5–6; Tye 2000: 61; Siegel 2006: 490; Prinz 2006: 452; and Bayne 2009: 390). Ned Block, for instance, writes, “[T]here is a difference in what it is like to hear sounds in French before and after you have learned the language” (1995: 234). It is tempting to think that this difference is explicable in terms of the fact that, after learning a language, a person hears the meanings of the words, where they do not before learning the language. On such a view, meanings would be part of the contents of auditory perception. However, O’Callaghan (2011) denies this (see also O’Callaghan 2015 and Reiland 2015). He argues that the difference is in fact due to a kind of perceptual learning. Specifically, through learning we come to hear phonological features specific to the new language. As O’Callaghan argues, these phonological features, not the meanings, explain what it’s like to hear a new language.
By contrast, Brogaard (forthcoming) argues that meanings are in fact part of the content of perception (see also Pettit 2010). After offering arguments against the opposing view, she relies on evidence about perceptual learning to help make the positive case for her view. In particular, she uses evidence about perceptual learning to rebut the view that we use background information about context and combine it with what we hear, in order to get meanings. Instead, she argues, language learning is perceptual in nature. She points to changes in how we perceive utterances, more in chunks rather than in parts, as a result of learning. Background information directly influences what we hear, she argues, altering how language sounds to us.
Both Siegel’s pine tree case and the case of hearing a new language fundamentally involve phenomenal contrasts. That is, the motivating intuition in both cases is that there is a contrast in sensory phenomenology between two perceptual experiences. Interestingly, in both cases the phenomenal contrast is due to learning. The question in both the pine tree case and the new language case is what explains the difference in sensory phenomenology. Siegel argues that the best explanation in the pine tree case is that the property of being a pine (and, more generally, natural kind properties) can come to be represented in perception. O’Callaghan (2011) argues that the best explanation for the difference in sensory phenomenology in the new language case is that we come to hear phonological features specific to the new language. Brogaard (forthcoming) argues that the best explanation in that case is that we come to hear meanings in the new language.
Recall that cases of cognitive penetration are cases where one’s beliefs, thoughts, or desires influence one’s perception (see Macpherson 2012: 24). One role of perceptual learning in the philosophical literature has been to explain away putative cases of cognitive penetration. For instance, it might seem at first glance that Siegel’s pine tree case is a case of cognitive penetration, a case where one’s newly acquired concept of a pine tree influences one’s perception. Connolly (2014b) and Arstila (2016), however, have both argued that the best way to understand Siegel’s pine tree case is not as a case of cognitive penetration, but rather through the particular mechanisms of perceptual learning. Connolly counts it as a case of attentional weighting, while Arstila understands it as involving both unitization and differentiation.
One reason why perceptual learning is a good instrument for explaining away putative cases of cognitive penetration is the following. In cases of perceptual learning, it is the external environment that drives the perceptual changes. As Raftopoulos puts it, “perceptual learning does not necessarily involve cognitive top-down penetrability but only data-driven processes” (2001: 493). For putative cases of cognitive penetration, the strategy for the perceptual learning theorist is to show how the perceptual changes involved may have been data-driven instead of top-down. Several philosophers have used this strategy at times, including Pylyshyn (1999: section 6.3), Brogaard and Gatzia (2015: 2), as well as Stokes (2015: 94), and Deroy (2013) might be interpreted in that way as well.
One exception to the trend of explaining away putative cases of cognitive penetration in terms of perceptual learning is Cecchi (2014). Cecchi argues that a particular case of perceptual learning—that found in Schwartz, Maquet, and Frith (2002)—should count as a case of cognitive penetration. The study in question found changes in the primary visual cortex due to learning, and also that these changes were brought about by higher areas in the brain influencing the primary visual cortex. Because the perceptual changes were the result of top-down influence, Cecchi argues that this case of perceptual learning should count as a case of cognitive penetration.
One traditional debate in the philosophy of science is whether scientific observation is permeated with the theory of the scientist, or theory-laden (see the entry on theory and observation in science ). As Raftopoulos and Zeimbekis point out, when asking whether observation is theory-laden, the answer will depend in part on what it means for a subject to possess a theory (2015: 18). On their view, theories can be tacit, rather than just “having a set of beliefs and concepts” (p. 18).
Assuming that theories can be held tacitly, perceptual learning might plausibly play a role in making observation theory laden. Raftopoulos and Zeimbekis, for instance, ask us to imagine a scientist who has undergone perceptual learning in her expert domain (2015: 19). Specifically, through repeated exposure to items in her expert domain, she has developed perceptual sensitivity to certain features, in accordance with her professional needs. This includes learned attention to particular dimensions, and involves physical changes early in her visual system (p. 19). As a result, the scientist might quite literally see the world differently within her expert domain than someone from outside her expertise would see it.
Such a case suggests that perceptual learning can make observation theory-laden. The scientist’s perceptual system comes to shape the kind of visual information that makes it into the scientist’s conscious perception, and does so based on her professional needs. As Raftopoulos and Zeimbekis put it, the case suggests
that non-cognitive, clearly perceptual influences on incoming visual information can be indirect bearers of the kinds of theoretical commitments that we usually think of as the content of conceptually couched theories. (2015: 19)
Although the case does not involve explicit beliefs directly influencing perception, arguably it involves a theory being held tacitly and appropriated into one’s perceptual system.
According to the modular view of the mind (Fodor 1983), the basic systems involved in perception are encapsulated from information outside of it, excluding its inputs (see Robbins 2015, for a summary of modularity). It might then seem at first glance that cases of perceptual learning challenge the view that the mind is modular, at least insofar as they involve the modulation of perception through any background theory that the subject has. However, it is important to note that Fodor himself seems to allow for such cases of perceptual learning. While he thinks that perception is synchronically impenetrable, he allows for the possibility of diachronic penetration, that is, cases where “experience and training can affect the accessibility of background theory to perceptual mechanisms” (1984: 39).
Why think that a modular view of the mind should allow for diachronic penetration? When Fodor allows for diachronic penetration, he does so because the alternative is to say that all modular systems are specified endogenously (1984: 39). Fodor admits that this alternative would be too extreme, and he points out, for instance, that children learn something from hearing a language. In other words, the modules for language are not just specified endogenously. However, Fodor is conservative about the scope of diachronic penetration, suggesting that it may only happen within strict limits, perhaps limits that are themselves endogenously defined (1984: 39–40).
Other philosophers have argued that diachronic penetration of perception undermines modularity. Churchland (1988), for instance, sees Fodor’s allowance of diachronic penetration as “grudgingly conceded,” and he argues that diachronic penetration is in fact widespread, rather than something that happens within strict limits (p. 176). One such case, raised by Churchland, is the case of perceiving music. Churchland argues that a person who knows the relevant music theory and vocabulary “perceives, in any composition whether great or mundane, a structure, development, and rationale that is lost on the untrained ear” (1988: 179). Fodor replies that it is unclear whether such cases are genuinely perceptual (1988: 195). He suggests another possibility, which is that the person who knows the relevant music theory does not perceive it differently, but rather forms different beliefs about the music. Furthermore, even if the case is genuinely perceptual, Fodor replies that it could be that a trained ear results simply from repeated exposure to the relevant music, rather than through knowledge of theory (1988: 195).
- Ahissar, Merav & Shaul Hochstein, 2004, “The Reverse Hierarchy Theory of Visual Perceptual Learning”, Trends in cognitive sciences , 8(10): 457–464. doi:10.1016/j.tics.2004.08.011
- Arstila, Valtteri, 2016, “Perceptual Learning Explains Two Candidates for Cognitive Penetration”, Erkenntnis , 81(6): 1151–1172. doi:10.1007/s10670-015-9785-3
- Bao, Min, Lin Yang, Cristina Rios, Bin He, & Stephen A. Engel, 2010, “Perceptual Learning Increases the Strength of the Earliest Signals in Visual Cortex”, The Journal of Neuroscience , 30(45): 15080–15084. doi:10.1523/JNEUROSCI.5703-09.2010
- Bayne, Tim, 2009, “Perception and the Reach of Phenomenal Content”, The Philosophical Quarterly , 59(236): 385–404. doi:10.1111/j.1467-9213.2009.631.x
- Biederman, Irving & Margaret M. Shiffrar, 1987, “Sexing Day-Old Chicks: a Case Study and Expert Systems Analysis of a Difficult Perceptual-Learning Task”, Journal of Experimental Psychology: Learning, Memory, and Cognition , 13(4): 640–645. doi:10.1037/0278-7393.13.4.640
- Block, Ned, 1995, “On a Confusion About a Function of Consciousness”, Behavioral and Brain Sciences , 18(2): 227–247. doi:10.1017/S0140525X00038188
- Brogaard, Berit, forthcoming, “In Defense of Hearing Meanings”, Synthese .
- Brogaard, Berit & Dimitria Electra Gatzia, 2015, “Is the Auditory System Cognitively Penetrable?” Frontiers in Psychology , 6: 1166. doi:10.3389/fpsyg.2015.01166
- Bryan, William Lowe & Noble Harter, 1897, “Studies in the Physiology and Psychology of the Telegraphic Language”, Psychological Review , 4(1): 27–53. doi:10.1037/h0073806
- –––, 1899, “Studies on the Telegraphic Language. The Acquisition of a Hierarchy of Habits”, Psychological Review , 6(4): 345–375. doi:10.1037/h0073117
- Bushnell, I.W.R., F. Sai, & J.T. Mullin, 1989, “Neonatal Recognition of the Mother’s Face”, British Journal of Developmental Psychology , 7(1): 3–15. doi:10.1111/j.2044-835X.1989.tb00784.x
- Carey, Susan, 2009, The Origin of Concepts , New York: Oxford University Press.
- Cecchi, Ariel S., 2014, “Cognitive Penetration, Perceptual Learning and Neural Plasticity”, dialectica , 68(1): 63–95. doi:10.1111/1746-8361.12051
- Chudnoff, Elijah, forthcoming, “The Epistemic Significance of Perceptual Learning”, Inquiry .
- Churchland, Paul M., 1988, “Perceptual Plasticity and Theoretical Neutrality: A Reply to Jerry Fodor”, Philosophy of Science , 55(2): 167–187. doi:10.1086/289425
- Connolly, Kevin, 2014a, “Multisensory Perception as an Associative Learning Process”, Frontiers in Psychology , 5: 1095, 1095. doi:10.3389/fpsyg.2014.01095
- –––, 2014b, “Perceptual Learning and the Contents of Perception”, Erkenntnis , 79(6): 1407–1418. doi:10.1007/s10670-014-9608-y
- –––, forthcoming, “Sensory Substitution and Perceptual Learning”, in Sensory Substitution and Augmentation , Fiona Macpherson (ed.), Oxford: Oxford University Press.
- Copenhaver, Rebecca, 2010, “Thomas Reid on Acquired Perception”, Pacific Philosophical Quarterly , 91(3): 285–312. doi:10.1111/j.1468-0114.2010.01368.x
- –––, 2016, “Additional Perceptive Powers: Comments on Van Cleve's Problems from Reid”, Philosophy and Phenomenological Research , 93(1): 218–224. doi:10.1111/phpr.12317
- Cowie, Fiona, 2016, “Innateness and Language”, The Stanford Encyclopedia of Philosophy (Winter 2016 Edition), Edward N. Zalta (ed.), URL = < https://plato.stanford.edu/archives/win2016/entries/innateness-language/ >.
- Crawford, Kate, 2016, “Artificial Intelligence’s White Guy Problem”, The New York Times , June 25, 2016, p. SR11.
- Deroy, Ophelia, 2013, “Object-Sensitivity Versus Cognitive Penetrability of Perception”, Philosophical Studies , 162(1): 87–107. doi:10.1007/s11098-012-9989-1
- Dretske, Fred, 2015, “Perception versus Conception: The Goldilocks Test”, in Zeimbekis & Raftopoulos 2015: pp. 163-173. doi:10.1093/acprof:oso/9780198738916.003.0007
- Dy, Jennifer G. & Carla E. Brodley, 2004, “Feature Selection for Unsupervised Learning”, Journal of Machine Learning Research , 5(Aug): 845–889. [ Dy & Brodley 2004 available online ]
- Fahle, Manfred, 2002, “Introduction”, in Manfred Fahle & Tomaso A. Poggio (eds.) Perceptual Learning , Cambridge, MA: MIT Press, pp. ix–xx.
- Fodor, Jerry, 1983, The Modularity of Mind , Cambridge, MA: MIT Press.
- Fodor, Jerry, 1984, “Observation Reconsidered”, Philosophy of Science , 51(1): 23–43. doi:10.1086/289162
- Fodor, Jerry, 1988, “A Reply to Churchland's ‘Perceptual Plasticity and Theoretical Neutrality’”, Philosophy of Science , 55(2): 188-198. doi:10.1086/289426
- Garraghty, Preston E. & Jon H. Kaas, 1992, “Dynamic Features of Sensory and Motor Maps”, Current Opinion in Neurobiology , 2(4): 522–527. doi:10.1016/0960-9822(92)90655-T
- Gauthier, Isabel & Michael J. Tarr, 1997, “Becoming a ‘Greeble’ Expert: Exploring Mechanisms for Face Recognition”, Vision Research , 37(12): 1673–1682. doi:10.1016/S0042-6989(96)00286-6
- Gendler, Tamar Szabo & John Hawthorne (eds), 2006, Perceptual Experience , Oxford: Oxford University Press. doi:10.1093/acprof:oso/9780199289769.001.0001
- Gibson, Eleanor J., 1963, “Perceptual Learning”, Annual Review of Psychology , 14: 29–56. doi:10.1146/annurev.ps.14.020163.000333
- –––, 1969, Principles of Perceptual Learning and Development , New York: Appleton-Century-Crofts.
- Gibson, Eleanor J. & Richard D. Walk, 1956, “The Effect of Prolonged Exposure to Visually Presented Patterns on Learning to Discriminate Them”, Journal of Comparative and Physiological Psychology , 49(3): 239–242. doi:10.1037/h0048274
- Gibson, Eleanor J., Cynthia J. Owsley, Arlene Walker, & Jane Megaw-Nyce, 1979, “Development of the Perception of Invariants: Substance and Shape”, Perception , 8(6): 609–19. doi:10.1068/p080609
- Gibson, James J. & Eleanor J. Gibson, 1955, “Perceptual Learning: Differentiation or Enrichment?” Psychological Review , 62: 32–41. doi:10.1037/h0048826
- Gilbert, Charles D., 1996, “Plasticity in Visual Perception and Physiology”, Current Opinion in Neurobiology , 6(2): 269–274.
- Gilbert, Charles D. & Wu Li, 2012, “Adult Visual Cortical Plasticity”, Neuron , 75(2): 250–264. doi:10.1016/j.neuron.2012.06.030
- Goldstone, Robert L., 1998, “Perceptual Learning”, Annual Review of Psychology , 49: 585–612. doi:10.1146/annurev.psych.49.1.585
- –––, 2003, “Learning to Perceive While Perceiving to Learn”, in Perceptual Organization in Vision: Behavioral and Neural Perspectives , Ruth Kimchi, Marlene Behrmann, & Carl R. Olson (eds), Mahwah, NJ: Lawrence Erlbaum Associates, pp. 233–278.
- –––, 2010, “Foreword” to Isabel Gauthier, Michael Tarr, & Daniel Bub, Perceptual Expertise: Bridging Brain and Behavior , Oxford/New York: Oxford University Press, p. v–ix. doi:10.1093/acprof:oso/9780195309607.001.0001
- Goldstone, Robert L., David W. Braithwaite, & Lisa A. Byrge, 2012, “Perceptual Learning”, in Norbert M. Seel (ed.), Encyclopedia of the Sciences of Learning , Heidelberg, Germany: Springer Verlag GmbH, pp. 2580–2583. doi:10.1007/978-1-4419-1428-6_147
- Goldstone, Robert L. & Lisa A. Byrge, 2015, “Perceptual Learning”, in The Oxford Handbook of the Philosophy of Perception , Mohan Matthen (ed.), Oxford: Oxford University Press, pp. 812–832. doi:10.1093/oxfordhb/9780199600472.013.029
- Griffiths, Paul, 2009, “The Distinction Between Innate and Acquired Characteristics”, The Stanford Encyclopedia of Philosophy (Fall 2009 Edition), Edward N. Zalta (ed.), URL = < http://plato.stanford.edu/archives/fall2009/entries/innate-acquired/ >.
- Hagemann, Norbert, Jörg Schorer, Rouwen Cañal-Bruland, Simone Lotz, & Bernd Strauss, 2010, “Visual Perception in Fencing: Do the Eye Movements of Fencers Represent Their Information Pickup?” Attention, Perception, & Psychophysics , 72(8): 2204–2214. doi:10.3758/BF03196695
- Hall, Geoffrey, 1991, Perceptual and Associative Learning , Oxford: Clarendon Press. doi:10.1093/acprof:oso/9780198521822.001.0001
- Hatfield, Gary, 2009, “Perception as Unconscious Inference”, in Gary Hatfield, Perception and Cognition: Essays in the Philosophy of Psychology , Oxford: Oxford University Press, pp. 124–152.
- Held, R., 1985, “Binocular Vision: Behavioral and Neuronal Development”, in Jacques A. Mehler, & Robin Fox (eds.), Neonate Cognition: Beyond the Blooming Buzzing Confusion , Hillsdale, NJ: Erlbaum, pp. 37–44.
- James, William, 1890, The Principles of Psychology , New York: Henry Holt and Company.
- Kellman, Philip J., 2002, “Perceptual Learning”, in Hal Pashler & Randy Gallistel (eds.), Stevens’ Handbook of Experimental Psychology: Learning, Motivation, and Emotion (Vol. 3, 3rd edition), New York, NY: John Wiley & Sons, pp. 259–299.
- Kellman, Philip J. & Patrick Garrigan, 2009, “Perceptual Learning and Human Expertise”, Physics of Life Reviews , 6(2): 53–84. doi:10.1016/j.plrev.2008.12.001
- Kellman, Philip J. & Christine M. Massey, 2013, “Perceptual Learning, Cognition, and Expertise”, in B.H. Ross (ed.), The Psychology of Learning and Motivation , Vol. 58, Academic Press, Elsevier Inc., pp. 117–165. doi:10.1016/B978-0-12-407237-4.00004-9
- Kellman, Philip J. & Elizabeth S. Spelke, 1983, “Perception of Partly Occluded Objects in Infancy”, Cognitive Psychology , 15(4): 483–524. doi:10.1016/0010-0285(83)90017-8
- LeCun, Yann, Yoshua Bengio, & Geoffrey Hinton, 2015, “Deep Learning”, Nature , 521(7553): 436–444. doi:10.1038/nature14539
- Logan, John S., Scott E. Lively, & David B. Pisoni, 1991, “Training Japanese Listeners to Identify English /r/ and /l/: A First Report”, The Journal of the Acoustical Society of America , 89(2): 874–886.
- Lyons, Jack C., 2005, “Perceptual Belief and Nonexperiential Looks”, Philosophical Perspectives , 19(1): 237–256.
- –––, 2009, Perception and Basic Beliefs: Zombies, Modules, and the Problem of the External World , New York: Oxford University Press. doi:10.1093/acprof:oso/9780195373578.001.0001
- Macpherson, Fiona, 2012, “Cognitive Penetration of Colour Experience: Rethinking the Issue in Light of An Indirect Mechanism”, Philosophy and Phenomenological Research , 84(1): 24–62. doi:10.1111/j.1933-1592.2010.00481.x
- Markie, Peter, 2015, “Rationalism vs. Empiricism”, The Stanford Encyclopedia of Philosophy (Summer 2015 Edition), Edward N. Zalta (ed.), URL = < http://plato.stanford.edu/archives/sum2015/entries/rationalism-empiricism/ >.
- Matthen, Mohan, 2014, “How to Be Sure: Sensory Exploration and Empirical Certainty”, Philosophy and Phenomenological Research , 88(1): 38–69. doi:10.1111/j.1933-1592.2011.00548.x
- –––, forthcoming, “Play, Skill, and the Origins of Perceptual Art”, British Journal of Aesthetics .
- Meltzoff, Andrew N. & M. Keith Moore, 1977, “Imitation of Facial and Manual Gestures by Human Neonates”, Science , 198(4312): 75–78. doi:10.1126/science.198.4312.75
- Nanay, Bence, 2017, “Perceptual Learning, the Mere Exposure Effect and Aesthetic Antirealism”, Leonardo , 50(1): 58–63. doi:10.1162/LEON_a_01082
- Nevatia, Ramakant, 1982, Machine Perception , Englewood Cliffs, NJ: Prentice-Hall.
- O’Callaghan, Casey, 2011, “Against Hearing Meanings”, Philosophical Quarterly , 61(245): 783–807. doi:10.1111/j.1467-9213.2011.704.x
- –––, 2015, “Speech Perception”, in The Oxford Handbook of the Philosophy of Perception , Mohan Matthen (ed.), Oxford: Oxford University Press, pp. 475–494. doi:10.1093/oxfordhb/9780199600472.013.032
- Orlandi, Nico, 2014, The Innocent Eye: Why Vision is Not a Cognitive Process , Oxford: Oxford University Press. doi:10.1093/acprof:oso/9780199375035.001.0001
- Peacocke, Christopher, 1992, A Study of Concepts , Cambridge, MA: MIT Press.
- Perrett, David I., P.A.J. Smith, Douglas David Potter, A.J. Mistlin, A.D. Head, & M.A. Jeeves, 1984, “Neurones Responsive to Faces in the Temporal Cortex: Studies of Functional Organization, Sensitivity to Identity and Relation to Perception”, Human Neurobiology , 3(4): 197–208.
- Pettit, Dean, 2010, “On the Epistemology and Psychology of Speech Comprehension”, The Baltic International Yearbook of Cognition, Logic and Communication , 5(Meaning, Understanding and Knowledge): 1–43. doi:10.4148/biyclc.v5i0.286
- Pourtois, Gilles, Karsten S. Rauss, Patrik Vuilleumier, & Sophie Schwartz, 2008, “Effects of Perceptual Learning on Primary Visual Cortex Activity in Humans”, Vision Research , 48(1): 55–62. doi:10.1016/j.visres.2007.10.027
- Prinz, Jesse, 2006, “Beyond Appearances: the Content of Sensation and Perception”, in Gendler & Hawthorne 2006: 434–459. doi:10.1093/acprof:oso/9780199289769.003.0013
- Pylyshyn, Zenon, 1999, “Is Vision Continuous with Cognition? The Case for Cognitive Impenetrability of Visual Perception”, Behavioral and Brain Sciences , 22(3): 341–365.
- Raftopoulos, Athanassios, 2001, “Is Perception Informationally Encapsulated? The Issue of the Theory-Ladenness of Perception”, Cognitive Science , 25(3): 423–451. doi:10.1207/s15516709cog2503_4
- Raftopoulos, Athanassios & John Zeimbekis, 2015, “The Cognitive Penetrability of Perception: An Overview”, in Zeimbekis & Raftopoulos 2015: pp. 1-56. doi:10.1093/acprof:oso/9780198738916.003.0001
- Reiland, Indrek, 2015, “On Experiencing Meanings”, The Southern Journal of Philosophy , 53(4): 481–492. doi:10.1111/sjp.12150
- Reid, Thomas, [1764] 1997, An Inquiry into the Human Mind on the Principles of Common Sense , D.R. Brookes (ed.), Edinburgh: Edinburgh University Press.
- –––, [1785] 2002, Essays on the Intellectual Powers of Man , D.R. Brookes (ed.), Edinburgh: Edinburgh University Press.
- Robbins, Philip, 2015, “Modularity of Mind”, The Stanford Encyclopedia of Philosophy (Summer 2015 Edition), Edward N. Zalta (ed.), URL = < http://plato.stanford.edu/archives/sum2015/entries/modularity-mind/ >.
- Sagi, Dov, 2011, “Perceptual Learning in Vision Research”, Vision Research , 51(13): 1552–1566. doi:10.1016/j.visres.2010.10.019
- Samet, Jerry, 2008, “The Historical Controversies Surrounding Innateness”, The Stanford Encyclopedia of Philosophy (Fall 2008 Edition), Edward N. Zalta (ed.), URL = < https://plato.stanford.edu/archives/fall2008/entries/innateness-history/ >.
- Schwartz, Sophie, Pierre Maquet, & Chris Frith, 2002, “Neural Correlates of Perceptual Learning: A Functional MRI Study of Visual Texture Discrimination”, Proceedings of the National Academy of Sciences , 99(26): 17137–17142. doi:10.1073/pnas.242414599
- Siegel, Susanna, 2006, “Which Properties Are Represented in Perception?” in Gendler & Hawthorne 2006: 481–503. doi:10.1093/acprof:oso/9780199289769.003.0015
- –––, 2010, The Contents of Visual Experience , Oxford: Oxford University Press.
- –––, 2012, “Cognitive Penetrability and Perceptual Justification”, Noûs , 46(2): 201–222. doi:10.1111/j.1468-0068.2010.00786.x
- –––, 2016, “The Contents of Perception”, The Stanford Encyclopedia of Philosophy (Spring 2016 Edition), Edward N. Zalta (ed.), URL = < http://plato.stanford.edu/archives/spr2016/entries/perception-contents/ >.
- –––, 2017, The Rationality of Perception , New York: Oxford University Press.
- Siewert, Charles P., 1998, The Significance of Consciousness , Princeton, NJ: Princeton University Press.
- Shiffrin, Richard M. & Walter Schneider, 1977, “Controlled and Automatic Human Information Processing: II. Perceptual Learning, Automatic Attending and a General Theory”, Psychological Review , 84(2): 127–190. doi:10.1037/0033-295X.84.2.127
- Slater, Alan, Anne Mattock, & Elizabeth Brown, 1990, “Size Constancy at Birth: Newborn Infants’ Responses to Retinal and Real Size”, Journal of Experimental Child Psychology , 49(2): 314–322.
- Smith Edward E. & Susan E. Haviland, 1972, “Why Words Are Perceived More Accurately Than Nonwords: Inference Versus Unitization”, Journal of Experimental Psychology , 92(1): 59–64
- Stanley, Jason & John W. Krakauer, 2013, “Motor Skill Depends on Knowledge of Facts”, Frontiers in Human Neuroscience , 7: 503 doi:10.3389/fnhum.2013.00503
- Stanley, Jason & Timothy Williamson, forthcoming, “Skill”, Noûs , early online 21 May 2016, doi:10.1111/nous.12144
- Stokes, Dustin, 2015, “Towards a Consequentialist Understanding of Cognitive Penetration”, in Zeimbekis & Raftopoulos 2015: pp. 75-100. doi:10.1093/acprof:oso/9780198738916.003.0003
- Strawson, Galen, [1994] 2010, Mental Reality , 2nd edition, Cambridge, MA: MIT Press.
- Tye, Michael, 2000, Consciousness, Color, and Content , Cambridge, MA: MIT Press.
- Vaassen, Bram M.K., 2016, “Basic Beliefs and the Perceptual Learning Problem: A Substantial Challenge for Moderate Foundationalism”, Episteme , 13(1): 133–149. doi:10.1017/epi.2015.58
- Webster, Michael A., 2012, “Evolving Concepts of Sensory Adaptation”, F1000 Biology Reports , 4: 21. doi:10.3410/B4-21 [ Webster 2012 available online ]
- Williams, A.M. & K. Davids, 1998, “Visual Search Strategy, Selective Attention, and Expertise in Soccer”, Research Quarterly for Exercise and Sport , 69(2): 111–128. doi:10.1080/02701367.1998.10607677
- Wu, Wayne, 2014, Attention , New York: Routledge.
- Zeimbekis, John & Athanassios Raftopoulos (eds.), 2015, The Cognitive Penetrability of Perception New Philosophical Perspectives , Oxford: Oxford University Press. doi:10.1093/acprof:oso/9780198738916.001.0001
How to cite this entry . Preview the PDF version of this entry at the Friends of the SEP Society . Look up topics and thinkers related to this entry at the Internet Philosophy Ontology Project (InPhO). Enhanced bibliography for this entry at PhilPapers , with links to its database.
- Freschi, Elisa., manuscript, “ The Refutation of Any Extra-Sensory Perception in Vedānta Deśika: A Philosophical Appraisal of Seśvaramīmāṃsā ad MS 1.1.4 .”
innateness: and contemporary theories of cognition | mind: modularity of | perception: experience and justification | perception: the contents of | science: theory and observation in
Acknowledgments
Special thanks for their comments to Susanna Siegel, Rebecca Copenhaver, and to the University of Pennsylvania Perceptual Learning Reading Group: Gary Hatfield, Adrienne Prettyman, Louise Daoust, Ting Fung Ho, Ben White, and Devin Curry. This entry was prepared with funding from the Cambridge New Directions in the Study of the Mind Project.
Copyright © 2017 by Kevin Connolly < kevinlconnolly @ gmail . com >
- Accessibility
Support SEP
Mirror sites.
View this site from another server:
- Info about mirror sites
The Stanford Encyclopedia of Philosophy is copyright © 2023 by The Metaphysics Research Lab , Department of Philosophy, Stanford University
Library of Congress Catalog Data: ISSN 1095-5054
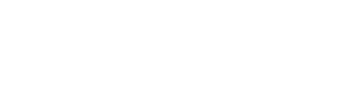
PERCEPTUAL CYCLE HYPOTHESIS
the theory that cognition impacts perceptual exploration but is thereby changed by real-world encounters, cultivating a cycle of attention , cognition, comprehension, and the authentic world wherein each impacts the others.
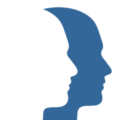
Leave a Reply
Your email address will not be published. Required fields are marked *
Latest Posts
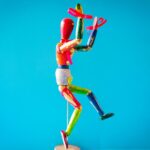
A Brief History of Brainwashing: The Science of Thought Control
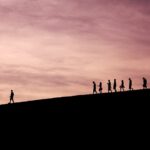
A Deep Dive into the Social Psychology of Leadership
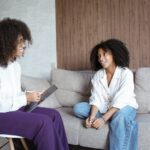
Counseling Approaches to Client Care: Theories to Apply in Practice
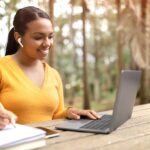
The Future Of Education: Can You Earn A Psychology Degree Online?
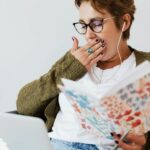
Insomnia & Mental Illness: What is the Correlation?
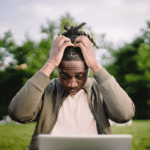
Stop Guessing: Here Are 3 Steps to Data-Driven Psychological Decisions
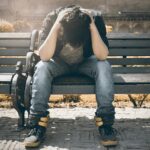
Getting Help with Grief: Understanding Therapy & How It Can Help
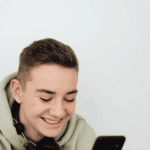
Exploring the Psychology of Risk and Reward
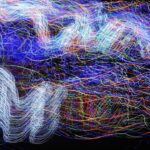
Understanding ADHD in Women: Symptoms, Treatment & Support
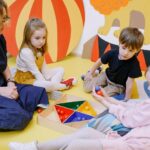
Meeting the Milestones: A Guide to Piaget's Child Developmental Stages
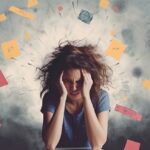
Counseling, Therapy, and Psychology: What Is The Difference?
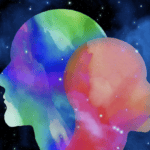
The Psychology of Metaphysical Belief Systems
Popular psychology terms, medical model, hypermnesia, affirmation, brainwashing, backup reinforcer, message-learning approach, affiliative behavior, social pressure, behavioral modeling, approach motivation.
The Common Kind Theory and The Concept of Perceptual Experience
- Original Research
- Published: 25 October 2021
- Volume 88 , pages 2847–2865, ( 2023 )
Cite this article
- Neil Mehta ORCID: orcid.org/0000-0002-6207-6778 1
238 Accesses
Explore all metrics
In this paper, I advance a new hypothesis about what the ordinary concept of perceptual experience might be. To a first approximation, my hypothesis is that it is the concept of something that seems to present mind-independent objects. Along the way, I reveal two important errors in Michael Martin’s argument for the very different view that the ordinary concept of perceptual experience is the concept of something that is impersonally introspectively indiscriminable from a veridical perception. This conceptual work is significant because it provides three pieces of good news for the common kind theorist.
This is a preview of subscription content, log in via an institution to check access.
Access this article
Price includes VAT (Russian Federation)
Instant access to the full article PDF.
Rent this article via DeepDyve
Institutional subscriptions
Similar content being viewed by others
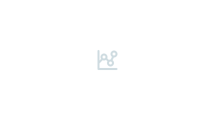
Property-Awareness and Representation
Phenomenal character and the epistemic role of perception, perceptual objectivity and the limits of perception.
Martin ( 2004 , p. 37).
I will be focusing on Martin’s most detailed presentation of the argument, which is in his (2004, pp. 47–52). But see also Martin ( 2006 ), which briefly revisits parts of this argument.
The common kind theory has so many advocates that it would be tedious to cite them all. Still, for some paradigms, see Tye ( 1995 ), Schellenberg ( 2018 ).
The notion of a fundamental kind has been developed in several subtly different ways. See for example Martin ( 2006 , pp. 360–361), Brewer ( 2011 , p. 3), Logue ( 2012 b, p. 174) and ( 2013 , p. 109).
Metaphysical disjunctivists (or those who accept some nearby view) include Hinton ( 1967 ), Campbell ( 2002 ), Martin ( 2004 ) and ( 2006 ), Snowdon ( 2005 ), Fish ( 2009 ), Nudds ( 2009 ), Brewer ( 2011 ), Logue ( 2012 ), Allen ( 2015 ), Genone ( 2016 ), Miracchi ( 2017 ), Moran ( 2018 ), French and Gomes ( 2019 ). For a bracingly clear overview of different forms of metaphysical disjunctivism, see Soteriou ( 2016 ).
I use the expressions reasonably natural property and natural kind in the sense of Lewis ( 1983 ).
I use the term “entity” as an especially broad sortal that includes properties, objects, events, states, etc.
Martin dubs this the “immodest view” (2004, pp. 47–48). However, since this is precisely the view that I wish to defend, I prefer the less prejudicial label given above. In addition, Martin does not speak of just one property E ; he speaks of a whole host of properties E 1 … E n . But you can think of E as the conjunction of E 1 … E n .
For a few advocates of the common kind theory, see fn. 3.
For advocates of metaphysical disjunctivism, see fn. 5.
Again, see Martin ( 2004 , pp. 75–76) and ( 2006 , §5). Martin takes inspiration from Hinton ( 1967 ). I have departed from Martin’s presentation in a few minor ways, however. First, Martin dubs this the “modest view,” but I will argue that there is nothing particularly modest about it; thus I prefer the more informative label given in the text. Second, Martin inquires into the ordinary concept of a perceptual experience of a street scene , but for our purposes I find it more helpful to inquire more generally into the ordinary concept of perceptual experience .
See Martin ( 2004 , pp. 74–81) and ( 2006 , pp. 379–96).
Martin ( 2004 , p. 49).
Ibid, p. 50.
Ibid, p. 49.
Ibid, pp. 49–50.
Ibid, p. 51.
I thank my undergraduate student Xianda Wen for the astute observation that seemings are sometimes inconsistent.
I owe this concern to an anonymous referee.
Martin ( 2004 , pp. 50–51).
Ibid, pp. 50–51.
At least, assuming that some perceptual experiences exist. (Otherwise there are no events that instantiate E , so telling whether or not an event instantiates these properties might turn out to be very easy).
Martin ( 2004 , pp. 51–52).
See their (2008, p. 75).
Ibid, p. 78.
I thank an anonymous referee for this suggestion.
My objection presupposes that sensations are not perceptual experiences. Can Martin reinstate his argument by denying this? Perhaps—but at this point in the dialectic, the onus is on him to defend this claim. He does not do so.
See Martin ( 2004 , pp. 37–38).
See Siegel ( 2004 , p. 94). For a response, see Martin ( 2004 , pp. 80–81). For what it is worth, I believe that Martin’s response does not handle all of the problematic cases.
See Siegel ( 2008 , pp. 218–223). For responses, see Nudds ( 2009 , pp. 342–343); Soteriou ( 2016 , ch. 6).
See Sturgeon ( 2008 , p. 134). For a response, see Nudds ( 2009 , p. 342).
See Siegel ( 2008 , pp. 211–214). For a response, see Nudds ( 2009 , pp. 342–343). For the record, I believe that Siegel’s objection is correct.
This phenomenon is well-known, though it has been called many different things—Millar ( 2014 , p. 240) gives an especially perspicuous description of it under the heading of object-immediacy . For other influential descriptions of this phenomenon, see Broad ( 1952 , p. 6); Alston ( 1999 , p. 182); Sturgeon ( 2000 , p. 9); Martin ( 2002 , p. 413); Levine ( 2006 , p. 179); and Brewer ( 2011 , p. 2).
For more discussion of these matters, see Mackie ( 2019 ).
As an anonymous referee observes, this view is by no means irresistible. Another option is to say that perceptual experiences and sensations both simply seem to present objects (while remaining silent on their mind-independence); perhaps perceptual experiences and sensations even belong to the same fundamental kind. If this is right, then we might instead consider:
The variant presentational semantic view : It is a conceptual truth that what it is to be a perceptual experience or sensation is to seem to present objects. (The property of seeming to present objects is thus experience-grounding.) In addition, this property is introspectible, and it is not perception-dependent. For the sake of simplicity, however, I will continue to work with the view in the text.
See Bayern et al. ( 2018 ).
Notice that, on this view, seeming (or purporting ) to present mind-independent objects does not require concept-possession, but introspectively seeming to present such objects does require concept-possession.
For the record, I am not just being coy here: I am not a representationalist. I prefer a pluralist theory of perception, one that blends certain elements of naïve realism and representationalism. See Mehta ( ms ).
Some would reject this last claim. For instance, some will think that veridical perceptions do not seem to present mind-independent objects, but just objects, simpliciter . I discuss this idea in more detail in fn. 40.
This is not quite right, since it is possible to hallucinate an impossible object such as an Escher staircase. But, borrowing an idea from Martin ( 2004 , pp. 80–81), the objection could be reformulated into something like this: surely what it is to seem to present mind-independent objects is just to be exhaustively decomposable into parts that each seem to be perceptions. I will ignore this nuance in what follows.
This seeming is not introspective, so we can still allow that a perception might introspectively seem to present mind- dependent objects. Again, this is one way to understand the case in which the subject mistakes a perception of a faint ringing sound for a ringing sensation.
It is worth mentioning an alternative approach. We might say that what it is to be a perceptual experience is to seem to present external (rather than mind-dependent) objects (see fn. 40); that what it is to be a perception is in fact to present external objects; and that what it is to be a sensation is in fact to present internal objects. Perhaps hallucinations are a subclass of sensations—the ones that in fact present internal objects but seem to present external objects. This approach can allow that some perceptions present, and correctly seem to present, objects that are external but mind-dependent. So this approach lets us reject the biconditional claim that something seems to present mind-independent objects just in case it seems to be a perception. The approach can also allow us to say that perceptions and sensations can be introspectively mistaken for one another, since the seemings invoked in the account are not introspective.
Some experiences might seem to present mind-independent objects and mind-dependent ones. How would I account for these? I would say that they are mixtures of perceptual experiences and sensations. (It is not surprising to posit mixed experiences. It is for instance entirely possible to mix perceptual experiences and imaginative ones, by imagining coffee in a cup that I see to be empty.) However, another option is to say that it is possible to perceive , in an unmixed way, mind-independent objects and mind-dependent ones, as long as all of these objects are external. See fns. 40 and 47 for a way to develop this idea.
See Martin ( 2004 , p. 71).
Ibid, pp. 68–70.
For other metaphysical disjunctivist attempts to fill this lacuna, see Alston ( 1999 , p. 191); Fish ( 2009 , p. 94); Allen ( 2015 ).
I use the terms reasonably natural property and natural kind in the sense of Lewis ( 1983 ).
For further discussion of fundamental kinds, see Mehta ( 2021 ).
Allen, K. (2015). Hallucination and imagination. Australasian Journal of Philosophy, 93 (2), 287–302.
Article Google Scholar
Alston, W. (1999). Back to the theory of appearing. Philosophical Perspectives, 13 , 181–203.
Google Scholar
Bayern, A. M. P., Danel, S., Auersperg, A. M. I., et al. (2018). Compound tool construction by New Caledonian crows. Scientific Reports, 8 (15676), 1–8.
Braddon-Mitchell, D. (2003). Qualia and analytical conditionals. Journal of Philosophy, 100 (3), 111–135.
Brewer, B. (2011). Perception and its objects . Oxford University Press.
Broad, C. (1952). Some elementary reflexions on sense-perception. Philosophy, 27 , 3–17.
Byrne, A., & Logue, H. (2008). Either/or. In A. Haddock & F. Macpherson (Eds.), Disjunctivism perception, action, knowledge (pp. 57–94). Oxford University Press.
Campbell, J. (2002). Reference and consciousness . Oxford University Press.
Chalmers, D. (2012). Constructing the world . Oxford University Press.
Fish, W. (2009). Perception, hallucination, and illusion . Oxford University Press.
French, C., & Gomes, A. (2019). How naïve realism can explain both the particularity and the generality of experience. Philosophical Quarterly, 69 (274), 41–63.
Genone, J. (2016). Recent work on naïve realism. American Philosophical Quarterly, 53 (1), 1–24.
Hinton, J. (1967). Visual experiences. Mind, 76 , 217–227.
Jackson, F. (1998). From metaphysics to ethics: A defence of conceptual analysis . Clarendon Press.
Kripke, S. (1972). Naming and necessity . Harvard University Press.
Levine, J. (2006). Conscious awareness and self-representation. In U. Kriegel & K. Williford (Eds.), Self-representational approaches to consciousness (pp. 173–198). MIT Press.
Lewis, D. (1983). New work for a theory of universals. Australasian Journal of Philosophy, 61 (4), 343–377.
Lewis, D. (1984). Putnam’s paradox. Australasian Journal of Philosophy, 62 (3), 221–236.
Logue, H. (2012). What should the naïve realist say about total hallucinations? Philosophical Perspectives, 26 , 173–199.
Logue, H. (2013). Good news for the disjunctivist about (one of) the bad cases. Philosophy and Phenomenological Research, 86 (1), 105–133.
Mackie, P. (2019). Perception, mind-independence, and Berkeley. Australasian Journal of Philosophy, 98 (3), 449–464.
Martin, M. (2002). The transparency of experience. Mind and Language, 17 (4), 376–425.
Martin, M. (2004). The limits of self-awareness. Philosophical Studies, 120 , 37–89.
Martin, M. (2006). On being alienated. In T. Gendler & J. Hawthorne (Eds.), Perceptual experience (pp. 354–410). Oxford University Press.
Mehta, N. (2021). “Naïve realism with many fundamental kinds.” Acta Analytica (online).
Mehta (ms). The many problems of perception.
Millar, B. (2014). The phenomenological directness of perceptual experience. Philosophical Studies, 170 , 235–253.
Miracchi, L. (2017). Perception first. Journal of Philosophy, 114 (12), 629–677.
Moran, A. (2018). Naïve realism, hallucination, and causation: A new response to the screening off problem. Australasian Journal of Philosophy, 97 (2), 368–382.
Nudds, M. (2009). Recent work in perception: Naïve realism and its opponents. Analysis Reviews, 69 (2), 334–346.
Schellenberg, S. (2018). The unity of perception: content, consciousness, evidence . Oxford University Press.
Siegel, S. (2004). Indiscriminability and the phenomenal. Philosophical Studies, 120 (1–3), 91–112.
Siegel, S. (2008). The epistemic conception of hallucination. In A. Haddock & F. Macpherson (Eds.), Disjunctivism (pp. 205–224). Oxford University Press.
Snowdon, P. (2005). The formulation of disjunctivism: A response to fish. Proceedings of the Aristotelian Society, 105 (1), 129–141.
Soteriou, M. (2016). Disjunctivism . Routledge.
Sturgeon, S. (2000). Matters of mind . Routledge.
Sturgeon, S. (2008). Disjunctivism about visual experience. In A. Haddock & F. Macpherson (Eds.), Disjunctivism: Perception, action, knowledge (pp. 112–143). Oxford University Pres.
Tye, M. (1995). Ten problems of consciousness: A representational theory of the phenomenal mind . MIT Press.
Download references
Author information
Authors and affiliations.
Yale-NUS College, Singapore, Singapore
You can also search for this author in PubMed Google Scholar
Corresponding author
Correspondence to Neil Mehta .
Additional information
Publisher's note.
Springer Nature remains neutral with regard to jurisdictional claims in published maps and institutional affiliations.
Rights and permissions
Reprints and permissions
About this article
Mehta, N. The Common Kind Theory and The Concept of Perceptual Experience. Erkenn 88 , 2847–2865 (2023). https://doi.org/10.1007/s10670-021-00480-z
Download citation
Received : 13 January 2021
Accepted : 10 October 2021
Published : 25 October 2021
Issue Date : October 2023
DOI : https://doi.org/10.1007/s10670-021-00480-z
Share this article
Anyone you share the following link with will be able to read this content:
Sorry, a shareable link is not currently available for this article.
Provided by the Springer Nature SharedIt content-sharing initiative
- Find a journal
- Publish with us
- Track your research
HYPOTHESIS AND THEORY article
Pleistocene hypothesis – moving savanna perceptual preference hypothesis beyond savanna.
- 1 Institute of Geography, Augsburg University, Augsburg, Germany
- 2 Faculty of Social Sciences/Psychology, Tampere University, Tampere, Finland
We provide an extension of the Savanna perceptual preference hypothesis (“Savanna Hypothesis”), supposing that interaction with landscapes offering survival advantage for human groups during evolution might have gradually evolved to permanent landscape preferences. This additional support is based on the palaeoenvironmental analysis of the spread of modern humans into Europe in the late Pleistocene and their living environments there. Our hypothesis is that the preference for park-like landscapes after African savannas experienced a kind of “refreshment” in the Pleistocene. Thus, preferences for certain types of natural settings and scenes may have a more continuous evolutionary history than previously thought. The extended Savanna Hypothesis termed “Pleistocene Hypothesis” might stimulate further work on this important topic linking human evolution and human environmental preferences.
Introduction
In this paper, we provide an extension of the perceptual Savanna Hypothesis supposing that human interaction with landscapes offering survival advantage during evolution might have gradually developed into enduring patterns to prefer certain landscapes. This additional support is based on the palaeoenvironmental analysis of the spread of modern humans into Europe and their living environments there. Different factors play a role in this process ( Klein, 2008 ). The role of climate forcing is one of them (e.g., Müller et al., 2011 ; Staubwasser et al., 2018 ). Another aspect is the innovation dynamic ( Shennan, 2001 ; Richerson et al., 2009 ), i.e., the acquired unique abilities to colonize new environments ( Hoffecker, 2009 ). The spread of early hominids can, however, also be explained by the acquisition of food, being essential for the development of the brain as the dispersal of early hominids is correlated to the historical migration of the African buffalo ( van Ginneken et al., 2017 ; van Ginneken, 2019 ). In this context, theories on the evolution of the human brain should further discuss the current way of landscape perception in relation to that of the hominids ( Chin et al., 2022 ).
But finally, when modern humans arrived in a new area what preferences did they have to answer the question: should I stay or should I go (further)?
The savanna hypothesis was originally formulated as an answer to why humans are bipedal. Thus, it places the agents of selection for bipedality on open grasslands, resulting from the transition of the human ancestors from an arboreal lifestyle to one on the savannas in response to the opening of the African landscape during long-term aridification of the continent ( Richmond et al., 2001 ). The second aspect of this hypothesis focuses on the psychological processes assuming that due to the long-term hominization in the East African savanna, such environments are part of general landscape preferences of humans. The basic claim of the Savanna Hypothesis is that humans have innate, even automatic tendencies toward preferring certain types of natural settings that provide food, water, and security for survival ( Ulrich, 1993 ). Ulrich (1983) has presented an integrated theory of esthetic and affective response to the natural environment assuming that landscape preferences can be defined as “the first level of reaction to the environment of generalized affect, such as liking or interest” simultaneous with or followed by approach-avoidance behavior ( Ulrich, 1983 , p. 90). These affective reactions to natural scenes are adaptive and foster well-being in terms of the total behavior of the individual. The initial affective reaction is elicited quickly by certain general properties or preferenda of the view, including gross structural aspects of settings, (e.g., focality, deflected vistas), gross depth properties that require little inference (spaciousness, ground surface texture conducive to movement) and general classes of environmental content, such as vegetation and water. Thus, in this theory, preferences are linked via preferenda to survival and well-being. Evolutionary psychology argues that many psychological mechanisms, such as preferences and emotions and behavioral strategies, are solutions to the adaptive problems our species has faced in natural evolution ( Buss, 1995 ). We believe that the scientific basis of a widespread Savanna hypothesis requires further study and we focus on theoretical research of evolutionary biology theories.
Fear and Preference, Savanna Hypothesis and Prospect Refuge Theory
For the longest time in human history our ancestors lived in hunter–gatherer bands, thus being intimately connected to other living organisms ( Wilson, 1984 ). Automatic reactions of fear and escape after the detection of predators were important agents of selection on ancestral humans ( Öhman and Mineka, 2001 ). Many studies stress the evolutionary origins of human fear of some animals and show evidence that people detect the presence of fear-relevant animals (i.e., snake, spider) faster than the presence of a pleasant stimulus (i.e., flower, mushroom) ( Öhman et al., 2001 ; Lipp et al., 2004 ). Even preschool children share this special attention to snakes and detect them faster than other stimuli (i.e., flowers, frogs, and caterpillars) ( LoBue and DeLoache, 2008 ). Thus, it forms an evolutionary advantage to recognize dangers quickly and to react to them as efficiently as possible. Therefore, wilderness may also be partly associated with death and mortality ( Koole and Van den Berg, 2005 ). On the other hand, research literature on preferences for natural settings shows that people generally prefer landscapes with a variety of landscape structures, open green spaces, differences in relief that enable views and orientation, as well as loose trees and the presence of water ( Ulrich, 1993 ; Hill and Daniel, 2007 ).
Such preferences seem to relate not only to savanna but rather to mosaic environments ( Domínguez-Rodrigo, 2014 ) and we develop this line of argument by first looking at the evidence on the preference of natural vs urban landscapes and then proceed to the preferred types and features of natural environments.
Several studies have consistently found empirical support for the assumption that people respond more positively to natural vs. urban environments ( Staats et al., 2003 ; Van den Berg et al., 2003 ; Berto, 2005 ). Urban environments in these studies refer to human-made, built environments, such as streetscapes and buildings. Many links between human well-being and nature contacts have been analyzed and numerous studies have highlighted the positive effects of different kinds of green spaces on human health, well-being and quality of life ( Hansmann et al., 2007 ; Abraham et al., 2010 ; Hartig et al., 2014 ; McMahan and Estes, 2015 ; Ideno et al., 2017 ; Twohig-Bennett and Jones, 2018 ; Bell et al., 2018 ; Grilli and Sacchelli, 2020 ; Rathmann et al., 2020 ; Rathmann, 2021 ). Supporting the notion of potentially hard-wired differences in brain activity, it has been shown that lower attentional demands for natural versus urban images can be detected within 1,200 milliseconds ( Grassini et al., 2019 ; see also Norwood et al., 2019 ). Rapid positive emotional reactions to natural scenes versus negative reactions to urban scenes are evoked in 200 ms ( Korpela et al., 2002 ). Savanna preference hypothesis also assumes that along with the preference reaction during evolution, a capacity for restorative, i.e., stress-reducing responding to certain natural settings has developed ( Ulrich, 1993 ). This would foster amelioration of stress responses after encounters with danger and threats, such as predators. Ample evidence shows that stress-reducing physiological responses, indexed by, e.g., heart rate variability, salivary cortisol, blood pressure and pulse rate, are detected more rapidly after a stressful situation while walking or sitting in natural settings than in urban settings ( Park et al., 2010 ; Bratman et al., 2012 ; Ideno et al., 2017 ; Twohig-Bennett and Jones, 2018 ; Mygind et al., 2019 ). The perception of environments’ “naturalness” and its biodiversity has also been associated with the restorative qualities of a setting ( Carrus et al., 2015 ).
To continue to the preferred types and features of landscapes, the theory of prospect and refuge can be traced back to the late nineteenth century and the anthropological belief in the human survival instinct leading to a stimulus, which directly connects human perceptions or reactions to environmental stimuli. The basic idea, that hominids have been living in the Savanna environments has started to be discussed since 1960, at least in archeology and history ( Bender et al., 2012 ), underlining the paleoanthropological perspective of the Savanna theory. The psychological perspective of this theory focuses on the needs of the hominids for survival.
The prospect-refuge theory, proposed by Appleton (1975 , 1996) , describes a human behavioral and psychological need for places that allow a person to see, but without being seen. The basic idea was to show a simple model, relating preferences to a typology of landscapes based on behavioral and biological sciences. The whole model was “an agent of simplification for explanatory purposes” ( Appleton, 1984 , p. 92). The reduction was made deliberately to facilitate an explanation. Environmental perception is the key to all adaptation processes and humans perceive their environment in another way than animals perceive their habitat. Some aspects of the habitat are more important for survival than others. “Prospect” and “refuge” are the important aspects of a landscape to improve the chances of survival. Further on, this theory tried to show a biological interpretation for landscape esthetics as it states that taste in art is an acquired preference for particular methods of satisfying inborn desires which are basically opportunity (prospect) and safety (refuge). Humans are attracted to specific circumstances (art, landscape) that have unoccluded vistas into the landscape (prospects further include hills, mountains and trees), visible places for easy refuge (e.g., climbable trees with dense canopies nearby, caves, dense vegetation) and additionally water, plants, prey species. Landscape preferences further include spaces, where we are rather on the edge than in the middle of a place, where we are most exposed and places where we are covered, compared to the open sky. Landscape preferences therefore focus on areas which are optimal for survival and reproduction. The Savanna Hypothesis ( Orians, 1980 ; Bender et al., 2012 ) argues that selection favored resource-rich environments whereas environments, lacking resources or with survival threats have been avoided. Such environments offered the essential landscape characteristics for survival of the early humans; the availability of resources, protection against predators, the possibility of orientation and overview in space are central requirements for a landscape that ensures the survival of early humans. These theoretical conceptions actually propose that not only certain landscape contents but certain structural properties might have been important for the development of permanent preferences. One such property has been described as “depth/spaciousness” characteristics that relate to surveillance, proximity to hidden threats, and escape opportunities ( Ulrich, 1993 ) or intermediate complexity or density of the scene ( Joye and van den Berg, 2011 ). Such structural emphasis may also fit with alternative theoretical explanations for the Savanna Hypothesis, such as the Perceptual Fluency Account (PFA). It states that natural scenes are affectively evaluated more positively than urban scenes because our visual system more fluently processes certain aspects of the visual structure of the former than of the latter ( Joye and van den Berg, 2011 ). In PFA, in contrast to psychophysiological and emotional theories supporting Savanna Hypothesis, it is the structure of the landscape, visual coherence and fractal patterns, rather than “unthreatening vegetated settings per se ” that might explain preference of greenspace ( Joye and van den Berg, 2011 ).
Emphasizing contents rather than structure, Heerwagen and Orians (1993) argue that our landscape preferences are innate, these preferences include open spaces of low grasses, the presence of water, flowering and fruiting plants, and evidence of animal life. Beside the prospect-refuge-theory and the Savanna Hypothesis, Kaplan’s landscape preference matrix theory ( Kaplan and Kaplan, 1989 ) and the stress recovery theory ( Ulrich et al., 1991 ) argue that humans prefer natural green environments. More importantly and in line with the concept of mosaic environments, in addition to the Savanna hypothesis, two other hypotheses with respect to the specific habitat where humans have evolved have been presented ( Han, 2007 ; Mangone et al., 2021 ). One option is the forest hypothesis, which argues that human evolution took place in closed, forested settings and the other one is the grassland–woodland hypothesis, which proposes that a mosaic of both settings was the adaptive environment for hominids.
Some of the empirical evidence for the preference for savanna environments is related to biomes as a whole but mainly it includes only some features of savanna environments. Concerning biomes, the evidence is mixed. In one study, photographs of five biomes, rain forest, deciduous forest, coniferous forest, savanna, and desert were rated by the inhabitants of the rainforest belt of Nigeria ( Falk and Balling, 2010 ). The results showed that savanna scenes were regarded as the most favorable place to live. In another study, college students’ psycho-physiological responses to the six major terrestrial biomes (desert, tundra, grassland, coniferous forest, deciduous forest, and tropical forest) showed that tundra and coniferous forest were the most favored biomes, whereas desert and grassland were the least favored ( Han, 2007 ). Yet another study with a student population showed that irrespective of familiarity, beaches and lakes were preferred more and marshes and swamps preferred less than the other six biome types (beach, lake, tropical and temperate forest, marsh, swamp, meadow, park as a representative of savanna, mountain, and river) ( Mangone et al., 2021 ). Concerning features of savanna, there is evidence on cross-cultural preferences for acacia-like Savanna trees ( Orians and Heerwagen, 1992 ). Lohr and Pearson-Mims (2006) consider people’s preference for trees with spreading cones comparable to an acacia. Sommer (1997) confirms this result within a cross-national study thus confirming the refuge dimension of Appleton’s theory as such a canopy can represent habitat and safety. Anecdotal evidence points to the fact that looking at trees outside a hospital room helps in recovering more quickly from hospital stay than patients looking at a brick wall ( Ulrich, 1984 ).
Moreover, the general question of the degree of our landscape preferences and behavior being innate versus learned during childhood is a part of a long debate. Humans have adapted to a broad range of conditions as social, cultural and natural selection unfold in tandem ( Hartig, 2021 ). Thus, landscape preferences might be determined by culture as some studies stress a relationship between childhood memories and preferences for specific environments ( Van den Berg et al., 1998 ) and others emphasize cultural determinants ( Bourassa, 1992 ; Howley, 2011 ; Joye and van den Berg, 2011 ; Ward Thompson, 2018 ; Silva et al., 2020 ; Hartig, 2021 ). Landscape preferences, explained by cultural-based elements must first explain the concept of landscape, and these discussions have a very long tradition, especially in geography ( Cosgrove, 2004 ; Wylie, 2007 ). The evolution of landscapes must be regarded as a reciprocal interplay of both ecological and cultural “factors.” Carl Sauer’s much-cited work on Sauer (1925) stresses the active agency of culture in shaping landscapes, rejecting environ mental determinism. Further, landscape is the esthetically perceived environment, therefore it affects human wellbeing. Wellbeing is regarded as an important factor in pro-environmental behavior across different cultures and countries ( Capstick et al., 2022 ) stressing the importance of human-nature bonds for environmental policy.
Due to the complexity of the concept of landscape, it must be distinguished from concepts such as place, space, or territory in a political context ( Menatti and Casado da Rocha, 2016 ). Landscape is the existentially experienced environment as a result of history, reflecting economy and society. And so are landscape preferences in this perspective.
The discussions about the different concepts of landscape are far beyond the scope of this contribution but such concepts of landscape offer the possibility of reconnection ecology, and psychology to current humanities concerns with culture, identity, meaning, and even ethics ( Menatti and Casado da Rocha, 2016 ). Environmental psychology is increasingly recognizing that human–environment interactions are culture-bound ( Tam and Milfont, 2020 ), thus discussing the cultural factors in landscape perception, preferences, and individual wellbeing.
Hartmann and Apaolaza-Ibáñez (2013) confirm the role of familiarity as there are preferences for images of lush green landscapes with water and familiar biomes (see also Mangone et al., 2021 ). Human nature attachment has been related to both evolution and cultural bonds to places, as landscape preferences are related to places where human beings feel safe and at home ( Adevi and Grahn, 2012 ). Differences in preferences for nature between demographic groups appear to be small ( Stamps, 1999 ) but differences in landscape preferences between Western students and non-Western students have been found ( Hägerhäll et al., 2018 ) or preferences for tropical forest landscapes ( Moura et al., 2018 ). Also, age-related changes in landscape preferences have been found ( Balling and Falk, 1982 ). Following from this “innate-learned debate” and the complex mix of social, cultural, and natural selection, our focus in this paper on a special preference for certain types of natural settings does not exclude or oppose potential preferences for (or evolutionary adaptation to) urban settings; neither do we maintain that preferences (= visual inclinations to prefer) for nature per se necessarily signify particular health benefits from nature (cf. Hartig, 2021 ). Although there is ample evidence that such health or wellbeing benefits do exist particularly in comparison to urban scenes ( Abraham et al., 2010 ; Hartig et al., 2014 ; Twohig-Bennett and Jones, 2018 ; Mygind et al., 2019 ) we emphasize here Ulrich’s (1983) theoretical notion that an individual’s affective reaction to a natural scene serves only as an action impulse for adaptive behavior which can be suppressed or denied, based on experience and learning.
To conclude, empirical evidence for preference for savanna-like environments is only partial and several types and features of natural environments attracting human preference have been presented. Thus, a very important and interesting observation in this respect is Domínguez-Rodrigo’s (2014) notion, based on palaeoecological evidence, that Savannas should be regarded as mosaic environments and not as open grasslands.
Several studies also show that human evolution took place in different biomes and not only in savanna environments. Hominids evolved in East Africa in an ecologically diverse setting, including grassland, savanna and different forest structures ( Kingston et al., 1994 ). But whereas the early Miocene mammalian faunas had a tropical-forest character, the Pliocene shows more faunas evolving a savanna-mosaic character ( Cerling et al., 1997 ). But as the interactions between climate change, ecology, and evolution are rather complex, more studies are needed to investigate this complex interplay ( Blumenthal et al., 2017 ).
We will further explore the possibilities of extending the Savanna Hypothesis including various environments with various structural properties, such as prospect and refuge, from an evolutionary perspective. With all the uncertainty in the reconstruction of the palaeoenvironmental conditions during hominization, the Savanna Hypothesis could be given new perspective by investigating the Pleistocene.
Beyond Savanna
In the warm periods of the Pleistocene, many of the northern hemispheric forest areas were apparently open woodlands with large open spaces, structurally somehow similar to Savannas. Based on the mega herbivore hypothesis, the forests were then under high grazing pressure from mega herbivores, i.e., large herbivores (e.g., forest elephants, forest rhinos, giant deer), which, depending on the author, are defined as weighing more than 800, 900 or 1,000 kg ( Malhi et al., 2016 ; van Valkenburgh et al., 2016 ). During the Pleistocene cold periods, however, largely open landscapes with other mega herbivores (e.g., wooly mammoths, wooly rhinoceroses, musk oxen, giant sloths) dominated. Generally, an alternation between open steppe/tundra and forested landscapes took place affecting human evolution ( Sanchez Goñi, 2020 ). Within a glacial period, warmer phases occurred in which not only tundra or steppe biomes were present, but also some patches of woodland and shrub stands leading to a complex mosaic of different plant communities. The best modern analog for this Pleistocene steppe-tundra environment is located in the Altai Mountains ( Chytrý et al., 2019 ). Figure 1 illustrates the complex interactions of mankind, climate change, the impact of megaherbivores on the landscape structures and the subjective perception of landscape.
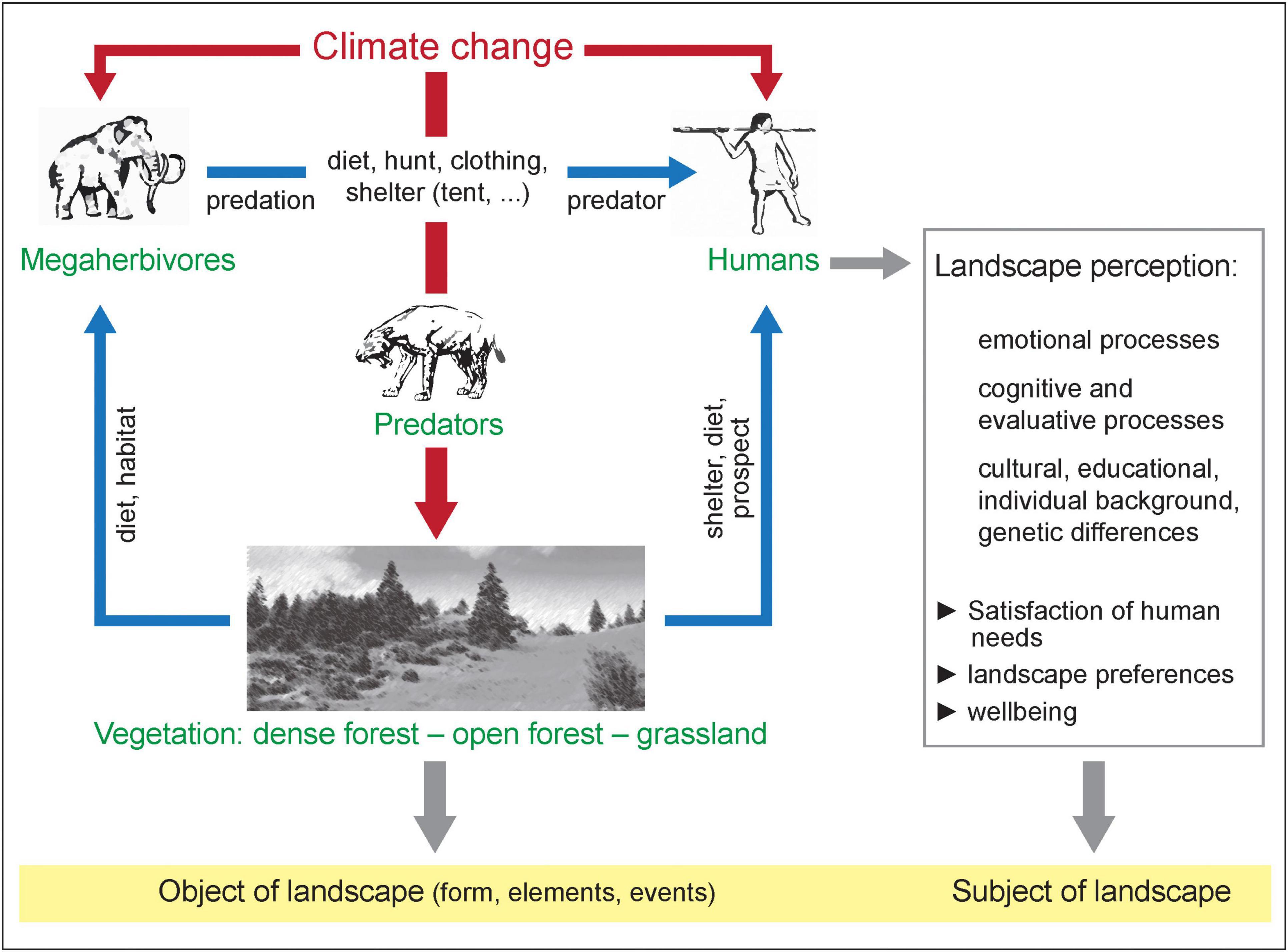
Figure 1. The simplified depiction of the interactions of mankind, climate change, the impact of mega herbivores on the landscape structures and the subjective perception of landscape. The focus is on the interactions between climate and the biosphere with human forcings affecting the environment and actively shaping their environment. Source: Winfried Weber, Institute of Geography and Geology, University of Würzburg, 2022. Reproduced with permission.
At the time of the last cold period, the early modern human settlement of southern Central Europe occurred during Marine Isotope Stage 3 in a medium-cold steppe-like environment with some boreal trees at climatically favorable sites ( Nigst et al., 2014 ). The rare palynological studies of Southern Central Europe confirm this vegetation reconstruction ( Brande, 1982 ; Müller et al., 2003 ; Stojakowits et al., 2021 ; Figure 2 ). Bielinis et al. (2018 , 2019) —based on the salutogenic landscape preference framework—have shown that even snow-covered forests and broad–leafed forests in winter can trigger positive emotions and can lead to psychological relaxation. Another study suggests lowering of blood pressure and even immune system effects of forests in winter ( Peterfalvi et al., 2021 ). Thus, we propose that Late Pleistocene environments might have partly contributed to the development of landscape preferences, although the most ancient preferences of Homo sapiens were formed in Africa during the two last glaciations (Riss and Würm) and interglacial stages (Mindel-Riss and Riss/Würm). The first traces of modern humans date to around 315,000 years ago ( Richter et al., 2017 ).
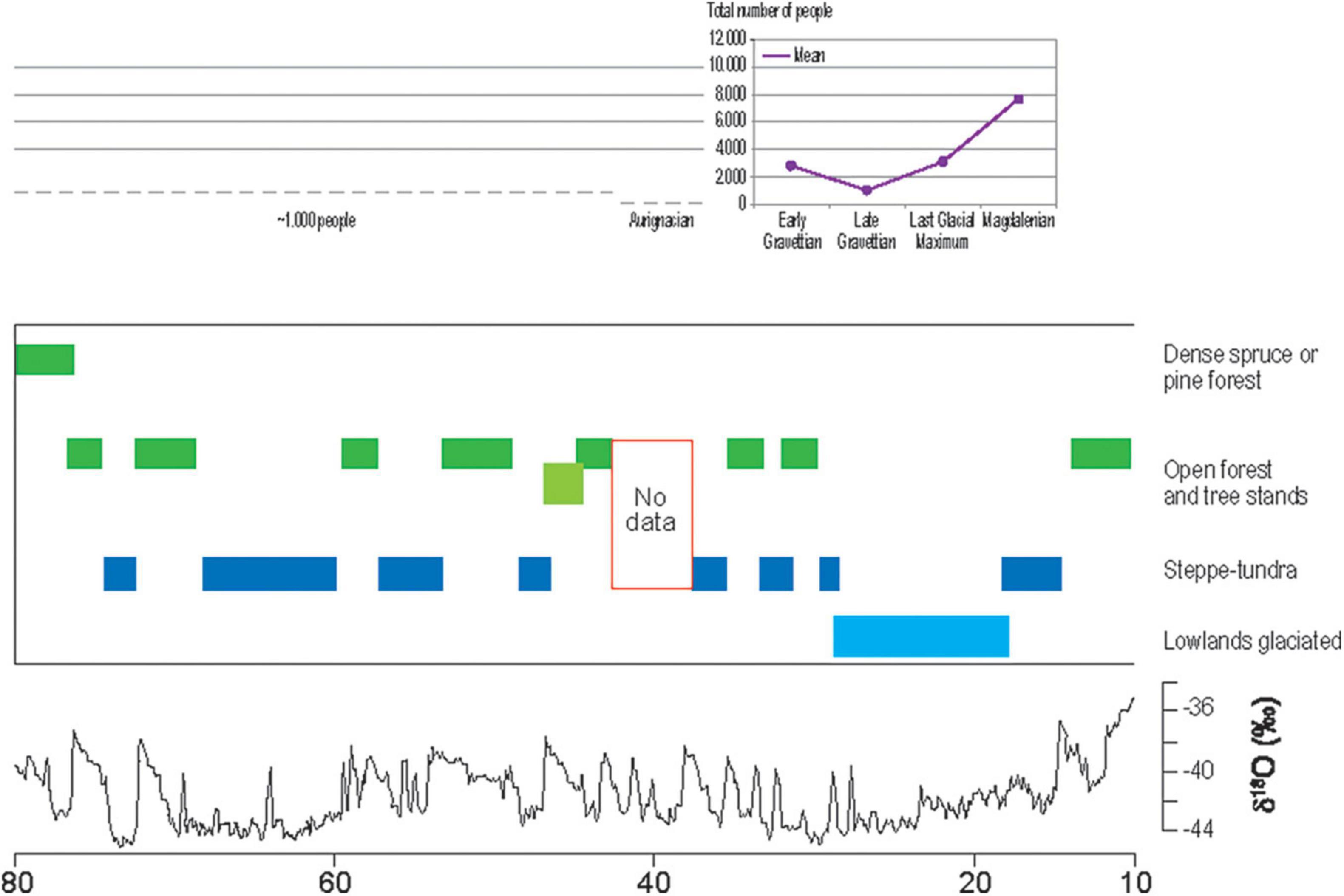
Figure 2. Vegetation and palaeoclimatic development in comparison to the estimated population from c. 80 to 10 ka (x axis). According to Heiri et al. (2014) , with additional palaeobotanical data ( Müller et al., 2003 ) and estimated total number of people for the Upper Danube region until c. 42 ka ( Müller-Beck, 1983 ), for the same region during the Aurignacian until c. 33 ka ( Schmidt and Zimmermann, 2019 ), the early Gravettian until c. 29 ka and late Gravettian until c. 25 ka ( Maier and Zimmermann, 2017 ), the LGM ( Maier et al., 2016 ), and Magdalenian ( Kretschmer, 2015 ; Maier, 2017 ). The Greenland ice core oxygen isotope record NGRIP is shown in the lower part of the figure according to Svensson et al. (2008) , supplemented by Rasmussen et al. (2014) .
According to genetic studies, modern humans likely mixed with Neanderthals ( Prüfer et al., 2014 ). Both, the early modern humans and the Neanderthals were shaped by open landscapes over many generations. After the ice retreat Mesolithic tribes lived in open landscapes until the reforestation with Betula trees took place in the Bølling Interstadial at around 14.5 ka ( Eusterhues et al., 2002 ). Later on, Pinus sylvestris also reimmigrated forming open pine-birch-forests until the onset of the Boreal at around 10.3 ka. Around 5,500 cal BC, the colonization of Neolithic tribes of the Linearbandkeramik (LBK) along the Danube started in Southern Central Europe ( Gronenborn, 2004 ). These people used to live in open grass steppe landscapes as testified by pollen analyses (e.g., Wick et al., 2003 ; Litt et al., 2009 ). They created similar kinds of open landscapes in their newly settled areas on a small scale- which were densely forested at that time—in order to practice farming. Open lands are preferred settlement regions for humans over many thousands of years dating back to the Late Pleistocene. Although wilderness seems to be more fascinating than cultural landscapes ( Barbiero and Berto, 2021 ) domestication of animals and plants during the Neolithic period might have contributed to favoring the cultural landscapes, over the wilderness landscapes.
Although the Savanna Hypothesis can be questioned in many ways, it may be extended by the integration of Pleistocene environments. Our hypothesis is, that the preference for mosaic, park-like landscapes experienced a kind of “refreshment” in the Pleistocene. We suggest a heuristic tool, as Appleton did, and not a comprehensive explanation of human behavior and perception, being aware that landscape preferences are not simply based on innate response to the environment. The extended Savanna Hypothesis, termed “Beyond-Savanna-Hypothesis” or according to our study “Pleistocene-Mosaic-Environments-Hypothesis,” might also stimulate work linking human evolution, human health, and general human-environment bond (c.f., Mangone et al., 2021 ; Chang et al., 2022 ). We propose empirical studies that would account for both genetic, environmental and developmental influences and their complex interactions on such preferences.
Data Availability Statement
The original contributions presented in the study are included in the article/supplementary material, further inquiries can be directed to the corresponding author/s.
Author Contributions
JR: basic idea, literature review, and text. KK: text on psychology. PS: text on paleoenvironments. All authors contributed to the article and approved the submitted version.
Conflict of Interest
The authors declare that the research was conducted in the absence of any commercial or financial relationships that could be construed as a potential conflict of interest.
Publisher’s Note
All claims expressed in this article are solely those of the authors and do not necessarily represent those of their affiliated organizations, or those of the publisher, the editors and the reviewers. Any product that may be evaluated in this article, or claim that may be made by its manufacturer, is not guaranteed or endorsed by the publisher.
Abraham, A., Sommerhalder, K., and Abel, T. (2010). Landscape and well-being: a scoping study on the health-promoting impact of outdoor environments. Int. J. Public Health 55, 59–69. doi: 10.1007/s00038-009-0069-z
PubMed Abstract | CrossRef Full Text | Google Scholar
Adevi, A. A., and Grahn, P. (2012). Preferences for landscapes: a matter of cultural determinants or innate reflexes that point to our evolutionary background?. Landsc. Res. 37, 27–49. doi: 10.1016/j.earlhumdev.2020.105191
Appleton, J. (1975). The Experience of Landscape . London: John Wiley.
Google Scholar
Appleton, J. (1984). Prospect and refuge re-visited. Landsc. J. 3, 91–103.
Appleton, J. (1996). The Experience of Landscape. – Revised Version. New York, NY: John Wiley & Sons Ltd.
Balling, J. D., and Falk, J. H. (1982). Development of visual preference for natural environments. Environ. Behav. 14, 5–28.
Barbiero, G., and Berto, R. (2021). Biophilia as evolutionary adaptation: an onto- and phylogenetic framework for biophilic design. Front. Psychol . 12:700709. doi: 10.3389/fpsyg.2021.700709
Bell, S. L., Foley, R., Houghton, F., Maddrell, A., and Williams, A. M. (2018). From therapeutic landscapes to healthy spaces, places and practices: a scoping review. Soc. Sci. Med. 196, 123–130. doi: 10.1016/j.socscimed.2017.11.035
Bender, R., Tobias, P. V., and Bender, N. (2012). The savannah hypotheses: origin, reception and impact on paleoanthropology. Hist. Philos. Life Sci. 34, 147–184.
Berto, R. (2005). Exposure to restorative environments helps restore attentional capacity. J. Environ. Psychol. 25, 249–259. doi: 10.1016/j.jenvp.2005.07.001
CrossRef Full Text | Google Scholar
Bielinis, E., Omelan, A., Boiko, S., and Bielinis, L. (2019). The restorative effect of staying in a broad-leaves forest on healthy young adults in Winter and Spring. Baltic For. 24, 218–227.
Bielinis, E., Takayama, N., Boiko, S., Omelan, A., and Bielinis, L. (2018). The effect of winter forest bathing on psychological relaxation of young polish adults. Urban For. Urban Green. 29, 276–283.
Blumenthal, S. A., Levin, N. E., Brown, F. H., Brugal, J. P., Chritz, K. L., Harris, J. M., et al. (2017). Aridity and hominin environments. Proc. Natl. Acad. Sci. 114, 7331–7336. doi: 10.1073/pnas.1700597114
Bourassa, S. (1992). The Aesthetics of Landscape. London: Bellhaven Press.
Brande, A. (1982). Vegetation and landscape changes at the paleolithic site of Mauern (Bavaria), early man news 5/6. Newsl. Hum. Paleoecol . 3–5.
Bratman, G. N., Hamilton, J. P., and Daily, G. C. (2012). The impacts of nature experience on human cognitive function and mental health. Ann. N. Y. Acad. Sci. 1249, 118–136. doi: 10.1111/j.1749-6632.2011.06400.x
Buss, D. M. (1995). Evolutionary psychology: a new paradigm for psychological science. Psychol. Inq. 1:1.30.
Capstick, S., Nash, N., Poortinga, W., Haggar, P., and Brügger, A. (2022). The connection between subjective wellbeing and pro-environmental behaviour: individual and cross-national characteristics in a seven-country study. Environ. Sci. Policy 133, 63–73.
Carrus, G., Scopelliti, M., Lafortezza, R., Colangelo, G., Ferrini, F., and Salbitano, F. (2015). Go greener, feel better? The positive effects of biodiversity on the well-being of individuals visiting urban and peri-urban green areas. Landsc.Urban Plan. 134, 221–228. doi: 10.1089/cyber.2013.0157
Cerling, T., Harris, J., MacFadden, B., Lea-key, M. G., Quade, J., Eh-leringer, J. R., et al. (1997). Global vegetation change through the Miocene/Pliocene boundary. Nature 389, 153–158. doi: 10.1007/s00114-008-0500-y
Chang, C.-C., Cox, D. T. C., Fan, Q., Nghiem, T. P. L., Tan, C. L. Y., Oh, R. R. Y., et al. (2022). People’s desire to be in nature and how they experience it are partially heritable. PLoS Biol. 20:e3001500. doi: 10.1371/journal.pbio.3001500
Chin, R., Chang, S. W. C., and Holmes, A. J. (2022). Beyond cortex: the evolution of the human brain. Psychol. Rev. [Online ahead of print]. doi: 10.1037/rev0000361
Chytrý, M., Horsák, M., Danihelka, J., Ermakov, N., German, D. A., Hájek, M., et al. (2019). A modern analogue of the Pleistocene steppe-tundra ecosystem in southern Siberia. Boreas 48, 36–56.
Cosgrove, D. (2004). Landscape and Landschaft. Bull. German Hist. Inst. 35, 57–71.
Domínguez-Rodrigo, M. (2014). Is the “savanna hypothesis” a dead concept for explaining the emergence of the earliest hominins? Curr. Anthropol. 55, 59–81.
Eusterhues, K., Lechterbeck, J., Schneider, J., and Wolf-Brozio, U. (2002). Late- and Post-Glacial evolution of Lake Steisslingen (I). Sedimentary history, palynological record and inorganic geochemical indicators. Palaeogeogr. Palaeoclimatol. Palaeoecol. 187, 341–371.
Falk, J. H., and Balling, J. D. (2010). Evolutionary influence on human landscape preference. Environ. Behav. 42, 479–493.
Grassini, S., Revonsuo, A., Castellotti, S., Petrizzo, I., Benedetti, V., and Koivisto, M. (2019). Processing of natural scenery is associated with lower attentional and cognitive load compared with urban ones. J. Environ. Psychol. 62, 1–11.
Grilli, G., and Sacchelli, S. (2020). Health benefits derived from forest: a review. Int. J. Environ. Res. Public Health 17:6125. doi: 10.3390/ijerph17176125
Gronenborn, D. (2004). Comparing contact-period archaeologies: the expansion of farming and pastoralist societies to continental temperate Europe and to southern Africa. Before Farm. 3, 1–35.
Hägerhäll, C. M., Ode Sang, Å, Englund, J.-E., Ahlner, F., Rybka, K., Huber, J., et al. (2018). Do humans really prefer semi-open natural landscapes? A cross-cultural reappraisal. Front. Psychol. 9:822. doi: 10.3389/fpsyg.2018.00822
Han, K.-T. (2007). Responses to six major terrestrial biomes in terms of scenic beauty, preference, and restorativeness. Environ. Behav. 39, 529–556.
Hansmann, R., Hug, S. M., and Seeland, K. (2007). Restoration and stress relief through physical activities in forests and parks. Urban For. Urban Green 6, 213–225. doi: 10.1016/j.ufug.2007.08.004
Hartig, T. (2021). “Restoration in nature: Beyond the conventional narrative,” in Nature and Psychology: Biological, Cognitive, Developmental, and Social , eds A. Schutte, J. Torquati, and J. Stevens (Cham, Switzerland: Springer International Publishing), 89–151.
Hartig, T., Mitchell, R., de Vries, S., and Frumkin, H. (2014). Nature and health. Annu. Rev. Public Health 35, 207–228.
Hartmann, P., and Apaolaza-Ibáñez, V. (2013). Desert or rain: standardisation of green advertising versus adaptation to the target audience’s natural environment. Eur. J. Mark. 47, 917–933. doi: 10.1108/03090561311308091
Heerwagen, J. H., and Orians, G. H. (1993). “Humans, habitats, and aesthetics,” in The Biophilia Hypothesis , eds S. R. Kellert and E. O. Wilson (Washington, DC: Washington Island Press), 138–172.
Heiri, O., Koinig, K. A., Spötl, C., Barrett, S. J., Brauer, A., Drescher-Schneider, R., et al. (2014). Palaeoclimate records 60–8 ka in the Austrian and Swiss Alps and their forelands. Quat. Sci. Rev. 106, 186–205.
Hill, D., and Daniel, T. C. (2007). Foundations for an ecological aesthetic: Can information alter landscape preferences? Soc. Nat. Resour. 1, 34–49. doi: 10.1080/08941920701655700
Hoffecker, J. F. (2009). The spread of modern humans in Europe. PNAS 106, 16040–16045.
Howley, P. (2011). Landscape aesthetics: assessing the general publics’ preferences towards rural landscapes. Ecol. Econ. 72, 161–169.
Ideno, Y., Hayashi, K., Abe, Y., Ueda, K., Iso, H., Noda, M., et al. (2017). Blood pressure-lowering effect of Shinrin-yoku (Forest bathing): a systematic review and meta-analysis. BMC Complement. Altern. Med. 17:409. doi: 10.1186/s12906-017-1912-z
Joye, Y., and van den Berg, A. (2011). Is love for green in our genes? A critical analysis of evolutionary assumptions in restorative environments research. Urban For. Urban Green. 10, 261–268.
Kaplan, R., and Kaplan, S. (1989). The Experience of Nature . New York, NY: Cambridge University Press.
Kingston, J. D., Marino, B. D., and Hill, A. (1994). Isotopic evidence for Neogene hominid paleoenvironments in the Kenya rift valley. Science 264, 955–959. doi: 10.1126/science.264.5161.955
Klein, R. G. (2008). Out of Africa and the evolution of human behavior. Evol. Anthropol. 17, 267–281.
Koole, S. L., and Van den Berg, A. E. (2005). Lost in the wilderness: terror management, action orientation, and nature evaluation. J. Pers. Soc. Psychol. 88, 1014–1028. doi: 10.1037/0022-3514.88.6.1014
Korpela, K. M., Klemettilä, T., and Hietanen, J. K. (2002). Evidence for rapid affective evaluation of environmental scenes. Environ. Behav. 34, 478–494. doi: 10.1007/s00426-006-0064-4
Kretschmer, I. (2015). Demographische Untersuchungen zu Bevölkerungsdichten, Mobilität und Landnutzung im späten Jungpaläolithikum. Kölner Studien zur Prähistorischen Archäologie 6. Rahden: Verlag Marie Leidorf.
Lipp, O. V., Derakshan, N., Waters, A. M., and Logies, S. (2004). Snakes and cats in the flower bed: fast detection is not specific to pictures of fear-relevant animals. Emotion 4, 233–250. doi: 10.1037/1528-3542.4.3.233
Litt, T., Krastel, S., Sturm, M., Kipfer, R., Örcen, S., Heumann, G., et al. (2009). ‘PALEOVAN’, International Continental Scientific Drilling Program (ICDP): site survey results and perspectives. Quat . Sci . Rev . 28, 1555–1567.
LoBue, V., and DeLoache, J. S. (2008). Detecting the snake in the grass: attention to fear-relevant stimuli by adults and young children. Psychol. Sci. 19, 284–289.
Lohr, V. I., and Pearson-Mims, C. H. (2006). Responses to scenes with spreading, rounded and conical tree forms. Environ. Behav. 38, 667–688.
Maier, A. (2017). Population and settlement dynamics from the gravettian to the magdalenian. Mitteilungen Gesellschaft Urgeschichte 26, 83–101.
Maier, A., Lehmkuhl, F., Ludwig, P., Melles, M., Schmidt, I., Shao, Y., et al. (2016). Demographic estimates of hunter-gatherers during the Last Glacial Maximum in Europe against the background of palaeoenvironmental data. Quat. Int. 425, 49–61.
Maier, A., and Zimmermann, A. (2017). Populations headed south? The Gravettian from a palaeodemographicpoint of view. Antiquity 91, 573–588.
Malhi, Y., Doughty, C. E., Galetti, M., Smith, F. A., Svenning, J. C., and Terborgh, J. W. (2016). Megafauna and ecosystem function from the Pleistocene to the Anthropocene. PNAS 113, 838–846. doi: 10.1073/pnas.1502540113
Mangone, G., Dopko, R. L., and Zelenski, J. M. (2021). Deciphering landscape preferences: investigating the roles of familiarity and biome types. Landsc. Urban Plann. 214:104189.
McMahan, E. A., and Estes, D. (2015). The effect of contact with natural environments on positive and negative affect: a meta-analysis. J. Posit. Psychol. 10, 507–519. doi: 10.1080/17439760.2014.994224
Menatti, L., and Casado da Rocha, A. (2016). Landscape and health: connecting psychology, aesthetics, and philosophy through the concept of Affordance . Front. Psychol. 7:571. doi: 10.3389/fpsyg.2016.00571
Moura, J. M. B., Ferreira Júnior, W. S., Silva, T. C., and Albuquerque, U. P. (2018). The influence of the evolutionary past on the mind: an analysis of the preference for landscapes in the human species. Front. Psychol . 9:2485. doi: 10.3389/fpsyg.2018.02485
Müller, U. C., Pross, J., and Bibus, E. (2003). Vegetation response to rapid climate change in Central Europe during the past 140,000 yr based on evidence from the Füramoos pollen record. Quat. Res. 59, 235–245.
Müller, U. C., Pross, J., Tzedakis, P. C., Gamble, C., Kotthoff, U., Schmiedl, G., et al. (2011). The role of climate of climate in the spread of modern humans into Europe. Quat. Sci. Rev. 30, 273–279.
Müller-Beck, H. (1983). “Sammlerinnen und Jäger von den Anfängen bis vor 35000 Jahren,” in Urgeschichte Baden-Württembergs , ed. H. Müller-Beck (Germany: Stuttgart), 214–272.
Mygind, L., Kjeldsted, E., Hartmeyer, R., Mygind, E., Stevenson, M. P., Quintana, D., et al. (2019). Effects of public green space on acute psychophysiological stress response: a systematic review and meta-analysis of the experimental and quasi-experimental evidence. Environ. Behav. 53, 184–226. doi: 10.1177/0013916519873376
Nigst, P. R., Haesaerts, P., Damblon, F., Frank-Fellner, C., Mallol, C., Viola, B., et al. (2014). Early modern human settlement of Europe north of the Alps occurred 43,500 years ago in a cold steppe-type environment. PNAS 111, 14394–14399. doi: 10.1073/pnas.1412201111
Norwood, M. F., Lakhani, A., Maujean, A., Zeeman, H., Olivia Creux, O., and Kendall, E. (2019). Brain activity, underlying mood and the environment: a systematic review. J. Environ. Psychol. 65:101321.
Orians, G. (1980). “Habitat selection: general theory and applications to human behavior,” in The Evolution of Human Social Behavior , ed. J. S. Lockard (Chicago, IL: Elsevier), 49–66.
Orians, G., and Heerwagen, J. H. (1992). “Evolved responses to landscapes,” in The Adapted Mind: Evolutionary Psychology and the Generation of Culture , eds J. H. Barkow, L. Cosmides, and J. Tooby (New York, NY: Oxford University Press), 555–579.
Öhman, A., Flykt, A., and Esteves, F. (2001). Emotion drives attention: detecting the snake in the grass. J. Exp. Psychol. 130, 466–478. doi: 10.1037/0096-3445.130.3.466
Öhman, A., and Mineka, S. (2001). Fears, phobias, and preparedness: toward an evolved module of fear and fear learning. Psychol. Rev. 108, 483–522. doi: 10.1037/0033-295X.108.3.483
Park, B. J., Tsunetsugu, Y., Kasetani, T., Kagawa, T., and Miyazaki, Y. (2010). The physiological effects of Shinrin-yoku (taking in the forest atmosphere or forest bathing): evidence from field experiments in 24 forests across Japan. EHPM 15, 18–26. doi: 10.1007/s12199-009-0086-9
Peterfalvi, A., Meggyes, M., Makszin, L., Farkas, N., Miko, E., Miseta, A., et al. (2021). Forest bathing always makes sense: blood pressure-lowering and immune system-balancing effects in late spring and winter in central Europe. Int. J. Environ. Res. Public Health 18:2067. doi: 10.3390/ijerph18042067
Prüfer, K., Racimo, F., Patterson, N., Jay, F., Sankararaman, S., Sawyer, S., et al. (2014). The complete genome sequence of a Neanderthal from the Altai Mountains. Nature 505, 43–49. doi: 10.1038/nature12886
Rasmussen, S. O., Bigler, M., Blockley, S., Blunier, T., Buchardt, S. L., Clausen, H., et al. (2014). A stratigraphic framework for abrupt climatic changes during the Last Glacial period based on three synchronized Greenland ice-core records: refining and extending the INTIMATE event stratigraphy. Quat. Sci. Rev. 106, 14–28.
Rathmann, J. (2021). Therapeutic Landscapes. Landscape and Health in an Interdisciplinary Perspective. Wiesbaden: Springer.
Rathmann, J., Beck, C., Flutura, S., Seiderer, A., Aslan, I., and André, E. (2020). Towards quantifying forest recreation: exploring outdoor thermal physiology and human well-being along exemplary pathways in a central European urban forest (Augsburg, SE-Germany). Urban For. Urban Green. 49:126622.
Richerson, P. J., Boyd, R., and Bettinger, R. L. (2009). Cultural innovations and demographic change. Hum. Biol. 81, 211–235. doi: 10.3378/027.081.0306
Richmond, B. G., Begun, D. R., and Strait, D. S. (2001). The origin of human bipedalism: the knuckle-walking hypothesis revisited. Yearb. Phys. Anthropol . 116, 70–105. doi: 10.1002/ajpa.10019.abs
Richter, D., Grün, R., Joannes-Boyau, R., Steele, T. E., Amani, F., Rué, M., et al. (2017). The age of the hominin fossils from Jebel Irhoud, Morocco, and the origins of the Middle Stone Age. Nature 546, 293–296. doi: 10.1038/nature22335
Sanchez Goñi, F. (2020). Regional impacts of climate change and its relevance to human evolution. Evol. Hum. Sci . 2:e55.
Sauer, C. (1925). The Morphology of Landscape. Berkeley, CA: University of California Press.
Schmidt, I., and Zimmermann, A. (2019). Population dynamics and socio-spatial organization of the Aurignacian: scalable quantitative demographic data for western and central Europe. PLoS One 14:e0211562. doi: 10.1371/journal.pone.0211562
Shennan, S. (2001). Demography and cultural innovation: a model and its implications for the emergence of modern human culture. Camb. Archaeol. J. 11, 5–16.
Silva, R. H., Ferreira Júnior, W. S., Moura, J. M. B., and Albuquerque, U. P. (2020). The link between adaptive memory and cultural attraction: new insights for evolutionary ethnobiology. Evol. Biol. 47, 273–284.
Sommer, R. (1997). Further cross-national studies of tree form preference. Ecol. Psychol. 2, 153–160. doi: 10.1207/s15326969eco0902_3
Staats, H., Kievet, A., and Hartig, T. (2003). Where to recover from attentional fatigue: an expectancy-value analysis of environmental preference. J. Environ.Psychol. 23, 147–157. doi: 10.1016/S0272-4944(02)00112-3
Stamps, I. I. I. A. E. (1999). Demographic effects in environmental aesthetics: a meta-analysis. J. Plann. Lit. 14, 155–175.
Staubwasser, M., Dr ǎ gusin, V., Onac, B. P., Assonov, S., Ersek, V., Hoffmann, D. L., et al. (2018). Impact of climate change on the transition of Neanderthals to modern humans in Europe. PNAS 115, 9116–9121. doi: 10.1073/pnas.1808647115
Stojakowits, P., Mayr, C., Ivy-Ochs, S., Preusser, F., Reitner, J., and Spötl, C. (2021). Environments at the MIS 3/2 transition in the northern Alps and their foreland. Quat. Int . 58, 99–113.
Svensson, A., Andersen, K. K., Bigler, M., Clausen, H. B., Dahl-Jensen, D., Davies, S. M., et al. (2008). A 60 000 year Greenland stratigraphic ice core chronology. Clim. Past. 4, 47–57.
Tam, K.-P., and Milfont, T. L. (2020). Towards cross-cultural environmental psychology: a state-of-the-art review and recommendations. J. Environ. Psychol. 71:101474.
Twohig-Bennett, C., and Jones, A. (2018). Health benefits of the great outdoors: a systematic review and meta-analysis of greenspace exposure and health outcomes. Environ. Res. 166, 628–637. doi: 10.1016/j.envres.2018.06.030
Ulrich, R. S. (1983). “Aesthetic and affective response to natural environment,” in Human behavior and Environment: Advances in Theory and Research , Vol. 6, eds I. Altman and J. F. Wohlwill (New York, NY: Plenum Press), 85–125. doi: 10.3389/fnhum.2021.676032
Ulrich, R. S. (1984). View through a window may influence recovery from surgery. Science 224, 420–421. doi: 10.1126/science.6143402
Ulrich, R. S. (1993). “Biophilia, biophobia, and natural landscapes,” in The Biophilia Hypothesis , eds S. R. Kellert and E. O. Wilson (Washington, DC: Washington Island Press), 73–137. doi: 10.3389/fpsyg.2020.00511
Ulrich, R. S., Simons, R. F., Losito, B. D., Fiorito, E., Miles, M. A., and Zelson, M. (1991). Stress recovery during exposure to natural and urban environments. J. Environ. Psychol. 11, 201–230.
Van den Berg, A. E., Koole, S. L., and Van der Wulp, N. Y. (2003). Environmental preference and restoration: (How) are they related. J. Environ. Psychol. 23, 135–146.
Van den Berg, A. E., Vlek, C. A. J., and Coeterier, J. F. (1998). Group differences in the aesthetic evaluation of nature development plans: a multi-level approach. J. Environ. Psychol. 18, 141–157.
van Ginneken, V. (2019). Multiple ‘genetic bottleneck theory’ of humans and the house mouse via chilling enzyme Δ12-desaturase. Gastroenterol. Hepatol. Int. J. 4:000156
van Ginneken, V., Van Meerveld, A., Wijgerde, T., Verheij, E., de Vries, E., and Van der Greef, J. (2017). Hunter-prey correlation between migration routes of African buffaloes and early hominids: evidence for the “Out of Africa” hypothesis. Integr. Mol. Med. 4, 1–5.
van Valkenburgh, B., Hayward, M. W., Ripple, W. J., Meloro, C., and Roth, V. L. (2016). The impact of large terrestrial carnivores on Pleistocene ecosystems. PNAS 113, 862–867. doi: 10.1073/pnas.1502554112
Ward Thompson, C. (2018). “Landscape perception and environmental psychology,” in The Routledge Companion to Landscape Studies , eds P. Howard, I. Thompson, E. Waterton, and M. Atha (London: Routledge), 19–38.
Wick, L., Lemcke, G., and Sturm, M. (2003). Evidence of Lateglacial and Holocene climatic change and human impact in eastern Anatolia: high-resolution pollen, charcoal, isotopic and geochemical records from the laminated sediments of Lake Van, Turkey. Holocene 13, 665–675.
Wilson, E. O. (1984). Biophilia. Cambridge: Harvard University Press.
Wylie, J. (2007). Landscape. London: Routledge.
Keywords : savanna hypothesis, landscape preferences, human health-environment relationships, paleoanthropology, therapeutic landscapes
Citation: Rathmann J, Korpela KM and Stojakowits P (2022) Pleistocene Hypothesis – Moving Savanna Perceptual Preference Hypothesis Beyond Savanna. Front. Psychol. 13:901799. doi: 10.3389/fpsyg.2022.901799
Received: 22 March 2022; Accepted: 09 May 2022; Published: 30 May 2022.
Reviewed by:
Copyright © 2022 Rathmann, Korpela and Stojakowits. This is an open-access article distributed under the terms of the Creative Commons Attribution License (CC BY) . The use, distribution or reproduction in other forums is permitted, provided the original author(s) and the copyright owner(s) are credited and that the original publication in this journal is cited, in accordance with accepted academic practice. No use, distribution or reproduction is permitted which does not comply with these terms.
*Correspondence: Joachim Rathmann, [email protected]
Disclaimer: All claims expressed in this article are solely those of the authors and do not necessarily represent those of their affiliated organizations, or those of the publisher, the editors and the reviewers. Any product that may be evaluated in this article or claim that may be made by its manufacturer is not guaranteed or endorsed by the publisher.
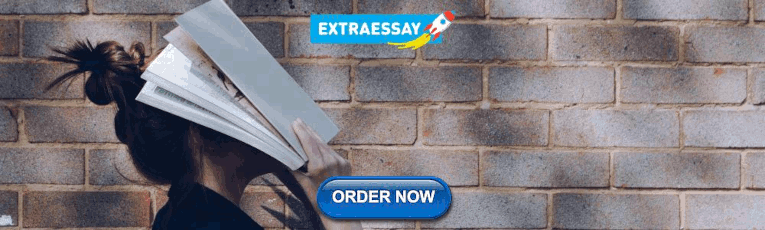
COMMENTS
Summary. A lot of information reaches the eye, but much is lost by the time it reaches the brain (Gregory estimates about 90% is lost). Therefore, the brain has to guess what a person sees based on past experiences. We actively construct our perception of reality. Richard Gregory proposed that perception involves a lot of hypothesis testing to ...
pattern perception: ability to discriminate among different figures and shapes. perceptual hypothesis: educated guess used to interpret sensory information. principle of closure: organize perceptions into complete objects rather than as a series of parts. proximity: things that are close to one another tend to be grouped together
Gustatory perception: awareness of flavor and taste on the tongue. Olfactory perception: smelling via the nose. Tactile perception: awareness of sensation on the skin. Vestibular sense: perception of balance and motion or physical energy. Proprioception: perceptual context of the body's position in space.
Perception refers to the way sensory information is organized, interpreted, and consciously experienced. Perception involves both bottom-up and top-down processing. Bottom-up processing refers to the fact that perceptions are built from sensory input. On the other hand, how we interpret those sensations is influenced by our available knowledge ...
The direct perception hypothesis: perceiving the intention of another's action hinders its precise imitation ... 3 School of Psychology, ... that social understanding is a direct perceptual achievement in most normal situations is thereby excluded by definition. This limited view of perceptual experience has important implications for how ...
If perception corresponds to hypothesis testing ( Gregory, 1980 ); then visual searches might be construed as experiments that generate sensory data. In this work, we explore the idea that saccadic eye movements are optimal experiments, in which data are gathered to test hypotheses or beliefs about how those data are caused.
Abstract. Philosophers concerned with perception traditionally consider phenomena of perception which may readily be verified by individual observation and a minimum of apparatus. Experimental psychologists and physiologists, on the other hand, tend to use elaborate experimental apparatus and sophisticated techniques, so that individual ...
The Perceptual Hypothesis opposes Inferentialism, which is the view that our knowledge of others' mental features is always inferential. The claim that some mental features are embodied is the claim that some mental features are realised by states or processes that extend beyond the brain. The view I discuss here is that the Perceptual ...
Perceptual psychology is a subfield of cognitive psychology that concerns the conscious and unconscious innate aspects of the human cognitive system: perception. A pioneer of the field was James J. Gibson. One major study was that of affordances, i.e. the perceived utility of objects in, or features of, one's surroundings. According to Gibson ...
The Perceptual Hypothesis opposes Inferentialism, which is the view that our knowledge of others' mental features is always inferential. The claim that some mental features are embodied is the claim that some mental features are realised by states or processes that extend beyond the brain. The view I discuss here is that the Perceptual ...
The simplicity principle, traditionally referred to as Occam's razor, is the idea that simpler explanations of observations should be preferred to more complex ones. In recent decades the principle has been clarified via the incorporation of modern notions of computation and probability, allowing a more precise understanding of how exactly ...
A perceptual set is a good example of what is known as top-down processing. In top-down processing, perceptions begin with the most general and move toward the more specific. Such perceptions are heavily influenced by context, expectations, and prior knowledge. If we expect something to appear in a certain way, we are more likely to perceive it ...
perception, in humans, the process whereby sensory stimulation is translated into organized experience. That experience, or percept, is the joint product of the stimulation and of the process itself. Relations found between various types of stimulation (e.g., light waves and sound waves) and their associated percepts suggest inferences that can ...
pattern perception: ability to discriminate among different figures and shapes perceptual hypothesis: educated guess used to interpret sensory information principle of closure: organize perceptions into complete objects rather than as a series of parts proximity: things that are close to one another tend to be grouped together
perception involves testing hypotheses, and that once a hypothesis is established it may prevent or delay the acceptance of an alternative hypothesis. This interpreta tion thus attributes the negative effect of prior exper-This research was supported by a grant to the senior author
Contemporary perceptual psychology uses Bayesian decision theory to develop Helmholtz's view that perception involves 'unconscious inference'. The science provides mathematically rigorous, empirically well-confirmed explanations for diverse perceptual constancies and illusions. The explanations assign a central role to mental representation.
According to Gibson, perceptual learning is " [a]ny relatively permanent and consistent change in the perception of a stimulus array, following practice or experience with this array…" (1963: 29). [ 1] Gibson's definition has three basic parts. First, perceptual learning is long-lasting. Second, it is perceptual.
To understand perception, the signal codes and the stored knowledge or assumptions used for deriving perceptual hypotheses must be discovered. Systematic perceptual errors are important clues for appreciating signal channel limitations, and for discovering hypothesis-generating procedures.
perceptual cycle hypothesis By N., Sam M.S. the theory that cognition impacts perceptual exploration but is thereby changed by real-world encounters, cultivating a cycle of attention , cognition, comprehension, and the authentic world wherein each impacts the others.
In this paper, I advance a new hypothesis about what the ordinary concept of perceptual experience might be. To a first approximation, my hypothesis is that it is the concept of something that seems to present mind-independent objects. Along the way, I reveal two important errors in Michael Martin's argument for the very different view that the ordinary concept of perceptual experience is ...
The Perceptual Hypothesis opposes Inferentialism, which is the view that our knowledge of others' mental features is always inferential. The claim that some mental features are embodied is the claim that some mental features are realised by states or processes that extend beyond the brain. The view I discuss here is that the Perceptual ...
1 Institute of Geography, Augsburg University, Augsburg, Germany; 2 Faculty of Social Sciences/Psychology, Tampere University, Tampere, Finland; We provide an extension of the Savanna perceptual preference hypothesis ("Savanna Hypothesis"), supposing that interaction with landscapes offering survival advantage for human groups during evolution might have gradually evolved to permanent ...