- Architecture and Design
- Asian and Pacific Studies
- Business and Economics
- Classical and Ancient Near Eastern Studies
- Computer Sciences
- Cultural Studies
- Engineering
- General Interest
- Geosciences
- Industrial Chemistry
- Islamic and Middle Eastern Studies
- Jewish Studies
- Library and Information Science, Book Studies
- Life Sciences
- Linguistics and Semiotics
- Literary Studies
- Materials Sciences
- Mathematics
- Social Sciences
- Sports and Recreation
- Theology and Religion
- Publish your article
- The role of authors
- Promoting your article
- Abstracting & indexing
- Publishing Ethics
- Why publish with De Gruyter
- How to publish with De Gruyter
- Our book series
- Our subject areas
- Your digital product at De Gruyter
- Contribute to our reference works
- Product information
- Tools & resources
- Product Information
- Promotional Materials
- Orders and Inquiries
- FAQ for Library Suppliers and Book Sellers
- Repository Policy
- Free access policy
- Open Access agreements
- Database portals
- For Authors
- Customer service
- People + Culture
- Journal Management
- How to join us
- Working at De Gruyter
- Mission & Vision
- De Gruyter Foundation
- De Gruyter Ebound
- Our Responsibility
- Partner publishers

Your purchase has been completed. Your documents are now available to view.
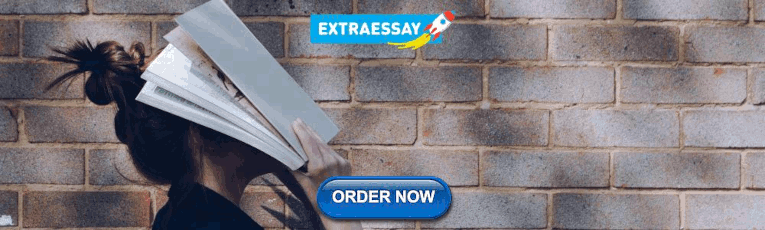
Linguistic Analysis
From data to theory.
- Annarita Puglielli and Mara Frascarelli
- X / Twitter
Please login or register with De Gruyter to order this product.
- Language: English
- Publisher: De Gruyter Mouton
- Copyright year: 2011
- Audience: Researchers, Scholars and Advanced Students of Linguistics concerned with Formal Analysis in a Typological, Comparative Perspective
- Front matter: 8
- Main content: 404
- Published: March 29, 2011
- ISBN: 9783110222517
- Published: March 17, 2011
- ISBN: 9783110222500
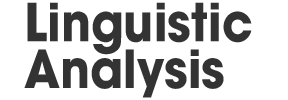
Welcome to Linguistic Analysis
A peer-reviewed research journal publishing articles in formal phonology, morphology, syntax and semantics. The journal has been in continuous publication since 1976. ISSN: 0098-9053
Please note that Volumes , Issues , Individual Articles , as well as a yearly Unlimited Access Pass (via IP Authentication or Username-and-Password ) to Linguistic Analysis are now available here for purchase and for download on this website. For more information on rates and ordering options, please visit the Rates page. We will continue to add new material so come back to visit. Please Contact us if you are interested in specific back issues.
Current Issue
Linguistic Analysis Volume 43 Issues 1 & 2 (2022)
Barcelona Conference on Syntax, Semantics, & Phonology , edited by Anna Paradis & Lorena Castillo-Ros.
This issue brings together a selection of ten papers presented at the 15th Workshop on Syntax, Semantics, and Phonology (WoSSP), held at the Universitat Autònoma de Barcelona, on June 28-29, 2018. WoSSP is a series of on-going workshops organized by PhD students for students who are working in any domain of generative linguistics, and which offers them a forum to share their work in progress . One of the main aims of the WoSSP conference is to provide a space where graduate students who wish to present their work may exchange ideas within different formal approaches to linguistic phenomena.
Read the Introduction
Issues in Preparation
Volume 43, 3-4: Dependency Grammars
This issue, edited by Timothy Osborne, brings together a selection of papers that examine dependency grammars from a variety of perspectives.
Volume 44, 1-2 Pot-pourri
A selection of orthodox and alternate linguistic perspectives, including an in-depth examination of phonology in classical Arabic poetry, and 3 article-length studies of English grammar by Michael Menaugh.
Note: Volume 43, 3-4, will be the last issue of the journal published in paper. Beginning with volume 44, 1-2, all issues will be available in electronic form only on this website <www.linguisticanalysis.com>. Interested parties will be able to purchase single articles, whole issues, or take advantage of the annual All-Access pass to everything.
Note: We are also uploading all past volumes and issues of the journal and expect this process to be completed by the end of 2023.
Thank you for your patience and continued support.
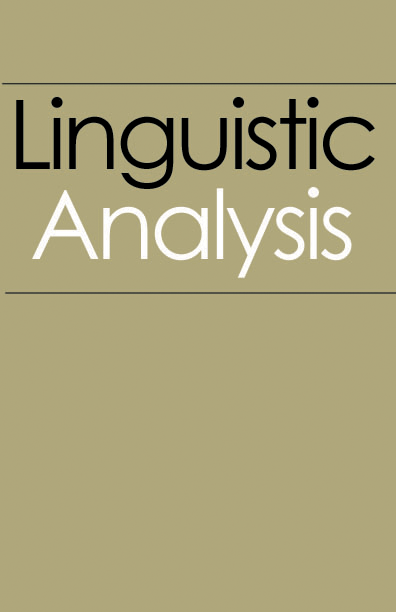
Thank you for visiting nature.com. You are using a browser version with limited support for CSS. To obtain the best experience, we recommend you use a more up to date browser (or turn off compatibility mode in Internet Explorer). In the meantime, to ensure continued support, we are displaying the site without styles and JavaScript.
- View all journals
- My Account Login
- Explore content
- About the journal
- Publish with us
- Sign up for alerts
- Open access
- Published: 26 February 2024
Prosody in linguistic journals: a bibliometric analysis
- Mengzhu Yan 1 &
- Xue Wu ORCID: orcid.org/0000-0001-6454-4208 1
Humanities and Social Sciences Communications volume 11 , Article number: 311 ( 2024 ) Cite this article
1324 Accesses
1 Altmetric
Metrics details
- Language and linguistics
The present study provides a systematic review of prosody research in linguistic journals through a bibliometric analysis. Using the bibliographic data from 2001 to 2021 in key linguistic journals that publish prosody-related research, this study adopted co-citation analysis and keyword analysis to investigate the state of the intellectual structure and the emerging trends of research on prosody in linguistics over the past 21 years. Additionally, this study identified the highly cited authors, articles and journals in the field of prosody. The results offer a better understanding of how research in this area has evolved and where the boundaries of prosody research might be pushed in the future.
Similar content being viewed by others
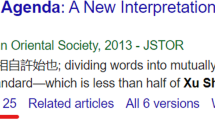
A systematic synthesis and analysis of English-language Shuōwén scholarship
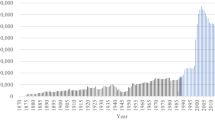
Numbers of articles in the three Japanese national newspapers, 1872–2021
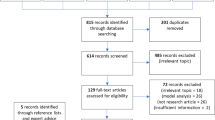
A systematic and interdisciplinary review of mathematical models of language competition
Introduction.
Prosody, often referred to as the music of speech, is defined as the organizational structure of speech, including linguistic functions such as tone, intonation, stress, and rhythm (Gussenhoven and Chen, 2020 ; Ladd, 2008). It has been well-established that prosody plays a key role in sentence processing in both L1 (first or native language) and L2 (second or non-native languages), including lexical activation and segmentation (e.g., Cutler and Butterfield, 1992 ; Cutler and Norris, 1988 ; Norris et al., 2006 ; Sanders et al., 2002 ), syntactic parsing (e.g., Cole et al., 2010a ; Frazier et al., 2006 ; Hwang and Schafer, 2006 ; Ip and Cutler, 2022 ; Lee and Watson, 2011 ; O’Brien et al., 2014 ; Roncaglia-Denissen et al., 2014 ; Schafer et al., 2000 ), information structure marking (e.g., Birch and Clifton, 1995 ; Breen et al., 2010 ; Calhoun, 2010 ; Clopper and Tonhauser, 2013 ; Katz and Selkirk, 2011 ; Kügler and Calhoun, 2020 ; Namjoshi and Tremblay, 2014 ; Steedman, 2000 ; Welby, 2003 ; Xu, 1999 ), and pragmatic information signaling such as speech attitudes, acts and emotion (e.g., Braun et al., 2019 ; Lin et al., 2020 ; Pell et al., 2011 ; Prieto, 2015 ; Repp, 2020 ).
Prosody has been investigated extensively given its significant status in language processing and its highly interdisciplinary nature involving linguistics, psychology, cognitive science, and computer science, especially with the advent of two early reviews: Shattuck-Hufnagel and Turk ( 1996 ) and Cutler et al. ( 1997 ). A decade later, more publications have provided comprehensive and state-of-the-art overviews of the theoretical and experimental advances of prosody (Cole, 2015 ; Wagner and Watson, 2010 ). However, to be best of our knowledge, no bibliometric overview of prosody research has been conducted to offer a better understanding of how research in this area has evolved and where the boundaries of prosody research might be pushed in the future.
The present study used a bibliometric approach which was initially used in library and information sciences for the analysis and classification of bibliographic material by sketching representative summaries of the extant literature (Broadus, 1987 ; Pritchard, 1969 ). Based on a large volume of bibliometric information, mathematical and statistical methods in bibliometric analysis make it possible to extract patterns that reveal the characteristics of publications in a specific discipline. In addition, with the assistance of network mapping techniques, bibliometric analysis can also be used to visualize the state of the intellectual structure of a specific research topic or field. In this study, we have used such an approach to perform co-citation analysis and keyword analysis to review publications on prosody in linguistic journals. Co-citation is a measure that gauges the connection between frequently referenced documents, with the intensity of co-citation determined by the frequency at which two documents have been jointly cited (Small and Sweeney, 1985 ). Co-citation analysis is important in bibliometric studies as “co-citation identifies relationships between papers which are regarded as important by authors in the specialty, but which are not identified by such techniques as bibliographic coupling or direct citation” (Small and Sweeney, 1985 , p. 19). Keyword analysis involves comparing the frequency of keywords in different periods, to identify significant changes to the key topics which is helpful in predicting the emerging trends of a research field (e.g., Lee, 2023 ; Lei and Liu, 2019a ; Zhang, 2019 ).
Bibliometric analysis has been widely used in different areas of linguistics. For instance, Zhang ( 2019 ) used this method to examine the field of second language acquisition (SLA); Lei and Liu ( 2019a ) rendered a bibliometric analysis of the 2005–2016 research trends in the field of applied linguistics; and Fu et al. ( 2021 ) employed this approach to analyze the evolution of the visual world recognition literature between 1999 and 2018. Since no bibliometric analysis has been conducted on prosody in linguistics, this present study takes the bibliometric approach to describe the state of the intellectual structure and the emerging trends of research on prosody in linguistics. The following research questions are addressed:
What is the research productivity of linguistic journals on prosody?
What is the intellectual structure in the field of prosody in terms of influential authors, references, and venues of publications?
What are the research trends of works on prosody in linguistics?
Methodology
The bibliometric data used in this study were retrieved from Web of Science (henceforth WoS) on 14 June 2022. There are three reasons why we used the database of WoS. First, WoS is a more widely used library resource than other databases such as Scopus and Google Scholar. For instance, the number of subscribers of WoS is two times larger than that of Scopus (Zhang, 2019 ). Second, only academic citations are provided by WoS. That is, compared to databases such as Google Scholar which provides mixed information of both academic and non-academic citations, WoS is more appropriate for calculating the scholarly values of the publications. Third, the availability of co-citation information in WoS makes it possible to conduct co-citation analysis which is one of the important bibliometric methods used in this study. As for the search terms, the present study used a combination of “prosod*” (In regular expressions, the asterisk (*) is used as a quantifier that specifies “zero or more” occurrences of the preceding character or group.), “autosegmental-metrical”, “metrical structure”, “accent”, “intonation*”, “stress”, “suprasegment*”, “F0” (fundamental frequency), “rhythm”, and “pitch” as the search query, and the Boolean OR operator was used to separate these terms. Moreover, the Boolean NOT operator was used to exclude research on “semantic prosody” Footnote 1 . The timeframe for the search was from January 2001 to December 2021.
Since the present study focuses on prosody research in linguistic journals, English research articles (excluding book reviews, editorial reviews, etc.) published in high-quality journals in the field of linguistics were included. Only published research articles were included to guarantee the quality and reliability of the publications under a strict quality control mechanism such as peer review (Zhu and Lei, 2021 ). As for the selection of high-quality journals, the current study chose SSCI-indexed Footnote 2 international journals in the field of linguistics for two reasons. First, those journals have rigorous peer-review processes. Second, most SSCI-indexed journals are accessible to worldwide academia. More than 200 SSCI-indexed journals in the field of linguistics have published research articles on prosody. However, some of those journals published less than three prosody-related articles in the past 21 years. A cut-off point of 30 articles per journal in the past 21 years was set to ensure that the majority of linguistic journals that published research on prosody are included for analysis in the current study. The cut-off point of 30 was set for two reasons. First, with this criterion, publications in the included journals cover more than 70% of the total publications. Another rationale behind our initial choice of 30 papers (as a rule of thumb) was to ensure a sufficient number of data points for robust statistical analysis and to focus on journals with a more substantial presence in the field of prosody. The list of journals included for and excluded from analysis in the present study can be found in the supplementary data.
Data cleaning
To avoid coding errors, data cleaning was performed using the measures proposed by Zhang ( 2019 ). Specifically, if different author names were used to refer to the same author, they were recoded to one unique version. For instance, “Elisabeth O. Selkirk”, “Selkirk, E. O.”, “Selkirk, EO”, “Selkirk, E.”, and “Selkirk, E” were all recoded as “Selkirk, E.”. Similarly, different keywords used to refer to the same concept were also recoded. For instance, “Event-related Potential”, “Event-related Potential (ERP)”, and “ERP” were all recoded as “Event-related Potential”. In addition, singular and plural forms of the same concept were identified and recoded as one. For instance, “boundary tone” and “boundary tones” referred to the same concept, hence all “boundary tones” were recoded to “boundary tone”. However, keywords that share a degree of similarity were not recoded as the same since their meanings can be different. For instance, “bilinguals” and “bilingualism” were kept as separate words since the former refers to people who speak more than two languages while the second exists as an ability of people or a characteristic of a community of people.
Data analysis
In this study, co-citation analysis and keyword analysis were performed. The data which spanned 21 years were divided into three periods (i.e. the 2001–2007 period, the 2008–2014 period, and the 2015–2021 period) and the results of the two forms of bibliometric analyses in the three periods are compared with each other to reveal important changes during the last 21 years.
Co-citation analysis assumes that if publications were frequently cited together, these publications would probably share similar themes (Hjørland, 2013 ). This technique is frequently used in previous bibliometric studies to reveal the intellectual structure in a particular research field (Rossetto et al., 2018 ; Zhang, 2019 ). Based on the references (i.e. papers that are cited by publications retrieved for the present study) in the surveyed articles, the co-citation network will cluster two publications together if they are co-cited by a third publication. The greatest strength of co-citation analysis is that apart from identifying the most influential authors, references, and venues of publications, it is also capable of discovering thematic clusters. It should be noted that clusters in the present bibliometric study are groups or sets of closely related items. Co-cited items will fall into the same cluster by using cluster techniques in VOS viewer (detailed information can be found in van Eck and Waltman, 2010 ).
The prosody-related articles published in linguistic journals between 2000 and 2021 cited more than 50,000 unique references. It would be impossible to interpret such a massive number of nodes if all the cited references were included in a network map. Therefore, when constructing the network maps in VOS viewer (van Eck and Waltman, 2017 ), we set a cutoff point at the values that could include the top 50 most-cited items in the maps in the present study to restrict the number of nodes following Zhang ( 2019 ).
Keyword analysis was used to identify important topics in publications retrieved for the present study in each period, and a cross-period comparison of the frequencies of those important topics was conducted to determine whether significant diachronic changes existed or not. The following four steps were used to conduct keyword analysis. First, author-supplied keywords and keywords-from-abstracts Footnote 3 were retrieved from each article. Keywords extracted from abstracts were utilized to augment the information provided by author-supplied keywords, compensating for either the absence of specified keywords in certain papers or when authors furnished a restricted set of keywords. The approach for extracting these keywords from abstracts was adapted from the methodology outlined in Zhang ( 2019 ). It is crucial to underscore that the keywords extracted from abstracts serve as supplementary additions to the author-provided keywords. Second, the raw frequency information of each keyword was computed. Third, the raw frequencies of each topic are normalized for the statistical test in the next step. Normalized frequencies of the topics were the prerequisite of a valid comparison since there were substantial differences in the number of publications in the three periods. We adopted the method proposed by Lei and Liu ( 2019b ) for the normalization. That is, for example, the normalized frequency of an author-supplied keyword in a period was calculated using the following formula: normalized frequency = (raw frequency in that period/total number of publications in that period) * 10,000. Last, a one-way chi-square test of the normalized frequencies of each topic in the three periods was conducted for the identification of significant cross-period differences between the research topics.
Results and discussion
In this section, the information about the productivity, authors, and affiliations of the retrieved publications is presented, followed by a co-citation analysis to visualize the intellectual structures in terms of influential publications, references, and authors, as well as the keyword analysis that could facilitate the identification of prominent topics in the field.
Annual volume of publications, authors, and their affiliations
A total number of 4598 publications on prosody in the SSCI-indexed key linguistic journals were retrieved. Figure 1 shows the annual productivity of prosody articles in linguistic journals. From early 2000 the publications exhibited an upward trend and remained at more than 300 publications per year since 2019 (see Fig. 1 ). A dip in terms of the number of publications in 2020 can be observed, likely due to the COVID-situation which slowed the research and publication process across the board.
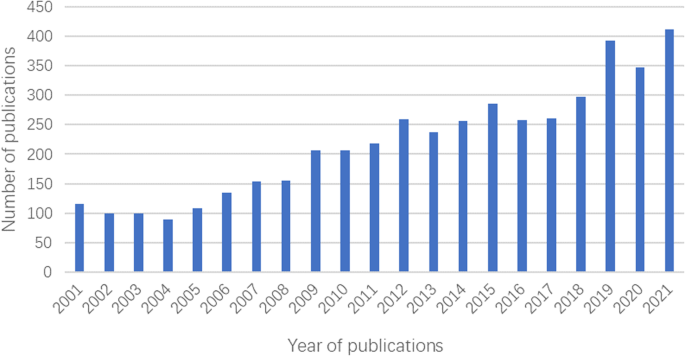
Distribution of prosody articles from 2001 to 2021.
Table 1 shows the top 25 prolific authors, regions, and institutions/authors’ affiliations with which the publications were associated. In terms of authors, 58 published more than 10 (10 included) articles, while 5279 authors published one article, the former only accounting for 0.81% of the total number of authors. Regarding the regions, the USA, Germany, England, Canada, the Netherlands, Australia, France, China, Spain, and Japan (in descending order in terms of being involved in publications related to prosody) topped the first ten and each was associated with more than 100 publications. When it comes to authors’ affiliations, the top five most prolific institutions published more than 100 articles. It is not surprising to note that the most prolific authors were highly associated with prolific regions and institutions.
Co-citation analysis and network mapping
Top-cited sources of publications.
Figures 2 – 4 show the network maps of the top 50 most-cited sources in the three periods (2001–2007, 2008–2014, 2015–2021), respectively, using the smart local moving algorithm (Waltman and van Eck, 2013 ). The term “sources” denotes the academic journals or books in which the references have been published. The density view is provided below in companion to illustrate the most-cited sources of publications in the respective period. The network maps show the major clusters of the top 50 most-cited sources in the three periods.
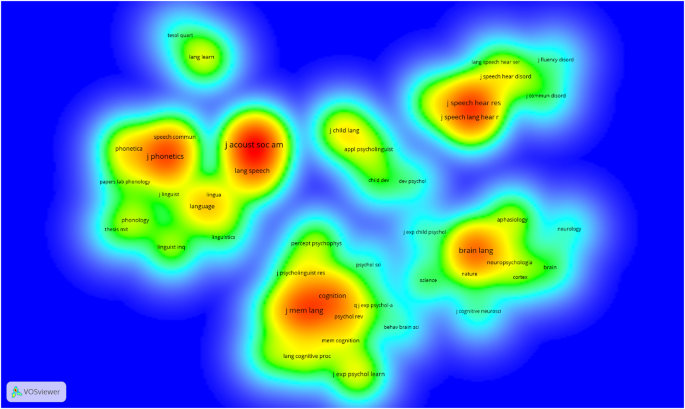
Network map of the most-cited sources of publications (2001‒2007).
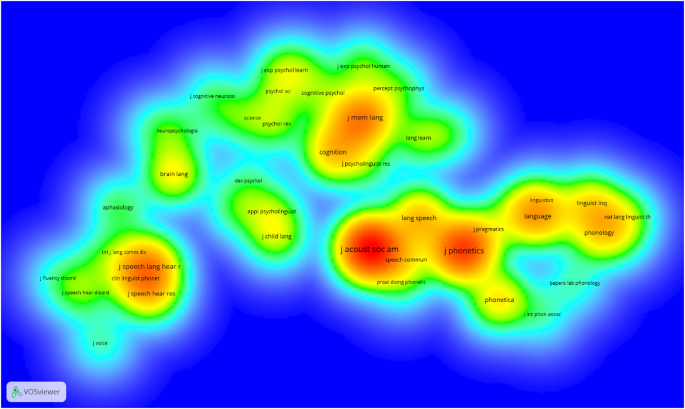
Network map of the most-cited sources of publications (2008‒2014).
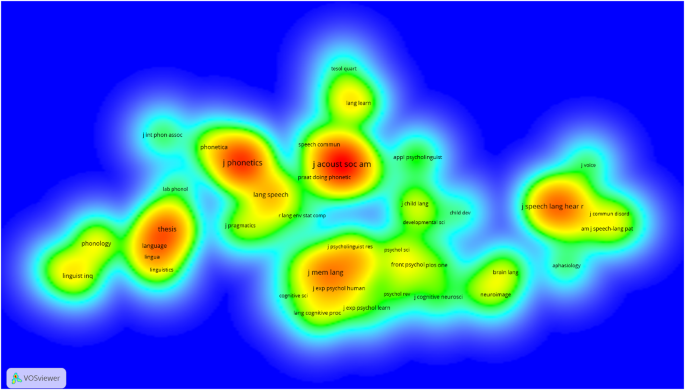
Network map of the most-cited sources of publications (2015‒2021).
Firstly, it is important to note that, as shown in Table 2 , the Journal of Acoustic Society of America and the Journal of Phonetics have remained to be the top two most-cited journals across the three periods. The number of citations in the two journals has increased sharply across the three periods (2329 citations in the first period, 6249 in the second, and 9978 in the third). Five other journals (i.e. Journal of Memory and Language , Journal of Speech Language Hearing Research , Language and Speech , Cognition , and Phonetica ) have always remained the top 10 most-cited sources. These journals publish works in the production and comprehension of language and speech (including prosody), serving as valuable venues for novice researchers pursuing research in this area. It is important to note that ‘thesis’ is one of the most-cited sources of publications, which is probably because certain doctoral theses (e.g. Rooth, 1985 ) by influential experts on prosody have received substantial and continuous attention over the years.
These network maps indicate not only sub-areas of prosody research but also an interesting merge and split of research areas across the three periods in this field. The first period (Fig. 2 ) indicates five major clusters Footnote 4 representing five main areas in prosody. From left to right, the first is related to the linguistic investigation (e.g., Journal of Phonetics , Journal of Acoustic Society of America , Language and Speech ); the second small cluster on the top relates to L2 learning (e.g., TESOL Quarterly , Language Learning ); the third cluster on the bottom concerns the psycholinguistic aspects (e.g., Journal of Memory and Language , Cognition , Language and Cognitive Processes ); the fourth widely-spread cluster on the top right of the map is language development/language disorder (e.g., Journal of Child Language and Journal of Speech Hearing Research ); the last cluster located at the bottom right of the map represents the neurolinguistic research on prosody (e.g., Brain and Language , Neuropsychologia ). The clusters in the second (Fig. 3 ) and third periods (Fig. 4 ) are similar to those in the first period (Fig. 2 ). However, several changes are worth noticing. For example, the second period witnessed a merge of psycholinguistic and neurolinguistic journals (top) which then became the largest cluster of all, dominating the whole map. In addition, the third period has again separated the experimental approach from the formal/theoretical approach (e.g., Laboratory Phonology vs. Linguistic Inquiry ). Consistent with the increasing references and authors in the L2 research area identified in further below sections, the cluster of L2 prosody of the network map has expanded slightly from 2001–2007 (top of Fig. 2 ) to the 2015–2021 period (top of Fig. 4 ).
The highly influential references
Figures 5 – 7 show the network map of the top 50 most-cited references in the three periods (2001–2007, 2008–2014, and 2015–2021), respectively. The network map in Fig. 5 shows four major clusters of the top 50 (out of 24,383) most-cited references that were cited 16 times or more between 2001 and 2007. Figure 6 has five clusters of the top 50 (out of 51,107) cited references that were cited 37 times or more. Figure 7 represents four clusters of the top 50 (out of 82,660) cited references that were cited at least 46 times.
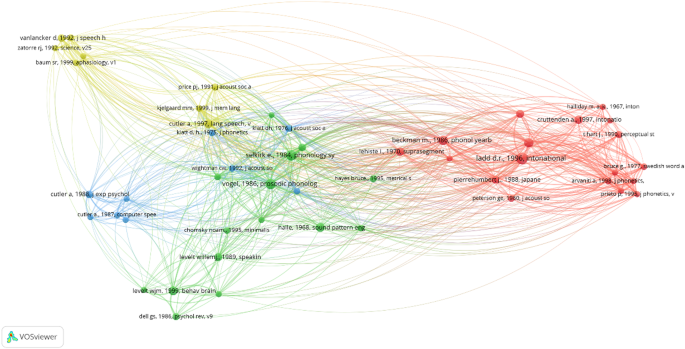
Network map of the most-cited references (2001‒2007).
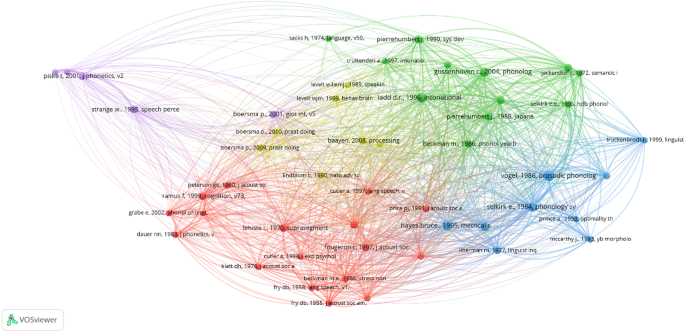
Network map of the most-cited references (2008‒2014).
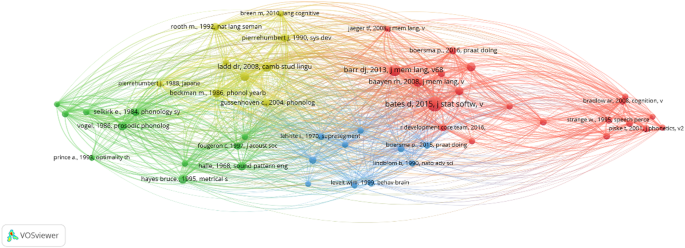
Network map of the most-cited references (2015‒2021).
Fifteen references have appeared on the top 50 list across all three periods, with Beckman and Pierrehumbert 1986 , Chomsky and Halle ( 1968 ), Hayes ( 1995 ), Ladd ( 1996 ; 2008 ), Pierrehumbert ( 1990 ); Selkirk ( 1984 ), and Nespor and Vogel ( 1986 ) remaining in the top 20 throughout (the top 10 references were listed in Table 3 ). Five publications by Cutler and colleagues ( 1986 ; 1987 ; 1988 ; 1992 ; 1997 ) and four publications by Ladd ( 1996 , 2008; Ladd et al., 1999 , 2000 ) were on the list. Two clusters led by Ladd ( 1996 / 2008 ) Footnote 5 and Nespor and Vogel ( 1986 ) representing intonational phonology and prosodic phonology were among the top three most-cited references between 2001 and 2007, and between 2008 and 2014, and continued to be popularly cited between 2015 and 2021 ranking 4th for Ladd and ranking 8th for Nespor and Vogel ( 1986 ). Some other important references in the same cluster as Ladd across the three periods are Pierrehumbert and Beckman ( 1988 ), and Pierrehumbert and Beckman ( 1988 ), whose works are associated with the “Autosegmental-Metrical” (AM) approach that describes prosody on autonomous tiers for metrical structure and tones. The same cluster in the third period (Fig. 7 ) also covered publications in information structure (e.g., Rooth, 1992 ) and the use of prosody in marking information structure (Breen et al., 2010 ). These publications only made their first appearance on the Top 50 list only between 2015 and 2021. The possible reason might be the recent interest in the acoustic realization of focus as well as testing the Roothian theory that focus indicates the presence of alternatives that are relevant for the interpretation of discourse in a range of languages (e.g., Braun et al., 2019 ; Braun and Tagliapietra, 2010 ; Gotzner, 2017 ; Repp and Spalek, 2021 ; Spalek et al., 2014 ; Tjuka et al., 2020 ; Yan and Calhoun, 2019 ; Yan et al., 2023 ).
The largest cluster is located on the right side of the 2015–2021 map (Fig. 7 ), and this appears to be the only cluster that emerged in the last period, indicating a general topic of statistical methods/tools such as mixed-effects modeling with crossed random effects for subjects and items (Baayen et al., 2008 ) and logit mixed models (Jaeger, 2008 ) Footnote 6 . This newly emerged cluster also indicates the importance of applying state-of-the-art statistics in prosody research. These two references have been influential in motivating researchers, especially psycholinguists and cognitive psychologists, to switch from ANOVA to MEM analysis, with the latter now being the dominant type of analysis. Some of the most-cited references in this cluster are concerned with tools commonly used in prosodic research and analysis such as R software (R Core Team, 2017 ) and Praat (Boersma and Weenink, 2018 ) Footnote 7 . Some focus on model fitting procedures, e.g., parsimonious mixed models (Bates et al., 2015 ) and ‘maximal’ models (Barr et al. 2013 ). Although Baayen et al. ( 2008 ) was already cited 56 times, ranking the 14th between 2008 and 2014, its citations doubled to 118 in the recent period, ranking the 5th between 2015 and 2021, with Bates et al. ( 2015 ) and Barr et al. ( 2013 ) ranking the second and the third with 287 and 177 citations, respectively.
The most influential authors
Table 4 shows the top 50 most-cited authors across the three periods. It is not surprising that some authors of the most-cited references discussed in the previous section are also the most-cited authors overall (e.g., D.R. Ladd, M. Beckman, J. Pierrehumbert, E. Selkirk). Twenty-one of the top 50 authors have remained very influential across the three periods, among whom nine authors have topped the first 20 in all three periods (i.e., A. Cutler, J. Flege, C. Gussenhoven, D.R. Ladd, M.J. Munro, J. Pierrehumbert, E. Selkirk, L.D. Shriberg, Y. Xu). A. Cutler and J. Flege have remained to be in the top five most-cited authors list across all three periods.
With the trend of applying mixed-effects models using R software in prosody research, Bates et al. ( 2015 ), Barr et al. ( 2013 ), Baayen et al. ( 2008 ), and R Core Team ( 2017 ) moved to the most-cited authors’ list in the third period, i.e., 2015–2021 (see the middle cluster in Fig. 10 , network map of the most-cited authors). Among the many researchers who became influential authors, S.A. Jun joined the bottom right cluster (see Fig. 10 ), and the other influential authors in this cluster have remained highly cited across three periods (i.e., Y. Xu, L.D. Shriberg, J. Pierrehumbert, C. Gussenhoven, D.R. Ladd, P. Prieto, A. Arvaniti, M. Beckman, and E. Grabe). Notably, at the bottom of the 2001–2007 map (Fig. 8 , network map of the most-cited authors) is the smallest cluster represented by F. Flege and M. J. Munro, most likely the L2 prosody cluster. The cluster has continuously expanded across the three periods (left side of the 2008–2014, Fig. 9 and 2015–2021 map, Fig. 10 ) and was joined by other researchers in similar fields such as T.M. Derwing, P.K. Kuhl, K. Saito. It is interesting to note that some researchers are notably prolific within specific areas, such as Flege and Munro in the realm of L2 prosody, while others, like Ladd and Pierrehumbert, hold influence across the broader spectrum of the field. This divergence could probably be detected through cluster analysis. For instance, the former might have citations concentrated within a single cluster, while the latter could be cited across multiple clusters (see Fig. 10 ).
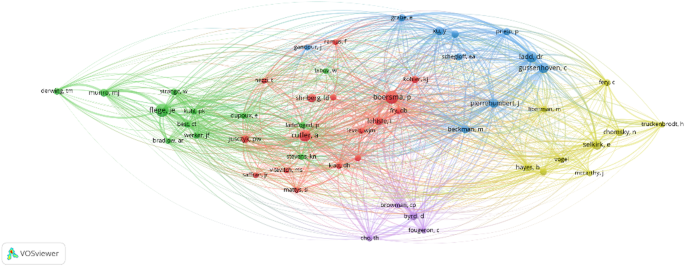
Network map of the most-cited authors (2001‒2007).
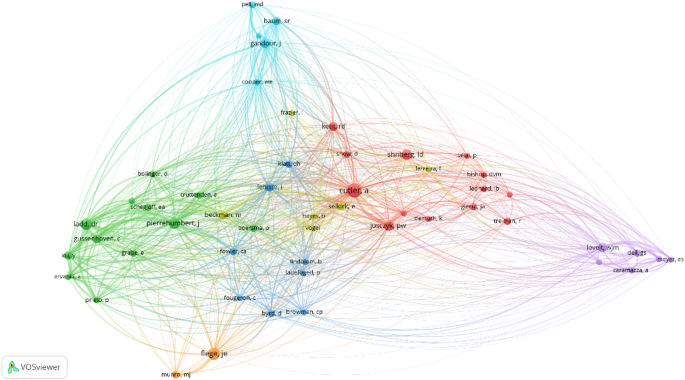
Network map of the most-cited authors (2008–2014).
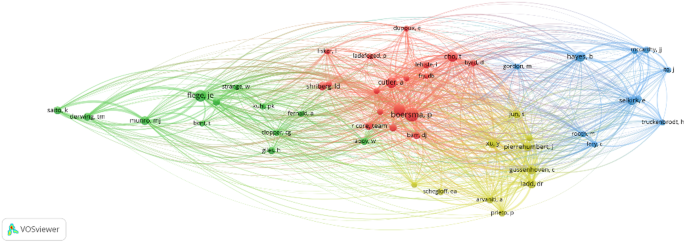
Network map of the most-cited authors (2015–2021).
Keyword analysis
Keywords in the retrieved publications across the three periods whose number of occurrences equal to or greater than 10 were submitted for Chi -square analysis to test for significant changes across the three periods. This resulted in a total of 207 author-supplied keywords (out of 7269, 2.85%) and 37 keywords-from-abstracts (out of 821, 4.50%). The cut-off point of 10 was chosen because we observed that the p -values of nearly all keywords with frequencies below 10 were larger than 0.05, indicating that the frequencies of these keywords remained stable across all three stages and did not undergo significant changes.
The results revealed that 61 keywords experienced a significant change in frequency ( p < 0.05) and the other keywords showed no significant change ( p ≥ 0.05) across the three periods. The results from keyword analysis uncovered important research trends in the field of prosody in the past 21 years. Firstly, it is unsurprising to note that the top ten most frequent author-supplied keywords (see Table 5 ) are closely related to (1) the concept of prosody (including prosody itself, intonation , phonology , stress and accent / accents ), (2) the two main aspects of the investigation of prosody (i.e., speech perception and speech production ), (3) the notion in information structure (i.e., focus ) that is usually signaled by prosody and widely studied by prosody researchers, (4) the language that is possibly most commonly investigated (i.e., English ) and (5) bilingualism , which appears to be widely researched, especially from the second period (2008 onwards). It is important to note that in Table 5 bilingualism was the only one on the top ten list whose frequency increased throughout the three periods, indicating the increasing significance of bilingual prosody research. Seven out of 10 topics have remained to be the most discussed throughout, while the other two topics have displayed a downward trend. The possible reasons for these trends will be discussed further below.
In the keyword analysis, as mentioned above, the biggest group contains words that remained unchanged in terms of the normed frequencies across the three periods, suggesting these topics are frequently discussed. One of the important findings is that the areas closely related to prosody, such as syntax (total count of author-supplied keywords across three periods: 50), morphology (53), lexical stress (52), and conversation analysis (59), turned out to be frequently discussed (≥30) research topics across the three periods. This suggests these areas have received constant attention in prosody research given the importance of prosody in these areas (Cole et al., 2010b ; Fodor, 1998 ; Harley et al., 1995 ; Kjelgaard and Speer, 1999 ; Pratt, 2018 ; Pratt and Fernandez, 2016 ; Selkirk, 2011 , 1984 ). Another key point to note is that keywords such as English , French , Dutch , Mandarin Chinese , and Japanese are languages that researchers in the field have maintained interest in throughout the history of prosody research. Among these languages, English has the most frequent occurrence, probably due to the importance of using prosody in language comprehension in English (as reviewed in Calhoun et al., 2023 ; Cole, 2015 ; Cutler et al., 1997 ) as well as its status as lingua franca leading to a large number of both L1 and L2 speakers. Other languages such as Mandarin Chinese (as a tonal language) and Japanese (as a pitch accent language) were also frequently investigated languages due to their typical prosodic features and their larger number of speakers (see Kügler and Calhoun, 2020 ). More importantly, intonation , fundamental frequency ( f0 ), accent ( s ), pitch accent Footnote 8 , stress and tone which are expected to be key topics in prosody research have indeed been shown to be the most-discussed throughout and continue to be the focus of prosody research.
We now turn to the keywords that have experienced a significant change, whose trends could be further divided into three groups. A sample of the three groups is provided in Table 6 . Group 1 displays a general increase across the three periods, Group 2 a general decrease, and Group 3 a rise across the first two periods followed by a fall in the third period (although all normed frequencies in the last period were higher than the first period). Group 1 concerns topics that involve a second language or more than one language: bilingualism , second language ( L2 ), second language acquisition , foreign accent ( s ) and cross-linguistic influence ( CLI ), suggesting that studying prosody in L2 or multilingual speakers beyond their native languages has gained more popularity across the three periods. This is probably because of the introduction of the L2 intonation learning theory (Mennen, 2015 ) which has been attested to be a useful model to predict difficulties that L2 learners encounter based on the intonation differences in learners’ L1 and L2. Group 1 also contains topics that might show newly developed directions in the last two decades: language attitudes , voice onset time ( VOT ), sound change , tone sandhi ( TS ), and syntax-phonology interface . Electroencephalography ( EEG ) is a topic in Group 1, indicating its rising importance in prosody research and its close connection to neuroscience to investigate brain activity in response to prosodic stimuli. This reflects the increasingly interdisciplinary nature of prosody research.
While some topics have gained increased attention across the three periods, some seem to experience a drop from the second period to the third period, following a rise from the first to the second period (Group 2). The representative topics in descending order in terms of frequencies are gesture , aphasia , language contact ( s ), and Cantonese . Many of these topics rose from no occurrence in the first period and maintained 10 or more occurrences in the second and third periods. It is within expectation to note that gesture , as a key visual cue, became more popular in the second period. It is probably because the research in this field was boosted by the publication of the special issue Audiovisual Prosody edited by Krahmer and Swerts ( 2009 ), and the special issue seems to have a lasting effect on this topic in the third period as it was still more frequent than in the first period. Further, aphasia , a commutative disorder resulting from brain damage, was one of the topics that were receiving less attention from the second to the third period. Inspection of the entire keyword list shows that in the third period, aphasia appeared in a number of other forms: primary progressive aphasia , aphasia rehabilitation , aphasia severity , deep dysphasia , fluent aphasia , music, and aphasia .
A number of topics seem to become less interesting to researchers in prosody and exhibit a decreasing trend in terms of frequencies (Group 3). Although prosody and phonology were highly frequent across the three periods, the normed frequencies nevertheless showed a downward trend. At first, it seemed impossible that the two became less important, however, it is reasonable that prosody and phonology were replaced by more specific terms such as F0 , pitch , or stress as keywords, and these terms were preferred for later empirical investigations of prosody. The frequencies of syllable and syllable structure decreased, possibly for similar reasons that these terms are relatively general and may have been substituted with more specific and relevant keywords such as onset , coda , or rhyme that are used in linguistic analysis to describe the components and organization of syllables.
Conclusion and implications
The present study has provided a systematic review of prosody research from 2001 to 2021 in linguistic journals through a bibliometric analysis. Based on the key findings in this study, several significant implications for prosody research have emerged. First, our results have shown a general rise of prosody-related publications in the past two decades, showing its increased significance in broader linguistic research. Second, the co-citation analysis has identified the most cited authors, references and journals, providing valuable information for scholars, especially novel researchers in the prosody field of where the most influential prosody research can be found, who is doing that research, and what areas that research covers. Another important finding worth noticing is that prosody research has witnessed a significant increase in statistical methods especially mixed effect models in the latest two periods (2008–2014, 2015–2021), compared to the previous period (2001–2007). This increase is likely due to the influential publication of the special issue Emerging Data Analysis in the Journal of Memory and Language in 2008. Therefore, it is reasonable to argue that as a unique mode of communication in academia, special issues are effective in highlighting essential or emerging research topics in a specific discipline.
Additionally, findings in the present bibliometric analysis shed light on research trends in prosody. For example, it reveals that intonation , stress and accent remained as the most-discussed topics across the three periods given their high relevance to prosody. It is also unsurprising that speech perception and production are also among the most-discussed topics. Some trends were observed by comparing the normed frequencies across the three periods. For instance, bilingualism has gained more popularity as a research topic from the second period, showing researchers’ increased focus on it given that more people are becoming bilingual or multilingual due to globalization. However, some languages (e.g. English, Chinese, and Japanese) always remain the most researched. The prevalence of English and Chinese might be partially attributed to extensive speaker and learner bases and existing extensive literature on prosody, while the rise of Japanese in prosody research could be attributed to the pioneering contributions made by Pierrehumbert and Beckman.
The bibliometrics-based method has gained popularity in the recent decade to offer a systematic review of research trends in many fields (e.g. Fu et al., 2021 ; Lei and Liu, 2019a ; Wu, 2022 ; Zhang, 2019 ). Although this quantitative analysis is based on a substantial number of research papers and reveals developmental patterns of a research topic across different periods, some limitations are observed in the present study. First, as this paper aims to review a large number of prosody-related studies and provide major trends of research on prosody, we have to acknowledge that the literature search does not guarantee that every piece of relevant literature can be covered, due to the selection of search terms and the authors’ choice of keywords in their publications. In the pursuit of a comprehensive understanding of prosody research, we acknowledge a limitation that our choice of search terms may not encapsulate the entire landscape of prosody-related concepts. For instance, concepts such as “duration” and “emphasis” play pivotal roles in prosody analysis but also may have potentially led to an overly broad search with irrelevant results. However, it should be noted that although these terms were not included as search terms, they appeared in our list of keywords given their relevance to prosody. Future studies could explore a broader spectrum of prosodic elements, thereby further advancing the field of prosody research.
Another possible limitation is the sources for the prosody-related articles: some of the prominent journals were excluded from the publication analysis or keyword analysis because they did not meet the criteria in the filtering process in the present study. For example, Journal of Acoustic Society of America is a usual place for prosodists to publish their high-quality research, Frontiers in Psychology published extensively on linguistics, and Linguistic Inquiry might be a major sources of citation. It should be noted that such journals do appear in the co-citation analysis if they are frequently cited, and the inclusion of such journals in the publication/keyword analyses in future studies might be beneficial. Additionally, given the quantitative nature of the study, a more detailed qualitative analysis is needed to complement it; and given the space limitations of the paper, it is not possible to delve into every aspect of the significant trends observed on prosody.
Furthermore, although the qualitative analysis of the research trends was supported by quantitative data, some extent of subjectivity was still involved. Therefore, the interpretations of research trends in our paper need to be confirmed or substantiated by other experts on prosody. It would be more helpful if bibliometric reviews could be read together with traditional reviews to gain a fuller picture of research in prosody.
Data availability
The data that support the findings of this study are available from the corresponding author upon reasonable request.
Semantic prosody refers to the phenomenon in linguistics where certain words or phrases evoke a specific positive or negative connotation due to their consistent co-occurrence with particular words or in certain contexts (Hunston, 2007 ; Omidian and Siyanova‐Chanturia, 2020 ). This term was deliberately excluded from the search since our research focuses on speech prosody rather than the connotation of words in the context that ‘semantic prosody’ refers to.
It should be noted for practical purposes, not all prestigious journals can be included. Therefore, we choose to gather data from SSCI journals as they are generally more relevant to the field of linguistics in comparison to SCI journals.
Keywords-from-abstracts are nouns and noun phrases extracted from abstracts. There were three steps in extracting keywords from abstracts. First, n-grams (up to four) of nouns and noun phrases were extracted from the POS-tagged abstract in each article. During this step, instances of an n-gram appearing multiple times within an abstract were consolidated into a single occurrence to prevent an overabundance of counts. Second, the authors manually checked and filtered the n-grams to identify keywords. Third, the keywords identified in the preceding step were refined through the removal of duplicated items found in the author-supplied keywords.
It is important to note that the identification of common themes within journals does not imply that the journals confined to a particular cluster exclusively cover those themes. Journals may also publish content that is relevant to other thematic clusters. For instance, research focusing on the second language (L2) acquisition of prosody through psycholinguistic methods could potentially find its place in more than one thematic cluster, reflecting the interdisciplinary nature of prosodic research and its capacity to contribute to multiple areas of study.
Ladd ( 2008 ) is the second edition of Ladd ( 1996 ).
These two seminal works were published thanks to the special issue Emerging Data Analysis in the Journal of Memory and Language edited by Kenneth I. Forster, and Michael E.J. Masson dedicated to mixed effect models (MEMs).
It is important to note that Praat exists in many versions, and we have amalgamated all versions in the analysis and decided to cite the 2018 version here as it received most citations among many versions.
As two terms “accent(s)” as used in L2 accent or foreign accent and “pitch accent” as used based on the AM model may indicate different concepts, e.g., “accent(s)”, they were listed separately.
Baayen RH, Douglas JD, Douglas MB (2008) Mixed-effects modeling with crossed random effects for subjects and items. J Mem Lang 59(4):390–412
Article Google Scholar
Barr DJ, Levy R, Scheepers C, Tily HJ (2013) Random effects structure for confirmatory hypothesis testing: keep it maximal. J Mem Lang 68(3):255–278
Bates D, Mächler M, Bolker B, Walker S (2015) Fitting linear mixed-effects models using Lme4. J Stat Softw 67(1):1–48. https://doi.org/10.18637/jss.v067.i01
Beckman ME, Pierrehumbert JB (1986) Intonational structure in Japanese and English. Phonology 3:255–309
Birch S, Clifton Jr C (1995) Focus, accent, and argument structure: effects on language comprehension. Lang Speech 38(Pt 4):365–391. https://doi.org/10.1177/002383099503800403
Article PubMed Google Scholar
Boersma P, Weenink D (2018) Praat: doing phonetics by computer [computer program]. Version 6.0. 37. Accessed 3 Feb 2018 http://www.praat.org
Braun B, Tagliapietra L (2010) The role of contrastive intonation contours in the retrieval of contextual alternatives. Lang Cogn Process 25(7–9):1024–1043. https://doi.org/10.1080/01690960903036836
Braun B, Dehé N, Neitsch J, Wochner D, Zahner K (2019) The prosody of rhetorical and information-seeking questions in German. Lang Speech 62(4):779–807. https://doi.org/10.1177/0023830918816351
Breen M, Fedorenko E, Wagner M, Gibson E (2010) Acoustic correlates of information structure. Lang Cogn Process 25(7–9):1044–1098
Broadus RN (1987) Toward a definition of “Bibliometrics. Scientometrics 12(5–6):373–379. https://doi.org/10.1007/BF02016680
Calhoun S (2010) The centrality of metrical structure in signaling information structure: a probabilistic perspective. Language 86(1):1–42. https://doi.org/10.1353/lan.0.0197
Calhoun S, Warren P, Yan M (2023) Cross-language influences in the processing of L2 prosody. In: Elgort I, Siyanova-Chanturia A, Brysbaert M (eds) Cross-language influences in bilingual processing and second language acquisition. John Benjamins Publishing Company, pp. 47–73
Chomsky N, Halle M (1968) The sound pattern of English. Harper & Row
Clopper CG, Tonhauser J (2013) The prosody of focus in Paraguayan Guaraní. Int J Am Linguist 79(2):219–251
Cole J (2015) Prosody in context: a review. Lang Cogn Neurosci 30(1–2):1–31. https://doi.org/10.1080/23273798.2014.963130
Cole J, Mo Y, Baek S (2010a) The role of syntactic structure in guiding prosody perception with ordinary listeners and everyday speech. Lang Cogn Process 25(7–9):1141–1177
Cole J, Mo Y, Baek S (2010b) The role of syntactic structure in guiding prosody perception with ordinary listeners and everyday speech. Lang Cogn Process 25(7–9):1141–1177
Cutler A, Carter DM (1987) The predominance of strong initial syllables in the English vocabulary. Comput Speech Lang 2(3–4):133–142
Cutler A, Norris D (1988) The role of strong syllables in segmentation for lexical access. J Exp Psychol: HPP 14:113–121
Google Scholar
Cutler A, Butterfield S (1992) Rhythmic cues to speech segmentation: evidence from juncture misperception. J Mem Lang 31(2):218–236
Cutler A, Dahan D, van Donselaar W (1997) Prosody in the comprehension of spoken language: a literature review. Lang Speech 40(2):141–201. https://doi.org/10.1177/002383099704000203
Cutler A, Dahan D, Van Donselaar W (1997) Prosody in the comprehension of spoken language: a literature review. Lang Speech 40(2):141–201
Cutler A, Mehler J, Norris D, Segui J (1986) The syllable’s differing role in the segmentation of French and English. J Mem Lang 25(4):385–400
Cutler A, Mehler J, Norris D, Segui J (1992) The monolingual nature of speech segmentation by bilinguals. Cogn Psychol 24:381–410
Article CAS PubMed Google Scholar
Fodor JD (1998) Learning to parse? J Psycholinguist Res 27(2):285–319
Frazier L, Katy C, Charles Jr C (2006) Prosodic phrasing is central to language comprehension. Trends Cogn Sci 10(6):244–249
Fu Y, Wang H, Guo H, Bermúdez-Margaretto B, Domínguez Martínez A (2021) What, where, when and how of visual word recognition: a bibliometrics review. Lang Speech 64(4):900–929. https://doi.org/10.1177/0023830920974710
Gotzner N (2017) Alternative sets in language processing: how focus alternatives are represented in the mind. Springer
Gussenhoven C (2004) The phonology of tone and intonation. Cambridge University Press
Gussenhoven C, Chen A (eds) (2020) The Oxford handbook of language prosody. Oxford University Press, Oxford, UK
Harley B, Howard J, Hart D (1995) Second language processing at different ages: do younger learners pay more attention to prosodic cues to sentence structure? Lang Learn 45(1):43–71. https://doi.org/10.1111/j.1467-1770.1995.tb00962.x
Hayes B (1995) Metrical stress theory: principles and case studies. University of Chicago Press, Chicago
Hjørland B (2013) Facet analysis: the logical approach to knowledge organization. Inf Process Manag 49(2):545–557. https://doi.org/10.1016/j.ipm.2012.10.001
Hunston S (2007) Semantic prosody revisited. Int J corpus Linguist 12(2):249–268
Hwang H, Schafer AJ (2006) Prosodic effects in parsing early vs. late closure sentences by second language learners and native speakers. In: Hoffmann R, Mixdorff H (eds) Speech prosody 2006, third international conference, paper 091. International Speech Communication Association, Dresden, Germany, May 2–5, 2006
Ip MHK, Cutler A (2022) Juncture prosody across languages: similar production but dissimilar perception. Lab Phonol 13(1)
Jaeger TF (2008) Categorical data analysis: away from ANOVAs (transformation or not) and towards logit mixed models. J Mem Lang 59(4):434–446
Article PubMed PubMed Central Google Scholar
Katz J, Selkirk E (2011) Contrastive focus vs. discourse-new: evidence from phonetic prominence in English. Language 87:771–816
Kjelgaard MM, Speer SR (1999) Prosodic facilitation and interference in the resolution of temporary syntactic closure ambiguity. J Mem Lang 40(2):153–194. https://doi.org/10.1006/jmla.1998.2620
Krahmer E, Swerts M (2009) Audiovisual prosody—introduction to the special issue. Lang Speech 52(2–3):129–133. https://doi.org/10.1177/0023830909103164
Kügler F, Calhoun S (2020) Prosodic encoding of information structure. In: Gussenhoven C, Chen A (eds) The Oxford handbook of language prosody. Oxford University Press, Oxford, UK, pp. 454–467
Ladd DR, Mennen I, Schepman A (2000) Phonological conditioning of peak alignment in rising pitch accents in Dutch. J Acoust Soc Am 107(5):2685–2696
Article ADS CAS PubMed Google Scholar
Ladd DR, Dan F, Hanneke F, Schepman A (1999) Constant “segmental anchoring” of F0 movements under changes in speech rate. J Acoust Soc Am 106(3):1543–1554
Ladd DR (1996, 2008) Intonational phonology, 2nd edn. Cambridge studies in linguistics. Cambridge University Press, Cambridge, New York
Lee D (2023) Bibliometric analysis of Asian ‘language and linguistics’ research: a case of 13 countries. Humanit Soc Sci Commun 10(1):1–23
Article MathSciNet CAS Google Scholar
Lee E-K, Watson DG (2011) Effects of pitch accents in attachment ambiguity resolution. Lang Cogn Process 26(2):262–297
Lehiste I (1970) Suprasegmentals. Massachusetts Institute of Technology, Diss
Lei L, Liu D (2019a) Research trends in applied linguistics from 2005 to 2016: a bibliometric analysis and its implications. Appl Linguist 40(3):540–561
Article ADS Google Scholar
Lei L, Liu D (2019b) The research trends and contributions of system’s publications over the past four decades (1973–2017): a bibliometric analysis. System 80:1–13
Levelt WJM, Roelofs A, Meyer AS (1999) A theory of lexical access in speech production. Behav Brain Sci 22(1):1–38
Lin Y, Ding H, Zhang Y (2020) Prosody dominates over semantics in emotion word processing: evidence from cross-channel and cross-modal stroop effects. J Speech Language Hear Res 63(3):896–912. https://doi.org/10.1044/2020_JSLHR-19-00258
Mennen I (2015) Beyond segments: towards a L2 intonation learning theory. In: Delais-Roussarie E, Mathieu A, Sophie H (eds) Prosody and language in contact. Prosody, phonology and phonetics. Springer, Berlin, Heidelberg
Namjoshi J, Tremblay A (2014) The processing of prosodic focus in French. Columbus, OH, USA
Nespor M, Vogel I (1986) Prosodic phonology. Foris publications, Dordrecht
Norris D, Cutler A, McQueen JM, Butterfield S (2006) Phonological and conceptual activation in speech comprehension. Cogn Psychol 53(2):146–193
O’Brien MG, Jackson CN, Gardner CE (2014) Cross-linguistic differences in prosodic cues to syntactic disambiguation in German and English. Appl Psycholinguist 35(1):27–70. https://doi.org/10.1017/S0142716412000252
Omidian T, Siyanova‐Chanturia A (2020) Semantic prosody revisited: Implications for language learning. TESOL Q 54(2):512–524
Pell MD, Jaywant A, Monetta L, Kotz SA (2011) Emotional speech processing: disentangling the effects of prosody and semantic cues. Cogn Emotion 25(5):834–853. https://doi.org/10.1080/02699931.2010.516915
Pierrehumbert J (1980) The phonology and phonetics of English intonation. Dissertation, Massachusetts Institute of Technology
Pierrehumbert J (1990) The meaning of intonational contours in the interpretation of discourse Janet Pierrehumbert and Julia Hirschberg. Intentions Commun 271
Pierrehumbert J, Beckman M (1988) Japanese tone structure. Linguistic Inquiry Monogr (15):1–282
Pratt E, Fernandez EM (2016) Implicit prosody and cue-based retrieval: L1 and L2 agreement and comprehension during reading. Front Psychol 7:1922. https://doi.org/10.3389/fpsyg.2016.01922
Pratt E (2018) Prosody in sentence processing. In: Fernandez EM, Smith Cairns H (eds) The handbook of psycholinguistics. John Wiley & Sons, Inc., Hoboken, NJ, pp. 365–391
Prieto P (2015) Intonational meaning. Wiley Interdiscip Rev: Cogn Sci 6(4):371–381. https://doi.org/10.1002/wcs.1352
Pritchard A (1969) Statistical bibliography or bibliometrics. J Documentation 25(4):348
R Core Team (2017) R: a language and environment for statistical computing. R Foundation for Statistical Computing, Vienna, Austria
Repp S (2020) The prosody of Wh-exclamatives and Wh-questions in German: speech act differences, information structure, and sex of speaker. Language Speech 63(2):306–361. https://doi.org/10.1177/0023830919846147
Repp S, Spalek K (2021) The role of alternatives in language. Front Commun 6:682009
Roncaglia-Denissen MP, Schmidt-Kassow M, Heine A, Kotz SA (2014) On the impact of L2 speech rhythm on syntactic ambiguity resolution. Second Lang Res 31(2):157–178. https://doi.org/10.1177/0267658314554497
Rooth M (1992) A theory of focus interpretation. Nat Lang Semant 1(1):75–116
Rooth M (1985) Association with focus. PhD thesis, University of Massachusetts, MA, USA
Rossetto DE, Bernardes RC, Borini FM, Gattaz CC (2018) Structure and evolution of innovation research in the last 60 years: review and future trends in the field of business through the citations and co-citations analysis. Scientometrics 115(3):1329–1363
Sanders LD, Helen JN, Marty GW (2002) Speech segmentation by native and non-native speakers: the use of lexical, syntactic, and stress-pattern cues. J Speech, Lang, Hearing Res 45(3):519–530
Schafer A, Carlson K, Clifton Jr H, Frazier L (2000) Focus and the interpretation of pitch accent: disambiguating embedded questions. Lang Speech 43(1):75–105
Selkirk E (2011) The syntax–phonology interface. In: Goldsmith JA, Riggle J, Yu ACL (eds) The handbook of phonological theory, vol 2. pp. 435–483
Selkirk E (1984) Phonology and syntax: the relation between sound and structure. The MIT Press, Cambridge
Shattuck-Hufnagel S, Turk AE (1996) A prosody tutorial for investigators of auditory sentence processing. J Psycholinguist Res 25(2):193–247
Small H, Sweeney E (1985) Clustering the science citation index® using co-citations: I. A comparison of methods. Scientometrics 7:391–409
Spalek K, Gotzner N, Wartenburger I (2014) Not only the apples: focus sensitive particles improve memory for information-structural alternatives. J Mem Lang 70:68–84
Steedman M (2000) Information structure and the syntax-phonology interface. Linguist Inq 31(4):649–689. https://doi.org/10.1162/002438900554505
Strange W (1995) Speech perception and linguistic experience: issues in cross-language research
Tjuka A, Nguyen HTT, Spalek K (2020) Foxes, deer, and hedgehogs: the recall of focus alternatives in Vietnamese
van Eck NJ, Waltman L (2010) Software survey: VOSviewer, a computer program for bibliometric mapping. Scientometrics 84(2):523–538
van Eck NJ, Waltman L (2017) Citation-based clustering of publications using CitNetExplorer and VOSviewer. Scientometrics 111(2):1053–1070. https://doi.org/10.1007/s11192-017-2300-7
Wagner M, Watson DG (2010) Experimental and theoretical advances in prosody: a review. Lang Cogn Process 25(7–9):905–945
Waltman L, van Eck NJ (2013) A smart local moving algorithm for large-scale modularity-based community detection. Eur Phys J B 86(11):1–14
Welby P (2003) Effects of pitch accent position, type, and status on focus projection. Lang Speech 46(1):53–81
Wu X (2022) Motivation in second language acquisition: a bibliometric analysis between 2000 and 2021. Front Psychol 13:1032316
Xu Y (1999) Effects of tone and focus on the formation and alignment of F0 contours. J Phon 27(1):55–105
Yan M, Calhoun S (2019) Priming effects of focus in Mandarin Chinese. Front Psychol 10:1985
Yan M, Calhoun S, Warren P (2023) The role of prominence in activating focused words and their alternatives in mandarin: evidence from lexical priming and recognition memory. Lang Speech 66(3):678–705. 00238309221126108
Zhang X (2019) A bibliometric analysis of second language acquisition between 1997 and 2018. Stud Second Lang Acquis 42(1):199–222. https://doi.org/10.1017/S0272263119000573
Zhu H, Lei L (2021) A dependency-based machine learning approach to the identification of research topics: a case in COVID-19 studies. Library Hi Tech
Download references
Acknowledgements
The study was supported by The National Social Science Fund of China (21CYY014).
Author information
Authors and affiliations.
School of Foreign Languages, Huazhong University of Science and Technology, 1037 Luoyu Road, Hongshan District, 430074, Wuhan, Hubei, China
Mengzhu Yan & Xue Wu
You can also search for this author in PubMed Google Scholar
Contributions
These authors contributed equally to this work.
Corresponding author
Correspondence to Xue Wu .
Ethics declarations
Competing interests.
The authors declare no competing interests.
Ethical approval
This article does not contain any studies with human participants performed by any of the authors.
Informed consent
Additional information.
Publisher’s note Springer Nature remains neutral with regard to jurisdictional claims in published maps and institutional affiliations.
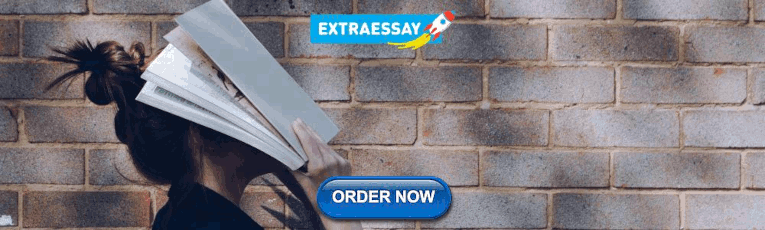
Supplementary information
Supplementary data, rights and permissions.
Open Access This article is licensed under a Creative Commons Attribution 4.0 International License, which permits use, sharing, adaptation, distribution and reproduction in any medium or format, as long as you give appropriate credit to the original author(s) and the source, provide a link to the Creative Commons licence, and indicate if changes were made. The images or other third party material in this article are included in the article’s Creative Commons licence, unless indicated otherwise in a credit line to the material. If material is not included in the article’s Creative Commons licence and your intended use is not permitted by statutory regulation or exceeds the permitted use, you will need to obtain permission directly from the copyright holder. To view a copy of this licence, visit http://creativecommons.org/licenses/by/4.0/ .
Reprints and permissions
About this article
Cite this article.
Yan, M., Wu, X. Prosody in linguistic journals: a bibliometric analysis. Humanit Soc Sci Commun 11 , 311 (2024). https://doi.org/10.1057/s41599-024-02825-9
Download citation
Received : 09 May 2023
Accepted : 13 February 2024
Published : 26 February 2024
DOI : https://doi.org/10.1057/s41599-024-02825-9
Share this article
Anyone you share the following link with will be able to read this content:
Sorry, a shareable link is not currently available for this article.
Provided by the Springer Nature SharedIt content-sharing initiative
Quick links
- Explore articles by subject
- Guide to authors
- Editorial policies

Financial Statement Analysis with Large Language Models
Analysis of news sentiments using natural language processing and deep learning
- Open access
- Published: 30 November 2020
- Volume 36 , pages 931–937, ( 2021 )
Cite this article
You have full access to this open access article
- Mattia Vicari 1 &
- Mauro Gaspari 2
12k Accesses
17 Citations
1 Altmetric
Explore all metrics
This paper investigates if and to what point it is possible to trade on news sentiment and if deep learning (DL), given the current hype on the topic, would be a good tool to do so. DL is built explicitly for dealing with significant amounts of data and performing complex tasks where automatic learning is a necessity. Thanks to its promise to detect complex patterns in a dataset, it may be appealing to those investors that are looking to improve their trading process. Moreover, DL and specifically LSTM seem a good pick from a linguistic perspective too, given its ability to “remember” previous words in a sentence. After having explained how DL models are built, we will use this tool for forecasting the market sentiment using news headlines. The prediction is based on the Dow Jones industrial average by analyzing 25 daily news headlines available between 2008 and 2016, which will then be extended up to 2020. The result will be the indicator used for developing an algorithmic trading strategy. The analysis will be performed on two specific cases that will be pursued over five time-steps and the testing will be developed in real-world scenarios.
Similar content being viewed by others
Stock Price Prediction Using Sentiment Analysis on News Headlines
Stock Direction Prediction Using Sentiment Analysis of News Articles
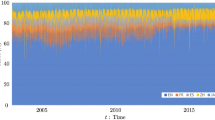
Enhanced news sentiment analysis using deep learning methods
Avoid common mistakes on your manuscript.
1 Introduction
Stock forecasting through NLP is at the crossroad between linguistics, machine learning, and behavioral finance (Xing et al. 2018 ). One of the main NLP techniques applied on financial forecasting is sentiment analysis (Cambria 2016 ) which concerns the interpretation and classification of emotions within different sources of text data. It is a research area revived in the last decade due to the rise of social media and cheap computing power availability (Brown 2016 ). Like products and services, market sentiments influence information flow and trading, thus trading firms hope to profit based on forecasts of price trends influenced by sentiments in financial news (Ruiz-Martínez et al. 2012 ). Is it possible to find predictive power in the stock market’s behavior based on them? It seems to be the case in the work “On the importance of text analysis for stock market prediction” by Lee and MacCartney ( 2014 ) that shows, based on text, an improved predictability in the performance of a security. Intuitively, the cause of the stocks’ fluctuation can be the aggregated behavior of the stockholders, who will act based on news (Xing et al. 2018 ). Although the predicting models reported in the literature have not been able to profit in the long run, many theories and meaningful remarks have been made from the financial markets’ data (Xing et al. 2018 ). One of the biggest differences between market sentiment problems and linguistics ones is that the ladder has some guarantee of having some type of structures (Perry 2016 ). There are many models that have been proposed and used in the recent years, each with their positive and negative aspects. Specifically, overly complicated models generally have poor performance, while simpler linear models rely on strong hypotheses, for example, a Gaussian distribution, which does not always apply in real-world cases (Xing et al. 2018 ). Deep learning seems to be the most fit for this purpose since it has the ability to analyze a great amount of data that NLP needs to understand context and grammatical structures. In this paper, we investigate this scenario, exploring DL for forecasting the market sentiment using news headlines. We assume a basic linguistic framework for preprocessing (stopword, lowercasing, removing or numbers and other special characters), and we reject the common assumption that “positive financial sentiment” = “positive words” and vice versa. The reason is that we do not know if that is the case, and we want the model to learn freely by itself. Taking this assumption into consideration, we are going to test two scenarios, both based on the news published today: case A tries to forecast the movement of the Dow Jones Industrial Average (DJIA) in the next four individual days; case B focuses on time intervals from today to the next 4 days. We discuss the obtained results, and we conclude the paper with a road map for the future.
2 Background
2.1 deep learning.
Supervised ML models create mechanisms that can look at examples and produce generalizations (Goldberg 2017 ). Deep learning (DL) Footnote 1 is a function that imitates the mechanisms of the human brain for finding patterns. Since our case is a binary classification problem (does the DJIA go up or down?), to test our results we used both a binary cross-entropy loss function:
And an accuracy metric: Footnote 2
They are both useful in different ways since the first is used in the training phase, while accuracy is intuitive as long as the classes considered are balanced, like in our case. The basic structure of a neural network (NN) is the neuron: it receives the signal, decides whether to pass the information or not, and sends it to the next neuron. Mathematically, the neuron structure takes some input values × and their relative weights w which are both initialized randomly, and thanks to an “activation function”, Footnote 3 which was the real game-changer (Thanaki 2018 ), the neurons have the ability to spot non-linear behaviors, that is why they are often addressed as being “Universal Function Approximators”. Since searching over the set of all the possible functions is a difficult task, we need to restrict our scope of action using smaller sets, but by doing so, we added an “inductive bias” (b) that must be taken into account. The most commonly used function for this purpose has the form (Goldberg 2017 ):
The parameters Footnote 4 have the purpose to minimize the loss function over the training set and the validation set (Goldberg 2017 ). How can NN learn? Through an optimization method that, thanks to a first-order iterative algorithm called gradient descent, can minimize the error function of our model until a local minimum is reached. Footnote 5 Backpropagation is the central mechanism of the learning process which calculates the gradient of the loss function and, by making extensive use of the chain rule, distributes it back through the layers of the NN and adjusts the weights of the neurons for the next iteration. The learning rate used during backpropagation starts with a value of 0.001 and is based on the adaptive momentum estimation (Adam), a popular learning-rate optimization algorithm. Traditionally, the Softmax function is used for giving probability form to the output vector (Thanaki 2018 ) and that is what we used. We can think of the different neurons as “Lego Bricks” that we can use to create complex architectures (Goldberg 2017 ). In a feed-forward NN, the workflow is simple since the information only goes…forward (Goldberg 2017 ). However, when humans read a book, for example, they comprehend every section, sentence or word taking into account what they saw previously (Olah 2015 ); therefore, a feed-forward NN is not fit for our purposes because it cannot “remember”. Recurrent neural networks (RNN), on the other hand, can catch the sequential nature of the input and can be thought of as multiple copies of the same network, each passing a message to a successor (Olah 2015 ). A well-known drawback of standard RNN is the vanishing gradients’ problem that can be dramatically reduced using, as we did, a gating-based RNN architecture called long short-term memory Footnote 6 (LSTM).
2.2 NLP and vectorization
Natural language processing (NLP) is a field of artificial intelligence (AI) focused on finding interactions between computers and the human language. Linguistics can be a slippery field for humans, and its intrinsic ambiguity is what makes NLP even more problematic for machines (Millstein 2017 ) since complexity can appear on many levels: morphological, lexical, syntactic, or semantic. Data preprocessing is a crucial method used to simplify raw text data. In NLP, words are the features Footnote 7 used to find sentiment, based on their frequency in a database (Velay and Daniel 2018 ). The goal of the language model that is to assign probabilistic sentiment to sentences by trying to capture its context, but to do so, the so-called Markov assumption Footnote 8 is necessary. We use encoding for creating word-embeddings, which are tools used to vectorize words into feature-vectors that a NN can use (Millstein 2017 ). Word-embeddings are representations of documents where vectors with small distances represent words with closely related meanings. These structures allow us to perform math on texts. With the recent advances in DL, word-embeddings are formed with more accuracy, and they make it easier to compute semantic similarities (Xing et al. 2018 ). Unfortunately, distributional methods can fall victim of different corpus biases, which can range from cultural to thematic: a common saying is that “Words are similar if used in similar contexts,” but linguistics is more complicated than it looks. Footnote 9 Each model has its pros and cons (Velay and Daniel 2018 ), the difference stays in the user’s ability to have control over its dataset (Goldberg 2017 ).
3 Learning trading indicators on news
A trading indicator is a call for action to buy/sell an asset given a specific condition. When it comes to short-term market behaviors, we are trying to profit on the investors’ “gut-feeling,” but since this phenomenon is something that cannot be unequivocally defined, we must reduce human judgment as much as possible by letting the algorithm learn directly. As shown in Fig. 1 , we will start by seeing the chosen dataset. Right after, we will analyze which preprocessing operations have been implemented to ease the computational effort for the model. Then we will see all the components of the DL model put in place and ultimately we will present the results with a real-case scenario.
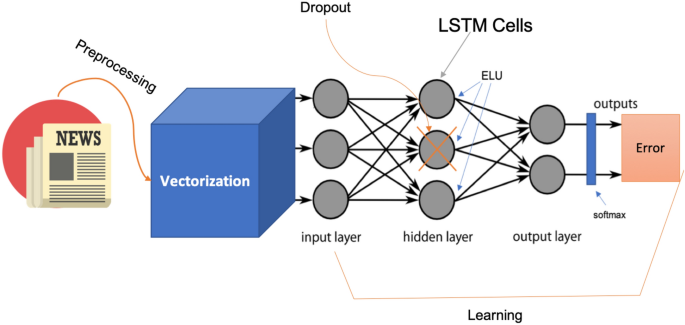
Model structure
When working with neural networks, we encounter some limitations that might affect our results (Thanaki 2018 ): the dimension of the data set, our computing-power availability and the complexity of our model. Just like in the work of Vargas et al. ( 2017 ), the dataset is based on news headlines; specifically from the DJIA Database which comes from Kaggle, which contains 25 daily news with economic content from 2008 to 2016 scraped by the author from the most upvoted by the community on Reddit WorldNews: https://www.kaggle.com/aaron7sun/stocknews/home .
Given the fact that the database stretches over almost a decade and contains, for each day considered, a conspicuous amount of news with inherent economic content, we decided that it represents a plausible research instrument. The database was labeled based on whether the DJIA increased or decreased over each time step considered.
4 The studied model
The focus is on aggregate market indicators and two cases are considered, namely cases A and B as shown, respectively, in Figs. 2 and 3 .
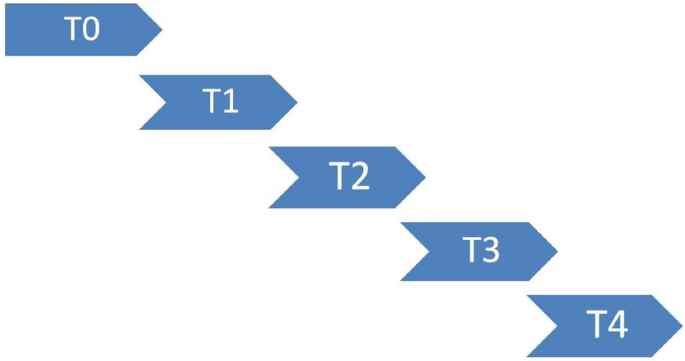
The T0 event, common in both instances, analyzes if, based on the news published today, today’s Adjusted closing price is higher than today’s opening price. While, based on the news published today, case A tries to forecast the movement of the DJIA in individual days, case B focuses on time intervals. After defining these market indicators, the preprocessing phase is crucial to reduce the number of independent variables, namely the word tokens, that the algorithms need to learn. At this stage, the news strings need to be merged to represent the general market indicator, from which stopwords, numbers and special elements (e.g. hashtags, etc.) were removed. In addition, every word has been lowercased and only the 3000 most frequent words have been taken into consideration and vectorized into a sequence of numbers thanks to a tokenizer. Furthermore, the labels are transformed into a categorical matrix with as many columns as there are classes, for our case two. The NN Footnote 10 presented in Fig. 1 starts with an embedding layer, which is the input of the model, whose job is to receive the two-dimensional matrix and output a three-dimensional one, which is randomly initialized with a uniform distribution. Then this 3D-matrix is sent to the hidden layer made of LSTM neurons whose weights are randomly initialized following a Glorot Uniform Initialization, which uses an ELU activation function and dropout. Finally, the output layer is composed of two dense neurons and followed by a softmax activation function. Once the model’s structure has been determined, it needs to be appropriately compiled using the ADAM optimizer for backpropagation, which provides a flexible learning rate to the model.
As shown in Fig. 4 , the database is then divided into training and validation set with an 80/20 split and evaluated by the binary cross-entropy and accuracy metrics that we previously discussed. Moreover, the training set is split into small pieces called batches (which, for instance, have a dimension of 64 for the T0 case) that are given to the computer one by one for 25 iterations in the training set and two epochs to ease the computational effort when updating the weights.
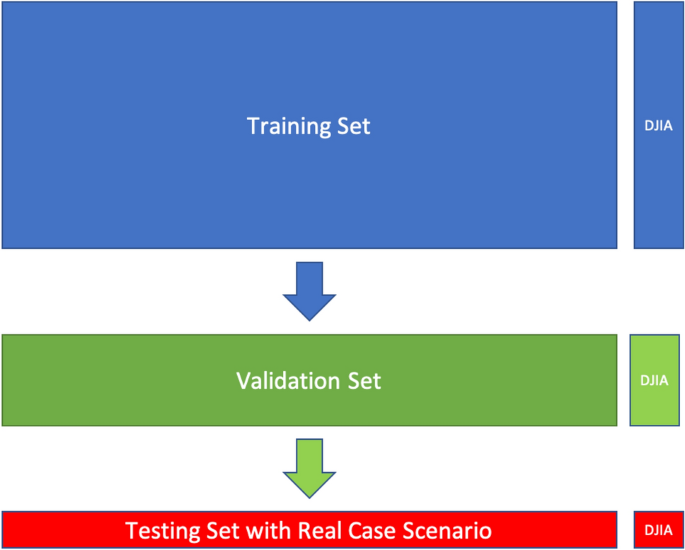
Structure of training, validation and testing sets with DJIA labels
Table 1 shows the level of accuracy obtained in this experiment relative to the validation sets:
Consistent with previous studies (Velay and Daniel 2018 ), we immediately notice that the accuracy is particularly low since, in both cases, our peaks stick around 58%, which is slightly higher than the flip of a coin.
Besides, in both versions of the model, the highest accuracy appears in the T0 case, behavior that suggests that forecasting attempts within shorter time periods should be preferred, confirming existing literature on the topic (Souma et al. 2019 ; Sohangir et al. 2018 ). Nevertheless, we can notice the tendency that the accuracy gradually decreases in case A, while case B shows a less evident decrease that ends with a final increase in T4. Given the low accuracy level, we asked ourselves: How would this model behave in a practical application? Thus, we chose specific news related to major political events that, from our perspective, might have affected the global markets. For this reason, the first testing case looks at major political events that might have caused relevant shifts in the balance of the world:
Start of Trump’s formal impeachment inquiry.
Large crowds of protesters gathered in Hong Kong.
Boris Johnson becomes prime minister.
Protests for George Floyd’s murder explode.
COVID-19 was declared a global pandemic by the WHO.
In the second testing category, we decided to look closer at Trump’s presidency. The events chosen were:
The Summit in Singapore between Trump and Kim Jong-Un.
Trump signs tariffs on steel and aluminum.
Trump formally announced US withdrawal from the Paris Agreements.
Trump signs a big tax cut that was beneficial to big corporations.
Trump starts the government shutdown to build the wall.
To be consistent with the training and validation set, we manually retrieved from the “News” section of Google 25 news focused on the date of every major event listed above. By comparing each prediction with how the DJIA behaved, we notice that the results are not significant because the results obtained did not show any consistent pattern. Therefore, we agree with Arora et al. ( 2019 ) when stating that financial information is extremely unpredictable and that the task of predicting stock movements remains open. For this reason, we thought it could be interesting to keep studying how such a model would behave over longer periods of time by feeding it with more data in the training phase. In fact, for image recognition experiments, DL learning is known for having better performance the more data it sees; therefore, we will follow the same path for financial forecasting. Thus, we decided to extend the original data set until August 2020 and test the same scenario using the same methodology. We retrieved 25 most upvoted daily news from the sub-Reddit/r/worldnews/, just like the author originally did, thanks to the data submissions available in the Pushshift data collections: https://www.files.pushshift.io/reddit/submissions/ .
To keep our results comparable, we kept the same NN structure as in the previous case. The results of the experiment using this extended data set in reported in Table 2 .
On the one hand, for the extended case A, the outcome is mixed and there is no added benefit to our initial model. On the extended case B, on the other hand, we notice an even worse forecasting performance. In addition, as in the previous test for individual news, the results obtained did not show any relevant pattern and are not significant. Unlike in the image-recognition field, which is known for improving its performance whenever a more extensive data-set is fed to the deep learning model, for our financial forecasting case we did not obtain the same performance improvement. Why despite increasing the dataset did we get worse results? We analyzed the datasets for the T0 case and the extended T0 case deeper.
The original dataset T0 gives us 2267 trading dates for the DJIA and the DJIA closed higher than the open on 1212 times and closed lower on 1055 days. This means that an algorithm which would guess “DJIA increase” every single day which would result in
True positives: 1212
True negatives: 0
False positives: 1055
False negatives: 0
At the same time, the extended T0 case shows us that the DJIA went up 1625 days and down 1367 times. Applying again an algorithm which would guess “DJIA increase” every single day would have these characteristics:
True positives: 1625
False positives: 1367
Therefore, we would have an accuracy of around 53% for the original T0 case and of around 54% for the extended T0 case, values that are both extremely close to the results we obtained through an optimized DL model. Thus, we presume that the algorithm did not learn anything other than the bias in the data. Further paths worth exploring would be testing DL models with different databases, that could be:
Using news more focused on a specific security or newspaper.
Testing cases right after the news comes out.
Exploiting a dataset with more news per day.
Exploring a wider variety of NLP methods in the preprocessing phase.
By removing the assumptions “positive financial sentiment” = “positive words”, forecasting sentiment becomes particularly difficult and an elaborated model such as DL the indicator appears weak (Sohangir et al. 2018 ). In fact, the closer we get into situations where human discretion is involved, the more unstable a model becomes (Goldfarb et al. 2018 ).
6 Discussion and future roadmap
Forecasting consists in grabbing data that we have to generate new information: this is a field that economics has been studying for years, and perhaps by combining the old logic of decision theory and DL methods, we may have a better understanding from a different perspective (Goldfarb et al. 2018 ). The benefit of DL is that it does not require lots of human effort to effectively provide outputs that were previously considered impossible and a human exclusive. Indeed, AI did not give us more intelligence, but more prediction capacity.
In fact, the innovation of this technology is not the math behind it that existed for decades, but the combination of this knowledge with the Big Data environments that we can access nowadays (Goldfarb et al. 2018 ). Economics says that by eliminating every obstacle to trading leads to market efficiency. Is it always true? Would the introduction of market agents using trading strategies based on DL and sentiment analysis increase market efficiency? The answer is “it depends”. By introducing a new investing technology we could indeed increase market liquidity and therefore stock markets’ efficiency as a whole. However, the stock market is a complex ecology of interacting players, all with their own strategies and things can go wrong very fast. Let us take a step back and analyze the situation with a few examples from the past that might help us better understand what to expect tomorrow (Buchanan 2012 ):
First, the belief that financial innovations such as derivatives could help us reach more market stability and efficiency dominated the last decades (Buchanan 2012 ). However, those instruments, passed a threshold, profoundly endangered the whole system in 2008 (Buchanan 2012 ).
Second, the innovation of High-Frequency Trading (HFT) has improved the stock market as well by reducing trading costs, enhance liquidity, making markets faster and more reactive in calm times, but they do the exact opposite in troubled ones; exactly when the market would need it the most (Buchanan 2012 ).
Third, hedge funds typically borrow money to attract investors and increase their profits. However, right before the “Quant meltdown” of August 2007 it was clear the strategies used became too similar, causing their margins to decrease. Therefore, to keep being appealing to investors, managers were slowly forced to increase leverage until it was unsustainable and everything collapsed (Buchanan 2012 ).
The belief that the world is becoming more efficient and stable than ever thanks to financial innovation (Buchanan 2012 ) can be misleading. Then why do we always seem surprised when such crises appear? (Buchanan 2012 ) It is not a coincidence that excessive efficiency can compromise stability (Buchanan 2012 ). Efficiency means doing more with less, while stability implies the opposite: some extra room to absorb a hit. Given the current hype for AI, these interconnected markets will have access to new ways to invest at incredible speed. In such a context, trading strategies based on sentiment may be particularly prone to the “herd behavior” assuming that these algorithms must train on datasets containing lots of news that may turn out to be extremely similar (Buchanan 2012 ). In this context, the hypothesis of a “splash crash” ranging across many asset classes does not seem impossible (Buchanan 2012 ). The global financial crisis has revealed the need to drastically rethink how we regulate the financial system to get ready for when the next recession will strike (Buchanan 2012 ). Innovation cannot be stopped, but acknowledging its limitations can help us find the best ways to reduce its weaknesses. The fight is rapidly shifting from humans towards machines, but they are playing with real businesses and people, and we must counteract effectively and rapidly in that regard (Buchanan 2012 ).
7 Conclusion
In conclusion, by removing the assumptions “positive financial sentiment” = “positive words”, financial forecasting becomes particularly difficult and even a DL model does not show better results than the flip of a coin for any of the cases or time-intervals studied. We acknowledge that there are many other paths that could potentially improve our results, but increasing the database size over a longer period of time, common practice in image-recognition problems, does not look like one of them. Despite that, we believe that deep learning methods could potentially be the cause of a relevant danger, due to its proneness towards the “herd behavior”, for the financial system as a whole and thus must be handled carefully.
Also known as a deep neural network or deep neural learning.
Where TP means’True Positives’, TN means’True Negatives’, FP means’False Positive’ and FN means’False negatives’.
The Activation Function chosen is the Exponential Linear Unit (ELU) since it shows better performance than its Rectified Linear Unit (ReLU) predecessor Hochreiter et al. ( 2016 ) by reducing the vanishing gradient problem and by accepting negative values.
Where x is the input, W are the weights, b is the inductive bias. Right after are explained the dimensions of the vectors that have been enumerated. These are all cases that we will go over later on but, for example: x in our case is a vector whose dimension represents a matrix whose dimensions are represented by the product between the maximum length of the sentences and the random initialization created using the embedding layer for each batch. The notation named ‘ d out ’ represents the dimension of the following layer that will receive the output of the current one.
For the sake of completeness, it does not tell us the minimum error immediately but shows us the proper direction we need to follow Thanaki ( 2018 ).
To avoid overfitting of the LSTM structure, we adopted a common regularization method called "Dropout".
Features in a model based on AI are individual measurable properties used as input to obtain an output Thanaki ( 2018 ); therefore, any attribute can be a feature as long as it is measurable and useful.
Which states that the probability of the next word is calculated by looking at the previous one.
The clearest example is polysemy.
The number of neurons, dropout and epochs named below represent the common T0 case, but have been fine-tuned for each other case using the "babysitting method".
Arora A, Datta A, Ding V (2019) Using news titles and financial features to predict intraday movements of the DJIA. https://www.web.stanford.edu/class/archive/cs/cs224n/cs224n.1194/reports/custom/15744462.pdf . Accessed 30 Sept 2020
Brown ED (2016) Analysis of Twitter messages for sentiment and insight for useinstockmarketdecisionmaking. Amazon Digital Services LLC, Seattle
Google Scholar
Buchanan M (2012) Forecast: what physics, meteorology and natural sciences can tell us about economics. Bloomsbury, London
Cambria E (2016) Affective computing and sentiment analysis. IEEE Intell Syst 31(2):102–107
Article Google Scholar
Goldberg Y (2017) Neural network methods for natural language processing. Morgan Claypool publishers, San Rafael
Book Google Scholar
Goldfarb A, Agrawal A, Gans J (2018) Prediction machines: the simple economics of artificial intelligence. Harvard Business Review Press, Cambridge
Hochreiter S, Clevert DA et al (2016) Fast and accurate deep network learning by exponential linear units. https://arxiv.org/abs/1511.07289
Lee S, MacCartney J (2014) On the importance of text analysis for stock price prediction. In: Language resources and evaluation conference (LREC)
Millstein F (2017) Natural language processing with python: natural language processing using NLTK. Packt Publishing, Birmingham
Olah C (2015) Understanding LSTM networks. http://www.colah.github.io/posts/2015-08-Understanding-LSTMs/ . Accessed 30 Sept 2020
Perry T (2016) Deep learning the stock market. https://www.medium.com/@TalPerry/deep-learning-the-stock-market-df853d139e02 . Accessed 30 Sept 2020
Ruiz-Martínez JM, Valencia-García R, García-Sánchez F (2012) Semantic-based sentiment analysis in financial news. In: Joint Proceedings of the 1st International Workshop on Finance and Economics on the Semantic Web (FEOSW 2012), 5th International Workshop on REsource Discovery (RED 2012) and 7th International Workshop on Semantic Business Process Management (SBPM 2012) in conjunction with 9th Extended Semantic Web Conference (ESWC 2012). CEUR. CEUR Workshop Proceedings. 862:38–51. http://ceur-ws.org/Vol-862/FEOSWp4.pdf . Accessed 30 Sept 2020
Sohangir S, Wang D et al (2018) Big data: deep learning for financial sentiment analysis. J Big Data. https://doi.org/10.1186/s40537-017-0111-6
Souma W, Vodenska I, Aoyama H (2019) Enhanced news sentiment analysis using deep learning methods. J Comput Soc Sci 2:33–46
Thanaki J (2018) Python natural language processing: advanced machine learning and deep learning techniques for natural language processing. CreateSpace Independent Publishing Platform, South Carolina
Vargas MR, de Lima BSLP, Evsukoff AG (2017) Deep learning for stock market prediction from financial news articles. In: 2017 IEEE international conference on computational intelligence and virtual environments for measurement systems and applications (CIVEMSA), pp 60–65
Velay M, Daniel F (2018) Using NLP on news headlines to predict index trends. Lusis https://arxiv.org/abs/1806.09533
Xing FZ, Cambria E, Welsch RE (2018) Natural language based financial forecasting: a survey. Artif Intell Rev 50:49–73. https://doi.org/10.1007/s10462-017-9588-9
Download references
Acknowledgements
This paper has been inspired from my master thesis that can be found here: https://www.github.com/Mattia9494/Sentiment-analysis-with-AI .
Open access funding provided by Alma Mater Studiorum - Università di Bologna within the CRUI-CARE Agreement.
Author information
Authors and affiliations.
University of Bologna, Bologna, Italy
Mattia Vicari
Department of Computer Science and Engineering, University of Bologna, Bologna, Italy
Mauro Gaspari
You can also search for this author in PubMed Google Scholar
Corresponding author
Correspondence to Mattia Vicari .
Additional information
Publisher's note.
Springer Nature remains neutral with regard to jurisdictional claims in published maps and institutional affiliations.
Rights and permissions
Open Access This article is licensed under a Creative Commons Attribution 4.0 International License, which permits use, sharing, adaptation, distribution and reproduction in any medium or format, as long as you give appropriate credit to the original author(s) and the source, provide a link to the Creative Commons licence, and indicate if changes were made. The images or other third party material in this article are included in the article's Creative Commons licence, unless indicated otherwise in a credit line to the material. If material is not included in the article's Creative Commons licence and your intended use is not permitted by statutory regulation or exceeds the permitted use, you will need to obtain permission directly from the copyright holder. To view a copy of this licence, visit http://creativecommons.org/licenses/by/4.0/ .
Reprints and permissions
About this article
Vicari, M., Gaspari, M. Analysis of news sentiments using natural language processing and deep learning. AI & Soc 36 , 931–937 (2021). https://doi.org/10.1007/s00146-020-01111-x
Download citation
Received : 29 October 2020
Accepted : 02 November 2020
Published : 30 November 2020
Issue Date : September 2021
DOI : https://doi.org/10.1007/s00146-020-01111-x
Share this article
Anyone you share the following link with will be able to read this content:
Sorry, a shareable link is not currently available for this article.
Provided by the Springer Nature SharedIt content-sharing initiative
- Deep learning
- Machine learning
- Natural language processing
- Trading signals
- Sentiment analysis
- Trading strategies
- Find a journal
- Publish with us
- Track your research
SYSTEMATIC REVIEW article
This article is part of the research topic.
Reviews in Gastroenterology 2023
Electrogastrography Measurement Systems and Analysis Methods Used in Clinical Practice and Research: Comprehensive Review Provisionally Accepted
- 1 VSB-Technical University of Ostrava, Czechia
The final, formatted version of the article will be published soon.
Electrogastrography (EGG) is a non-invasive method with high diagnostic potential for the prevention of gastroenterological pathologies in clinical practice. In this paper, a review of the measurement systems, procedures, and methods of analysis used in electrogastrography is presented. A critical review of historical and current literature is conducted, focusing on electrode placement, measurement apparatus, measurement procedures, and time-frequency domain methods of filtration and analysis of the non-invasively measured electrical activity of the stomach.As a result a total of 129 relevant articles with primary aim on experimental diet were reviewed in this study. Scopus, PubMed and Web of Science databases were used to search for articles in English language, according to the specific query and using PRISMA method. The research topic of electrogastrography has been continuously growing in popularity since the first measurement by professor Alvarez 100 years ago and there are many researchers and companies interested in EGG nowadays. Measurement apparatus and procedures are still being developed in both commercial and research settings. There are plenty variable electrode layouts, ranging from minimal numbers of electrodes for ambulatory measurements to very high numbers of electrodes for spatial measurements. Most authors used in their research anatomically approximated layout with 2 active electrodes in bipolar connection and commercial electrogastrograph with sampling rate of 2 or 4 Hz. Test subjects were usually healthy adults and diet was controlled. However, evaluation methods are being developed at a slower pace and usually the signals are classified only based on dominant frequency. The main review contributions include the overview of spectrum of measurement systems and procedures for electrogastrography developed by many authors, but a firm medical standard has not yet been defined. Therefore, it is not possible to use this method in clinical practice for objective diagnosis.
Keywords: electrogastrography, non-invasive method, Measurement systems, Electrode placement, Measurement apparatus, Signal processing
Received: 19 Jan 2024; Accepted: 03 Jun 2024.
Copyright: © 2024 Oczka, Augustynek, Penhaker and Kubicek. This is an open-access article distributed under the terms of the Creative Commons Attribution License (CC BY) . The use, distribution or reproduction in other forums is permitted, provided the original author(s) or licensor are credited and that the original publication in this journal is cited, in accordance with accepted academic practice. No use, distribution or reproduction is permitted which does not comply with these terms.
* Correspondence: Dr. Jan Kubicek, VSB-Technical University of Ostrava, Ostrava, 708 33, Moravian-Silesian Region, Czechia
People also looked at
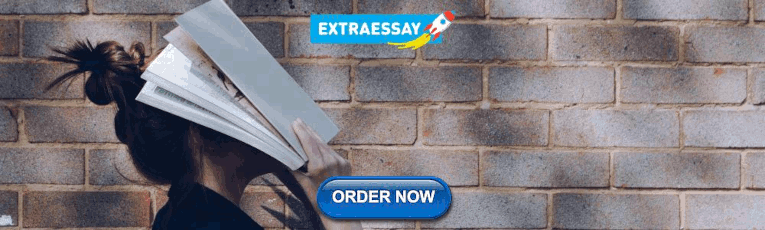
IMAGES
VIDEO
COMMENTS
By linking language analysis to current trends in artificial intelligence, such as sentiment analysis or intent recognition, the field becomes more relatable and appealing to a wider audience [6]. 6.
The Journal of Linguistics is an open access journal that is concerned with all branches of theoretical linguistics. Preference is given to original Research Articles which present analyses or generalisations based on sound empirical work, which results in making a clear contribution to current debate in theoretical linguistics.Papers should be accessible to non-specialist linguists with an ...
RESEARCH METHODS IN. LINGUISTICS: AN O VERVIEW. Snejana Obey d. Depart ment of Engli sh Studie s, Konstant in Preslav sky Unive rsity of S humen, B ulgaria. Abstract: The paper o ers a short ...
Explore the latest full-text research PDFs, articles, conference papers, preprints and more on LINGUISTIC ANALYSIS. Find methods information, sources, references or conduct a literature review on ...
In a recent paper, King and Mackey call ... New to this edition are chapters on mixed methods research and analysis, narrative inquiry, ethics in applied linguistics research, and developing a research project, as well as chapters in areas of research which include researching language learning strategies, young learners, teachers' beliefs ...
The English language (including Old English and Middle English) has existed for a small fraction of human history, and approximately 5% of people today speak English as a first language, yet English speakers probably account for more than 99% of language-analysis research published in psychology journals (Lewis, 2009).
2 Ethics in linguistic research Penelope Eckert 11 3 Judgment data Carson T. Schütze and Jon Sprouse 27 4 Fieldwork for language description Shobhana Chelliah 51 ... 18 Constructing and supporting a linguistic analysis John Beavers and Peter Sells 397 19 Modeling in the language sciences Willem Zuidema and Bart de Boer 422 20 Variation analysis
For most research in cognitive and social psychology, psycholinguistics, and cognitive science at large, text analysis has primarily focused on a very small and very specific part of human language, that of formal, written English from a WEIRD (Western, Educated, Industrialized, Rich and Democratic) population (Blasi et al., 2022; Henrich et al., 2010; Kučera & Mehl, 2022; Levisen, 2019).
This book reconsiders the classic topics of linguistic analysis and reflects on universal aspects of language from a typological and comparative perspective. The aim is to show the crucial interactions which occur at the different levels of grammar (phonology, morphology, lexicon, syntax and pragmatics), illustrating their various roles in the structural organization of the sentence and ...
The application of full-text analysis to understand the relationship between linguistic characteristics and article views and downloads has not been thoroughly investigated. To address this research gap, full-text analysis is used to explore linguistic characteristics of highly browsed and downloaded papers.
Welcome to Linguistic Analysis. A peer-reviewed research journal publishing articles in formal phonology, morphology, syntax and semantics. The journal has been in continuous publication since 1976. ... Volume 43, 3-4, will be the last issue of the journal published in paper. Beginning with volume 44, 1-2, all issues will be available in ...
Besides rhetorical structure analysis, linguistic realizations in RA abstracts including tense and voice are also important and have been increasingly focused on by several research studies because they ... Santos M. (1996). The text organization of research papers abstracts in applied linguistics. Text, 16, 481-499. Google Scholar. Suntara W ...
Linguistics, Medicine. Frontiers in Education. 2022. TLDR. This corpus-based cross-disciplinary study investigated the syntactic structures and rhetorical combinations of 200 research article titles in two disciplines, namely, medicine and applied linguistics, and confirmed the verbal structures of single-unit and the rhetorical combination of ...
linguistics = 50,003) of all citations acquired by Asian 'language and ' research was internationally cited. Among the 13 target countries, a comparison of the raw citation counts indicates ...
Abstract. This chapter offers an introduction to corpus linguistics as a methodology for studying language, literature, and other fields in the humanities. It defines corpus linguistics, explores ...
The present study provides a systematic review of prosody research in linguistic journals through a bibliometric analysis. Using the bibliographic data from 2001 to 2021 in key linguistic journals ...
JOURNAL OF LANGUAGE AND LINGUISTIC STUDIES ISSN: 1305-578X Journal of Language and Linguistic Studies, 12(2), 117-134; 2016 ELT research in Turkey: A content analysis of selected features of published articles Oktay Yağız a *, Burcu Aydınb, Ahmet Selçuk Akdemir c a Ataturk University, Erzurum,Turkey b Adnan Menderes University,Aydın,Turkey
Research on COVID-19 has drawn the attention of scholars around the world since the outbreak of the pandemic. Several literature reviews of research topics and themes based on scientometric indicators or bibliometric analyses have already been conducted. However, topics and themes in linguistic-specific research on COVID-19 remain under-studied.
Structured science summaries or research contributions using properties or dimensions beyond traditional keywords enhance science findability. Current methods, such as those used by the Open Research Knowledge Graph (ORKG), involve manually curating properties to describe research papers' contributions in a structured manner, but this is labor-intensive and inconsistent among human domain ...
In order to fill this void, in this paper, we provide the way for an integration of linguistic methods into organizational research by revealing the contributions of natural language processing ...
This research used the theory semantic of figurative language from Perrine (2018) to categorize the utterance that appeared in the data source into the function and reason of figurative language. A qualitative research design was employed in conducting this study followed by document analysis and observation as the instruments.
We aimed to examine the differences in articles, peer review and editorial processes in Medical and Health Sciences vs. Social Sciences. Our data source was Open Research Central (ORC) portal, which hosts several journal platforms for post-publication peer review, allowing the analysis of articles from their submission, regardless of the publishing outcome. The study sample included 51 ...
Financial Statement Analysis with Large Language Models Alex G. Kim , Maximilian Muhn , Valeri V. Nikolaev GPT outperforms financial analysts in predicting companies' earnings changes, especially when analysts struggle, with accuracy comparable to state-of-the-art machine learning models.
1. Linguistic theories, approaches and methods. Hans-Jörg Schmid, München. 1. Introduction: General aims of linguistic theorizing. According to the sociologist Kurt Lewin (1952: 169), "there ...
What We Do. CERT experts are a diverse group of researchers, software engineers, security analysts, and digital intelligence specialists working together to research security vulnerabilities in software products, contribute to long-term changes in networked systems, and develop cutting-edge information and training to improve the practice of cybersecurity.
Stock forecasting through NLP is at the crossroad between linguistics, machine learning, and behavioral finance (Xing et al. 2018).One of the main NLP techniques applied on financial forecasting is sentiment analysis (Cambria 2016) which concerns the interpretation and classification of emotions within different sources of text data.It is a research area revived in the last decade due to the ...
Electrogastrography (EGG) is a non-invasive method with high diagnostic potential for the prevention of gastroenterological pathologies in clinical practice. In this paper, a review of the measurement systems, procedures, and methods of analysis used in electrogastrography is presented. A critical review of historical and current literature is conducted, focusing on electrode placement ...
Patricia L. Dunmire*. Kent State University. Abstract. This essay overviews the body of research known as political discourse analysis (PDA). I begin by. situating this work within the linguistic ...