Thank you for visiting nature.com. You are using a browser version with limited support for CSS. To obtain the best experience, we recommend you use a more up to date browser (or turn off compatibility mode in Internet Explorer). In the meantime, to ensure continued support, we are displaying the site without styles and JavaScript.
- View all journals
- My Account Login
- Explore content
- About the journal
- Publish with us
- Sign up for alerts
- Open access
- Published: 29 September 2020
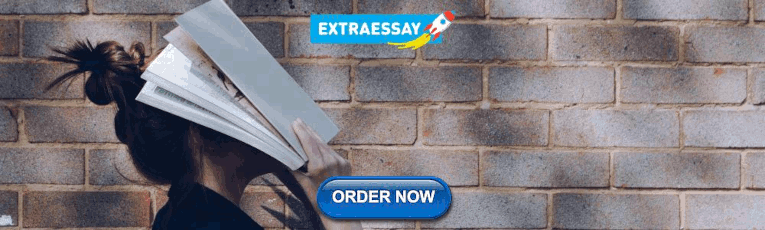
Machine learning prediction in cardiovascular diseases: a meta-analysis
- Chayakrit Krittanawong 1 , 9 ,
- Hafeez Ul Hassan Virk 2 ,
- Sripal Bangalore 3 ,
- Zhen Wang 4 , 5 ,
- Kipp W. Johnson 6 ,
- Rachel Pinotti 7 ,
- HongJu Zhang 8 ,
- Scott Kaplin 9 ,
- Bharat Narasimhan 9 ,
- Takeshi Kitai 10 ,
- Usman Baber 9 ,
- Jonathan L. Halperin 9 &
- W. H. Wilson Tang 10
Scientific Reports volume 10 , Article number: 16057 ( 2020 ) Cite this article
51k Accesses
193 Citations
29 Altmetric
Metrics details
- Cardiovascular diseases
- Computational biology and bioinformatics
- Machine learning
Several machine learning (ML) algorithms have been increasingly utilized for cardiovascular disease prediction. We aim to assess and summarize the overall predictive ability of ML algorithms in cardiovascular diseases. A comprehensive search strategy was designed and executed within the MEDLINE, Embase, and Scopus databases from database inception through March 15, 2019. The primary outcome was a composite of the predictive ability of ML algorithms of coronary artery disease, heart failure, stroke, and cardiac arrhythmias. Of 344 total studies identified, 103 cohorts, with a total of 3,377,318 individuals, met our inclusion criteria. For the prediction of coronary artery disease, boosting algorithms had a pooled area under the curve (AUC) of 0.88 (95% CI 0.84–0.91), and custom-built algorithms had a pooled AUC of 0.93 (95% CI 0.85–0.97). For the prediction of stroke, support vector machine (SVM) algorithms had a pooled AUC of 0.92 (95% CI 0.81–0.97), boosting algorithms had a pooled AUC of 0.91 (95% CI 0.81–0.96), and convolutional neural network (CNN) algorithms had a pooled AUC of 0.90 (95% CI 0.83–0.95). Although inadequate studies for each algorithm for meta-analytic methodology for both heart failure and cardiac arrhythmias because the confidence intervals overlap between different methods, showing no difference, SVM may outperform other algorithms in these areas. The predictive ability of ML algorithms in cardiovascular diseases is promising, particularly SVM and boosting algorithms. However, there is heterogeneity among ML algorithms in terms of multiple parameters. This information may assist clinicians in how to interpret data and implement optimal algorithms for their dataset.
Similar content being viewed by others
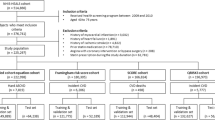
Pre-existing and machine learning-based models for cardiovascular risk prediction
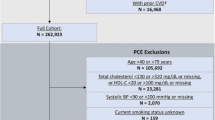
Machine learning and atherosclerotic cardiovascular disease risk prediction in a multi-ethnic population
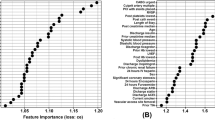
Interpretable machine learning models for predicting in-hospital and 30 days adverse events in acute coronary syndrome patients in Kuwait
Introduction.
Machine learning (ML) is a branch of artificial intelligence (AI) that is increasingly utilized within the field of cardiovascular medicine. It is essentially how computers make sense of data and decide or classify a task with or without human supervision. The conceptual framework of ML is based on models that receive input data (e.g., images or text) and through a combination of mathematical optimization and statistical analysis predict outcomes (e.g., favorable, unfavorable, or neutral). Several ML algorithms have been applied to daily activities. As an example, a common ML algorithm designated as SVM can recognize non-linear patterns for use in facial recognition, handwriting interpretation, or detection of fraudulent credit card transactions 1 , 2 . So-called boosting algorithms used for prediction and classification have been applied to the identification and processing of spam email. Another algorithm, denoted random forest (RF), can facilitate decisions by averaging several nodes. While convolutional neural network (CNN) processing, combines several layers and apples to image classification and segmentation 3 , 4 , 5 . We have previously described technical details of each of these algorithms 6 , 7 , 8 , but no consensus has emerged to guide the selection of specific algorithms for clinical application within the field of cardiovascular medicine. Although selecting optimal algorithms for research questions and reproducing algorithms in different clinical datasets is feasible, the clinical interpretation and judgement for implementing algorithms are very challenging. A deep understanding of statistical and clinical knowledge in ML practitioners is also a challenge. Most ML studies reported a discrimination measure such as the area under an ROC curve (AUC), instead of p values. Most importantly, an acceptable cutoff for AUC to be used in clinical practice, interpretation of the cutoff, and the appropriate/best algorithms to be applied in cardiovascular datasets remain to be evaluated. We previously proposed the methodology to conduct ML research in medicine 6 . Systematic review and meta-analysis, the foundation of modern evidence-based medicine, have to be performed in order to evaluate the existing ML algorithm in cardiovascular disease prediction. Here, we performed the first systematic review and meta-analysis of ML research over a million patients in cardiovascular diseases.
This study is reported in accordance with the Preferred Reporting Information for Systematic Reviews and Meta-Analysis (PRISMA) recommendations. Ethical approval was not required for this study.
Search strategy
A comprehensive search strategy was designed and executed within the MEDLINE, Embase, and Scopus databases from database inception through March 15, 2019. One investigator (R.P.) designed and conducted the search strategy using input from the study’s principal investigator (C.K.). Controlled vocabulary, supplemented with keywords, was used to search for studies of ML algorithms and coronary heart disease, stroke, heart failure, and cardiac arrhythmias. The detailed strategy is available from the reprint author. The full search strategies can be found in the supplementary documentation.
Study selection
Search results were exported from all databases and imported into Covidence 9 , an online systematic review tool, by one investigator (R.P.). Duplicates were identified and removed using Covidence's automated de-duplication functionality. The de-duplicated set of results was screened independently by two reviewers (C.K. and H.V.) in two successive rounds to identify studies that met the pre-specified eligibility criteria. In the initial screening, two investigators (C.K. and H.V.) independently examined the titles and abstracts of the records retrieved from the search via the Covidence portal and used a standard extraction form. Conflicts were resolved through consensus and reviewed by other investigators. We included abstracts with sufficient evaluation data, including methodology, the definition of outcomes, and an appropriate evaluation matrix. Studies without any kind of validation (external validation or internal validation) were excluded. We excluded reviews, editorials, non-human studies, letters without sufficient data.
Data extraction
We extracted the following information, if possible, from each study: authors, year of publication, study name, test types, testing indications, analytic models, number of patients, endpoints (CAD, AMI, stroke, heart failure, and cardiac arrhythmias), and performance measures ((AUC, sensitivity, specificity, positive cases (the number of patients who used the AI and were positively diagnosed with the disease), negative cases (the number of patients who used the AI and were negative with the AI test), true positives, false positives, true negatives, and false negatives)). CAD was defined as coronary artery stenosis > 70% using angiography or FFR-based significance. Cardiac arrhythmias included studies involving bradyarrhythmias, tachyarrhythmias, atrial, and ventricular arrhythmias. Data extraction was conducted independently by at least two investigators for each paper. Extracted data were compared and reconciled through consensus. In case studies which did not report positive and negative cases, we manually calculated by standard formulae using statistics available in the manuscripts or provided by the authors. We contacted the authors if the data of interest were not reported in the manuscripts or abstracts. The order of contact originated with the corresponding author, followed by the first author, and then the last author. If we were unable to contact the authors as specified above, the associated studies were excluded from the meta-analysis (but still included it in the systematic review). We also excluded manuscripts or abstracts without sufficient evaluation data after contacting the authors.
Quality assessment
We created the proposed guidance quality assessment of clinical ML research based on our previous recommendation (Table 1 ) 6 . Two investigators (C.K. and H.V.) independently assessed the quality of each ML study by using our proposed guideline to report ML in medical literature (Supplementary Table S1 ). We resolved disagreements through discussion amongst the primary investigators or by involving additional investigators to adjudicate and establish a consensus. We scored study quality as low (0–2), moderate (2.5–5), and high quality (5.5–8) as clinical ML research.
Statistical analysis
We used symmetrical, hierarchical, summary receiver operating characteristic (HSROC) models to jointly estimate sensitivity, specificity, and AUC 10 . \({Sen}_{i}\) and \({Spc}_{i}\) denote the sensitivity and specificity of the i th study. \({\sigma }_{Sen}^{2}\) is the variance of \({\mu }_{Sen}\) and \({\sigma }_{Spc}^{2}\) is the variance of \({\mu }_{spc}\) .
The HSROC model for study i fits the following
\({\pi }_{i1}\) = \({Sen}_{i}\) and \({\pi }_{i0}\) =1- \({Spc}_{i}\) . \({X}_{ij}=-\frac{1}{2}\) when no disease and \({X}_{ij}=\frac{1}{2}\) for those with disease. And \({\theta }_{i}\) and \({\alpha }_{i}\) follow normal distribution.
We conducted subgroup analyses stratified by ML algorithms. We assessed the performances of a subgroup-specific and statistical test of interaction among subgroups. We performed all statistical analyses using OpenMetaAnalyst for 64-bit (Brown University), R version 3.2.3 (Metafor and Phia packages), and Stata version 16.1 (Stata Corp, College Station, Texas). The meta-analysis has been reported in accordance with the Meta-analysis of Observational Studies in Epidemiology guidelines (MOOSE) 11 .
Study search
The database searches between 1966 and March 15, 2019, yielded 15,025 results. 3,716 duplicates were removed by algorithms. After the screening process, we selected 344 articles for full-text review. After full text and supplementary review, we excluded 289 studies due to insufficient data to perform meta-analytic approaches despite contacting corresponding authors. Overall, 103 cohorts (55 studies) met our inclusion criteria. The disposition of studies excluded after the full-text review is shown in Fig. 1 .
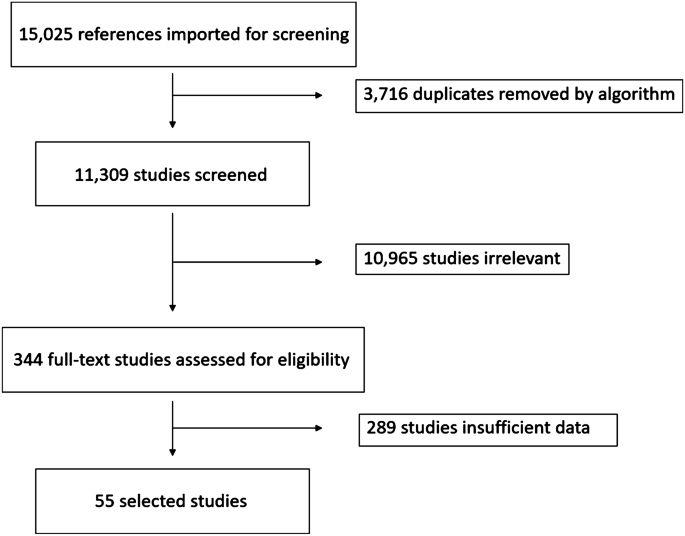
Study design. This flow chart illustrates the selection process for published reports.
Study characteristics
Table 2 shows the basic characteristics of the included studies. In total, our meta-analysis of ML and cardiovascular diseases included 103 cohorts (55 studies) with a total of 3,377,318 individuals. In total, 12 cohorts assessed cardiac arrhythmias (3,144,799 individuals), 45 cohorts are CAD-related (117,200 individuals), 34 cohorts are stroke-related (5,577 individuals), and 12 cohorts are HF-related (109,742 individuals). The characteristics of the included studies are listed in Table 2 . We performed post hoc sensitivity analysis, excluding each study, and found no difference among the results.
ML algorithms and prediction of CAD
For the CAD, 45 cohorts reported a total of 116,227 individuals. 10 cohorts used CNN algorithms, 7 cohorts used SVM, 13 cohorts used boosting algorithm, 9 cohorts used custom-built algorithms, and 2 cohorts used RF. The prediction in CAD was associated with pooled AUC of 0.88 (95% CI 0.84–0.91), sensitivity of 0.86 (95% CI 0.77–0.92), and specificity of 0.70 (95% CI 0.51–0.84), for boosting algorithms and pooled of AUC 0.93 (95% CI 0.85–0.97), sensitivity of 0.87 (95% CI 0.74–0.94), and specificity of 0.86 (95% CI 0.73–0.93) for custom-built algorithms (Fig. 2 ).
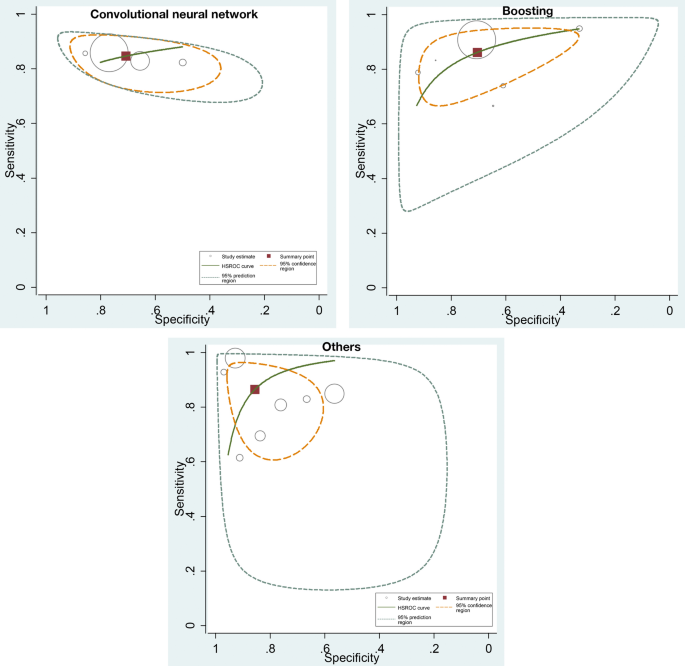
ROC curves comparing different machine learning models for CAD prediction. The prediction in CAD was associated with pooled AUC of 0.87 (95% CI 0.76–0.93) for CNN, pooled AUC of 0.88 (95% CI 0.84–0.91) for boosting algorithms, and pooled of AUC 0.93 (95% CI 0.85–0.97) for others (custom-built algorithms).
ML algorithms and prediction of stroke
For the stroke, 34 cohorts reported a total of 7,027 individuals. 14 cohorts used CNN algorithms, 4 cohorts used SVM, 5 cohorts used boosting algorithm, 2 cohorts used decision tree, 2 cohorts used custom-built algorithms, and 1 cohort used random forest (RF). For prediction of stroke, SVM algorithms had a pooled AUC of 0.92 (95% CI 0.81–0.97), sensitivity 0.57 (95% CI 0.26–0.96), and specificity 0.93 (95% CI 0.71–0.99); boosting algorithms had a pooled AUC of 0.91 (95% CI 0.81–0.96), sensitivity 0.85 (95% CI 0.66–0.94), and specificity 0.85 (95% CI 0.67–0.94); and CNN algorithms had a pooled AUC of 0.90 (95% CI 0.83–0.95), sensitivity of 0.80 (95% CI 0.70–0.87), and specificity of 0.91 (95% CI 0.77–0.97) (Fig. 3 ).
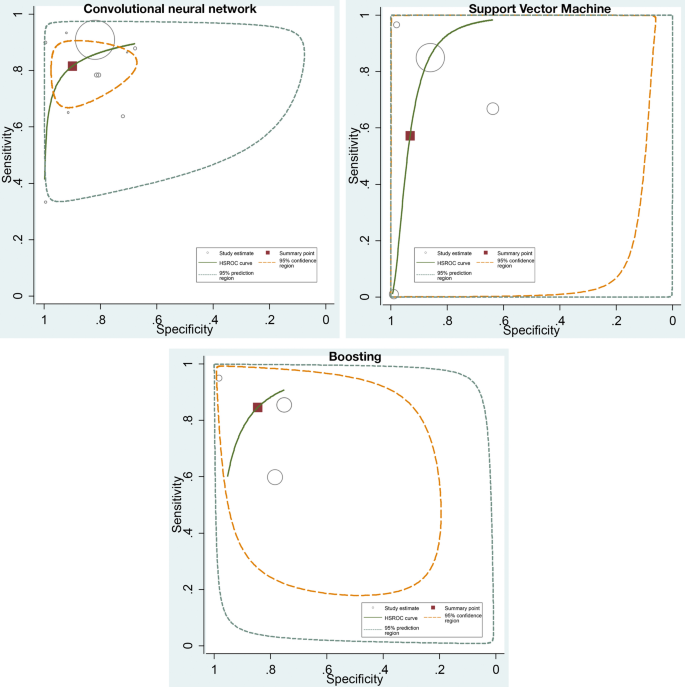
ROC curves comparing different machine learning models for stroke prediction. The prediction in stroke was associated with pooled AUC of 0.90 (95% CI 0.83–0.95) for CNN, pooled AUC of 0.92 (95% CI 0.81–0.97) for SVM algorithms, and pooled AUC of 0.91 (95% CI 0.81–0.96) for boosting algorithms.
ML algorithms and prediction of HF
For the HF, 12 cohorts reported a total of 51,612 individuals. 3 cohorts used CNN algorithms, 4 cohorts used logistic regression, 2 cohorts used boosting algorithm, 1 cohort used SVM, 1 cohort used in-house algorithm, and 1 cohort used RF. We could not perform analyses because we had too few studies (≤ 5) for each model.
ML algorithms and prediction of cardiac arrhythmias
For the cardiac arrhythmias, 12 cohorts reported a total of 3,204,837 individuals. 2 cohorts used CNN algorithms, 2 cohorts used logistic regression, 3 cohorts used SVM, 1 cohort used k-NN algorithm, and 4 cohorts used RF. We could not perform analyses because we had too few studies (≤ 5) for each model.
To the best of our knowledge, this is the first and largest novel meta-analytic approach in ML research to date, which drew from an extensive number of studies that included over one million participants, reporting ML algorithms prediction in cardiovascular diseases. Risk assessment is crucial for the reduction of the worldwide burden of CVD. Traditional prediction models, such as the Framingham risk score 12 , the PCE model 13 , SCORE 14 , and QRISK 15 have been derived based on multiple predictive factors. These prediction models have been implemented in guidelines; specifically, the 2010 American College of Cardiology/American Heart Association (ACC/AHA) guideline 16 recommended the Framingham Risk Score, the United Kingdom National Institute for Health and Care Excellence (NICE) guidelines recommend the QRISK3 score 17 , and the 2016 European Society of Cardiology (ESC) guidelines recommended the SCORE model 18 . These traditional CVD risk scores have several limitations, including variations among validation cohorts, particularly in specific populations such as patients with rheumatoid arthritis 19 , 20 . Under some circumstances, the Framingham score overestimates CVD risk, potentially leading to overtreatment 20 . In general, these risk scores encompass a limited number of predictors and omit several important variables. Given the limitations of the most widely accepted risk models, more robust prediction tools are needed to more accurately predict CVD burden. Advances in computational power to process large amounts of data has accelerated interest in ML-based risk prediction, but clinicians typically have limited understanding of this methodology. Accordingly, we have taken a meta-analytic approach to clarify the insights that ML modeling can provide for CVD research.
Unfortunately, we do not know how or why the authors of the analyzed studies selected the chosen algorithms from the large array of options available. Researchers/authors may have selected potential models for their databases and performed several models (e.g., running parallel, hyperparameter tuning) while only reporting the best model, resulting in overfitting to their data. Therefore, we assume the AUC of each study is based upon the best possible algorithm available to the associated researchers. Most importantly, pooled analyses indicate that, in general, ML algorithms are accurate (AUC 0.8–0.9 s) in overall cardiovascular disease prediction. In subgroup analyses of each ML algorithms, ML algorithms are accurate (AUC 0.8–0.9 s) in CAD and stroke prediction. To date, only one other meta-analysis of the ML literature has been reported, and the underlying concept was similar to ours. The investigators compared the diagnostic performance of various deep learning models and clinicians based on medical imaging (2 studies pertained to cardiology) 21 . The investigators concluded that deep learning algorithms were promising but identified several methodological barriers to matching clinician-level accuracy 21 . Although our work suggests that boosting models and support vector machine (SVM) models are promising for predicting CAD and stroke risk, further study comparing human expert and ML models are needed.
First, the results showed that custom-built algorithms tend to perform better than boosting algorithm for CAD prediction in terms of AUC comparison. However, there is significant heterogeneity among custom-built algorithms that do not disclose their details. The boosting algorithm has been increasingly utilized in modern biomedicine 22 , 23 . In order to implement in clinical practice, the essential stages of designing a model and interpretation need to be uniform 24 . For implementation in clinical practice, custom-built algorithms must be transparent and replicated in multiple studies using the same set of independent variables.
Second, the result showed that boosting algorithms and SVM provides similar pooled AUC for stroke prediction. SVMs and boosting shared a common margin to address the clinical question. SVM seems to perform better than boosting algorithms in patients with stroke perhaps due to discrete, linear data or a proper non-linear kernel that fits the data better with improved generalization. SVM is an algorithm designed for maximizing a particular mathematical function with respect to a given collection of data. Compared to the other ML methods, SVM is more powerful at recognizing hidden patterns in complicated clinical datasets 2 , 25 . Both boosting and SVM algorithms have been widely used in biomedicine and prior studies showed mixed results 26 , 27 , 28 , 29 , 30 . SVM seems to outperform boosting in image recognition tasks 28 , while boosting seems to be superior in omic tasks 27 . However, in subgroup analysis, using research questions or types of protocols or images showed no difference in algorithm predictions.
Third, for heart failure and cardiac arrhythmias, we could not perform meta-analytic approaches due to the small number of studies for each model. However, based on our observation in our systematic review, SVM seems to outperform other predictive algorithms in detecting cardiac arrhythmias, especially in one large study 31 . Interestingly, in HF, the results are inconclusive. One small study showed promising results from SVM 32 . CNN seems to outperform others, but the results are suboptimal 33 . Although we assumed all reported algorithms have optimal variables, technical heterogeneity exists in ML algorithms (e.g., number of folds for cross-validation, bootstrapping techniques, how many run time [epochs], multiple parameters adjustments). In addition, optimal cut off for AUC remained unclear in clinical practice. For example, high or low sensitivity/specificity for each test depends on clinical judgement based on clinically correlated. In general, very high AUCs (0.95 or higher) are recommended, and it is known that AUC 0.50 is not able to distinguish between true and false. In some fields such as applied psychology 34 , with several influential variables, AUC values of 0.70 and higher would be considered strong effects. Moreover, standard practice for ML practitioners recommended reporting certain measures (e.g., AUC, c-statistics) without optimal sensitivity and specificity or model calibration, while interpretation in clinical practice is challenging. For example, the difference in BNP cut off for HF patients could result in a difference in volume management between diuresis and IV fluid in pneumonia with septic shock.
Compared to conventional risk scores, most ML models shared a common set of independent demographic variables (e.g., age, sex, smoking status) and include laboratory values. Although those variables are not well-validated individually in clinical studies, they may add predictive value in certain circumstances. Head-to-head studies comparing ML algorithms and conventional risk models are needed. If these studies demonstrate an advantage of ML-based prediction, the optimal algorithms could be implemented through electronic health records (EHR) to facilitate application in clinical practice. The EHR implementation is well poised for ML based prediction since the data are readily accessible, mitigating dependency on a large number of variables, such as discrete laboratory values. While it may be difficult for physicians in resource-constrained practice settings to access the input data necessary for ML algorithms, it is readily implemented in more highly developed clinical environments.
To this end, the selection of ML algorithm should base on the research question and the structure of the dataset (how large the population is, how many cases exist, how balanced the dataset is, how many available variables there are, whether the data is longitudinal or not, if the clinical outcome is binary or time to event, etc.) For example, CNN is particularly powerful in dealing with image data, while SVM can reduce the high dimensionality of the dataset if the kernel is correctly chosen. While when the sample size is not large enough, deep learning methods will likely overfit the data. Most importantly, this study's intent is not to identify one algorithm that is superior to others.
Limitations
Although the performance of ML-based algorithms seems satisfactory, it is far from optimal. Several methodological barriers can confound results and increase heterogeneity. First, technical parameters such as hyperparameter tuning in algorithms are usually not disclosed to the public, leading to high statistical heterogeneity. Indeed, heterogeneity measures the difference in effect size between studies. Therefore, in the present study, heterogeneity is inevitable as several factors can lead to this (e.g., fine-tuning models, hyperparameter selection, epochs). It is also a not good indicator to use as, in our HSROC model, we largely controlled the heterogeneity. Second, the data partition is also arbitrary because of no standard guidelines for utilization. In the present study, most included studies use 80/20 or 70/30 for training and validation sets. In addition, since the sample size for each type of CVD is small, the pooled results could potentially be biased. Third, feature selection methodologies, and techniques are arbitrary and heterogeneous. Fourth, due to the ambiguity of custom-built algorithms, we could not classify the type of those algorithms. Fifth, studies report different evaluation matrices (e.g., some did not report positive or negative cases, sensitivity/specificity, F-score, etc.). We did not report the confusion matrix for this meta-analytic approach as it required aggregation of raw numbers from studies without adjusting for difference between studies, which could result in bias. Instead, we presented pooled sensitivity and specificity using the HSROC model. Although ML algorithms are robust, several studies did not report complete evaluation metrics such as positive or negative cases, Beyes, bias accuracy, or analysis in the validation cohort since there are many ways to interpret the data depending on the clinical context. Most importantly, some analyses did not correlate with the clinical context, which made it more difficult to interpret. The efficacy of meta-analysis is to increase the power of the study by using the same algorithms. In addition, clinical data are heterogeneous and usually imbalanced. Most ML research did not report balanced accuracy, which could mislead the readers. Sixth, we did not register the analysis in PROSPERO. Finally, some studies reported only the technical aspect without clinical aspects, likely due to a lack of clinician supervision.
Although there are several limitations to overcome to be able to implement ML algorithms in clinical practice, overall ML algorithms showed promising results. SVM and boosting algorithms are widely used in cardiovascular medicine with good results. However, selecting the proper algorithms for the appropriate research questions, comparison to human experts, validation cohorts, and reporting of all possible evaluation matrices are needed for study interpretation in the correct clinical context. Most importantly, prospective studies comparing ML algorithms to conventional risk models are needed. Once validated in that way, ML algorithms could be integrated with electronic health record systems and applied in clinical practice, particularly in high resources areas.
Noble, W. S. Support vector machine applications in computational biology. Kernel Methods Comput. Biol. 71 , 92 (2004).
Google Scholar
Aruna, S. & Rajagopalan, S. A novel SVM based CSSFFS feature selection algorithm for detecting breast cancer. Int. J. Comput. Appl. 31 , 20 (2011).
Lakhani, P. & Sundaram, B. Deep learning at chest radiography: Automated classification of pulmonary tuberculosis by using convolutional neural networks. Radiology 284 , 574–582 (2017).
PubMed Google Scholar
Yasaka, K. & Akai, H. Deep learning with convolutional neural network for differentiation of liver masses at dynamic contrast-enhanced CT: A preliminary study. Radiology 286 , 887–896 (2018).
Christ, P. F. et al. Automatic Liver and Lesion Segmentation in CT Using Cascaded Fully Convolutional Neural Networks and 3D Conditional Random Fields. International Conference on Medical Image Computing and Computer-Assisted Intervention 415–423 (Springer, Berlin, 2016).
Krittanawong, C. et al. Deep learning for cardiovascular medicine: A practical primer. Eur. Heart J. 40 , 2058–2073 (2019).
PubMed PubMed Central Google Scholar
Krittanawong, C., Zhang, H., Wang, Z., Aydar, M. & Kitai, T. Artificial intelligence in precision cardiovascular medicine. J. Am. Coll. Cardiol. 69 , 2657–2664 (2017).
Krittanawong, C. et al. Future direction for using artificial intelligence to predict and manage hypertension. Curr. Hypertens. Rep. 20 , 75 (2018).
Covidence systematic review software. Melbourne AVHIAawcoAD.
Rutter, C. M. & Gatsonis, C. A. A hierarchical regression approach to meta-analysis of diagnostic test accuracy evaluations. Stat. Med. 20 , 2865–2884 (2001).
CAS PubMed Google Scholar
Stroup, D. F. et al. Meta-analysis of observational studies in epidemiology: A proposal for reporting. Meta-analysis Of Observational Studies in Epidemiology (MOOSE) group. JAMA 283 , 2008–2012 (2000).
Wilson, P. W. et al. Prediction of coronary heart disease using risk factor categories. Circulation 97 , 1837–1847 (1998).
Goff, D. C. Jr. et al. 2013 ACC/AHA guideline on the assessment of cardiovascular risk: A report of the American College of Cardiology/American Heart Association Task Force on Practice Guidelines. J. Am. Coll. Cardiol. 63 , 2935–2959 (2014).
Conroy, R. M. et al. Estimation of ten-year risk of fatal cardiovascular disease in Europe: The SCORE project. Eur. Heart J. 24 , 987–1003 (2003).
Hippisley-Cox, J. et al. Predicting cardiovascular risk in England and Wales: Prospective derivation and validation of QRISK2. BMJ (Clinical research ed) 336 , 1475–1482 (2008).
Greenland, P. et al. 2010 ACCF/AHA guideline for assessment of cardiovascular risk in asymptomatic adults: A report of the American College of Cardiology Foundation/American Heart Association Task Force on Practice Guidelines. Circulation 122 , e584-636 (2010).
Hippisley-Cox, J., Coupland, C. & Brindle, P. Development and validation of QRISK3 risk prediction algorithms to estimate future risk of cardiovascular disease: Prospective cohort study. BMJ (Clinical research ed) 357 , j2099 (2017).
Piepoli, M. F. et al. 2016 European Guidelines on cardiovascular disease prevention in clinical practice: The Sixth Joint Task Force of the European Society of Cardiology and Other Societies on Cardiovascular Disease Prevention in Clinical Practice (constituted by representatives of 10 societies and by invited experts) Developed with the special contribution of the European Association for Cardiovascular Prevention & Rehabilitation (EACPR). Eur. Heart J. 37 , 2315–2381 (2016).
Kremers, H. M., Crowson, C. S., Therneau, T. M., Roger, V. L. & Gabriel, S. E. High ten-year risk of cardiovascular disease in newly diagnosed rheumatoid arthritis patients: A population-based cohort study. Arthritis Rheum. 58 , 2268–2274 (2008).
Damen, J. A. et al. Performance of the Framingham risk models and pooled cohort equations for predicting 10-year risk of cardiovascular disease: A systematic review and meta-analysis. BMC Med. 17 , 109 (2019).
Liu, X. et al. A comparison of deep learning performance against health-care professionals in detecting diseases from medical imaging: A systematic review and meta-analysis. Lancet Digit. Health 1 , e271–e297 (2019).
Mayr, A., Binder, H., Gefeller, O. & Schmid, M. The evolution of boosting algorithms. From machine learning to statistical modelling. Methods Inf. Med. 53 , 419–427 (2014).
Buhlmann, P. et al. Discussion of “the evolution of boosting algorithms” and “extending statistical boosting”. Methods Inf. Med. 53 , 436–445 (2014).
Natekin, A. & Knoll, A. Gradient boosting machines, a tutorial. Front. Neurorobot. 7 , 21–21 (2013).
Noble, W. S. What is a support vector machine?. Nat. Biotechnol. 24 , 1565–1567 (2006).
Zhang H, & Gu C. Support vector machines versus Boosting.
Ogutu, J. O., Piepho, H. P. & Schulz-Streeck, T. A comparison of random forests, boosting and support vector machines for genomic selection. BMC Proc. 5 (Suppl 3), S11 (2011).
Sun, T. et al. Comparative evaluation of support vector machines for computer aided diagnosis of lung cancer in CT based on a multi-dimensional data set. Comput. Methods Programs Biomed. 111 , 519–524 (2013).
Huang, M.-W., Chen, C.-W., Lin, W.-C., Ke, S.-W. & Tsai, C.-F. SVM and SVM ensembles in breast cancer prediction. PLoS One 12 , e0161501–e0161501 (2017).
Caruana R, Karampatziakis N, & Yessenalina A. An empirical evaluation of supervised learning in high dimensions. In Proceedings of the 25th International Conference on Machine Learning: ACM , 2008, 96–103.
Hill, N. R. et al. Machine learning to detect and diagnose atrial fibrillation and atrial flutter (AF/F) using routine clinical data. Value Health 21 , S213 (2018).
Rossing, K. et al. Urinary proteomics pilot study for biomarker discovery and diagnosis in heart failure with reduced ejection fraction. PLoS One 11 , e0157167 (2016).
Golas, S. B. et al. A machine learning model to predict the risk of 30-day readmissions in patients with heart failure: A retrospective analysis of electronic medical records data. BMC Med. Inform. Decis. Mak. 18 , 44 (2018).
Rice, M. E. & Harris, G. T. Comparing effect sizes in follow-up studies: ROC Area, Cohen’s d, and r. Law Hum Behav. 29 , 615–620 (2005).
Download references
There was no funding for this work.
Author information
Authors and affiliations.
Section of Cardiology, Baylor College of Medicine, Houston, TX, USA
Chayakrit Krittanawong
Harrington Heart & Vascular Institute, Case Western Reserve University, University Hospitals Cleveland Medical Center, Cleveland, OH, USA
Hafeez Ul Hassan Virk
Department of Cardiovascular Diseases, New York University School of Medicine, New York, NY, USA
Sripal Bangalore
Robert D. and Patricia E. Kern Center for the Science of Health Care Delivery, Rochester, MN, USA
Division of Health Care Policy and Research, Department of Health Sciences Research, Mayo Clinic, Rochester, MN, USA
Department of Genetics and Genomic Sciences, Institute for Next Generation Healthcare, Icahn School of Medicine at Mount Sinai, New York, NY, USA
Kipp W. Johnson
Levy Library, Icahn School of Medicine at Mount Sinai, New York, NY, USA
Rachel Pinotti
Division of Cardiovascular Diseases, Mayo Clinic, Rochester, MN, USA
HongJu Zhang
Department of Cardiovascular Diseases, Icahn School of Medicine at Mount Sinai, Mount Sinai Hospital, Mount Sinai Heart, New York, NY, USA
Chayakrit Krittanawong, Scott Kaplin, Bharat Narasimhan, Usman Baber & Jonathan L. Halperin
Department of Cardiovascular Medicine, Heart and Vascular Institute, Cleveland Clinic, Cleveland, OH, USA
Takeshi Kitai & W. H. Wilson Tang
You can also search for this author in PubMed Google Scholar
Contributions
C.K., H.H., S.B., Z.W., K.W.J., R.P., H.Z., S.K., B.N., T.K., U.B., J.L.H., W.T. had full access to all of the data in the study and take responsibility for the integrity of the data and the accuracy of the data analysis. Study concept and design: C.K., H.H., K.W.J., Z.W. Acquisition of data: C.K., H.H., R.P., H.J., T.K. Analysis and interpretation of data: B.N., Z.W. Drafting of the manuscript: C.K., H.H., S.B., U.B., J.L.H., T.W. Critical revision of the manuscript for important intellectual content: T.W., Z.W. Study supervision: C.K., T.W.
Corresponding author
Correspondence to Chayakrit Krittanawong .
Ethics declarations
Competing interests.
The authors declare no competing interests.
Additional information
Publisher's note.
Springer Nature remains neutral with regard to jurisdictional claims in published maps and institutional affiliations.
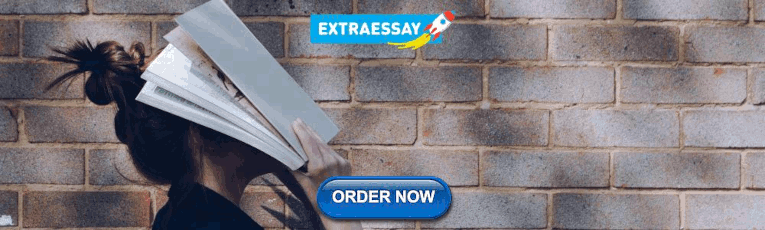
Supplementary information
Supplementary file1, rights and permissions.
Open Access This article is licensed under a Creative Commons Attribution 4.0 International License, which permits use, sharing, adaptation, distribution and reproduction in any medium or format, as long as you give appropriate credit to the original author(s) and the source, provide a link to the Creative Commons licence, and indicate if changes were made. The images or other third party material in this article are included in the article's Creative Commons licence, unless indicated otherwise in a credit line to the material. If material is not included in the article's Creative Commons licence and your intended use is not permitted by statutory regulation or exceeds the permitted use, you will need to obtain permission directly from the copyright holder. To view a copy of this licence, visit http://creativecommons.org/licenses/by/4.0/ .
Reprints and permissions
About this article
Cite this article.
Krittanawong, C., Virk, H.U.H., Bangalore, S. et al. Machine learning prediction in cardiovascular diseases: a meta-analysis. Sci Rep 10 , 16057 (2020). https://doi.org/10.1038/s41598-020-72685-1
Download citation
Received : 27 April 2020
Accepted : 24 August 2020
Published : 29 September 2020
DOI : https://doi.org/10.1038/s41598-020-72685-1
Share this article
Anyone you share the following link with will be able to read this content:
Sorry, a shareable link is not currently available for this article.
Provided by the Springer Nature SharedIt content-sharing initiative
This article is cited by
Causal machine learning for predicting treatment outcomes.
- Stefan Feuerriegel
- Dennis Frauen
- Mihaela van der Schaar
Nature Medicine (2024)
Adverse Effects of Meditation: Autonomic Nervous System Activation and Individual Nauseous Responses During Samadhi Meditation in the Czech Republic
- Silvie Kotherová
- Jakub Cigán
- Milan Sepši
Journal of Religion and Health (2024)
Cardiovascular disease incidence prediction by machine learning and statistical techniques: a 16-year cohort study from eastern Mediterranean region
- Kamran Mehrabani-Zeinabad
- Nizal Sarrafzadegan
BMC Medical Informatics and Decision Making (2023)
Risk prediction of heart failure in patients with ischemic heart disease using network analytics and stacking ensemble learning
- Minghui Shen
Predictis: an IoT and machine learning-based system to predict risk level of cardio-vascular diseases
- Muhammad Nazrul Islam
- Kazi Rafid Raiyan
- Angshu Bikash Mandol
BMC Health Services Research (2023)
By submitting a comment you agree to abide by our Terms and Community Guidelines . If you find something abusive or that does not comply with our terms or guidelines please flag it as inappropriate.
Quick links
- Explore articles by subject
- Guide to authors
- Editorial policies
Sign up for the Nature Briefing newsletter — what matters in science, free to your inbox daily.

Analysis and Prediction of Heart Attack using Machine Learning Models
Ieee account.
- Change Username/Password
- Update Address
Purchase Details
- Payment Options
- Order History
- View Purchased Documents
Profile Information
- Communications Preferences
- Profession and Education
- Technical Interests
- US & Canada: +1 800 678 4333
- Worldwide: +1 732 981 0060
- Contact & Support
- About IEEE Xplore
- Accessibility
- Terms of Use
- Nondiscrimination Policy
- Privacy & Opting Out of Cookies
A not-for-profit organization, IEEE is the world's largest technical professional organization dedicated to advancing technology for the benefit of humanity. © Copyright 2024 IEEE - All rights reserved. Use of this web site signifies your agreement to the terms and conditions.
Prediction of the Risk of Heart Attack Using Machine Learning Techniques
- Conference paper
- First Online: 12 October 2022
- Cite this conference paper
- Pinaki Ghosh 42 , 43 ,
- Umesh Kumar Lilhore 42 , 44 , 46 ,
- Sarita Simaiya 42 ,
- Atul Garg 42 ,
- Devendra Prasad 42 , 45 &
- Ajay Kumar 42
Part of the book series: Lecture Notes in Electrical Engineering ((LNEE,volume 907))
459 Accesses
2 Citations
Heart attack is the number one cause of global mortality. As per World Health Organization (WHO), 17.9 million lives are lost each year from heart-related diseases, representing 32% of all deaths worldwide. The cases of heart attacks are increasing day by day at an alarming rate. It is essential to predict any such risks. This paper is focused on which patients are more likely to have heart disease. In this paper, numerous machine learning models for anticipating the risk of heart attack are addressed. Such algorithms are implemented on a wide range of datasets and the results are compared.
This is a preview of subscription content, log in via an institution to check access.
Access this chapter
- Available as PDF
- Read on any device
- Instant download
- Own it forever
- Available as EPUB and PDF
- Compact, lightweight edition
- Dispatched in 3 to 5 business days
- Free shipping worldwide - see info
- Durable hardcover edition
Tax calculation will be finalised at checkout
Purchases are for personal use only
Institutional subscriptions
World Health Organization (WHO), Cardiovascular Diseases (CVDs), Fact sheets, 11 June 2021. https://www.who.int/news-room/fact-sheets/detail/cardiovascular-diseases-(cvds) . Accessed 28 June 2021
National Heart, Lung, and Blood Institute (NHLBI), “Heart Failure,” https://www.nhlbi.nih.gov/health-topics/heart-failure . Accessed 28 June 2021
Datta P, Sharma B (2017) A survey on IoT architectures, protocols, security, and smart city-based applications. In: 8th international conference on computing, communication and networking technologies (ICCCNT), Proceedings, pp 1–5. https://doi.org/10.1109/ICCCNT.2017.8203943
Ghosh P, Mahesh TR (2015) Smart City: Concept and Challenges. Int J Advanc Eng Technol Sci (IJAETS), 1 (1): 25–27
Google Scholar
Ghosh P, Prasad D, Guleria K (2020) An m-IoT framework for remote monitoring of ECG signals. J Advanc Res Dynam Control Syst (JARDCS) 12(8):296–300. https://doi.org/10.5373/JARDCS/V12I8/20202477
Article Google Scholar
Sarker IH (2021) Machine learning: algorithms, real-world applications and research directions. SN Comput Sci 2 (160). https://doi.org/10.1007/s42979-021-00592-x
R Chhabra, S Verma, and C R Krishna, “A survey on driver behavior detection techniques for intelligent transportation systems,” 7th International Conference on Cloud Computing, Data Science & Engineering - Confluence, 2017, pp. 36–41. https://doi.org/10.1109/CONFLUENCE.2017.7943120
Mohammed Abdul Khaleel (2013) Sateesh Kumar Pradham and G N Dash, “A Survey of Data Mining Techniques on Medical Data for Finding Locally Frequent Diseases.” International Journal of Advanced Research in Computer Science and Software Engineering 3(8):149–153
Alshurafa N, Sideris C, Pourhomayoun M (2017) HaikKalantarian, Majid Sarrafzadeh, and Jo-Ann Eastwood, “Remote Health Monitoring Outcome Success Predictionusing Baseline and First Month Intervention Data.” IEEE J Biomed Health Inform 21(2):507–514. https://doi.org/10.1109/JBHI.2016.2518673
L Sathish Kumar and A Padmapriya, “Prediction for Common Disease using ID3 Algorithm in Mobile Phone and Television”, International Journal of Computer Applications, 50(4), 2012, pp. 30–33
M A Nishara Banu and B Gomathy, “Disease Forecasting System using Data Mining Methods”, International Conference on Intelligent Computing Applications (ICICA), 2014, doi: https://doi.org/10.1109/ICICA.2014.36
Wiharto, Hari Kusnanto, and Herianto, “Intelligence System for Diagnosis Level of Coronary Heart Disease with K-Star Algorithm”, Healthcare Informatics Research, 22 (1), 2016, DOI: https://doi.org/10.4258/hir.2016.22.1.30
Jayshril S Sonawane and D R Patil, “Prediction of Heart Disease using Linear Vector Quantization Algorithm”, Conference on IT in Business, Industry, and Government (CSIBIG), 2014, doi: https://doi.org/10.1109/CSIBIG.2014.7056973
S Madeh Piryonesi and Tamer E El-Diraby, “Role of Data Analytics in Infrastructure Asset Management: Overcoming Data Size and Quality Problems”, Journal of Transportation Engineering, Part B: Pavements, 146 (2), 2020, doi: https://doi.org/10.1061/JPEODX.0000175 .
Juliana Tolles and William J Meurer, “Logistic Regression Relating Patient Characteristics to Outcomes”, The Journal of the American Medical Association (JAMA), 316 (5), 2016, pp. 533–4, doi: https://doi.org/10.1001/jama.2016.7653 .
Trivedi NK, Simaiya S, Lilhore UK, Sharma SK (2020) An efficient credit card fraud detection model based on machine learning methods. International Journal of Advanced Science and Technology 29(5):3414–3424
Patil V, Lilhore UK (2018) A survey on different data mining & machine learning methods for credit card fraud detection. International Journal of Scientific Research in Computer Science, Engineering and Information Technology 3(5):320–325
UCI Machine Learning Repository, “Heart Failure Clinical Records Dataset”, 5 February 2020, Available at: https://archive.ics.uci.edu/ml/datasets/Heart+failure+clinical+records , (Accessed on 27 June 2021).
Pawar N, Lilhore UK, Agrawal N (2017) A hybrid ACHBDF load balancing method for optimum resource utilization in cloud computing. Int J Scientif Res Comput Sci Eng Informat Technol (IJSRCSEIT), ISSN 2456, 3307:367–373
Guleria K, Sharma A, Lilhore UK, Prasad D (2020) Breast cancer prediction and classification using supervised learning techniques. J Comput Theor Nanosci 17(6):2519–2522
Lilhore UK, Simaiya S, Guleria K, Prasad D (2020) An efficient load balancing method by using machine learning-based VM Distribution and dynamic resource mapping. J Comput Theor Nanosci 17(6):2545–2551
Sharma SK, Lilhore UK, Simaiya S, Trivedi NK (2021) An improved random forest algorithm for predicting the COVID-19 pandemic patient health. Ann Romanian Soc Cell Biol:67–75
Lilhore UK, Simaiya S, Prasad D, Verma DK (2021) Hybrid weighted random forests method for prediction & classification of online buying customers. J Informat Technol Manag 13(2):245–259
Trivedi NK, Simaiya S, Lilhore UK, Sharma SK (2021) COVID-19 Pandemic: role of machine learning & deep learning methods in diagnosis. Int J Cur Res Rev| 13(06):150
Simaiya S, Lilhore UK, Prasad D, Verma DK (2021) MRI brain tumour detection & image segmentation by hybrid hierarchical K-means clustering with FCM based machine learning model. Ann Romanian Soc Cell Biol:88–94
Download references
Author information
Authors and affiliations.
Chitkara University Institute of Engineering and Technology, Chitkara University, Punjab, India
Pinaki Ghosh, Umesh Kumar Lilhore, Sarita Simaiya, Atul Garg, Devendra Prasad & Ajay Kumar
Institute of Advance Computing, SAGE University, Bhopal, India
Pinaki Ghosh
KIET Group of Institutions, Delhi-NCR, Ghaziabad, India
Umesh Kumar Lilhore
Department of Computer Science and Engineering, Panipat Institute of Engineering & Technology, Panipat, India
Devendra Prasad
KIET Group of Institutions, Delhi-NCR Ghaziabad, (UP), India
You can also search for this author in PubMed Google Scholar
Corresponding author
Correspondence to Umesh Kumar Lilhore .
Editor information
Editors and affiliations.
School of Information Technology, Rajiv Gandhi Technical University, Bhopal, Madhya Pradesh, India
Sanjeev Sharma
Department of Creative Technologies and Product Design, National Taipei University of Business, Taiwan, Taiwan
Sheng-Lung Peng
Department of Computer Science and Engineering, Rajiv Gandhi Technical University, Bhopal, Madhya Pradesh, India
Jitendra Agrawal
Department of Computer Science and Engineering, Oriental Institute of Science and Technology, Bhopal, Madhya Pradesh, India
Rajesh K. Shukla
Department of Information Technology, Haiphong University, Haiphong, Vietnam
Dac-Nhuong Le
Rights and permissions
Reprints and permissions
Copyright information
© 2022 The Author(s), under exclusive license to Springer Nature Singapore Pte Ltd.
About this paper
Cite this paper.
Ghosh, P., Lilhore, U.K., Simaiya, S., Garg, A., Prasad, D., Kumar, A. (2022). Prediction of the Risk of Heart Attack Using Machine Learning Techniques. In: Sharma, S., Peng, SL., Agrawal, J., Shukla, R.K., Le, DN. (eds) Data, Engineering and Applications. Lecture Notes in Electrical Engineering, vol 907. Springer, Singapore. https://doi.org/10.1007/978-981-19-4687-5_47
Download citation
DOI : https://doi.org/10.1007/978-981-19-4687-5_47
Published : 12 October 2022
Publisher Name : Springer, Singapore
Print ISBN : 978-981-19-4686-8
Online ISBN : 978-981-19-4687-5
eBook Packages : Computer Science Computer Science (R0)
Share this paper
Anyone you share the following link with will be able to read this content:
Sorry, a shareable link is not currently available for this article.
Provided by the Springer Nature SharedIt content-sharing initiative
- Publish with us
Policies and ethics
- Find a journal
- Track your research
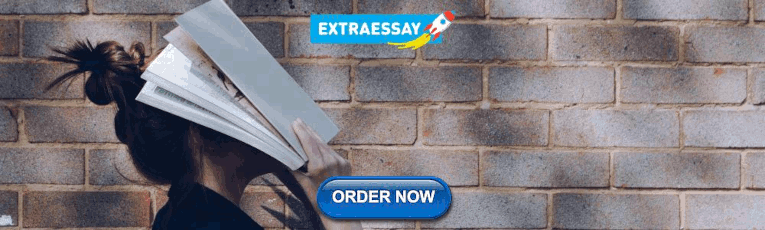
IMAGES
COMMENTS
Heart Attack Research. The NHLBI leads and supports basic research, clinical trials, and large long-term studies related to heart attacks. Our research has helped scientists and doctors better understand the causes of heart attacks and has led to improved treatment options for this condition. We continue to fund research to help find ways to ...
Search for more papers by this author , Aaron W. Aday. Aaron W. Aday. Search for more papers by this author , ... The number of people in the United States dying of a heart attack each year has dropped from 1 in 2 to now 1 in 8. 5. ... Cohorts for Heart and Aging Research in Genomic Epidemiology-Atrial Fibrillation:
Heart disease has several types in which coronary artery disease (CAD) is the common one that can lead to chest pain, stroke, and heart attack. The other types of heart disease include heart ...
Myocardial infarction, or heart attack, is one of the world's biggest killers. An analysis of spatial and single-cell changes to human tissue after a heart attack provides insights into disease ...
Explore the latest full-text research PDFs, articles, conference papers, preprints and more on HEART ATTACK. Find methods information, sources, references or conduct a literature review on HEART ...
Most importantly, pooled analyses indicate that, in general, ML algorithms are accurate (AUC 0.8-0.9 s) in overall cardiovascular disease prediction. In subgroup analyses of each ML algorithms ...
The heart is the most important chunk of the human body. It acts as a pump and helps to circulate the blood throughout the whole body. Over the last few years, heart attacks have become the leading cause of mortality worldwide, and it takes millions of lives each year. Machine learning is a type of artificial intelligence that allows applications to become more accurate at predicting results ...
Background: The American Heart Association, in conjunction with the National Institutes of Health, annually reports the most up-to-date statistics related to heart disease, stroke, and cardiovascular risk factors, including core health behaviors (smoking, physical activity, diet, and weight) and health factors (cholesterol, blood pressure, and glucose control) that contribute to cardiovascular ...
During a heart attack, some people experience these symptoms. Additionally, pain that spreads to the arm, dizziness or light headedness, throat, snoring, and sweating can occur. Heart attacks, strokes, and coronary heart disease, also known as heart failure and coronary artery disease, are much more common in people over 65 than in younger people.
The medical checkup of Blood-pressure level, cholesterol level and heart rate on daily basis along with meditation can help you prevent the major heart attacks. Discover the world's research 25 ...
Effect of Incorporating 1 Avocado per Day Versus Habitual Diet on Vascular Function in Adults With Abdominal Obesity: An Ancillary Study of HAT, a Randomized Controlled Trial. Kristin M. Davis, Kristina S. Petersen, Nirupa R. Matthan, Richard S. Legro and. Penny M. Kris‐Etherton. Journal of the American Heart Association.
Our paper is part of the research on the detection and prediction of heart disease. It is based on the application of Machine Learning algorithms, of which w e have. chosen the 3 most used ...
3 Methodology. The main focus of this research is to identify a machine learning technique that can correctly predict the risk of heart attack based upon the given data. The following machine learning algorithms are used for this research. Naïve Bayes: This approach is based on Bayes' theorem. This is a.
In this paper, the risk factors that causes heart disease is considered and predicted using K-means algorithm and the analysis is carried out using a publicly available data for heart disease. ... Male genders are more likely to experience heart attack than females. ... International Journal of Advanced Research in Computer Engineering ...
In conclusion, the "Heart Attack Risk Prediction using Retinal Eye Images" project stands as a testament to the potential of advanced imaging technologies in improving cardiovascular health outcomes. It has the potential to significantly impact public health by reducing the burden of heart attacks and related
In the recent years, substantial research studies have been carried out to build methods for diagnosing heart diseases in early stages. Various feature selection techniques were applied in the research carried out by Takci. 21 (2018), and the resulting prediction system attained an accuracy of 84.81%.
Symptoms are subjective experiences that may indicate disease or significant change in health status. Symptoms have been linked to cardiovascular disease (CVD) since Egyptian physicians and Hippocrates described fatigue and dyspnea, respectively, as being related to the failing heart. 1,2 In a contemporary view of CVD, symptoms often are critical elements of the diagnosis, evaluation ...
Short bursts of anger may temporarily damage the ability of blood vessels to properly dilate, a function believed to be pivotal in preventing arteries from hardening, new research suggests. The findings, published Wednesday in the Journal of the American Heart Association, may help explain how anger contributes to the risk of having a heart attack.
Background: The American Heart Association, in conjunction with the National Institutes of Health, annually reports on the most up-to-date statistics related to heart disease, stroke, and cardiovascular risk factors, including core health behaviors (smoking, physical activity, diet, and weight) and health factors (cholesterol, blood pressure, and glucose control) that contribute to ...
Women have also been under-represented in research, which has had an impact on what we know about heart attacks, according to Dr Bart. "Even now, most clinical trials only enrol about a quarter of ...
Many fewer Americans are falling prey to the most dangerous form of heart attack, a new study says. STEMI (ST‐segment-elevation myocardial infarction) heart attacks have declined by nearly 50% ...