
An official website of the United States government
The .gov means it’s official. Federal government websites often end in .gov or .mil. Before sharing sensitive information, make sure you’re on a federal government site.
The site is secure. The https:// ensures that you are connecting to the official website and that any information you provide is encrypted and transmitted securely.
- Publications
- Account settings
Preview improvements coming to the PMC website in October 2024. Learn More or Try it out now .
- Advanced Search
- Journal List

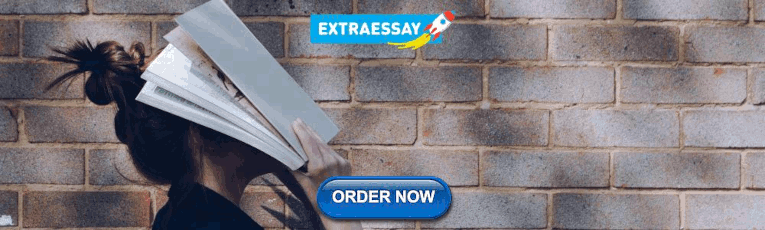
Case selection and causal inferences in qualitative comparative research
Thomas plümper.
1 Department of Socioeconomics, Vienna University of Economics and Business, Vienna, Austria
Vera E. Troeger
2 Department of Economics, University of Warwick, Coventry, United Kingdom
Eric Neumayer
3 Department of Geography and Environment, London School of Economics and Political Science, London, United Kingdom
Associated Data
Replication files for the Monte Carlo simulations can be accessed here: Troeger, Vera Eva; Plümper, Thomas; Neumayer, Eric, 2017, "Replication Data for: Case selection and causal inferences in qualitative comparative research", doi: 10.7910/DVN/3H5EDP , Harvard Dataverse, V1.
Traditionally, social scientists perceived causality as regularity. As a consequence, qualitative comparative case study research was regarded as unsuitable for drawing causal inferences since a few cases cannot establish regularity. The dominant perception of causality has changed, however. Nowadays, social scientists define and identify causality through the counterfactual effect of a treatment. This brings causal inference in qualitative comparative research back on the agenda since comparative case studies can identify counterfactual treatment effects. We argue that the validity of causal inferences from the comparative study of cases depends on the employed case-selection algorithm. We employ Monte Carlo techniques to demonstrate that different case-selection rules strongly differ in their ex ante reliability for making valid causal inferences and identify the most and the least reliable case selection rules.
Introduction
We demonstrate that the validity of causal inferences based on the qualitative comparison of cases depends on the data-generating process and on the choice of case-selection algorithm. While the first factor is beyond the influence of scientists, researchers can freely choose the algorithm that determines the selection of cases. Of course, methodologists have long since been aware of the importance of case-selection for qualitative comparative research [ 1 , 2 , 3 ]. One can trace back systematic theoretical and methodological reasoning on case-selection to at least John Stuart Mill [ 4 ]. After all this time, one might expect that the optimal case-selection algorithms are known. Yet, this is only partially the case. We offer one of the first rigorous analyses of the relative performance of both simple and more complex case-selection rules under conditions of relevance to real world comparative research [ 5 ].
Specifically, we vary the size of the total set of cases from which specific cases are selected, we vary the degrees to which the causal factor of interest is correlated with confounding factors, and we vary the “signal-to-noise ratio”, that is, the (relative) strength of the effect of the causal factor of interest. Using a Monte Carlo design we compare the relative performance of 11 case-selection algorithms, partly following suggestions of qualitative methodologists and partly derived from common practice in comparative case analyses. The very best case-selection algorithm results in an estimated average effect that is almost a hundred times closer to the true effect than the worst algorithm. We also evaluate the conditions conducive to higher validity of causal inferences from qualitative comparative research. We find that the best selection algorithms exhibit relatively high ex ante reliability for making valid inferences if: a) the explanatory variable of interest exerts a strong influence on the dependent variable relative to random noise and confounding factors, b) the variable of interest is not too strongly correlated with confounding variables, and c) the dependent variable is not dichotomous. More importantly, while the best algorithms are still fairly reliable even in the presence of strong stochastic influences on the dependent variable and other complications, the worst algorithms are highly unreliable even if the conditions are met under which qualitative comparative research works best.
Our research contributes to both qualitative and quantitative methodological debates. Quantitative researchers assume that it is impossible to derive valid causal inferences from qualitative comparative research methods. However, we argue that this assumption is outdated because the concept of causality as regularity [ 6 , 4 , 7 ] has been superseded by the concept of causality as counterfactual effect [ 8 , 9 , 10 ]. In fact, the counterfactual concept of causation requires only a single case for causal inference if only it were possible to observe the counterfactual [ 11 , 12 , 13 ]. In the absence of directly observable counterfactual outcomes, the closest methodological equivalents according to the ‘identification school’ are randomization of treatment [ 14 ] and stratification of treatment and control group [ 15 ] through case-selection. It is this latter research strategy of rule- or model-based case-selection that demands a re-evaluation of qualitative comparative designs.
The logic of causal inference typically invoked by quantitative methodologists therefore also applies to qualitative comparative methods: if two or more cases are identical in all relevant dimensions but vary in the treatment, causal inference is internally valid. In addition, our research demonstrates that if these two cases are sampled so that the difference in the treatment is maximized the precision of the computed causal effect is large. We understand of course that these optimal conditions often do not exist and that selected cases vary in more dimensions than the treatment effect. Analyzing how different case-selection rules perform as a function of different conditions in which they must operate is exactly the purpose of our contribution.
As for the debate amongst qualitative methodologists, our results first and foremost speak to qualitative comparative researchers who, in the tradition of John Stuart Mill, draw inferences from the comparison of two sufficiently similar cases that vary in respect to the variable of interest (the ‘treatment’). Yet, the research design logic supported by our results also applies to scholars who compare a single case at two or more different points in time with a ‘treatment’ occurring in between the first and the last observation of the selected single case. These research designs are comparative in nature, and thus our findings that inferences are most likely to be valid if researchers maximize the variance of the variable of interest and minimize the variance of the confounding factors for selecting the case or cases they analyze over time also holds for a comparison of two different observations in time of a single case.
Yet, our research also contrasts with some of the acquired wisdom of qualitative methodologists. We agree that qualitative research, including the in-depth study of one or more cases and the comparative study of cases, can serve many other purposes and are, arguably, better suited for inductive purposes such as theory and concept development [ 16 , 17 ]. Qualitative research often seeks to generate ideas about the data-generating process so that little knowledge of the data-generating process can be assumed to exist prior to the case selection. Clearly, the logic of case selection for deductive causal inference research differs from the logic of case selection for inductive research. We therefore do not believe that our results can or indeed should be extended to inductive research. Importantly, however, many empirical qualitative researchers do make causal inferences and generalize their findings from the analyzed cases to a broader population. Our analysis enables those qualitative researchers who do wish to make causal inferences based on the comparative analysis of cases to understand how case-selection rules differ with respect to their ex ante reliability for detecting the direction and strength of a causal effect. Crucially, given limited knowledge about the data-generating process, we show that the relatively best-performing algorithms remain best-performing no matter what the underlying data-generating process (of those we have analyzed).
Qualitative researchers might struggle with a second aspect of our research design. Qualitative comparative researchers hardly ever estimate the strength of an effect and thus an analysis of effect strengths must seem irrelevant for them (but see [ 18 ]). Yet, we do not compute the effect strength from a comparison of two cases to tempt qualitative researchers to quantify effect strengths. We merely compute the effect strength and compare it to the assumed true effect size to have an indicator against which we can judge the ex ante reliability of selection algorithms. Computing the effect size is a tool, not the goal. Even if qualitative comparative researchers only intend to make inferences on the direction of a causal effect, they should agree that the expected deviation of an implied effect strength estimate from the truth–called root mean squared error by the quantitative tribe–is a good indicator for the relative ex ante reliability of case-selection algorithms: The larger this deviation, the more likely that even the inferred direction of an effect is wrong.
The paper is organized as follows: the next section shows that the now dominant modern concept of causality as counterfactual analysis implies that one can make causal inferences based on qualitative comparative analysis. One cannot make such inferences with certainty, however, and the validity of inferences will crucially depend on how cases are selected. We review what methodologists have advised on the selection of cases in qualitative comparative research in section 3. This informs our choice of selection algorithms that we subject to Monte Carlo analysis, though we also add some original algorithms to test whether and, if so, how much better they can perform. Section 4 describes these algorithms, the Monte Carlo design and how we evaluate the relative performance of the case-selection algorithms. Section 5 presents results from the Monte Carlo simulations.
Causal inference and qualitative comparative research
Causality as regularity dominated the philosophy of science at least from Hume to Popper. Hume [ 5 ] argued that scientists cannot have knowledge of causality beyond observed regularities in associations of events. He therefore suggests inferring causality through a systematic comparison of situations in which the presumed causal factor is present or absent, or varies in strength. The concept of causality as regularity became the central element of Hempel and Oppenheim’s [ 19 ] deductive-nomological model of scientific explanation. Hempel was also the first to develop the concept further to include statistical inference [ 20 ]. In Popper’s conception of a non-degenerative research program [ 7 ], a single falsification effectively leads to the rejection of the tested hypothesis or, worse, the theory from which the hypothesis derives. The “regularity” perspective culminates in the definition of science as “unbroken, natural regularity” [ 21 ].
This “strict regularity” concept of causality had ambiguous implications for comparative social science qualitative researchers’ ability to make causal inferences. On the one hand, the analysis of a small number of cases cannot establish regularity. On the other hand, if, conversely, a single deviant case suffices to refute a causal claim or even a theory, as Popper believes, then strength in numbers does not exist [ 22 , 23 , 17 ]. The “strict regularity” perspective is dead, however, because a) not all regularities are causal (“correlation is not causation”) and b) causality can be probabilistic rather than deterministic and can thus exist without strict regularity.
Probabilistic causal mechanisms paved the way for an interpretation of regularity as statistical regularity. Yet, not even the brilliant idea of statistical inference saved the regularity concept of causality. If correlation is not causality, then high correlation does not imply causality either and low correlation and statistical insignificance may indicate low-probability causality and a lack of sufficient variation rather than the absence of causality. Eventually, this insight eliminated the support for the causality as regularity view.
Over the last three decades, the concept of causality as regularity was replaced by the counterfactual concept of causality, also called the potential outcomes framework. Its understanding of causality is tautological: causality exists if a cause exerts a causal effect on the outcome, and a cause exerts a causal effect on the outcome when the relation is causal. This tautology seems to be the main reason why scholars advancing the counterfactual perspective [ 9 , 10 , 24 , 25 ] focus on causal inference and the identification of causal effects rather than on causality itself [ 24 ].
According to the counterfactual concept of causality, causality is perfectly identified if one observes the outcome given treatment and the outcome given no treatment at the same time for the same person(s). Naturally, this is impossible. Hence, a counterfactual analysis starts with a ‘missing data’ problem and then immediately turns to ‘second-best’ options for inferring causality. If one cannot observe the potential or counterfactual outcome for any one single case, then one needs to resort to comparing the outcomes of different cases. This raises the challenge that either one must make sure that the cases compared are equal or sufficiently similar in all dimensions that matter or that one can render the influence of all potential confounders irrelevant. Otherwise, no causal effect has been ‘identified’.
The approach generally preferred by identification scholars–what they call the “gold standard”–aspires to render potential confounders irrelevant by randomizing treatment across a large number of cases in a controlled experiment (but see [ 25 , 26 ]). Though practically all actual experiments fall way short of the ideal of experimental designs, the randomization of treatments in a sample where N approaches infinity guarantees that the treatment will be uncorrelated with both observable and, crucially, unobservable confounders. Because of this lack of correlation with any potential confounder, any observable difference in outcomes between the two groups must be due to the treatment. If one assumes causal homogeneity among cases and assumes away that potential confounders might condition the effect of treatment, then ideal experiments will not only have identified a cause-effect relationship but will also allow the calculation of the unbiased effect size.
Clearly, from the experimentalist viewpoint, qualitative small-N comparative research is useless for causal inferences. In fact, so is everything else. Its diehard proponents explicitly argue that experiments are a necessary condition for causal inference. For example, Light, Singer, and Willett [ 27 ] claim that “to establish a causal link, you must conduct an experiment (…). Only experimental inquiries allow you to determine whether a treatment causes an outcome to change.” This claim wrongly assumes that identification is a necessary condition for causal inference, whereas in fact perfect identification is only a necessary condition for making causal inferences that are valid with certainty. The idea that one can only make causal inferences if scientists are certain about having identified a cause-effect relationship via experiments is absurd, however. If the claim was correct, scientists would not be able to infer that more education causes higher lifetime income, or that smoking causes lung cancer. For that matter, social scientists would not be able to explore much of interest. The quest for causal inference in the social sciences is not about certainty; it is about how to deal with uncertainty and how much uncertainty about the validity of inferences can be tolerated.
More importantly, making certainty a prerequisite for causal inference runs into a logical problem for the social sciences because experiments that social scientists are able to conduct do not generate inferences that are valid with certainty. Even ignoring causal heterogeneity and potential conditionalities [ 28 ], the confounding-factors problem can only be solved asymptotically, that is, by increasing the sample size to infinity. With a finite number of participants, randomization of treatment does not suffice to render treatment uncorrelated to unobserved confounders like mood, experience, knowledge, or intelligence, and often to even observed confounders like age, sex, income, or education. As a remedy, many experimenters control for observable differences in addition to randomizing treatment. Since it is impossible to control for all factors that influence human behavior, not least because some of them may be unobserved, the problem of confounders can be reduced but not eliminated by experiments. Yet, if experiments only increase the probability that causal inferences are correct, then the strict dichotomy between experiments and all other research methods that Light, Singer, and Willett make is unjustified.
The second approach to solving the “missing data” problem in the counterfactual concept of causality argues that causal effects are identified if cases can be selected so as to guarantee that all the relevant properties of the treatment group exactly match the properties of the control group [ 29 , 30 , 31 ]. Identification via selection or matching on the properties of the treatment and control groups requires perfect knowledge of all the factors that influence outcomes and also that one can match cases on these properties. As with experiments, falling short of this ideal will mean that a causal effect has not been identified with certainty, but does not render causal inference impossible. For experimentalists, matching is far inferior to experiments because they doubt one can know all the relevant properties (one can know the so-called data-generating process) and even if one could know these properties, one cannot measure all of these properties, some of which are unobservable, and thus one cannot match on them.
This second approach substitutes impossible counterfactual analyses with a possible analysis of cases that have been carefully selected to be homogeneous with respect to confounding variables. This strategy is obviously encouraging for causal inference based on case comparison. Nothing in this matching approach suggests that the validity of causal inferences depends on the number of cases. If cases are homogeneous, causal inferences based on small-N qualitative comparative methods become possible, and the validity of these causal inferences depends on the employed selection rule.
Qualitative comparative researchers have always made arguments that closely resemble matching [ 5 ]: if two cases are identical in all relevant dimensions but vary in the dimension of interest (the treatment), then it is possible to directly infer causality and to compute a causal effect size. This possibility does not imply that causal inference from qualitative comparative research is optimal or easy, however. Of course, there is the issue of knowing all relevant dimensions and finding at least two cases which are identical in all these dimensions. There are other difficulties, too: First, if causal processes are stochastic, as they are bound to be, then a single small-N comparative analysis, which cannot control for noise and random errors, will not reveal the truth but some random deviation from the truth. Matching cases in a quantitative analysis with large N therefore can be superior—though the greater difficulty of adequately matching a larger number of cases means that any positive effect on the validity of causal inferences from efficiency gains may be defeated by the negative effect due to problems in matching. Second, perfect homogeneity among cases on all confounding factors can only be achieved if researchers know the true data-generating process, which is unlikely to be the case even if qualitative researchers argue that their in-depth study of cases allow them to know much more about this process than quantitative researchers do [ 32 , 33 ]. In the absence of knowledge of the true data-generating process, qualitative comparative researchers should make sure that selected cases do not differ in respect to known strong confounding factors. The potential for bias grows with the strength of the potentially confounding factor (for which no controls have been included), and the size of the correlation between the variable of interest and the confounder.
Case-selection and qualitative comparisons
Methodological advice on the selection of cases in qualitative research stands in a long tradition. John Stuart Mill in his A System of Logic , first published in 1843, proposed five methods meant to enable researchers to make causal inferences: the method of agreement, the method of difference, the double method of agreement and difference, the method of residues, and the method of concomitant variation [ 4 ]. Methodologists have questioned and criticized the usefulness and general applicability of Mill’s methods [ 34 , 35 ]. However, without doubt Mill’s proposals had a major and lasting impact on the development of the two most prominent modern methods, namely the “most similar” and “most different” comparative case-study designs [ 1 , 36 , 37 ].
Yet, as Seawright and Gerring [ 3 ] point out, these and other methods of case-selection are “poorly understood and often misapplied”. Qualitative researchers mean very different things when they invoke the same terms “most similar” or “most different” and usually the description of their research design is not precise enough to allow readers to assess exactly how cases have been chosen. Seawright and Gerring have therefore provided a formal definition and classification of these and other techniques of case-selection. They [ 3 ] suggest that “in its purest form” the “most similar” design chooses cases which appear to be identical on all controls ( z ) but different in the variable of interest ( x ). Lijphart [ 1 ] suggested what might be regarded a variant of this method that asks researchers to maximize “the ratio between the variance of the operative variables and the variance of the control variables”.
Naturally, the “most similar” technique is not easily applied because researchers find it difficult to match cases such that they are identical on all control variables. As Seawright and Gerring [ 3 ] concede: “Unfortunately, in most observational studies, the matching procedure described previously–known as exact matching–is impossible.” This impossibility has three sources: first, researchers usually do not know the true model and thus cannot match on all control variables. Second, even if known to affect the dependent variable, many variables remain unobserved. And third, even if all necessary pieces of information are available, two cases that are identical in all excluded variables may not exist.
Qualitative comparative researchers prefer the “most similar” technique, despite ambiguity in its definition and practical operationalization, to its main rival, the “most different” design. Seawright and Gerring [ 3 ] believe that this dominance of “most similar” over “most different” design is well justified. Defining the “most different” technique as choosing two cases that are identical in the outcome y and in the main variable of interest x but different in all control variables z , they argue that this technique does not generate much leverage. They criticize three points: first, the chosen cases never represent the entire population (if x can in fact vary in the population). Second, the lack of variation in x renders it impossible to identify causal effects. And third, elimination of rival hypotheses is impossible. As Gerring [ 38 ] formulates poignantly: “There is little point in pursuing cross-unit analysis if the units in question do not exhibit variation on the dimensions of theoretical interest and/or the researcher cannot manage to hold other, potentially confounding, factors constant.”
For comparative case studies, Seawright and Gerring also identify a third selection technique, which they label the “diverse” technique. It selects cases so as to “represent the full range of values characterizing X, Y, or some particular X/Y relationship” [ 3 ]. This definition is somewhat ambiguous and vague (“some particular relationship”), but one of the selection algorithms used below in our MC simulations captures the essence of this technique by simultaneously maximizing variation in y and x .
Perhaps surprisingly, King, Keohane and Verba’s [ 39 ] seminal contribution to qualitative research methodology discusses case-selection only from the perspective of unit homogeneity–broadly understood as constant effect assumption–and selection bias–defined as non-random selection of cases that are not statistically representative of the population. Selecting cases in a way that does not avoid selection bias negatively affects the generalizability of inferences. Random sampling from the population of cases would clearly avoid selection bias. Thus, given the prominence of selection bias in King et al.’s discussion of case-selection, the absence of random sampling in comparative research may appear surprising. But it is not. Random selection of cases leads to inferences which are correct on average when the number of conducted case studies approaches infinity, but the sampling deviation is extremely large. As a consequence, the reliability of single studies of randomly sampled cases remains low. The advice King and his co-authors give on case-selection, then, lends additional credibility to commonly chosen practices by qualitative comparative researchers, namely to avoid truncation of the dependent variable, to avoid selection on the dependent variable, while at the same time selecting according to the categories of the “key causal explanatory variable”. King et al. [ 39 ] also repeatedly claim that increasing the number of observations makes causal inferences more reliable. Qualitative methodologists have argued that this view, while correct in principle, does not do justice to qualitative research [ 40 , 41 , 42 ]. More importantly, they also suggest that the extent to which the logic of quantitative research can be superimposed on qualitative research designs has limits.
While there is a growing consensus on the importance of case-selection for comparative research, as yet very little overall agreement has emerged concerning the use of central terminology and the relative advantages of different case-selection rules. Scholars largely agree that random sampling is unsuitable for qualitative comparative research (but see [ 5 ]), but disagreement on sampling on the dependent variable, and the appropriate use of information from observable confounding factors persists. Our Monte Carlo analysis will shed light on this issue by exploring which selection algorithms are best suited under a variety of assumptions about the data-generating process.
A Monte Carlo analysis of case-selection algorithms
In statistics, Monte Carlo experiments are employed to compare the performance of estimators. The term Monte Carlo experiments describes a broad set of techniques that randomly draw values from a probability distribution to add error to a predefined equation that serves as data-generating process. Since the truth is known, it is straightforward to compare the estimated or computed effects to the true effects. An estimator performs the better the smaller the average distance between the estimated effect and the truth. This average distance is usually called the root mean squared error.
Our Monte Carlo experiments follow this common practice in statistics and merely replace the estimators by a case-selection rule or algorithm. We compare selection rules commonly used in applied qualitative comparative research, as well as various simple permutations and extensions. Without loss of generality, we assume a data-generating process in which the dependent variable y is a linear function of a variable of interest x , a control variable z and an error term ε . Since we can interpret z as a vector of k control variables, we can generalize findings to analyses with multiple controls.
Case-selection algorithms
Ignoring for the time being standard advice against sampling on the dependent variable, researchers might wish to maximize variation of y , maximize variation of x , minimize variation of z or some combination thereof. Employing addition and subtraction, the two most basic functions to aggregate information on more than one variable, leads to seven permutations of information from which to choose; together with random sampling this results in eight simple case-selection algorithms–see Table 1 . The mathematical description of the selection algorithms, as shown in the last column of the table, relies on the set-up of the Monte Carlo analyses (described in the next section). In general, for each variable we generate Euclidean distance matrices, which are N×N matrices representing the difference or distance in a set of cases i and j forming the case-dyad ij . Starting from these distance matrices, we select two cases that follow a specific selection rule. For example, max(x) only considers the explanatory variable of interest, thereby ignoring the distance matrices for the dependent variable y and the control variable z . With max(x) , we select the two cases that represent the cell of the distance matrix with the largest distance value. We refrain from analyzing case-selection algorithms for qualitative research with more than two cases. Note, however, that all major results we show here carry over to selecting more than two cases based on a single algorithm. However, we do not yet know whether all our results carry over to analyses of more than two cases when researchers select cases based on different algorithms–a topic we will revisit in future research.
Algorithm 1 does not use information (other than that a case belongs to the population), and samples cases randomly. We include this algorithm for completeness and because qualitative methodologists argue that random sampling–the gold standard for sampling in quantitative research–does not work well in small-N comparative research.
We incorporate the second algorithm–pure sampling on the dependent variable without regard to variation of either x or z –for the same completeness reason. Echoing Geddes [ 43 ], many scholars have argued that sampling on the dependent variable biases the results [ 39 , 44 , 45 ]. Geddes demonstrates that “selecting on the dependent variable” lies at the core of invalid results generated from qualitative comparative research in fields as diverse as economic development, social revolution, and inflation.
But does Geddes’s compelling critique of sampling on the dependent variable imply that applied researchers should entirely ignore information on the dependent variable when they also use information on the variable of interest or the confounding factors? Algorithms 5, 6, and 8 help us to explore this question. These rules include selection on the dependent variable in addition to selection on x and/or z . Theoretically, these algorithms should perform better than the algorithm 2, but we are more interested in analyzing how these biased algorithms perform in comparison to their counterparts, namely algorithms 3, 4, and 7, which, respectively, maximize variation of x , minimize variation of z , and simultaneously maximize variation of x and minimize variation of z , just as algorithms 5, 6 and 8 do, but this time without regard to variation of y .
Theoretically, one would expect algorithm 7 to outperform algorithms 3 and 4. Qualitative methodlogists such as Gerring and Seawright and Gerring [ 17 , 3 ] expect this outcome and we concur. Using more information must be preferable to using less information when it comes to sampling. This does not imply, however, that algorithm 7 necessarily offers the optimal selection rule for qualitative comparative research. Since information from at least two different variables has to be aggregated, researchers have at their disposal multiple possible algorithms that all aggregate information in different ways. For example, in addition to the simple unweighted sum (or difference) that we assume in Table 1 , one can aggregate by multiplying or dividing the distances, and one can also weight the individual components.
Lijphart [ 1 ] has suggested an alternative function for aggregation, namely maximizing the ratio of the variance in x and z : max[dist(x)/dist(z)] . We include Lijphart’s suggestion as our algorithm 9 even though it suffers from a simple problem which reduces its usefulness: when the variance of the control variable z is smaller than 1.0, the variance of what Lijphart calls the operative variable x becomes increasingly unimportant for case-selection (unless of course the variation of the control variables is very similar across different pairs of cases). We solve this problem by also including in the competition an augmented version of Lijphart’s suggestion. This algorithm 10 adds one to the denominator of the algorithm proposed by Lijphart: max[dist(x)/(1+dist(z))] . Observe that adding one to the denominator prevents the algorithm from converging to min[dist(z)] when dist(z) becomes small. Finally, we add a variance-weighted version of algorithm 7 as our final algorithm 11 to check whether weighting improves on the simple algorithms. Table 2 summarizes the additional analyzed algorithms that aggregate information using more complicated functions.
Note that thus far we have given the selection algorithms formal and technical labels, avoiding terminology of case-selection rules commonly used in the literature. Nevertheless, there are connections between some of the above algorithms and the terminology commonly used in the literature. For example, algorithms 2, 3 and 5 are variants of selection rules described by Seawright and Gerring [ 3 ] as “diverse” case-selection rules. Algorithms 2, 5, 6, and 8 all use information on variation of the dependent variable and are thus variants of selection on the dependent variable. More importantly, algorithms 4 and 7 as well as algorithms 9 to 11 seem to be variants of the most similar design. However, we do not call any of these algorithms “selection on the dependent variable” or “most similar”. The reason is that, as discussed above, there is a lack of consensus on terminology and different scholars prefer different labels and often mean different things when they invoke rules such as “sampling on the dependent variable” or “most similar”.
The Monte Carlo design
The use of Monte Carlo techniques may appear to be strange to qualitative researchers. However, Monte Carlo simulations are perfectly suited for the purpose of exploring the ex ante reliability of case-selection algorithms. As we have explained above, Monte Carlo simulations provide insights into the expected accuracy of inferences given certain pre-defined properties of the data-generating process. While they are commonly used to compare estimators, one can equally use them to compare the performance of different sampling rules.
Monte Carlo simulations allow us to systematically change the data-generating process, and to explore the comparative advantages of different selection algorithms depending on the assumptions we make about the data-generating process. Possible systematic changes include variation in the assumed level of correlation between explanatory variables, the relative importance of uncertainty, the level of measurement error, and so on. Unsystematic changes are modelled by repeated random draws of the error term.
Specifically, we define various data-generating processes from which we draw a number of random samples, and then select two cases from each sample according to a specific algorithm, as defined above. As a consequence of the unaccounted error process, the computed effects from the various Monte Carlo simulations will deviate somewhat from the truth. Yet, since we confront all selection algorithms to the same set of data-generating processes, including the same error processes, performance differences must result from the algorithms themselves. These differences occur because different algorithms will select different pairs of cases i and j , and, as a consequence, the computed effect and the distance of this effect from the true effect differ. Our analysis explores to what extent a comparison of two cases allows researchers to estimate the effect that one explanatory variable, called x , exerts on a dependent variable, called y . We assume that this dependent variable y is a function of x , a single control variable z , which is observed, and some error term ε : y i = βx i + γz i + ε i , where β, γ represent coefficients and ε is an iid error process. Obviously, as var( ε ) approaches zero, the data-generating process becomes increasingly deterministic. We follow the convention of quantitative methodology and assume that the error term is randomly drawn from a standard normal distribution. Note, however, that since we are not interested in asymptotic properties of case-selection algorithms, we could as well draw the error term from different distributions. This would have no consequence other than adding systematic bias to all algorithms alike. The process resembles what Gerring and McDermott [ 46 ] call a “spatial comparison” (a comparison across n observations), but our conclusions equally apply to “longitudinal” (a comparison across t periods) and “dynamic comparisons” (a comparison across n · t observations). We conducted simulations with both a continuous and a binary dependent variable. We report results for the continuous variable in detail in the next section and briefly summarize the results for the binary dependent variable with full results reported in the appendices.
There are different ways to think about the error term. First, usually scientists implicitly assume that the world is not perfectly determined and they allow for multiple equilibria which depend on random constellations or the free will of actors. In this respect, the error term accounts for the existence of behavioral randomness. Second, virtually all social scientists acknowledge the existence of systematic and unsystematic measurement error. The error term can be perceived as accounting for information that is partly uncertain. And third, the error term can be interpreted as model uncertainty–that is, as unobserved omitted variables also exerting an influence on the dependent variable. Only if randomness and free will, measurement error, and model uncertainty did not exist, would the inclusion of an error term make no sense.
We always draw x and z from a normal distribution, but, of course, alternative assumptions are possible. Given the low number of observations, it comes without loss in generality that we draw ε from a normal distribution with mean zero and standard deviation of 1.5; and, unless otherwise stated, all true coefficients take the value of 1.0; the standard deviation of variables is 1.0; correlations are 0.0; and the number of observations N, representing the size of the sample from which researchers can select cases, equals 100.
Evaluating the results from the Monte Carlo simulations
We compare the reliability of inference on effect strength. Specifically, the effect size of x on y from a comparative case study with two cases equals
where subscripts [ i , j ] represent the two selected cases. We take the root mean squared error (RMSE) as our measure for the reliability of causal inference as it reacts to both bias and inefficiency. The RMSE is defined as
This criterion not only incorporates bias (the average deviation of the computed effect from the true effect), but also accounts for inefficiency, which is a measure of the sampling variation of the computed effect that reflects the influence of random noise on the computed effect. Qualitative researchers cannot appropriately control for the influence of noise on estimates. The best they can do to account for randomness is to choose a case-selection algorithm that responds less than others to noise. Naturally, these are case-selection algorithms that make best use of information. In quantitative research, the property characterizing the best use of information is called efficiency , and we see no reason to deviate from this terminology.
Results from the Monte Carlo analysis of case-selection algorithms
We conduct three sets of MC simulations, in which we vary the parameters of the data-generating process, and evaluate the effect of this variation on the precision with which the algorithms approach the true coefficients together with the efficiency of the estimation. In each type of analysis we draw 1,000 samples from the underlying data-generating process. In the first set of simulations, we change the number of observations from which the two cases are chosen ( i = 1,…N), thereby varying the size of the sample, i.e., the total number of cases from which researchers can select two cases. In the second set of simulations, we vary the correlation between x and z –that is, the correlation between the variable of interest and the confounding factor. In the final set of simulations, we vary the variance of x and thus the effect size or explanatory power of x relative to the effect size of the confounding factor z .
Analyzing the impact of varying the number of analyzed cases on the validity of inferences in qualitative comparative research may seem strange at first glance. After all, qualitative researchers usually study a fairly limited number of cases. In fact, in our Monte Carlo analyses we generate effects by looking at a single pair of cases selected by each of the case-selection algorithms. So why should the number of cases from which we select the two cases matter? The reason is that if qualitative researchers can choose from a larger number of cases about which they have theoretically relevant information, they will be able to select a better pair of cases given the chosen algorithm. The more information researchers have before they select cases, the more reliable their inferences should thus become. In other words, N does not represent the number of cases analyzed, but the number of the total set of cases from which the analyzed cases are chosen.
By varying the correlation between x and the control variable z we can analyze the impact of confounding factors on the performance of the case-selection algorithms. With increasing correlation, inferences should become less reliable. Thereby, we look at the effect of potential model misspecification on the validity of inference in qualitative comparative research. While quantitative researchers can eliminate the potential for bias from correlated control variables by including these on the right-hand-side of the regression model, qualitative researchers have to use appropriate case-selection rules to reduce the potential for bias.
Finally, in varying the standard deviation of x we analyze the impact of varying the strength of the effect of the variable of interest on the dependent variable. The larger this relative effect size of the variable of interest, the more reliable causal inferences should become. The smaller the effect of the variable of interest x on y in comparison to the effect on y of the control or confounding variables z , the harder it is to identify the effect correctly, and the less valid the inferences become–especially when the researcher does not know the true specification of the model.
Table 3 reports the Monte Carlo results obtained when we only vary the size of the sample from which we draw the two cases we compare. In this set of simulations, we do not allow for systematic correlation between the variable of interest x and the confounding factor z . The deviations of computed effects from the true effect occur because of “normal” sampling error, and how efficiently the algorithm deals with the available information.
Note: corr(x,z) = 0, SD(x) = 1
The table displays the root mean squared error. Smaller numbers indicate higher reliability.
Observe, first, that of the basic case-selection algorithms, max(x)min(z) performs up to 100 times better with respect to the average deviation from the true effect (the root mean squared error) than the poorest-performing competitors, namely random , which draws two cases randomly from the sample, and max(y) , which purely selects on the dependent variable. The drawback from selecting on the dependent variable declines if researchers additionally take into account variation of x and/or variation of z , but these algorithms 5, 6, and 8 are typically inferior to their counterparts 3, 4, and 7, which ignore variation of the dependent variable. Accordingly, selection on the dependent variable not only leads to unreliable inferences that are likely to be wrong, it also makes other selection algorithms less reliable. Hence, researchers should not pay attention to variation in the dependent variable y when they select cases. By selecting cases on the variable of interest x while at the same time controlling for the influence of confounding factors, researchers are likely to choose cases which vary in their outcome if x indeed exerts an effect on y .
Maximizing variation of x while at the same time minimizing variation of z appears optimal. Algorithm 7 uses subtraction as a basic function for aggregating information from more than one variable. Would using a more complicated function dramatically improve the performance of case-selection? The results reported in Table 3 show that, at least for this set of simulations, this is not the case. Algorithm 7 performs roughly 10 percent better than the augmented version of Lijphart’s proposal ( augmented lijphart ), and while algorithm 11, the variance-weighted version of algorithm 7, is very slightly superior, not much separates the performance of the two.
Another interesting finding from Table 3 is that only four algorithms become systematically more reliable when the population size from which we draw two cases increases. These four algorithms are: max(x) , max(x)min(z) and its weighted variant, weighted max(x)min(z) , as well as augmented lijphart . Algorithms need to have a certain quality to generate, in expectation, improvements in the validity of causal inferences when the population size becomes larger. Random selection, for example, only improves on average if the increase in population size leads to relatively more “onliers” than “outliers”. This may be the case, but there is no guarantee. When researchers use relatively reliable case-selection algorithms, however, an increase in the size of the sample, on which information is available, improves causal inferences unless one adds extreme outliers to the sample. Inferences become more reliable if cases are selected from a larger sample of cases for which researchers have sufficient information. We are not making any normative claim about enlarging the population size, because the improvements of enlarging the population from which cases are selected has to be discounted by the deteriorations caused by an increase in case heterogeneity caused by an enlarged sample.
The results from Table 3 support King, Keohane and Verba’s [ 39 ] arguments against both random selection and sampling on the dependent variable. At first sight, our results seem to differ from Herron and Quinn’s [ 5 ] finding that “simple random sampling outperforms most methods of case selection” even when the number of analyzed cases “is as small as 5 or 7”. However, our results are consistent with Herron and Quinn’s finding that random sampling is not reliable when the number of cases is two. In fact, the number of cases required to make random sampling a viable strategy depends on the heterogeneity of cases and the signal-to-noise ratio of the causal effect of interest: the more homogeneous and stronger the effect researchers are interested in, the better the performance of random selection of cases and the lower the number of cases for sufficiently reliable inferences.
In Table 4 , we report the results of Monte Carlo simulations from varying the correlation between the variable of interest x and the confounding factor z .
Note: SD(x) = 1.0, N = 100, SD(z) = 1.0, Varying Correlation (x,z). The table displays the root mean squared error. Smaller numbers indicate higher reliability.
Note that all substantive results from Table 3 remain valid if we allow for correlation between the variable of interest and the confounding factor. In particular, algorithm 11, which weights the individual components of the best-performing simple case-selection algorithm 7, performs only very slightly better; while the performance gap between simple algorithm max(x)min(z) , based on subtraction, and the augmented Lijphart algorithm ( augmented lijphart ), which uses the ratio as aggregation function, increases only marginally. Table 4 also demonstrates that correlation between the variable of interest and confounding factors renders causal inferences from qualitative comparative research less reliable. Over all simulations and algorithms, the RMSE increases by at least 100 percent when the correlation between x and z increases from 0.0 to either -0.9 or +0.9.
Finally, we examine how algorithms respond to variation in the strength of the effect of the variable of interest. In this final set of simulations for which results are reported in Table 5 we vary the standard deviation of the explanatory factor x ; a small standard deviation indicates a small effect of x on y relative to the effect exerted from z on y . The results show that the performance of all case-selection algorithms suffers from a low “signal-to-noise” ratio. As one would expect, the smaller the effect of the variable of interest x on y relative to the effect of z on y , the less reliable the causal inferences from comparative case study research becomes. Yet, we find that the algorithms which performed best in the previous two sets of simulations also turn out to be least vulnerable to a small effect of the variable of interest. Accordingly, while inferences do become more unreliable when the effect of the variable of interest becomes small relative to the total variation of the dependent variable, comparative case studies are not simply confined to analyzing the main determinant of the phenomenon of interest if one of the top performing case-selection algorithms are used. As in the previous sets of simulations, we find that little is gained by employing more complicated functions for aggregating information from more than one variable as, for example, the ratio ( augmented lijphart ) or weighting by the variance of x and z ( weighted max(x)min(z) ). Sticking to the most basic aggregation function has little cost, if any.
Note: corr(x,z) = 0.0, N = 100, SD(z) = 1.0, Varying SD(x)
We now briefly report results from additional Monte Carlo simulations which we show in full in the appendix to the paper ( S1 File ). First, weighting x and z by their respective sample range becomes more important when the data-generating process includes correlation between x and z and the effect of x on y is relatively small (see Table A in S1 File ). In this case, weighting both the variation of x and z before using the max(x)min(z) selection rule for identifying two cases slightly increases the reliability of causal inferences.
Second, we also conducted the full range of Monte Carlo simulations with a dichotomous dependent variable (see Tables B- E in S1 File ). We find that the algorithms that perform best with a continuous dependent variable also dominate with respect to reliability when we analyze dichotomous dependent variables. Yet, causal inferences from qualitative comparative case study research become far less reliable when the dependent variable is dichotomous for all selection algorithms compared to the case of a continuous dependent variable. The root mean squared error roughly doubles for the better-performing algorithms. As a consequence, causal inferences with a binary dependent variable and an additional complication (either a non-trivial correlation between x and z or a relatively small effect of x on y ) are not reliable. Accordingly, qualitative researchers should not throw away variation by dichotomizing their dependent variable. Where the dependent variable is dichotomous, qualitative comparative research is confined to what most qualitative researchers actually do in these situations: trying to identify strong and deterministic relationships or necessary conditions [ 47 , 48 ]. In both cases, the strong deterministic effect of x on y compensates for the low level of information in the data.
Case-selection rules employed in qualitative research resemble ‘matching’ algorithms developed by identification scholars in quantitative research and thus can be employed to derive causal inferences. They also share their most important shortcoming: the extent to which causal inferences from selected samples are valid is partly determined by the extent of knowledge of the data-generating process. The more is known about the “true model”, the better researchers can select cases to maximize the ex ante reliability of their causal inferences.
Our major contribution has been to guide qualitative comparative researchers on what are the selection rules with the highest ex ante reliability for the purpose of making causal inferences under a range of conditions regarding the underlying data-generating process. The validity of causal inferences from qualitative comparative research will necessarily always be uncertain but following our guidance will allow qualitative comparative researchers to maximize the imperfect validity of their inferences.
Qualitative comparative researchers can take away six important concrete lessons from our Monte Carlo simulations: First, ceteris paribus, selecting cases from a larger set of potential cases gives more reliable results. Qualitative researchers often deal with extremely small samples. Sometimes nothing can be done to increase sample size, but where there are no binding constraints it can well be worth the effort expanding the sample from which cases can be selected. Second, for all the better-performing selection algorithms, it holds that ignoring information on the dependent variable for the purpose of selecting cases makes inferences much more reliable. Tempting though it may seem, qualitative comparative researchers should not select on the dependent variable at all. Third, selecting cases based on both the variable of interest and confounding factors improves the ex ante reliability of causal inferences in comparison to selection algorithms that consider just the variable of interest or just confounding factors–even if this means that one no longer chooses the cases that match most closely on confounding factors. These algorithms are relatively best-performing, no matter what the underlying data-generating process (of those we have analyzed). This is a crucial lesson because qualitative comparative researchers might not have much knowledge about the kind of data-generating process they are dealing with. Fourth, correlation between the variable of interest and confounding factors renders the selection algorithms less reliable. The same holds if the analyzed effect is weak. This reinforces existing views that qualitative case comparison is most suitable for studying strong and deterministic causal relationships [ 47 , 48 ]. Fifth, the reliability of case-selection rules depends on the variation in the dependent variable scholars can analyze. Accordingly, unless there are very strong over-riding theoretical or conceptual reasons, throwing away information by dichotomizing the dependent variable is a bad idea. A continuous dependent variable allows for more valid inferences; a dichotomous dependent variable should only be used if there is no alternative. Sixth, employing basic functions for aggregating information from more than one variable (such as maximizing the difference between variation of x and variation of z ) does not reduce by much the ex ante reliability of case-selection compared to more complicated aggregation functions (such as maximizing the ratio or the variance-weighted difference). The only exceptions occur if x and z are highly correlated and the effect of x on y is relatively small compared to the effect of z on y . As a general rule, one does not lose much by opting for the most basic aggregation function.
In conclusion, our Monte Carlo study is broadly consistent with the views of qualitative methodologists. After all, the best- or nearly best-performing algorithms in our analysis of alternative selection algorithms appear to be variants of the most similar design, which in turn draws on Przeworski and Teune’s [ 35 ] and Lijphart’s [ 49 ] suggestions for case-selection. However, we are the first to provide systematic evidence that upholds existing recommendations in the presence of stochastic error processes. In addition, we demonstrated that simple functions for linking variation of the explanatory variable with variation of the confounding variables perform relatively well in general. There is little reason to resort to more advanced functions unless the explanatory variable has a weak effect and is strongly correlated with the confounding variables. One important area for further analysis comes from settings in which comparative qualitative researchers assess claims about two or more causal factors interacting with each other.
Supporting information
Funding statement.
The authors received no funding for this work.
Data Availability
- Find My Rep
You are here
Configurational Comparative Methods Qualitative Comparative Analysis (QCA) and Related Techniques
- Benoît Rihoux - Université Catholique de Louvain, Belgium
- Charles C. Ragin - University of California, Irvine, USA
- Description
This book is useful for UG students and I will recommend it for those students that want to conduct research between Qual and Quant. However, I believe it would be more useful to MSc or MBA students. This is something I hadn't thought about before and I will consider it now.
This is a very important book on configurational comparative methods.
The book comprises of different essays about QCA, fsQCA, mvQCA, ... and is a useful resource to get started with configurational methods as it covers different aspects within one book.
I have not got the support that I want for this book--so I will use it as a recommended text-not a primary textbook.
Sample Materials & Chapters
Introduction
Ch. 1 - Qualitative Comparative Analysis (QCA) as an Approach
Ch. 3 - Crisp-Set Qualitative Comparative Analysis (csQCA)
For instructors
Please select a format:
Select a Purchasing Option
- Electronic Order Options VitalSource Amazon Kindle Google Play eBooks.com
Related Products

SAGE Research Methods is a research methods tool created to help researchers, faculty and students with their research projects. SAGE Research Methods links over 175,000 pages of SAGE’s renowned book, journal and reference content with truly advanced search and discovery tools. Researchers can explore methods concepts to help them design research projects, understand particular methods or identify a new method, conduct their research, and write up their findings. Since SAGE Research Methods focuses on methodology rather than disciplines, it can be used across the social sciences, health sciences, and more.
With SAGE Research Methods, researchers can explore their chosen method across the depth and breadth of content, expanding or refining their search as needed; read online, print, or email full-text content; utilize suggested related methods and links to related authors from SAGE Research Methods' robust library and unique features; and even share their own collections of content through Methods Lists. SAGE Research Methods contains content from over 720 books, dictionaries, encyclopedias, and handbooks, the entire “Little Green Book,” and "Little Blue Book” series, two Major Works collating a selection of journal articles, and specially commissioned videos.
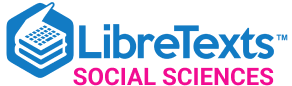
- school Campus Bookshelves
- menu_book Bookshelves
- perm_media Learning Objects
- login Login
- how_to_reg Request Instructor Account
- hub Instructor Commons
Margin Size
- Download Page (PDF)
- Download Full Book (PDF)
- Periodic Table
- Physics Constants
- Scientific Calculator
- Reference & Cite
- Tools expand_more
- Readability
selected template will load here
This action is not available.
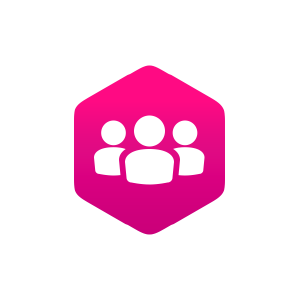
2.3: Case Selection (Or, How to Use Cases in Your Comparative Analysis)
- Last updated
- Save as PDF
- Page ID 150427
\( \newcommand{\vecs}[1]{\overset { \scriptstyle \rightharpoonup} {\mathbf{#1}} } \)
\( \newcommand{\vecd}[1]{\overset{-\!-\!\rightharpoonup}{\vphantom{a}\smash {#1}}} \)
\( \newcommand{\id}{\mathrm{id}}\) \( \newcommand{\Span}{\mathrm{span}}\)
( \newcommand{\kernel}{\mathrm{null}\,}\) \( \newcommand{\range}{\mathrm{range}\,}\)
\( \newcommand{\RealPart}{\mathrm{Re}}\) \( \newcommand{\ImaginaryPart}{\mathrm{Im}}\)
\( \newcommand{\Argument}{\mathrm{Arg}}\) \( \newcommand{\norm}[1]{\| #1 \|}\)
\( \newcommand{\inner}[2]{\langle #1, #2 \rangle}\)
\( \newcommand{\Span}{\mathrm{span}}\)
\( \newcommand{\id}{\mathrm{id}}\)
\( \newcommand{\kernel}{\mathrm{null}\,}\)
\( \newcommand{\range}{\mathrm{range}\,}\)
\( \newcommand{\RealPart}{\mathrm{Re}}\)
\( \newcommand{\ImaginaryPart}{\mathrm{Im}}\)
\( \newcommand{\Argument}{\mathrm{Arg}}\)
\( \newcommand{\norm}[1]{\| #1 \|}\)
\( \newcommand{\Span}{\mathrm{span}}\) \( \newcommand{\AA}{\unicode[.8,0]{x212B}}\)
\( \newcommand{\vectorA}[1]{\vec{#1}} % arrow\)
\( \newcommand{\vectorAt}[1]{\vec{\text{#1}}} % arrow\)
\( \newcommand{\vectorB}[1]{\overset { \scriptstyle \rightharpoonup} {\mathbf{#1}} } \)
\( \newcommand{\vectorC}[1]{\textbf{#1}} \)
\( \newcommand{\vectorD}[1]{\overrightarrow{#1}} \)
\( \newcommand{\vectorDt}[1]{\overrightarrow{\text{#1}}} \)
\( \newcommand{\vectE}[1]{\overset{-\!-\!\rightharpoonup}{\vphantom{a}\smash{\mathbf {#1}}}} \)
Learning Objectives
By the end of this section, you will be able to:
- Discuss the importance of case selection in case studies.
- Consider the implications of poor case selection.
Introduction
Case selection is an important part of any research design. Deciding how many cases, and which cases to include, clearly help determine the outcome of our results. Large-N research is when the number of observations or cases is large enough that we would need mathematical, usually statistical, techniques to discover and interpret any correlations or causations. In order for a large-N analysis to yield any relevant findings, a number of conventions need to be observed.
- First, the sample needs to be representative of the studied population. Thus, if we wanted to understand the long-term effects of COVID, we would need to know the approximate details of those who contracted the virus. Once the parameters of the population are known, we can then determine a sample that represents the larger population. For example, women make up 55% of all long-term COVID survivors. Thus, any sample we generate needs to be at least 55% women.
- Second, some kind of randomization technique needs to be involved. In other words, there must be randomly selected people within the sample. Randomization would help to reduce bias in the study. Also, when cases (people with long-term COVID) are randomly chosen they need to ensure a fairer representation of the studied population.
- The sample needs to be large enough, hence the large-N designation, for any conclusions to have any external validity. Generally speaking, the larger the number of observations/cases in the sample, the more validity in the study. There is no magic number. However, the sample of long-term COVID patients should be at least over 750 people, with an aim of around 1,200 to 1,500 people.
When it comes to comparative politics, we rarely ever reach the numbers typically used in large-N research. There are approximately 195 fully recognized countries, a dozen partially recognized countries, and even fewer areas or regions of study, such as Europe or Latin America. Given this, what is the strategy when one case, or a few cases, is being studied? What happens if we are only wanting to know the COVID-19 response in the United States, and not the rest of the world? How do we randomize to ensure the results are not biased? These questions are legitimate issues that many comparativist scholars face when completing research.
Does randomization work with case studies? Gerring suggests that it does not, as “any given sample may be widely representative” (pg. 87). Thus, random sampling is not a reliable approach when it comes to case studies. And even if the randomized sample is representative, there is no guarantee that the gathered evidence would be reliable.
In large-N research, potential errors and/or biases may be ameliorated (make better or more tolerable), especially if the sample is large enough. Incorrect or biased inferences are less of a worry when we have 1,500 cases versus 15 cases. In small -N research, case selection simply matters much more.
According to Blatter and Haverland (2012), “case studies are ‘case-centered’, whereas large-N studies are ‘variable-centered’". In large-N studies, the concern is with conceptualization and operationalization of variables. So which data should be included in the analysis of long-term COVID patients? A survey might be an option, with appropriately constructed questions. Why? For almost all survey-based large-N research, the question responses become the coded variables used in the statistical analysis.
Case selection can be driven by a number of factors in comparative politics.
- First, it can derive from the interests of the researcher(s). For example, if the researcher lives in Germany, they may want to research the spread of COVID-19 within the country, possibly using a subnational approach comparing infection rates among German states.
- Second, case selection may be driven by area studies. Researchers may pick areas of study due to their personal interests. For example, an European researcher may study COVID-19 infection rates among European Union member-states.
- Compare their similarities or their differences.
- Compare the typical or atypical (deviate from the norm).
Types of Case Studies: Descriptive vs. Causal
John Gerring (2017) suggests that the central question posed by the researcher dictates the aim of the case study. Is the study meant to be descriptive? If so, what is the researcher looking to describe? How many cases (countries, incidents, events) are there? Or is the study meant to be causal, where the researcher is looking for a cause and effect? Given this, Gerring categorizes case studies into two types: descriptive and causal.
Descriptive case studies are “not organized around a central, overarching causal hypothesis or theory” (pg. 56). Researchers simply seek to describe what they observe. They are useful for transmitting information regarding the studied political phenomenon. For a descriptive case study, a scholar might choose a case that is considered typical of the population, such as the effects of the pandemic on medium-sized cities in the US. This city would have to exhibit the tendencies of medium-sized cities throughout the entire country.
First, we would have to conceptualize what we mean by ‘a medium-size city’.
Second, we would then have to establish the characteristics of medium-sized US cities, so that our case selection is appropriate. Alternatively, cases could be chosen for their diversity . In keeping with our example, maybe we want to look at the effects of the pandemic on a range of US cities, from small, rural towns, to medium-sized suburban cities to large-sized urban areas.
Causal case studies are “organized around a central hypothesis about how X affects Y” (pg. 63). The context around a specific political phenomenon allows for researchers to identify the aspects that set up the conditions, and the mechanisms for that outcome to occur. Scholars refer to this as the causal mechanism . Remember, causality is when a change in one variable verifiably causes an effect or change in another variable. Thus, Gerring divides the mechanisms into three categories. The differences revolve around how the central hypothesis is utilized in the study.
- Exploratory case studies are used to identify a potential causal hypothesis. Researchers will single out the independent variables that seem to affect the outcome, or dependent variable. Context is more about hypothesis generating as opposed to hypothesis testing. Case selection can vary widely depending on the goal of the researcher. For example, if the scholar is looking to develop an ‘ideal-type’, they might seek out an extreme case. Thus, if we want to understand the ideal-type capitalist system, we would investigate a country that practices a pure or ‘extreme’ form of the economic system.
- Estimating case studies start with a hypothesis already in place. The goal is to test the hypothesis through collected data/evidence. Researchers seek to estimate the ‘causal effect’. In other words, is the relationship between the independent and dependent variables positive, negative, or none existent.
- Diagnostic case studies help to “confirm, disconfirm, or refine a hypothesis” (Gerring 2017). Case selection can vary. For example, scholars can choose a least-likely case, or a case where the hypothesis is confirmed even though the context would suggest otherwise. A good example would be looking at Indian democracy, which has existed for over 70 years. India has a high level of ethnolinguistic diversity, is relatively underdeveloped economically, and has a low level of modernization through large swaths of the country. All of these factors strongly suggest that India should not have democratized, should have failed to stay a democracy in the long-term, or have disintegrated as a country.
Most Similar/Most Different Systems Approach
Single case studies are valuable as they provide an opportunity for in-depth research on a topic that requires it. However, in comparative politics, our approach is to compare. Given this, we are required to select more than one case. Challenges quickly emerge. First, how many cases do we pick? Second, how do we apply the case selection techniques, descriptive vs. causal? Do we pick two extreme cases if using an exploratory approach, or two least-likely cases if choosing a diagnostic case approach?
English scholar John Stuart Mill developed several approaches to comparison with the explicit goal of isolating a cause within a complex environment. Two of these methods, the 'method of agreement' and the 'method of difference' have influenced comparative politics.
- In the 'method of agreement', two or more cases are compared for their commonalities. The scholar looks to isolate the common characteristic, or variable, which is then established as the cause for their similarities.
- In the 'method of difference', two or more cases are compared for their differences. The scholar looks to isolate the characteristic, or variable, that the cases do not have in common.
From these two methods, comparativists have developed two approaches.
What Is the Most Similar Systems Design (MSSD)?
Derived from Mill’s ‘method of difference’, the Most Similar Systems Design Design (MSSD) compares cases but the outcomes differ in result. In this approach, an attempt is made to keep as many of the variables the same across the selected cases. Remember, the independent variable (cause) is the factor that doesn’t depend on changes in other variables. The dependent variable (effect) is affected by, or dependent on, the independent variable. In a most similar systems approach, the variables of interest should remain the same.
There is no national healthcare system in the United States. Meanwhile, New Zealand, Australia, Ireland, UK, and Canada have robust, publicly accessible national health systems. All these countries have similar systems: English heritage and language use, liberal market economies, strong democratic institutions, and high levels of wealth and education. Yet, despite these similarities, the end results vary. The US does not look like its peer countries.
Just for fun! Try your hand at some cause-and-effect scenarios:
Source: Upperelementary Snapshots
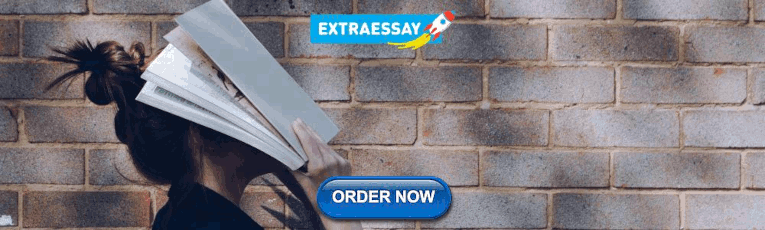
What Is the Most Different Systems Design (MDSD)?
In a Most Different System Design, the cases selected are different from each other, but result in the same outcome. Thus, the dependent variable is the same. Different independent variables exist between the cases, such as democratic v. authoritarian regime, liberal market economy v. non-liberal market economy. Or it could include other variables such as societal homogeneity (uniformity) vs. societal heterogeneity (diversity), where a country may find itself unified ethnically/religiously/racially, or fragmented along those same lines.
An example would be countries that are classified as economically liberal. The Heritage Foundation lists countries Singapore, Taiwan, Estonia, Australia, New Zealand, Switzerland, Chile, and Malaysia as either free or mostly free. Yet, these countries differ greatly from one another. Singapore and Malaysia are considered flawed or illiberal democracies (see chapter 5 for more discussion), whereas Estonia is still classified as a developing country. Australia and New Zealand are wealthy, Malaysia is not. Chile and Taiwan became economically free countries under authoritarian military regimes, which is not the case for Switzerland. In other words, why do we have different systems producing the same outcome?
Comparative Designs
- First Online: 18 January 2019
Cite this chapter
- Oddbjørn Bukve 2
965 Accesses
A comparative design involves studying variation by comparing a limited number of cases without using statistical probability analyses. Such designs are particularly useful for knowledge development when we lack the conditions for control through variable-centred, quasi-experimental designs. Comparative designs often combine different research strategies by using one strategy to analyse properties of a single case and another strategy for comparing cases. A common combination is the use of a type of case design to analyse within the cases, and a variable-centred design to compare cases. Case-oriented approaches can also be used for analysis both within and between cases. Typologies and typological theories play an important role in such a design. In this chapter I discuss the two types separately.
This is a preview of subscription content, log in via an institution to check access.
Access this chapter
- Available as EPUB and PDF
- Read on any device
- Instant download
- Own it forever
- Durable hardcover edition
- Dispatched in 3 to 5 business days
- Free shipping worldwide - see info
Tax calculation will be finalised at checkout
Purchases are for personal use only
Institutional subscriptions
Later Ragin has developed the method to make it possible to use continuous variables and a probability logic, so-called fuzzy-set logic (Ragin, 2000 ).
Boolean algebra describes logical relations in a similar way that ordinary algebra describes numeric relations.
Bukve, O. (2001). Lokale utviklingsnettverk ein komparativ analyse av næringsutvikling i åtte kommunar. Høgskulen i Sogn og Fjordane, Sogndal.
Google Scholar
Collier, R. B., & Collier, D. (1991). Shaping the political arena: Critical junctures, the labor movement, and regime dynamics in Latin America . Princeton, NJ: Princeton University Press.
Dion, D. (1998). Evidence and inference in the comparative case study. (Case studies in politics). Comparative Politics, 30 , 127.
Article Google Scholar
George, A. L., & Bennett, A. (2005). Case studies and theory development in the social sciences . Cambridge, MA: MIT Press.
Goggin, M. L. (1986). The “too few cases/too many variables” problem in implementation research. The Western Political Quarterly, 39 , 328–347.
Landman, T. (2008). Issues and methods in comparative politics: An introduction (3rd ed.). Milton Park, Abingdon, Oxon: Routledge.
Book Google Scholar
Lange, M. (2013). Comparative-historical methods . Los Angeles: Sage.
Luebbert, G. M. (1991). Liberalism, fascism, or social democracy: Social classes and the political origins of regimes in interwar Europe . New York: Oxford University Press.
Matland, R. E. (1995). Synthesizing the implementation literature: The ambiguity-conflict model of policy implementation. Journal of Public Administration Research and Theory: J-PART, 5 (2), 145–174.
Paige, J. (1975). Agrarian revolution: Social movements and export agriculture in the underdeveloped world . New York: Free Press.
Przeworski, A., & Teune, H. (1970). The logic of comparative social inquiry . New York: Wiley.
Ragin, C. C. (1987). The comparative method . Berkeley, CA: University of California Press.
Ragin, C. C. (2000). Fuzzy-set social science . Chicago, IL: University of Chicago Press.
Ragin, C. C., & Amoroso, L. M. (2011). Constructing social research . Thousand Oaks, CA: Pine Forge Press.
Skocpol, T. (1979). States and social revolutions: A comparative analysis of France, Russia, and China . Cambridge: Cambridge University Press.
Weber, M. (1971). Makt og byråkrati: essays om politikk og klasse, samfunnsforskning og verdier . Oslo, Norway: Gyldendal.
Wickham-Crowley, T. P. (1992). Guerrillas and revolution in Latin America: A comparative study of insurgents and regimes since 1956 . Princeton, NJ: Princeton University Press.
Download references
Author information
Authors and affiliations.
Western Norway University of Applied Sciences, Sogndal, Norway
Oddbjørn Bukve
You can also search for this author in PubMed Google Scholar
Rights and permissions
Reprints and permissions
Copyright information
© 2019 The Author(s)
About this chapter
Bukve, O. (2019). Comparative Designs. In: Designing Social Science Research. Palgrave Macmillan, Cham. https://doi.org/10.1007/978-3-030-03979-0_9
Download citation
DOI : https://doi.org/10.1007/978-3-030-03979-0_9
Published : 18 January 2019
Publisher Name : Palgrave Macmillan, Cham
Print ISBN : 978-3-030-03978-3
Online ISBN : 978-3-030-03979-0
eBook Packages : Political Science and International Studies Political Science and International Studies (R0)
Share this chapter
Anyone you share the following link with will be able to read this content:
Sorry, a shareable link is not currently available for this article.
Provided by the Springer Nature SharedIt content-sharing initiative
- Publish with us
Policies and ethics
- Find a journal
- Track your research
(Stanford users can avoid this Captcha by logging in.)
- Send to text email RefWorks EndNote printer
Configurational comparative methods : qualitative comparative analysis (QCA) and related techniques
Available online.
- Sage Research Methods
At the library

Green Library
More options.
- Find it at other libraries via WorldCat
- Contributors
Description
Creators/contributors, contents/summary.
- INTRODUCTION - Benoit Rihoux and Charles Ragin
- 1. Qualitative Comparative Analysis (QCA) as an Approach - Dirk Berg-Schlosser, Gisele De Meur, Benoit Rihoux & Charles Ragin
- 2. Comparative Research Design: Case and Variable Selection - Dirk Berg-Schlosser & Gisele De Meur
- 3. Crisp-Set Qualitative Comparative Analysis (CSQCA) - Benoit Rihoux & Gisele De Meur
- 4. Multi-Value QCA (MVQCA) - Lasse Cronqvist & Dirk Berg-Schlosser
- 5. Qualitative Comparative Analysis Using Fuzzy Sets (FSQCA) - Charles C. Ragin
- 6. A Commented Review of Applications - Sakura Yamasaki & Benoit Rihoux
- 7. Addressing the Critiques of QCA - Gisele De Meur, Benoit Rihoux & Sakura Yamasaki
- 8. Conclusions - The Way(s) Ahead - Benoit Rihoux, Charles Ragin and Sakura Yamasaki FURTHER RESOURCES FOR CONFIGURATIONAL COMPARATIVE METHODS - Damien Bol and Sakura Yamasaki GLOSSARY - Sakura Yamasaki, Benoit Rihoux, Gisele De Meur and Charles C. Ragin THEMATIC AND AUTHOR INDEX ABOUT THE AUTHORS A Commented Review of Applications - Sakura Yamasaki, Benoit Rihoux Addressing the Critiques of QCA - Gisele De Meur, Benoit Rihoux, Sakura Yamasaki Comparative Research Design: Case and Variable Selection - Dirk Berg-Schlosser, Gisele De Meur Conclusions - The Way(s) Ahead - Benoit Rihoux, Charles Ragin, Sakura Yamasaki, Damien Bol Crisp-Set Qualitative Comparative Analysis - Benoit Rihoux, Gisele De Meur Multi-Value QCA (mvQCA) - Lasse Cronqvist, Dirk Berg-Schlosser Qualitative Comparative Analysis (QCA) as an Approach - Dirk Berg-Schlosser, Gisele De Meur, Benoit Rihoux, Charles Ragin.
- (source: Nielsen Book Data)
Bibliographic information
Browse related items.

- Stanford Home
- Maps & Directions
- Search Stanford
- Emergency Info
- Terms of Use
- Non-Discrimination
- Accessibility
© Stanford University , Stanford , California 94305 .
Comparative Research Design: Case and Variable Selection
D Berg-Schlosser , GD Meur
展开
This chapter discusses the research design and general instrument characteristics of the present study. The sample selection process as well as general characteristics of the firms of the final sample are also introduced.

通过 文献互助 平台发起求助,成功后即可免费获取论文全文。
我们已与文献出版商建立了直接购买合作。
你可以通过身份认证进行实名认证,认证成功后本次下载的费用将由您所在的图书馆支付
您可以直接购买此文献,1~5分钟即可下载全文,部分资源由于网络原因可能需要更长时间,请您耐心等待哦~
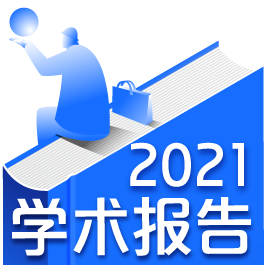
百度学术集成海量学术资源,融合人工智能、深度学习、大数据分析等技术,为科研工作者提供全面快捷的学术服务。在这里我们保持学习的态度,不忘初心,砥砺前行。 了解更多>>
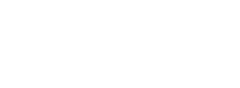
©2024 Baidu 百度学术声明 使用百度前必读
- Dissertations
- Advanced Search
- 【Created on October 31, 2023】 Integration of CiNii Dissertations and CiNii Books into CiNii Research
- Impact of the Release of the New "NDL Search" on CiNii Services
Comparative Research Design: Case and Variable Selection
- Dirk Berg-Schlosser
- Gisèle De Meur
Configurational Comparative Methods: Qualitative Comparative Analysis (QCA) and Related Techniques 19-32, 2009
SAGE Publications, Inc.
Citations (1) *help
Details 詳細情報について.
- CRID 1360579818526612480
- DOI 10.4135/9781452226569.n2
- Web Site http://journals.sagepub.com/doi/pdf/10.4135/9781452226569.n2
- Export to RefWorks
- Export to EndNote
- Export to Mendeley
- Export as RDF
- Show Refer/BibIX
- Show BibTeX
- Show JSON-LD
Report a problem
- Edit article detail
Project list
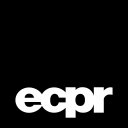
Install the app
Install this application on your home screen for quick and easy access when you’re on the go.
Just tap Share then “Add to Home Screen”
You are currently browsing offline
Discover ECPR's Latest Methods Course Offerings
I agree to receive your newsletters and accept the data privacy statement.
We use Brevo as our email marketing platform. By clicking below to submit this form, you acknowledge that the information you provided will be transferred to Brevo for processing in accordance with their terms of use .
Comparative Research Designs
Member rate £492.50 Non-Member rate £985.00
Save £45 Loyalty discount applied automatically* Save 5% on each additional course booked
*If you attended our Methods School in July/August 2023 or February 2024.
Course Dates and Times
Monday 17 – Friday 21 February 2019, 14:00 – 17:30 (finishing slightly earlier on Friday) 15 hours over five days

Institution: Université catholique de Louvain
- Need to Know
This course teaches you how to conceive and conduct the most appropriate comparative research design, broadly defined as any research enterprise that comprises at least two ‘cases’ or units of analysis. It answers fundamental questions, including:
- What is comparison?
- Why compare; what is the value of comparison?
- What should be the mindset of a good comparative researcher?
- What is the link between a research puzzle and the choice for a comparative research design – and what would the alternative(s) be?
- At which level(s) should ‘cases’ be envisaged?
We will examine the practicalities of different types of comparative research, following these hands-on steps:
- prior arbitrations and ‘casing’, i.e. the definition of cases;
- case selection, through basic or advanced strategies;
- collecting and managing comparative data;
- comparative data analysis (qualitative, QCA and quantitative options).
The course alternates between lectures and interactive sessions, giving ample time for questions, open discussions, and solution-finding for your individual projects.
By the end of this course, you will know how to conceive and conduct the most appropriate comparative research design – the latter broadly defined as any research enterprise that comprises at least two ‘cases’ or units of analysis.
Tasks for ECTS Credits
2 credits (pass/fail grade):
- Complete the short pre-course survey
- Read the daily texts (see reading list) in advance of each class
- Attend at least 90% of course hours
- Deliver the four daily assignments from Monday to Thursday (to be delivered by noon the next day, Tuesday to Friday)
4 credits As above, plus write up a take-home paper to be evaluated by the teaching team. The format, focus, evaluation criteria, submission deadline etc. will be explained on Days 1 and 5. There is some flexibility in terms of focus (more details on Day 5), with – among other possibilities – a paper laying out out all the main elements of one’s CRD, or a paper focusing on one specific step of one‘s CRD.
Instructor Bio
Benoît Rihoux is a full professor of political science whose research interests include political parties, new social movements, organisational studies, political change, and policy processes.
He is manager of the COMPASSS international research group on comparative methods, in the development and refinement of which he plays a leading role, bringing together scholars from Europe, North America and Japan in particular.
Benoît is a convenor of international methods initiatives more generally, and has published Innovative Comparative Methods for Policy Analysis: Beyond the Quantitative-Qualitative Divide (Springer/Kluwer, ed. with Heike Grimm 2006) and Configurational Comparative Methods: Qualitative Comparative Analysis (QCA) and Related Techniques (Sage, ed. with Charles Ragin 2009).
He has published extensively on systematic comparative methods (QCA in particular) and their applications in diverse fields – especially policy- and management-related – with interdisciplinary teams.
This course will teach you to how conceive and conduct the most appropriate comparative research design – broadly defined as any research enterprise that comprises at least two ‘cases’ or observations. It will cover fundamental questions upstream of practical and hands-on choices:
- Why compare; what is the added value of comparison?
- What are the logical underpinnings and mental operations behind comparison?
- What should be the mindset of a good comparative researcher?
- What should be his/her goals?
- What is the link between a research puzzle and the choice for a comparative research design?
- What would be the alternative(s)?
- Does one conceive and does one perform comparison in the same way when the ‘cases’ are situated at the micro (i.e. individuals), meso (e.g. organisations) or macro (e.g. political or policy systems) levels?
We will examine in detail the practicalities of different types of comparative research designs, by following all the hands-on steps:
- prior arbitrations and ‘casing’, i.e. the definition of the cases
- case selection, through more basic or more advanced strategies
- collecting and managing comparative data
Steps 1 and 2 will be examined in greater detail. Each session allows time for open discussions and interaction.
Day 1 After introducing the practical and organisational aspects of the course, we will frame comparative research in the broader context of a comparative approach . This means considering some epistemological issues underpinning comparison. Starting from the discussion of comparison as a basic mental operation, we will progress to comparison in the social sciences, then to political science specifically. One core focus will be on the different goals of comparison, with practical examples. To conclude, we will discuss a first series of participants’ projects, focusing on the goals pursued (why go for a comparative research design?).
Day 2 We locate comparative research designs within the whole range of possible designs. We present the practical steps of a good comparative research design, focusing on the major arbitrations. We also have a first look at Step 1 operations that lie upstream of case selection, such as the formulation of the research question(s) and hypotheses, the correct use of concepts for the purpose of comparison, the number of cases one will be able to manage, and the choice between cross-country or within-country case selection. We conclude by discussing a second series of participants’ projects, with a focus on upstream arbitrations.
Day 3 We continue examining Step 1 operations, and deepen the question of 'what is a case?' within a comparative research design – with an emphasis on core arbitrations such as depth vs breadth and cross-country vs within-country vs within-system casing and case selection. Then we’ll systematically survey all the main options for the core Step 2 operation: case selection. We first envisage rather basic or simple strategies of case selection, from very small-N to very large-N, and following different criteria; the pros and cons of each strategy will also be discussed. We conclude by discussing a second series of participants’ projects, with a focus on casing and case selection
Day 4 We turn to more refined strategies, in particular considering time/sequence and multilevel phenomena, and discussing the pros and cons of each. Then we look at hands-on tricks of the trade for collecting and managing data in a comparative research (Step 3) – including ways to troubleshoot and to make case selection adjustments as your research develops. A fourth interactive section around participants’ projects will focus on case selection and data collection/management.
We examine different ways to engage in comparative data analysis, envisaging three main families of options:
- case-oriented (or qualitative) analyses
- Qualitative Comparative Analysis (QCA) for systematic cross-case comparison
- statistical/quantitative analyses.
We will examine the pros and cons of each, as well as the potential difficulties of sequencing different data analysis techniques in a mixed- or multi-method design. In particular, we'll discuss the potential of sequencing QCA with single case studies, in small- or intermediate-N designs. In the second part of the morning session, we revisit some core points – with a focus on the strengths of comparative research designs, but even more on main perils or caveats of comparison. You will become more aware of ways to mis-compare – and avoid it in your own research.
Finally, in an open interactive session, we discuss points still to be clarified, points of debate or disagreements, remaining questions and answers about participants’ projects, etc.
You are encouraged to bring your own research questions and hypotheses, first thoughts and difficulties (if any) in case definition and case selection, and (if applicable) any data you have already compiled. The course is designed to help you make the most appropriate choices in comparative research design. You will be able to reflect and to work on your own project as we follow the sequence of fundamental and then applied steps. Whenever possible, we’ll use input from participants’ own projects during the interactive parts of each one of the five sessions.
Connections with other courses (see also 'Courses before' and 'Courses after', below):
This course can be taken as a standalone course, but it has been designed as an introductory course, particularly for Summer School courses – in particular Methodologies of Case Studies , QCA and Fuzzy Sets and Mixed Methods Designs (exact course titles may change).
This is not a specialist QCA course. Some main features of QCA (as an approach & set of techniques) will be presented at introductory level, but if you want hands-on QCA training, follow Eva Thomann's week-long Introduction to QCA course, or the two-week QCA course at the Summer School.
The course may also be of interest for scholars engaged in ‘thick’ observational work (e.g. ethnography, participant observation, interviews) or in in-depth single case studies (using e.g. process tracing), as well as those interested in formalised or statistical approaches (large-N statistical techniques, experiments), especially if their populations and/or samples are not so obvious to circumscribe.
Little specific knowledge is expected. Prior training in qualitative and/or quantitative methods is of course an asset, but by no means a requirement.
You should simply be willing to reflect openly about your research design – there is no best or one-size-fits-all approach.
Software Requirements
No particular software will be used intensively throughout the course, apart from the usual suites (such as MS Office).
We will discuss the strengths and limitations of different software to compile, store and manage numerical and non-numerical data about a certain number of cases (from small-N to larger-N situations) – primarily Excel, Access, SPSS & NVivo, but these software packages will not be used hands-on in the lab.
Hardware Requirements
Please bring your own laptop. No specific technical requirements.
Further readings (recommended – not compulsory. A full, more extensive list of other related readings will be made available during the course):
Bartolini, S. (1993) On time and comparative research Journal of Theoretical Politics 5(2) : 131–167
Becker, H.S. (1998) Tricks of the trade: how to think about your research while you're doing it Chicago: University of Chicago Press
Brady, H. and Collier, D. (2010) Rethinking social inquiry: Diverse tools, shared standards New York: Rowman & Littlefield Publishers
Byrne, D. and Ragin, C. (2009) The Sage handbook of case-based methods London: Sage
Della Porta, D. and Keating, M. (2008) Approaches and methodologies in the social sciences: A pluralist perspective Cambridge: Cambridge University Press
George, A.L. and Bennett, A. (2005) Case studies and theory development in the social sciences Cambridge, MA: MIT Press
Gerring, J. (2007) Case study research: principles and practices Cambridge: Cambridge University Press
Mahoney, J. and Rueschemeyer, D. (2003) Comparative historical analysis in the social science s Cambridge: Cambridge University Press
Przeworski, A. and Teune, H. (1970) The logic of comparative social inquiry New York: Wiley-Interscience
Ragin, C.C. and Becker, H.S. (1992) What is a case? Exploring the foundations of social inquiry Cambridge: Cambridge University Press
Teune, H. (1990) Comparing countries: lessons learned In: Oyen, E., (Ed.) Comparative methodology: theory and practice in international social research , pp. 38–62 London: Sage.
Recommended Courses to Cover Before this One
Winter School
Foundations of set-theoretic and case-oriented thinking and methodology
Philosophy and Methodology of the Social Sciences: A Pluralistic Framework
Tools for the analysis of complex social system: an introduction
Automated web data collection with R
Introduction to Qualitative Data Analysis with Atlas.ti
Introduction to NVivo for Qualitative Data Analysis
Advanced Multi-Method Research
Introduction to MAXQDA, a Qualitative and Mixed Methods Data Analysis Software
Process tracing (introductory or advanced)
Summer School
Recommended Courses to Cover After this One
Methodologies of Case Studies
QCA and Fuzzy Sets
Mixed Methods Designs
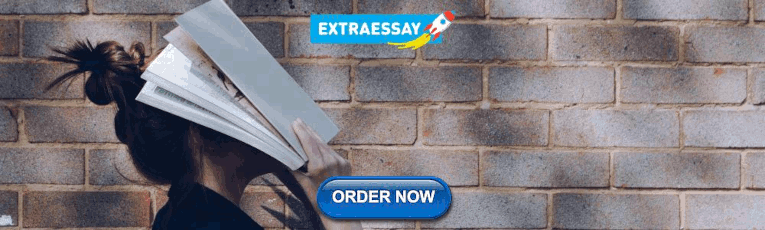
IMAGES
VIDEO
COMMENTS
In book: Configurational comparative methods: Qualitative comparative analysis (QCA) and related techniques (pp.19-32) Publisher: Sage. Editors: B. Rihoux, Charles C. Ragin. Authors: Dirk Berg ...
Comparative Research Designs and Case Selection Each empirical field of study can be described by the cases ("units") analysed, the characteristics of cases ("variables") being considered and the number of times each unit is observed ("observations"). In macro-qualitative small-N situations, which is the domain we are concerned
The potential of consecutive qualitative comparative analysis as a systematic strategy for configurational theorizing. Qualitative comparative analysis is gradually becoming more established in the evaluation field. The purpose of this article is to highlight the potential for evaluation research of engaging in….
A second conceptual challenge that researchers must resolve is the selection of variables on which to match. As with model specification in a ... the Case Selector can help facilitate more careful case selection and ideally can help to improve the usefulness of the comparative case study research design as a tool in the social scientist's ...
Abstract. Each empirical field of study can be described by the cases ("units") analysed, the characteristics of cases ("variables") being considered and the number of times each unit is observed ("observations"). In macro-qualitative small-N situations, which is the domain we are concerned with here, both case selection and ...
Case-selection and qualitative comparisons. Methodological advice on the selection of cases in qualitative research stands in a long tradition. John Stuart Mill in his A System of Logic, first published in 1843, proposed five methods meant to enable researchers to make causal inferences: the method of agreement, the method of difference, the double method of agreement and difference, the ...
Configurational Comparative Methods paves the way for an innovative approach to empirical scientific work through a strategy that integrates key strengths of both qualitative (case-oriented) and quantitative (variable-oriented) approaches. This first-of-its-kind text is ideally suited for "small-N" or "intermediate-N" research situations, which ...
Comparative Research Designs and Case Selection. January 2012. DOI: 10.1057/9781137283375_3. In book: Mixed Methods in Comparative Politics (pp.32-40) Authors: Dirk Berg-Schlosser. Philipps ...
Introduction. Case selection is an important part of any research design. Deciding how many cases, and which cases, to include, will clearly help determine the outcome of our results. If we decide to select a high number of cases, we often say that we are conducting large-N research.
Recent years have witnessed a host of innovations for conducting research with qualitative comparative analysis (QCA). Concurrently, important issues surrounding its uses have been highlighted. ... "Comparative Research Design: Case and Variable Selection." Pp. 19-32 in Configurational Comparative Methods. Qualitative Comparative Analysis ...
Incorrect or biased inferences are less of a worry when we have 1,500 cases versus 15 cases. In small -N research, case selection simply matters much more. According to Blatter and Haverland (2012), "case studies are 'case-centered', whereas large-N studies are 'variable-centered'". In large-N studies, the concern is with ...
This brings causal inference in qualitative compara-tive research back on the agenda since comparative case studies can identify counterfac-tual treatment effects. We argue that the validity of causal inferences from the comparative study of cases depends on the employed case-selection algorithm. We employ Monte Carlo techniques to demonstrate ...
Paired comparisons have long been a staple of research in political science. Comparative politics, in particular, is commonly defined by the comparative method, one approach to paired comparison (see Lijphart Reference Lijphart 1971, 682; 1975, 163; Slater and Ziblatt Reference Slater and Ziblatt 2013).Despite their widespread use, however, we lack a "theory of practice" (Tarrow Reference ...
A comparative design involves studying variation by comparing a limited number of cases without using statistical probability analyses. Such designs are particularly useful for knowledge development when we lack the conditions for control through variable-centred, quasi-experimentaldesigns. Comparative designs often combine different research ...
Selection of cases that take on similar values of confounding variables, but different values of a key independent variable. Confounds are "holds constant" because they take on the same values in all of the cases. This is the design recommended by King, Keohane, and Verba.
The Case Selector is primarily a tool for comparative (most similar and most different) designs. The data generated through this tool is not structured to facilitate other types of case study designs. To select crucial cases, extreme cases, or typical cases the techniques outlined by Gerring (2001) may be more useful.
Comparative research design: Case and variable selection. In Rihoux, B. & Ragin, C. C. (eds.), Configurational comparative methods. Qualitative comparative analysis (QCA) and related techniques (pp. 19-32). Configurational comparative methods. Qualitative comparative analysis (QCA) and related techniques. ... Journal of Comparative Policy ...
Comparative Research Design: Case and Variable Selection - Dirk Berg-Schlosser & Gisele De Meur; 3. Crisp-Set Qualitative Comparative Analysis (CSQCA) - Benoit Rihoux & Gisele De Meur ... Benoit Rihoux and Charles C. Ragin, along with their contributing authors, offer both a basic, comparative research design overview and a technical and hands ...
Berg-Schlosser D., De Meur G. (2009) 'Comparative Research Design: Case and Variable Selection', in Rihoux B., Ragin C. (eds.) Configurational Comparative Methods. Qualitative Comparative Analysis (QCA) and Related Techniques. Thousand Oaks: Sage.
For case-study analysis, it is often the rareness of the value that makes a case valuable, not its positive or negative value (contrast Emigh 1997; Mahoney and Goertz 2004; Ragin 2000: 60; Ragin 2004: 126). Large-N Analysis. As we have said, extreme cases lie far from the mean of a variable. _.
382. 作者:. D Berg-Schlosser , GD Meur. 摘要:. This chapter discusses the research design and general instrument characteristics of the present study. The sample selection process as well as general characteristics of the firms of the final sample are also introduced. 出版时间:. 2009/01/01.
Comparative Research Design: Case and Variable Selection. DOI Web Site 1 Citations. Dirk Berg-Schlosser. ... Configurational Comparative Methods: Qualitative Comparative Analysis (QCA) and Related Techniques 19-32, 2009 SAGE Publications, Inc. ...
Hands-on comparative research design, step 2 Case selection - more advanced strategies (45 minutes) ... Comparative research design: case and variable selection In Rihoux, B. and Ragin, C.C., (Eds.) Configurational comparative methods: Qualitative Comparative Analysis (QCA) and related techniques, pp. 19-32